Whistle while you mesh: Simcenter STAR-CCM+ model-driven adaptive mesh refinement (AMR)
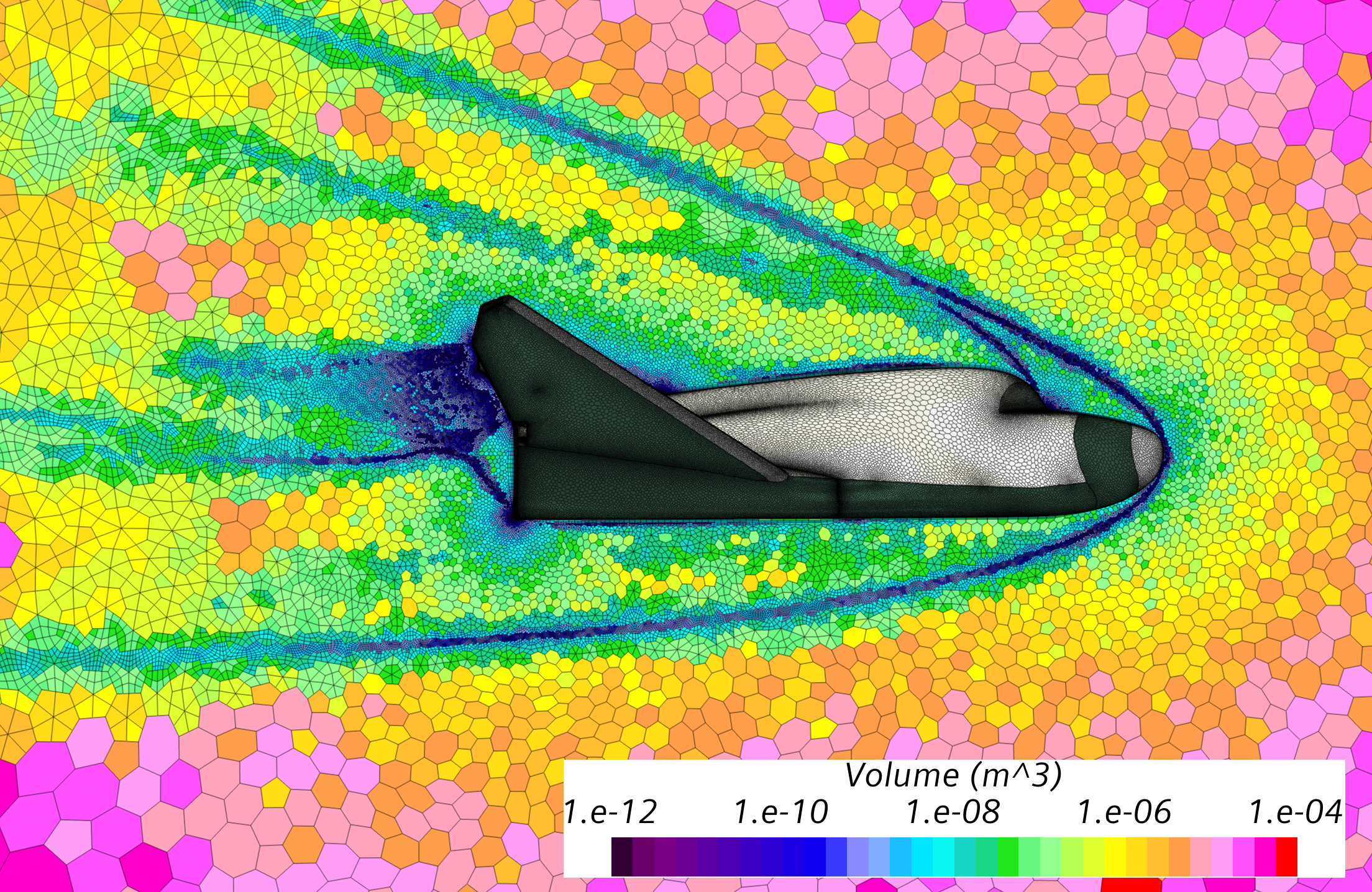
“That’s one small step for [a] man, one giant leap for mankind.”
