The transformative impact of AI in CFD
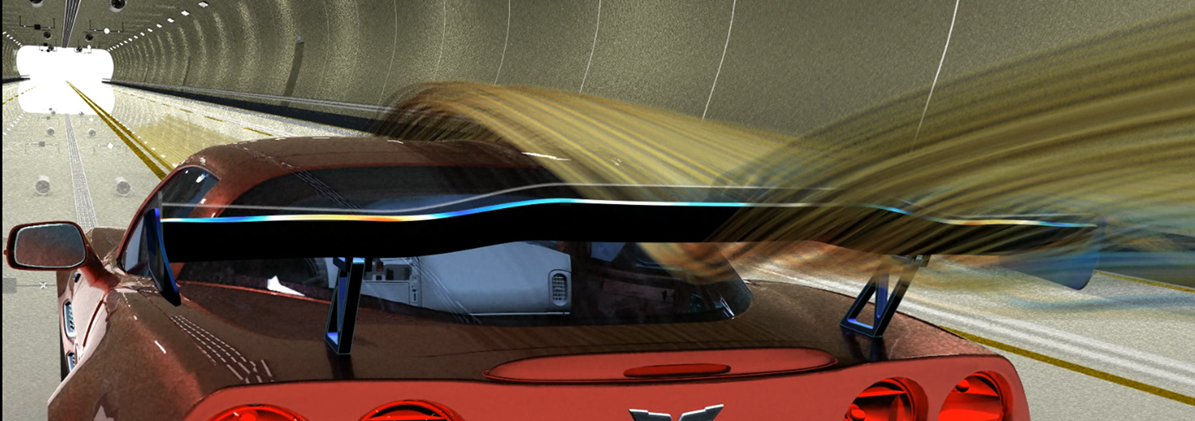
Artificial intelligence (AI) has become a ubiquitous force in the engineering world, radically transforming how companies operate and how employees work. As we move further into 2025, the influence of AI will only continue to grow, ushering in a new era of increased efficiency, productivity, and innovation. Nvidia CEO Jensen Huang has predicted recently that computing power driving advances in generative AI is expected to improve “a millionfold” over the next 10 years, driven by a “fourfold” increase in computing power annually.
Driving this rapid advancement is the exponential growth in computing power over the past decade. Today, the world’s leading supercomputers are capable of performing over 1 quintillion (1,000,000,000,000,000,000) calculations per second – a staggering increase from just a decade ago. This massive boost in raw computing power has enabled the training of ever-larger and more complex AI models, allowing them to tackle increasingly sophisticated tasks.
Powering this AI revolution are not just traditional CPUs, but also specialized hardware like graphics processing units (GPUs) and application-specific integrated circuits (ASICs) designed explicitly for machine learning workloads. These chips can perform the parallel processing required for training and running AI models much more efficiently than general-purpose CPUs.
In addition to advancements in hardware, the rise of cloud-based platforms, such as Simcenter X, has also played a pivotal role in democratizing access to immense AI computing power. Researchers and businesses can now rent GPU clusters and supercomputing resources on-demand from cloud providers, allowing them to train large-scale models without the need for massive upfront investments.
Optimizing business processes with AI
Simcenter Engineering Services has demonstrated that the influence of AI extends far beyond digital assistants and personal productivity (4 Myths about AI in CFD). Businesses are increasingly deploying AI-powered systems to optimize core operations and decision-making. AI algorithms can analyze massive amounts of data to uncover insights that inform everything from supply chain management to marketing strategies to financial forecasting. Engineering design decisions based on heat transfer and computational fluid dynamics (CFD) simulations are no exception. AI algorithms now support the entire simulation workflow chain, from data management and part shape recognition to real-time flow and thermal results predictions and optimization.
AI/ML in CFD
The synergy of CFD and machine learning promises to deliver faster solutions to well-known complex industrial fluid flow and heat transfer problems – most notably, design optimization of transient problems, such as vehicle aerodynamics and passenger cabin comfort.
Vehicle aerodynamics
Transient aerodynamic simulations, especially those involving complex geometries, require significant computational resources in terms of processing power and memory. Running these simulations at a large scale can be computationally intensive and time-consuming. Additionally, transient simulations often require very small time increments to capture dynamic air behavior accurately. This can lead to long simulation times, particularly for problems with a wide range of time scales. Additionally, the large amount of data generated by large-scale transient CFD simulations can be difficult to manage, store, and analyze.
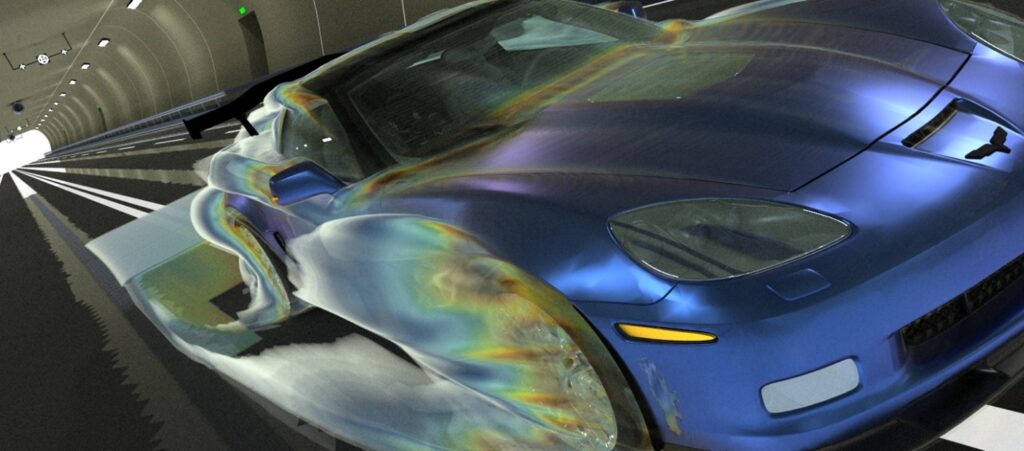
AI algorithms address this problem by providing inferred CFD results, including pressure distributions and air velocities, enabling the calculation of drag and lift coefficients across various design parameters. The AI models are trained on a set of simulation data, which consists of point clouds extracted from simulations at both the surface and volume levels, along with scalar values such as pressure, wall shear stress, and the three velocity components.
To develop accurate AI models, it is crucial to execute the training process efficiently. Simcenter Engineering Services addresses this need by employing a hybrid technique designed to cover the vast design space effectively within a set number of evaluations.
Initially, a collection of design points is established. These can either be provided by the user as seeds or generated through advanced techniques like Latin hypercube sampling methods. Once established, these initial points serve as inputs to an adaptive sampling study. This study is pivotal as it intelligently selects and populates additional design points aligned with user-defined objectives. Such objectives may include the exploration of regions where there are considerable shifts in response or areas where global and local gradient variations occur.
The AI model itself is principally trained using spatial data from the surface and volume mesh cells. In addition, it incorporates feature-defining scalars, such as surface normals, alongside the scalars intended for prediction by the AI model. This comprehensive approach ensures that the model can accurately predict complex engineering scalars spanning over varied design changes. The image below demonstrates an example of the training and inference stages of an AI model, showcasing its application in utilizing spatial coordinates, surface normals, and wall distance to predict engineering scalars like pressure and velocity vectors.
Training workflow

