Fasten your seat belts: the coupled solver is taking off on GPU!
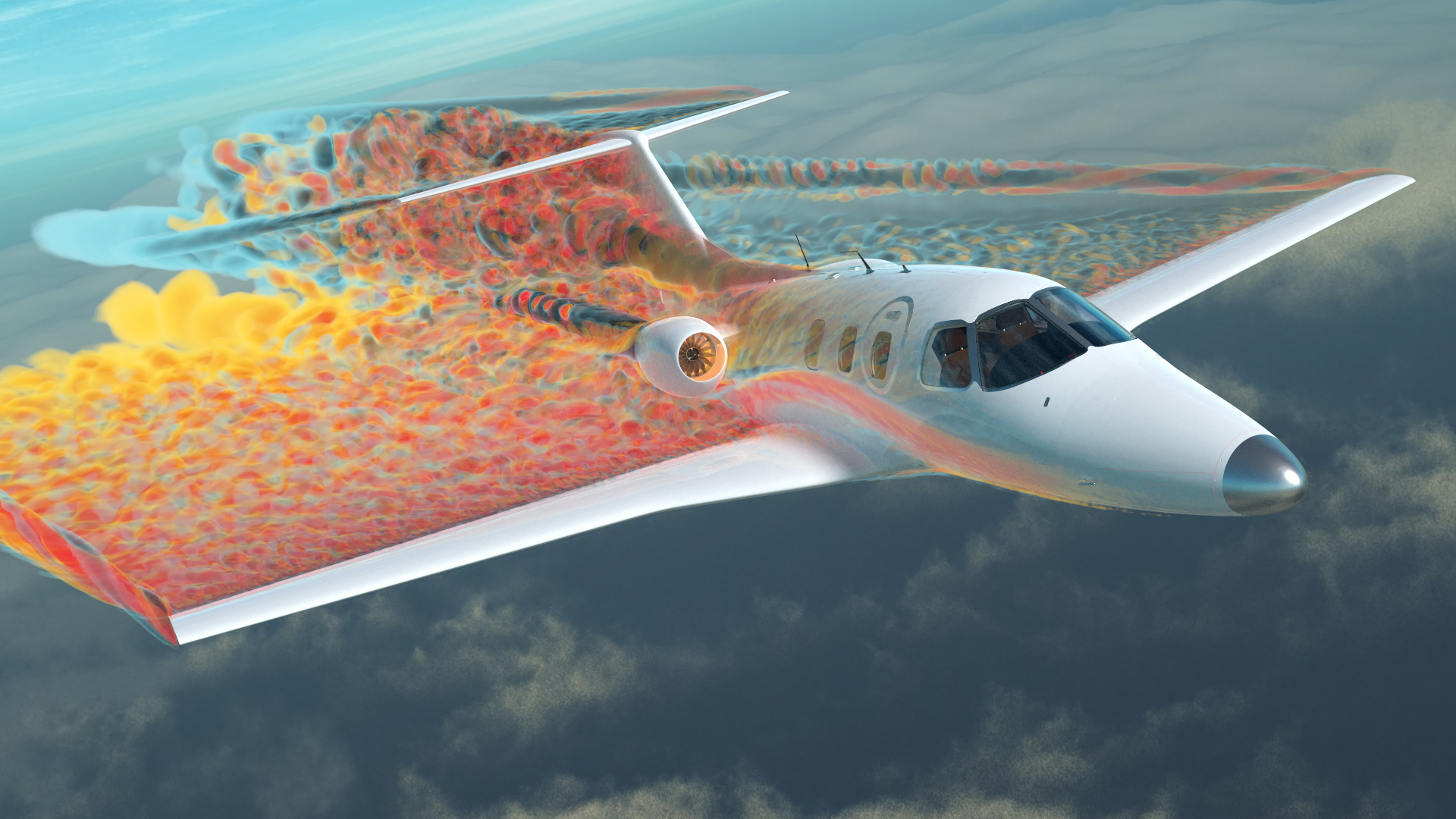
Cabin crew, please take your seats for take-off.
Pilots around the world
Do you see yourself on a plane listening to these words coming loud and clear out of the speakers? After all those long queues and waiting you have done, expecting your turn, and then the next one, and the next once again, these are really the only words you want to hear. We are ready to take off! In that moment, the captain’s announcement fills the air with a sense of anticipation and adventure, and you anxiously wait for the instant when wheels leave the ground.