Neil Armstrong
Neil Armstrong perfectly captured the wonder of the moment as he spoke those famous words while becoming the first human to set foot on the moon on July 20, 1969. Fifty years later, the Apollo 11 spaceflight is still considered an engineering marvel, and the Saturn V rocket that launched it is considered the most powerful launch vehicle to date. A recent visit to the Apollo 11 exhibit at the Kennedy Space Center gave me an appreciation for the extreme engineering and precision required to achieve the ambitious feat of landing humans on the moon and bringing them back home safely. I was astonished to find that the on-board guidance computer had only about 1/100,000 times the processing power of my smart phone [Link]. The phenomenal increase in computational power in the last several decades paved the way for high-fidelity computational fluid dynamic (CFD) simulations on complex geometries. I started pondering how the development of modern-day launch vehicles benefits from simulation-driven design to create superior designs at far reduced costs and increased operational safety.
The Grand Challenge
In the product development process, high-fidelity CFD simulation helps bridge a critical gap by providing detailed insight into the flow physics. However, the flow solution is only as good as the underlying computational mesh. As mentioned in CFD Vision 2030 Study: A Path to Revolutionary Computational Aerosciences [1], mesh generation and maintenance pose a significant bottleneck in the CFD workflow. In order to employ predictive CFD simulations early and often in the design phase, the following aspects of the mesh generation process need to be improved.
- Manual intervention: Manual mesh generation is a labor-intensive and iterative process.
Capturing the relevant flow features around complex geometries takes an iterative process of mesh generation and solution interrogation. Efficient mesh generation for a given application tends to be a craft learnt over time.
- Computational hurdle: Capturing the relevant flow features of varying length scales requires refinement zones that quickly escalate the cell count.
Not knowing the flow solution a-priori demands that the mesh be refined down to the smallest length scale of interest for increased accuracy. For unsteady flows and moving geometries, the restrictions on the cell size drastically increase the cell count.
The Solution – Adaptive Mesh Refinement (AMR)
Recognizing that mesh generation is only a means to an end, AMR aims to alleviate the aforementioned hurdles. AMR refines the computational mesh dynamically only in areas where needed i.e., to capture the relevant flow features while recovering coarser cells elsewhere. It is common to employ user-defined AMR criteria to capture strong gradients in the flow. However, the burden is left to the user to come up with suitable refinement criteria that reduce the discretization error in the mesh while avoiding over- or under-refining flow features.
With the 2020.1 release of Simcenter STAR-CCM+, users can put their mesh refinement worries behind, sit back, and whistle while they mesh, thanks to advanced model-driven AMR criteria!
Reduce manual intervention with model-driven AMR
While the idea of AMR is not new, Simcenter STAR-CCM+ takes it to another level by enabling advanced model-driven AMR that refines the mesh automatically with minimal user intervention until the final accuracy levels are met. For example, the free-surface driven AMR intelligently refines the cells ahead of the fluid and air interface. The overset-driven AMR automatically ensures that the cell sizes at the overset interface are compatible. These model-driven AMR criteria are often usable out of the box with no additional fine-tuning or exploratory runs. Simcenter STAR-CCM+ AMR works on polyhedral and trimmed meshes and can be run with both steady and transient simulations. Automatic re-partitioning is enabled to maintain good parallel performance. The benefits of model-driven AMR are:
- Enhanced ease of use
- Reduced engineer time
- Fewer cells and as a result reduced computational expense
- Same accuracy as a static “best-practice” tailored mesh
Finally, the next-gen AMR applicable on industrial geometries is here to stay! Several different refinement techniques are available as described below:
Model-based AMR for multiphase VOF
AMR driven by the multiphase Volume of Fluid (VOF) model allows the user to start with a coarse mesh and intelligently refines the cells ahead of the free-surface. The result is a sharp resolution of the VOF front with far fewer cells, while at the same time delivering a significant speed-up. This feature can be used along-side adaptive time-step to allow refinement in space and time. It is demonstrated by the fuel tank sloshing example below, which can be run 2.6x faster with maintained accuracy using AMR.
Model-based AMR for Overset meshes
For moving geometries employing overset interfaces, users will observe an improved ease of set-up of overset regions. Overset model-driven AMR automatically matches the cell sizes between the coarser background grid and the finer overset grids at the interface, removing cell size restrictions on the background grid. As a result, a significant reduction in engineering time and computational expense are observed. This is demonstrated by the Fridsma benchmark below, which showed a reduced “numerical ventilation” and resulted in 2.7x faster than a non-AMR case.
User-defined field-functions
User-defined AMR criteria using field-functions can work reasonably well for problems where solution features are easily associated with strong gradients. Example: gradient of Mach number to capture a shock, Laplacian of total pressure to capture wake behind a vehicle.