Inference workflow
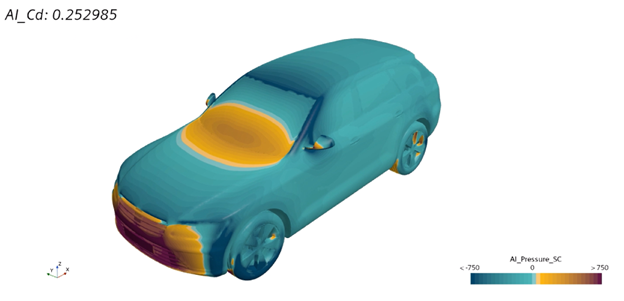
Passenger comfort
Running transient CFD simulations for a vehicle passenger comfort analysis is often necessary and comes with many unique challenges. The vehicle cabins are known to have intricate geometries with many small features, such as vents, seats, and other interior components that must be captured in detail using high-resolution meshes. For unsteady flow, this meshing requirement can be quite computationally demanding.
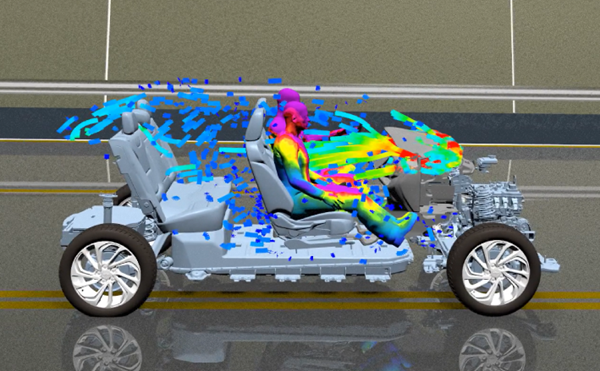
Additionally, the cabin passengers must be modeled in good detail, with separate output required for the manikin head, neck, torso, hands and feet. This level of refinement is necessary because human beings feel most comfortable within a relatively narrow range of ambient temperatures. Even small deviations outside this range can start to feel uncomfortable. Adding to the complexity is the fact that manikin surface temperatures can be influenced by very small deviations of the environment conditions, such as air flow, temperature, humidity, solar radiation, vehicle speed and acceleration.
Recognizing these challenges, Simcenter Engineering Services is actively exploring reduced order models and AI/ML-based solutions specifically tailored to address challenges in passenger comfort analysis. One area of focus involves the use of reduced order models (ROM), incorporating both static ROMs and proper orthogonal decomposition (POD) interpolation.
Static ROMs are used to predict key scalar metrics, like temperature or velocity, in a text-based format that provides essential quantitative insights. On the other hand, POD interpolation is employed to predict spatially varying 2D scalar fields. This approach enables the determination of key scalar metrics, such as cabin temperature and velocities, in two dimensions based on input parameters like solar loads, the sun’s relative position, and flow characteristics. Both reduced order models can be generated using simulation (and test) data for training.
The combination of these two methods provides a simplified yet effective means to analyze complex data, ensuring that critical passenger comfort parameters are accurately assessed and optimized. These and other types of reduced order models can be generated using Simcenter Reduced Order Modeling tool available in the Simcenter portfolio.
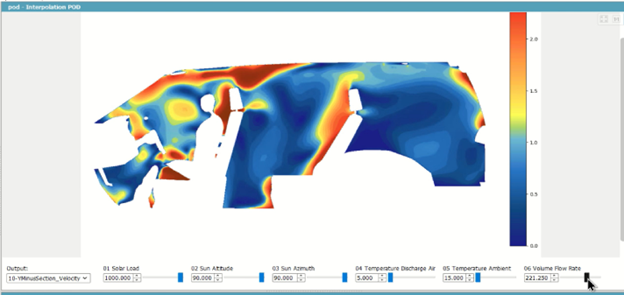
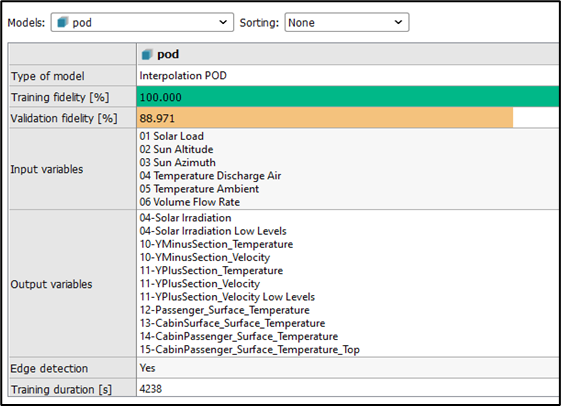
Complex 3D modeling
AI algorithms can enhance and accelerate the model-building phase of complex 3D thermal simulations. In vehicle thermal and energy management (VTM/VEM), accurate part-to-part contact through CAD imprinting is crucial to capturing heat conduction paths throughout the vehicle assembly. Achieving high-fidelity results often requires manual review and improvement of hundreds of poor-quality imprints, leading to extended project turnaround times.
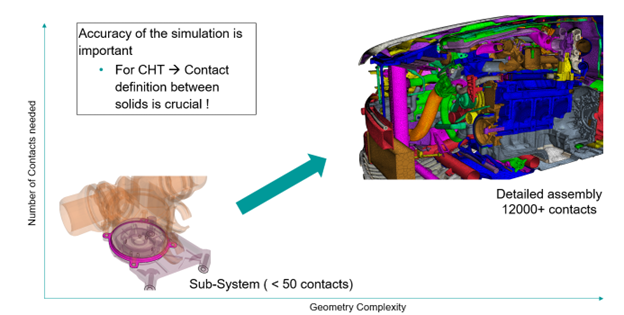
To improve efficiency and reduce turnaround times, Simcenter Engineering Services is developing custom automation and “smart logic” to support the CAD imprint and review process. This leverages AI/ML-based procedures within Simcenter STAR-CCM+. Various “modalities” can be employed for inference, as illustrated below.

