The evolution of machine learning (ML) in the physical design and verification of semiconductor packages
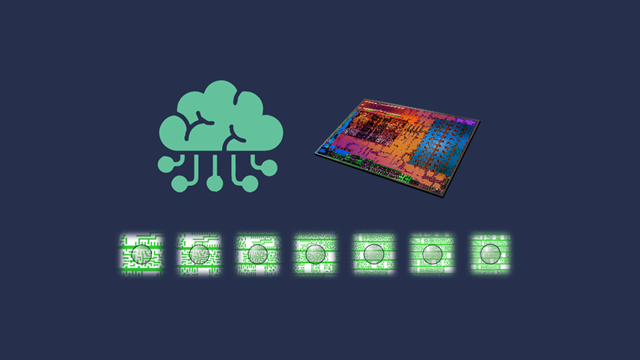
Innovation in physical verification has evolved in parallel with Moore’s Law since the fabless model emerged. From simple Boolean layer derivation and measurement checking, it progressed to more sophisticated DFM-era embedded equations and “recommended rules.” The next step introduced pattern matching and clustering to enable hot-spot checking. The latest evolution leverages ML techniques to address complex problems where the specification itself is not straightforward, integrating these capabilities across Siemens’ technology offerings.
How does ML leverage artificial intelligence?
Physical verification and EDA have long incorporated forms of AI, using rules and heuristics to capture human knowledge of manufacturing and layout analysis. Computational models and pattern matching are more elaborate ways of emulating this human expertise. ML is another approach to capturing and emulating human knowledge, with its own advantages and disadvantages depending on the specific application and challenges.
The choice of technique – traditional rules, patterns, or ML – depends on factors like how readily the problem can be defined, the available data, and the required precision. There is no expectation that existing methods will be entirely replaced by ML, as each approach has its own merits for different use cases in physical verification and EDA.
What key experiences does a designer deal with while doing layout verification?
Siemens employs a range of techniques to address various physical verification and EDA challenges, including:
- Computational and statistical modeling
- Pattern recognition
- ML
- Intelligent automation
- Cognitive computing
- Knowledge capture
The choice of technique depends on the specific problem, available data, and required precision. These methods work in tandem, with knowledge capture feeding back into the upstream processes to continuously improve the overall physical verification and EDA capabilities.
The goal is to leverage the strengths of each approach – from computational modeling to ML – to tackle the evolving challenges in layout analysis, manufacturing and reliability. This integrated, multi-pronged strategy allows Siemens to provide comprehensive solutions for the complex issues faced in modern semiconductor design and fabrication.
What is Siemens’ solution for IC packaging ML applications?
Siemens’ semiconductor package planning and prototyping solution utilizes the STCO (System Technology Co-Optimization) methodology, initially defined by IMEC. In this solution, ML techniques have demonstrated superior performance compared to traditional algorithms, particularly in terms of execution time and quality of results. One example is the process of assigning signals and nets to devices with millions of pins, as well as optimizing system-level and package connectivity to improve signal performance and routability.
With the vast number of signals, nets, and pins in modern designs, ML-based approaches can shorten the optimization process and achieve higher design quality, including shorter interconnects for lower power, better signal integrity, and improved routability and overall PPA. Siemens continues to explore other use cases where ML can provide significant benefits, though the details remain proprietary for now.
View the infographic
Explore this infographic to learn more about the evolution of ML in the physical design and verification of semiconductor packages at Siemens.
Learn more
Discover why semiconductor-enabled products and systems demand AI and ML in the physical design and verification of IC Packaging. Read blog.
To learn more about how Siemens 3D IC heterogeneous semiconductor packaging solutions catapult design teams into the future of IC design, visit our 3D IC Homepage
To learn about our semiconductor packaging design and verification solutions, Click here.
Bookmark our semiconductor packaging blog site to keep up to date on the latest news, resources, events and more.