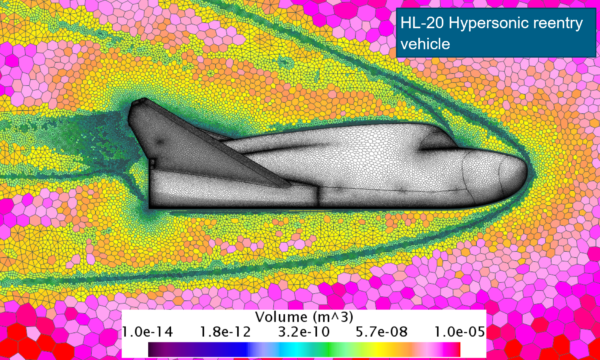
Pushing the envelope by combining different criteria
Users can add a combination of multiple criteria per simulation to reap the most benefit on complex computational models. In case of multiple refinements, the criteria requesting the smallest size wins. For coarsening, all the criteria must agree to coarsen.
An example of the Delta rocket stage separation demonstrates the refinement in the background mesh as the boosters are separating, using the model-driven AMR for overset meshes. Additional user-defined criteria based on Mach gradient and pressure gradients are used to capture the moving shock and body interactions.
The oil jet lubrication application below demonstrates the power of the Simcenter STAR-CCM+ AMR. It utilizes the free-surface model-driven AMR to capture the oil jet, the overset model-driven AMR to model the rotating gears and user-defined field-function AMR to model the tight clearances between the intruding gear teeth pair. This case when compared with a static mesh delivered a 10x reduction in cell count and a 6x reduction in computational time for the same accuracy.
The Epiphany
Inspired by my rendezvous with Apollo 11 at the Kennedy Space Center, I returned to my office with a clear goal in mind – to run an angle of attack sweep of a re-usable launch vehicle at atmospheric re-entry conditions using Simcenter STAR-CCM+ AMR. A single static mesh suitable for the entire angle of attack range would require large zones of uniform refinements to capture the shock and wakes for the entire sweep. I wanted to test if AMR could allow me to dynamically refine a single mesh for each condition. This would make aero database generation very efficient by completely avoiding remeshing.
I set out with the HL-20 and decided to run an angle of attack sweep from –10 to 30 deg at Mach 10 using the in-built Design Manager functionality of Simcenter STAR-CCM+. To my excitement, the simulations worked great starting from a single coarse initial mesh and using the gradient of Mach Number as an adaptation criterion to refine both the shocks and wake features throughout the domain.
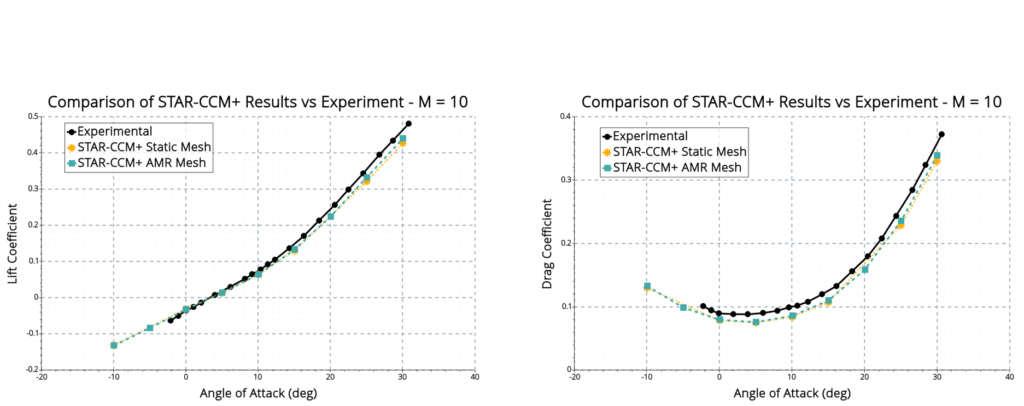
The lift and drag coefficients from the design sweep agreed closely with the test data. It was easier and faster to run the sweep using AMR, because I knew that I could let AMR handle the mesh refinements at each condition, almost like it was my “meshing guidance system.” Mission accomplished!
References:
[1] Slotnick, Jeffrey, Abdollah Khodadoust, Juan Alonso, David Darmofal, William Gropp, Elizabeth Lurie, and Dimitri Mavriplis. “CFD vision 2030 study: a path to revolutionary computational aerosciences.” (2014).
Comments
Comments are closed.
Excellent…Thank you.
Congratulations! Here’s to a bright future for adaptive refinement.