Weaving digital threads with machine learning for turbomachines
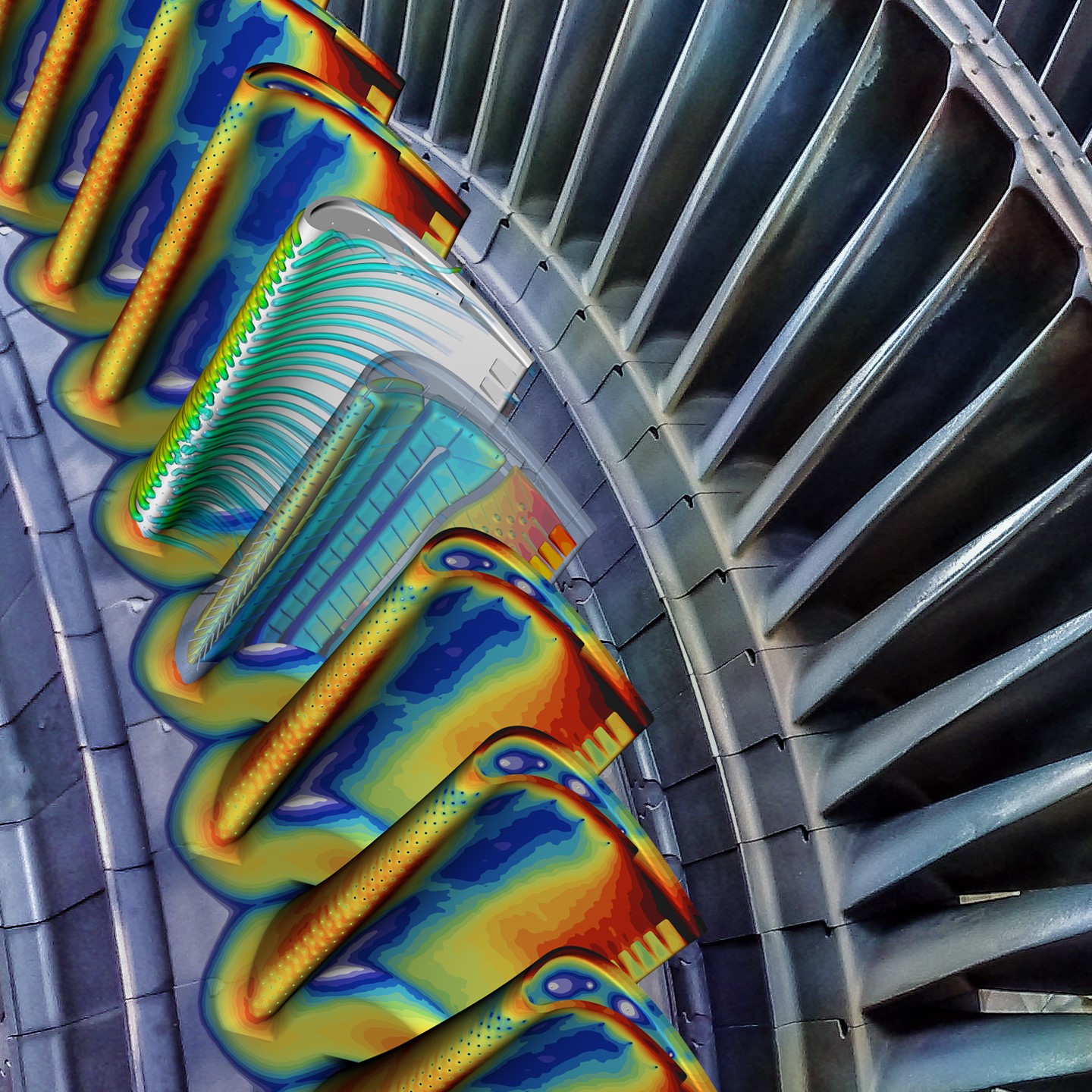
How can one combine the past, the present and the future? Depending on your point of view, things can be either changing rapidly or remaining stagnate. Is there anything we can do about the current situation? Can you change your or others’ destiny? In old Norse mythology, destiny was determined by the threads woven by the Norns and decided long before you were born. They controlled the past, present and your future and there was nothing you could do to change it.
This type of thinking is not as common anymore. A more recent and modern Swede famously said, loosely translated, “Most things are still undone. Wonderful future!”. This quote comes from the late and great Ingvar Kamprad, founder of IKEA. Ingvar certainly thought there were always improvements to be done and better products to be made. When writing this, we have just switched to the year 2024 according to the Gregorian calendar and it is at this time of the year that we all want to have an optimistic view on the new year to come.