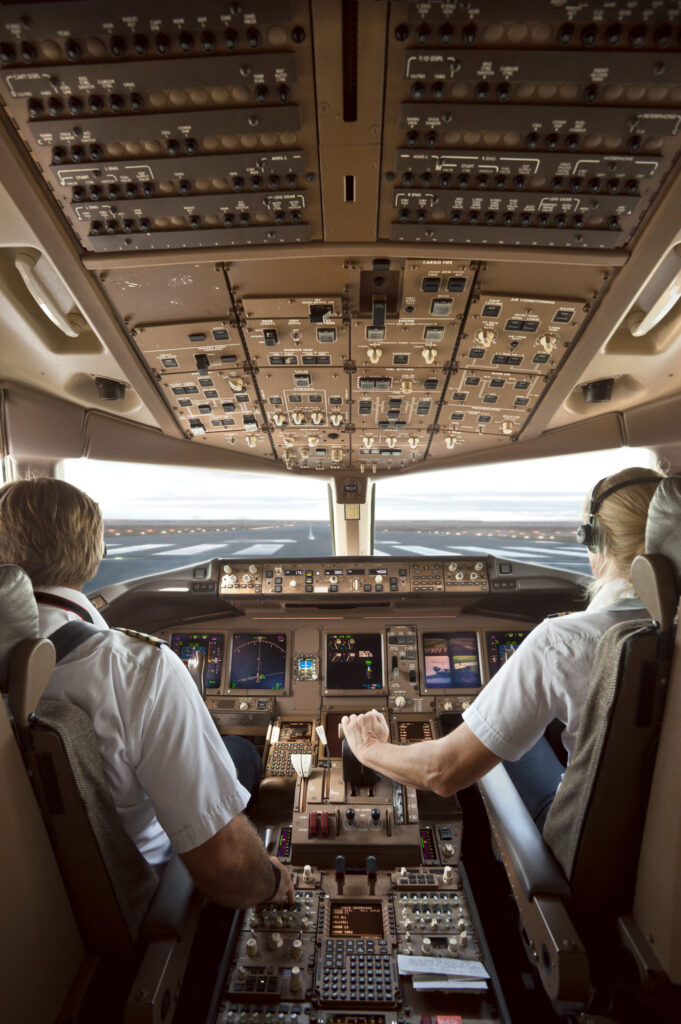
Today, I feel some of the same thrill waiting for the upcoming release of Simcenter STAR-CCM+ 2306. So, fasten your seat belt and come fly with me! With our new GPU capabilities, you will experience a tool that will boost you ahead, expand your goals and turn your product development workflow into a sustainable success story. The countdown has begun, and we are here to be your trusted co-pilots on this extraordinary journey.
GPU-native coupled flow and energy solver
In Simcenter STAR-CCM+ 2306, we continue to broaden our portfolio of GPU-based solvers with a big leap forward for many relevant industrial applications: the GPU-native coupled flow and energy solver.
The coupled solver in Simcenter STAR-CCM+ is a very robust and efficient density-based solver that has been for years the best practice for several industrial applications, amongst which: automotive vehicle external aerodynamics, aerospace aerodynamics, turbomachinery aero performance and Conjugate Heat Transfer (CHT) blade cooling. Compared to the segregated solver, some of its greatest advantages are the linear scalability of CPU time with cell count and the ability to achieve deeper (and faster) residuals convergence, especially for steady state flows. The recent introduction of the Automatic CFL control has further improved its ease of use and accessibility, by ensuring a straight out-of-the-box convergence for a variety of flow problems.
In Simcenter STAR-CCM+ 2306 all this will be available on GPU, providing you with a solution for faster turnaround time and lower costs per simulation. Moreover, we ensure CPU-equivalent flow solutions by maintaining a unified codebase, hence providing a seamless user experience and consistent results irrespective of the hardware technology used.
Let’s have a deep dive and get some simulations off the ground!
Faster aerospace aerodynamics on GPU: the High Lift CRM
The High Lift Common Research Model (CRM-HL | NASA Common Research Model) is a variant of the base CRM case, developed by NASA with the intent of providing the aerospace community with an open-source wing-body high lift reference for validation of CFD codes. Geometry and experimental measurements are publicly available and have been the main test case of the AIAA High Lift Prediction Workshops over the last years. The workshop series aim to evaluate the effectiveness of current CFD technology in predicting high-lift aerodynamics for swept wings, develop modeling guidelines and improve prediction accuracy for practical design. Leveraging a fully automated CAD-to-mesh workflows, the efficiency of the coupled solver, and best-in-class turbulence models, Simcenter STAR-CCM+ provides outstanding capabilities for the simulation of these flows and was one of the four selected RANS best practices among the participant at the 4th HLPW.