Once the AI model is trained, contact validity can be inferred through a custom frontend plugin using the selected modality.

This approach integrates both tabular and image-based data training to derive inferences from the ML model, expediting and simplifying the modeling process.
For straightforward contact validity predictions, a simple classifier model using features from a text-based file is sufficient. However, when dealing with complex contact patches where additional context, such as the identity of contacting parts, is necessary, an image-based model offers greater advantages. This model can analyze visual data to assess the spatial and structural relationships between components, providing a more nuanced understanding of contact validity.
The inference pipeline for both tabular and image-based model are shown below:
Inference pipeline for tabular-based model

Inference pipeline for image-based model

This innovative approach not only speeds up the modeling process but also enhances the accuracy and reliability of thermal simulations, ultimately leading to improved vehicle design.
The path forward
There’s no doubt that AI is reshaping the engineering landscape in profound ways. Savvy leaders and innovators are embracing AI as a transformative opportunity, strategically deploying AI to augment and empower their workforce.
As an industry trailblazer Simcenter Engineering Services is leading by example, empowering our employees and our customers with AI-driven tools and capabilities, enabling the engineering community with the ability to unlock unprecedented levels of productivity, creativity, and business success.
Contact Sales or email us at engineeringservices.sisw@siemens.com for more information on Siemens Digital Industries Software products, solutions and services.