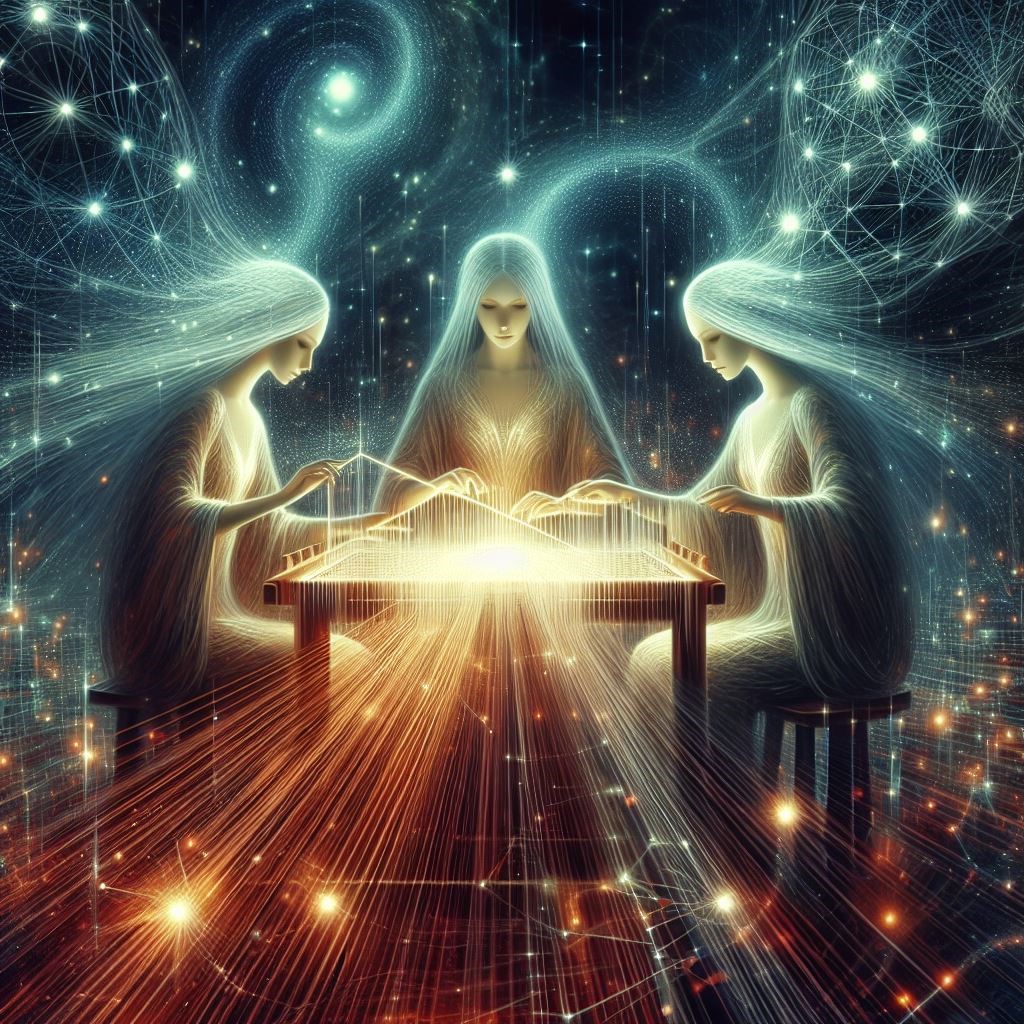
When it comes to new engineering and simulation techniques, one cannot avoid talking about what happens within artificial intelligence (AI) and machine learning (ML) and what effects this might have on future products. With this technological revolution, we are bound to see so many advancements in the coming years. Pair this with Siemens digital thread technology connecting computer-aided design (CAD) to computer-aided engineering (CAE) and manufacturing (CAM) and you will have a competitive advantage and will be able to bring new products faster to market.
Let’s look at a few examples from the turbomachinery industry. I asked Dall-E to generate IKEA-like instructions on how to assemble a jet engine. Figure 2 shows my first attempts:
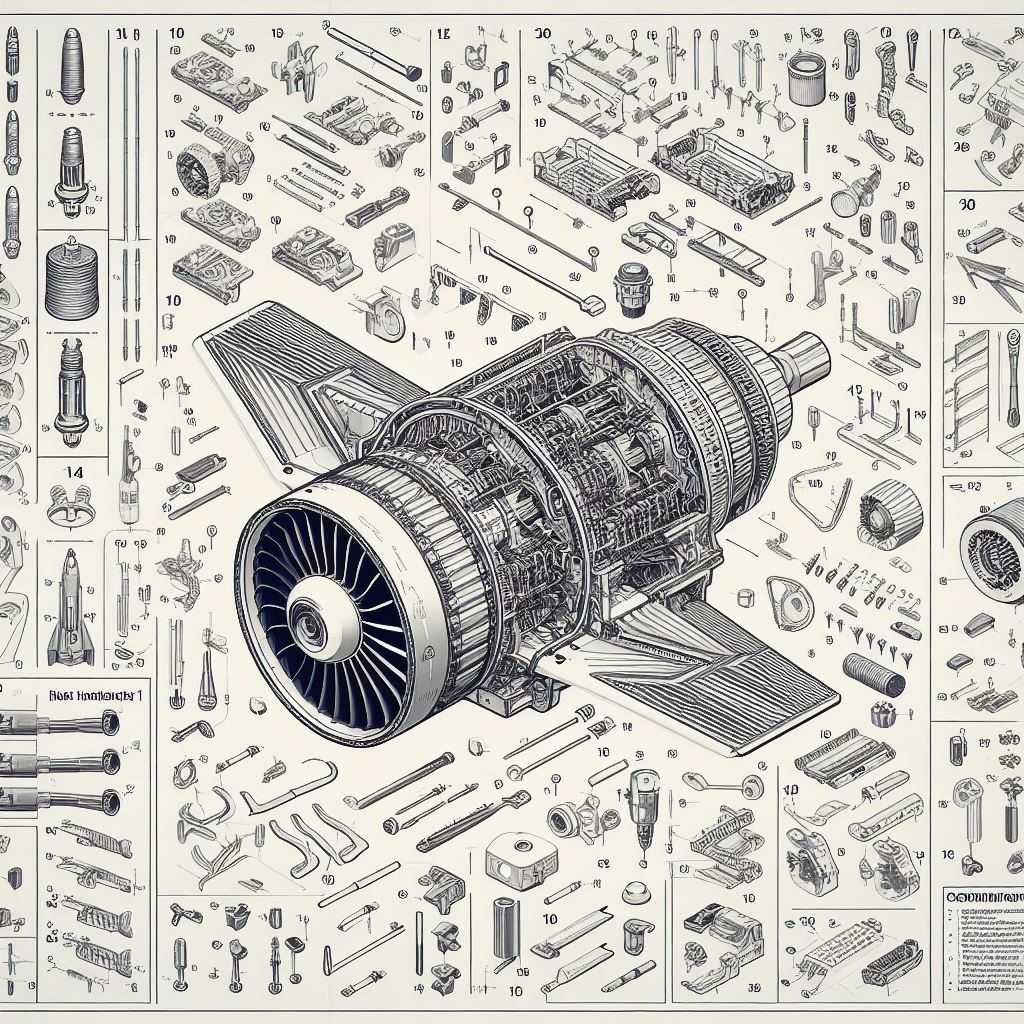
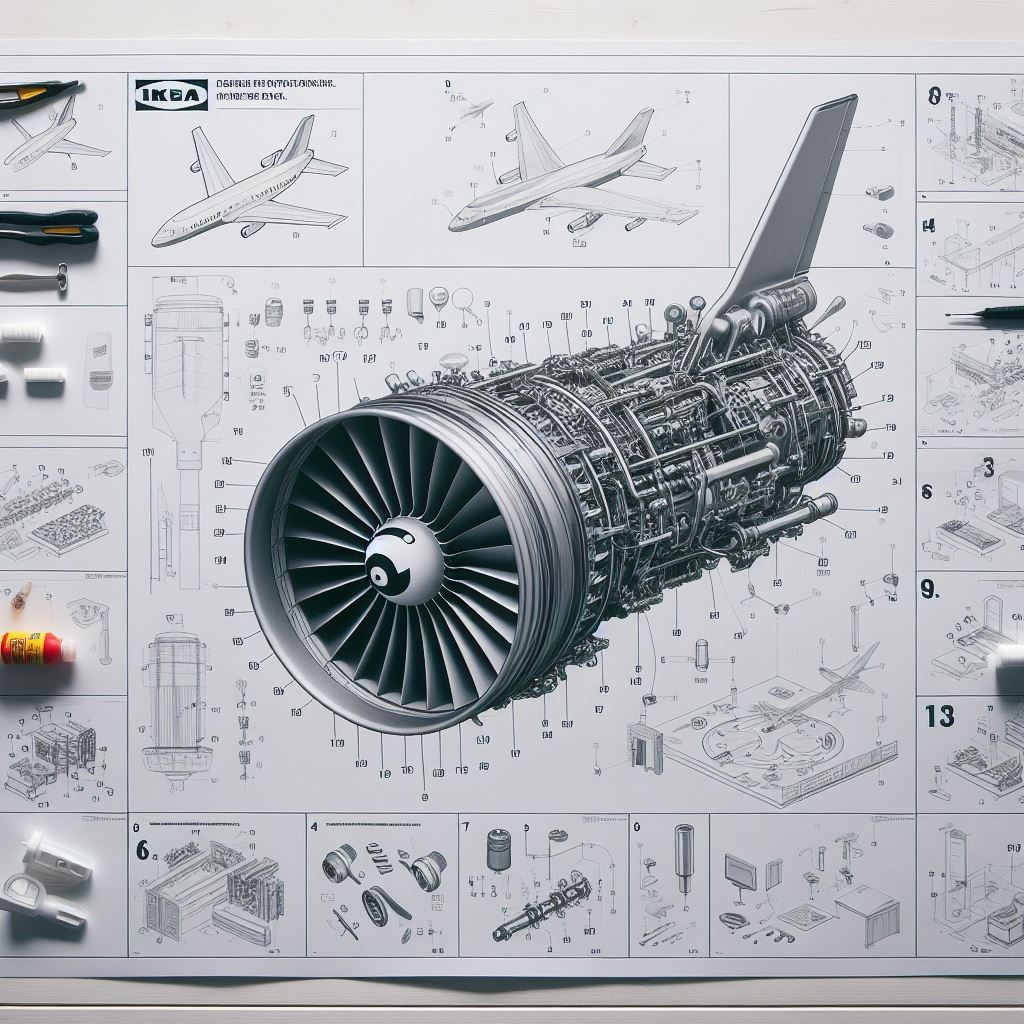
For now, it looks like I will still be able to keep my job for a few more years as the machines might still need engineers to help them make better machines.
A look at the current state of the art for gas turbine design workflow
Figure 3 shows the “classical” approach of a CAD image from a jet engine assembly using NX. Designing a gas turbine in the past would take several years and would not always be a success. Thanks to digital tools, we can improve on today’s design quite easily with a multidisciplinary approach of design an optimization.
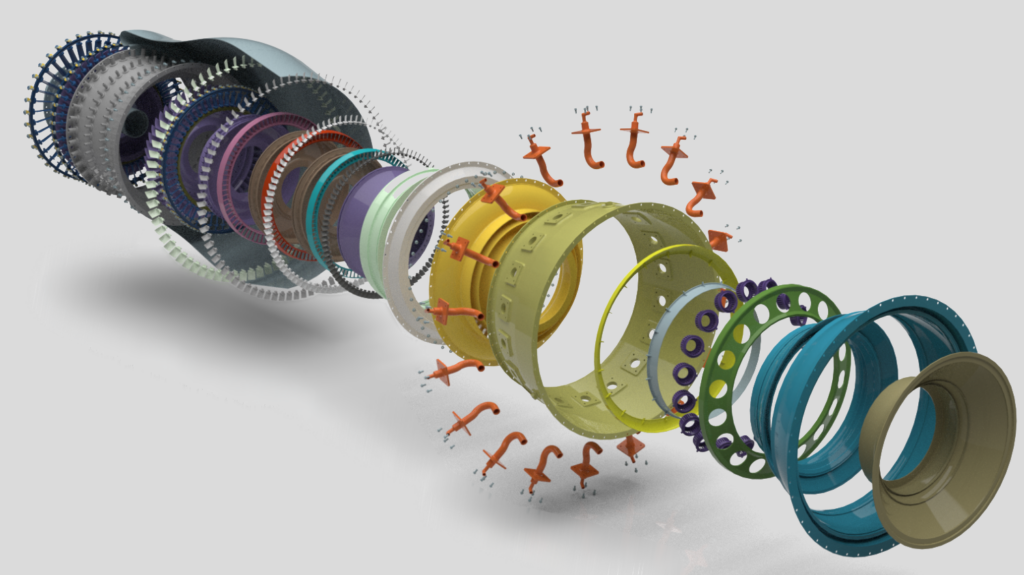
Even though it is very advanced physics and complex geometries, one can today combine several of these steps in an automated way. Keeping the CAD alive, boundary conditions and various versions stay totally in your control. The design process of a component is shown in the schematic of Figure 4. This is done by joining the CAD from NX to various CAE simulation tools like Simcenter STAR-CCM+ and Simcenter 3D. The automation and optimization are handled by HEEDS and all data is managed by Teamcenter.
It really does not matter if it is higher efficiency through aerodynamics, improved mechanical integrity and durability, reducing cooling air usage or new combustion fuels; they all affect each other and there is no way to be competitive and innovative unless correctly using modern multidisciplinary design space exploration methods.
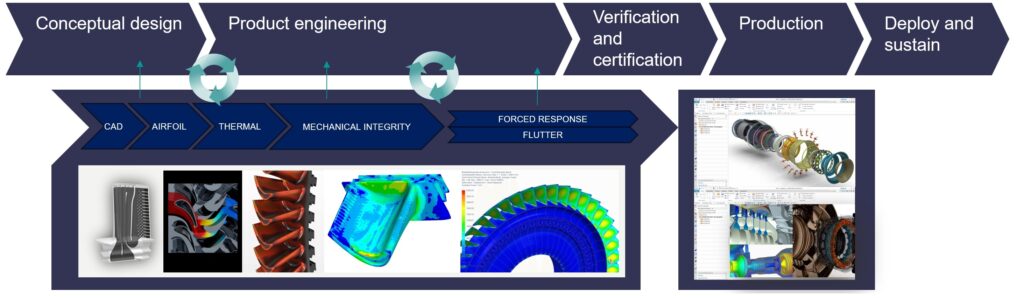
In order to effectively do product development, we want to evaluate as many designs as early on in the process as possible. Taking the next steps into the future means combining this with machine learning, since the design space can become large quickly and with many disciplines involved. What if we could have a machine learning algorithm train itself in real time on the design space that is currently being evaluated with computational fluid dynamics (CFD) or finite element method (FEM)?
An improvement on multidisciplinary design optimization for future product engineering
For that, we have two proofs of concept that are related to turbomachinery. One is to optimize a water pump efficiency at a flow rate of 110 kg/s and 1200 rpm. We worked on a parametrized model with 12 geometric variables and the number of blades.
HEEDS, a comprehensive multi-disciplinary design analysis and optimization (MDAO) software, uses its default search method, SHERPA, to conduct multiple search strategies simultaneously, and it dynamically adapts to the problem as it learns about the design space. With SHERPA, HEEDS can discover 300 design variations in 40 hours. With the introduction of HEEDS AI Simulation Predictor, an add-on extension in HEEDS, SHERPA’s search technology is significantly enhanced. Some CFD simulations are replaced by AI evaluations conducted through an automatically trained AI model, leveraging insights gained from early simulations – revolutionizing this process. In this case, it counted 151 CFD runs while 149 were done with AI evaluation (for a total of 300). This took roughly 20 hours reaching the same results and saving 49% in time. The pump’s efficiency increased by 3% and head by 10%.
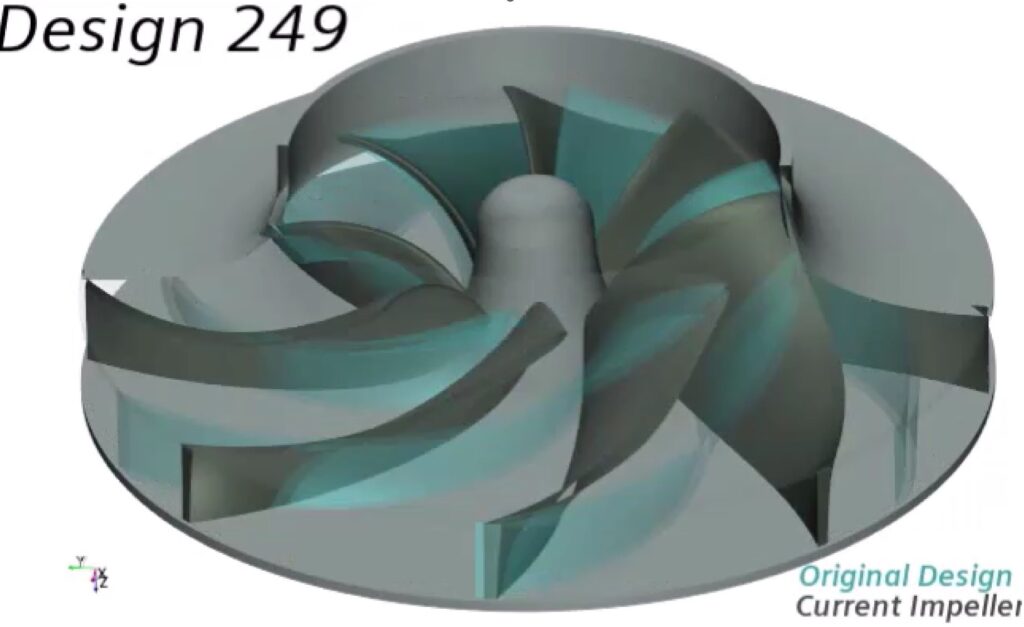
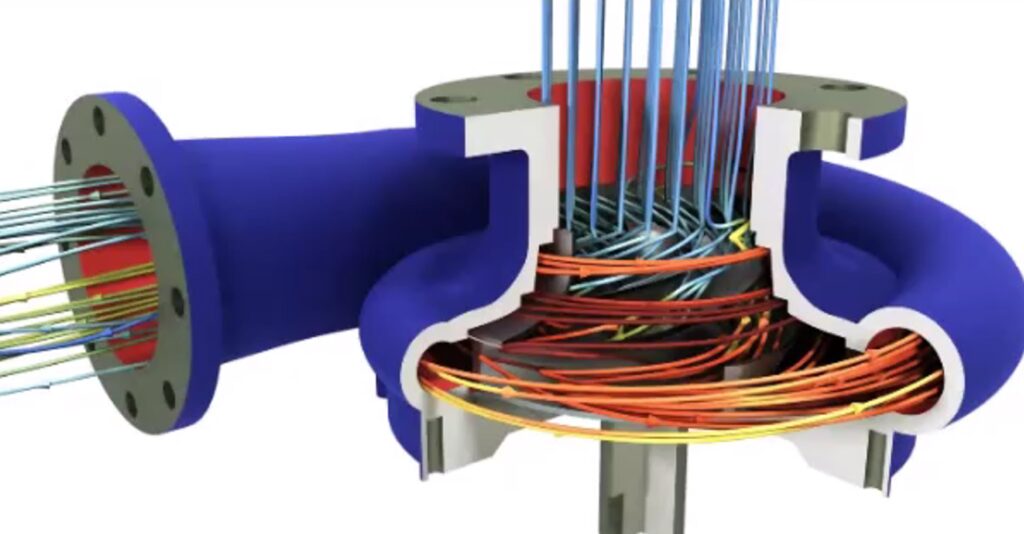
Figure 5: Water pump – design space exploration with HEEDS AI Simulation Predictor – CAD and CFD results
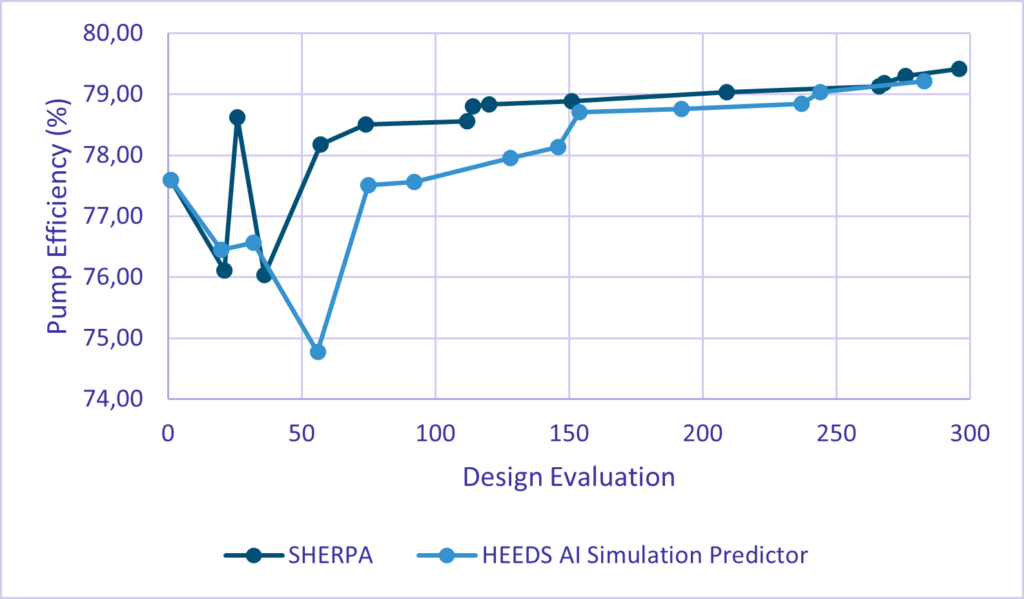
The second case is a gas turbine blade for cooling optimization. Here, the objective is to minimize blade temperature and minimize cooling air mass flow. A parametrized CAD from NX is used to simulate in Simcenter STAR-CCM+. The CAD has 34 parametrized characteristics on the serpentine channel with changes of cooling ribs and shower head holes (see Figure 7). The 500 design evaluations done for this case experienced an approximate 38% time save, skipping CFD simulations with AI and still reaching the same best solution. This might mean 20 days of time saved if 160 cores are used for each simulation. This way, you could easily save weeks and months on projects and get a better product faster to market.
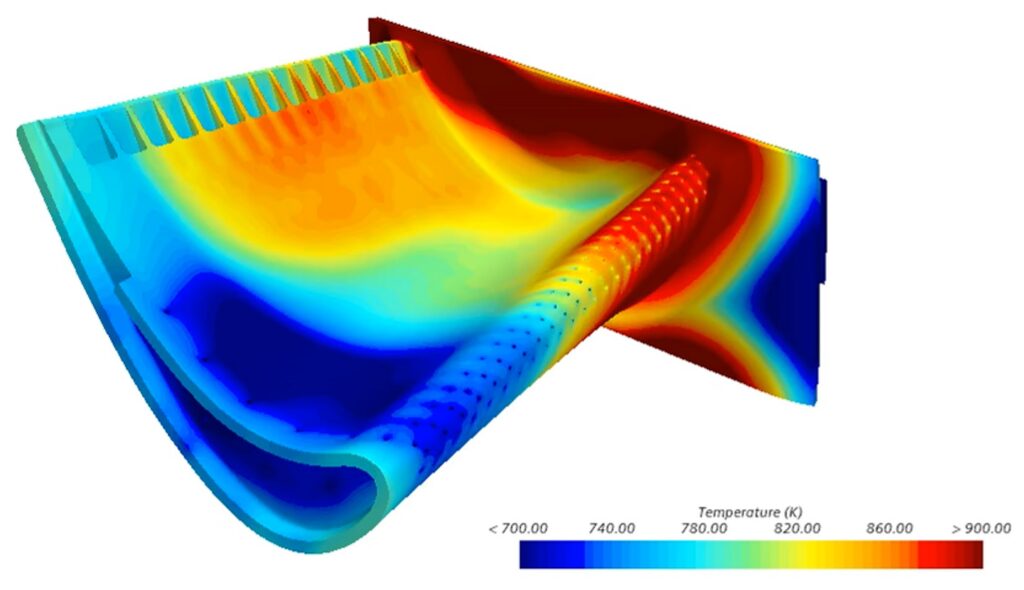
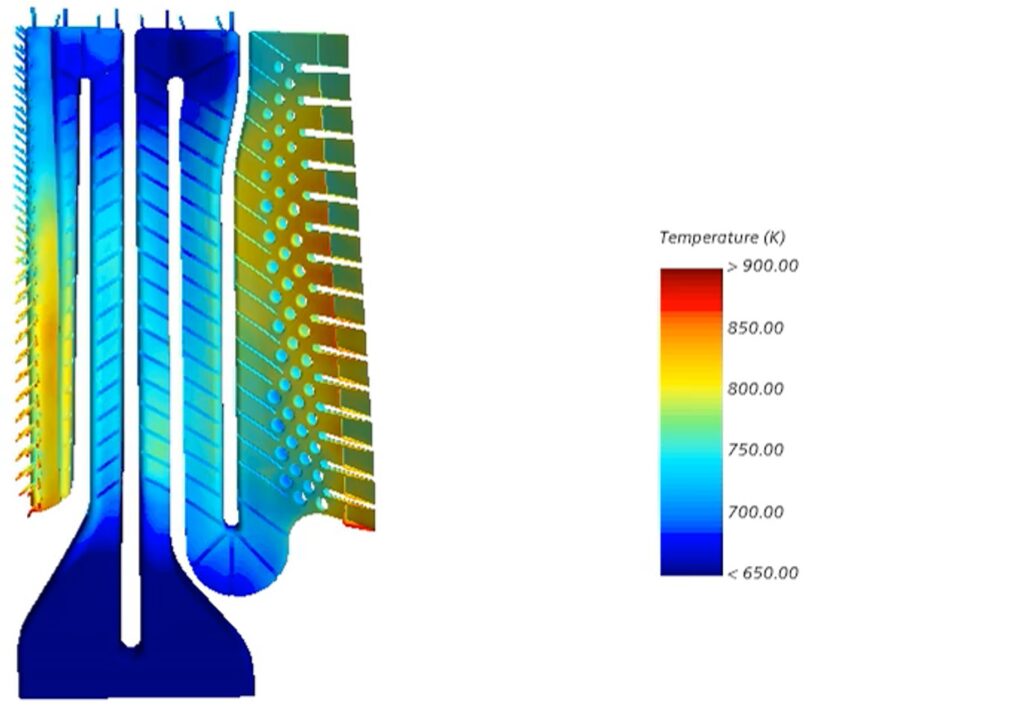
Figure 7: External and internal temperature for conjugate heat transfer turbine blade design space exploration with HEEDS AI Simulation Predictor, NX and Simcenter STAR-CCM+.
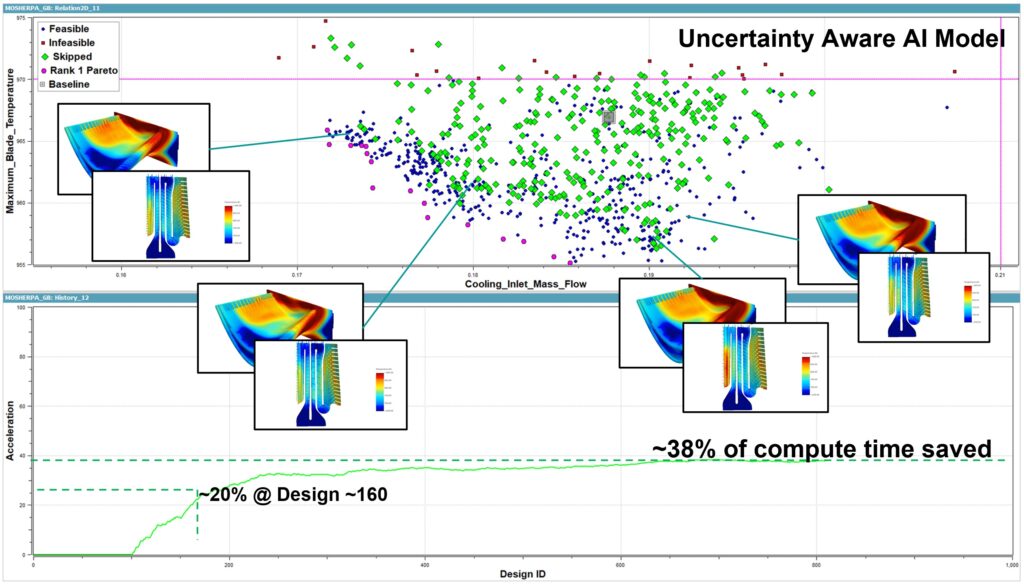
From these first examples of adding AI and machine learning to an already impressive CAD-CAE workflow, one can already see the potential and how easy it is to get started without being a machine learning or optimization expert. How big the revolution of AI and ML will be and the impact it will have on the fate of the mechanical industry is too early to say. But we already know that it will be the key to staying in front of the competition. In conclusion, my advice would be summarized like this:
- Learn from what we have done in the past, as wisdom is important.
- Use this in a digital thread to improve on our old products and methods.
- Take control of your destiny to create a wonderful future!
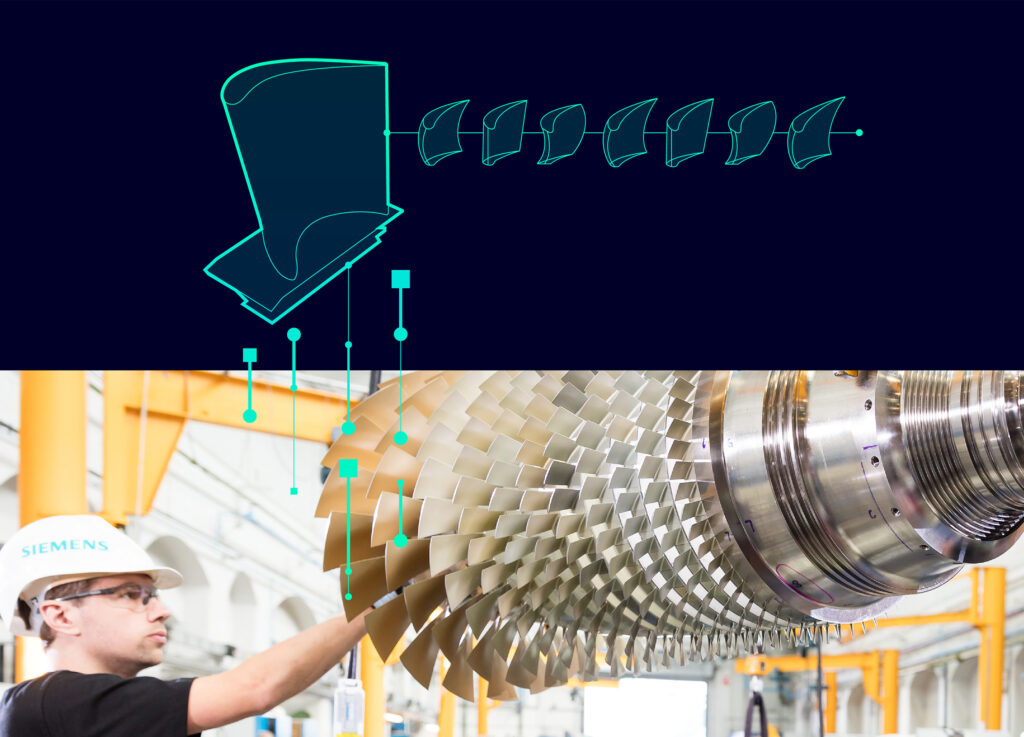
Acknowledgements:
I would like to acknowledge the great engineering work of Gabriel Amine-Eddine, Jeremy Hanke and René Braun.
You might also be interested in…
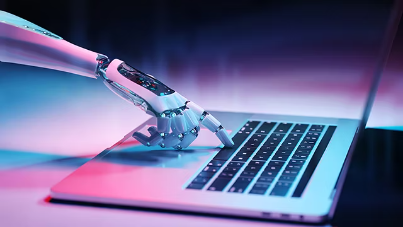
Solution
Unleash innovation with AI/ML and Simcenter
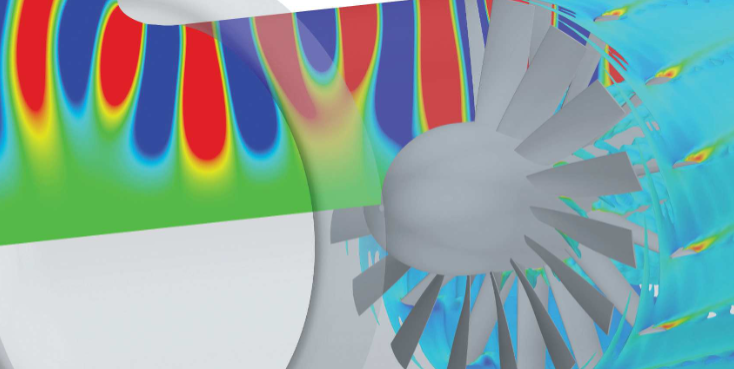
White paper
Reducing aircraft engine noise