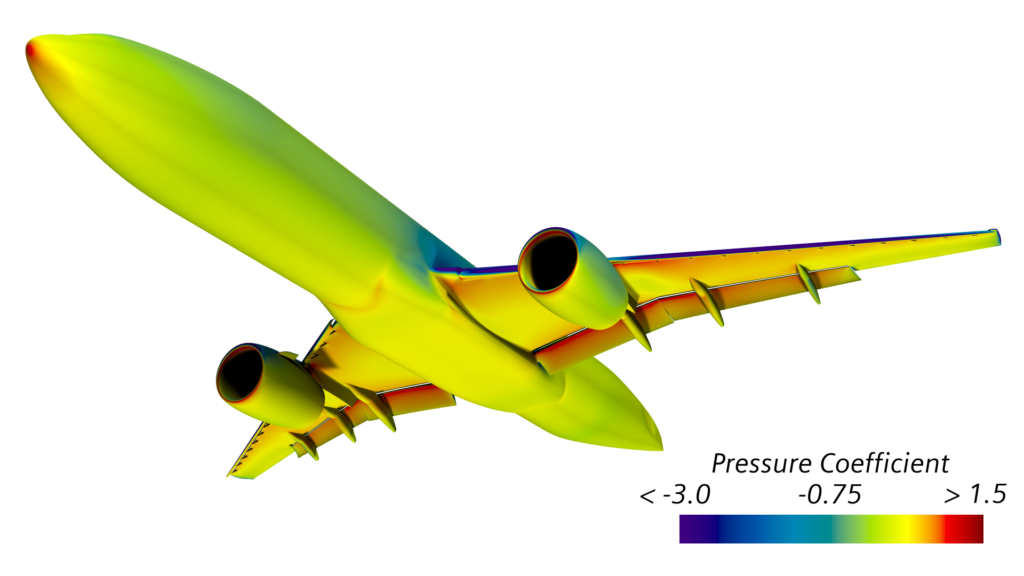
This case is considered here to analyze the performance of the coupled solver on GPU. The computational mesh generated in Simcenter STAR-CCM+ consists of 110M polyhedral cells and prism layers and is run in RANS steady state with the SST-K-Omega turbulence model with a modified a1 coefficient. The simulation is stopped when asymptotic convergence is reached for drag, lift, and pitch moment coefficients. Running on 24 NVIDIA A100 GPUs, this run is completed in only 40 minutes! Quite an impressive turnaround time, considering that the equivalent number of state-of-the-art CPU cores to equal this performance would be close to 3800.
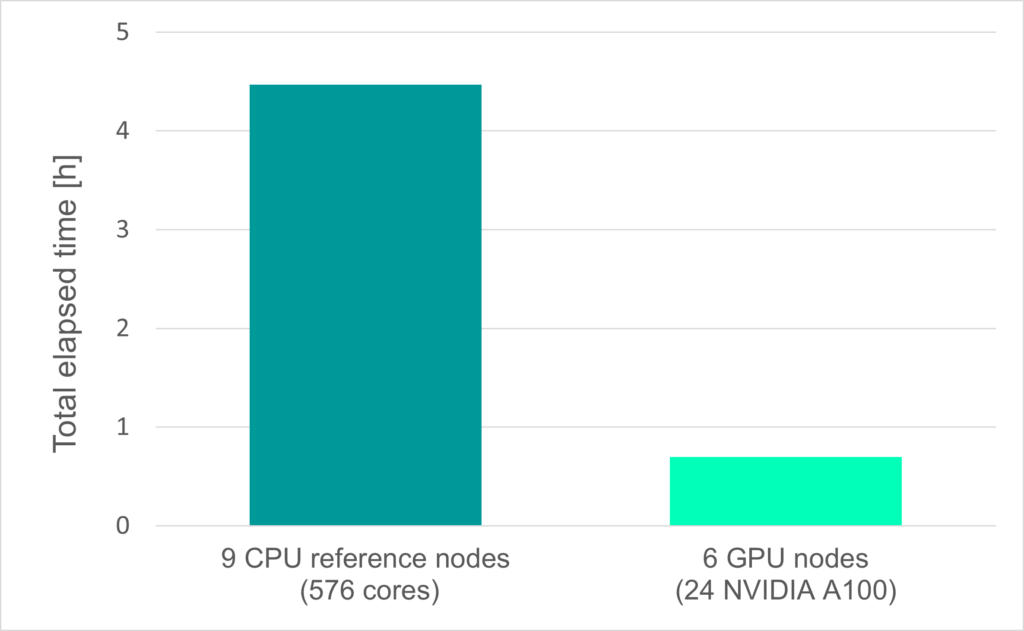
Converging the flight trails
Ensuring consistent results is paramount for CFD engineers, whether they choose to run on CPU or GPU technology. In various scenarios, CPU hardware may still be the preferred solution and recent advances in technology have demonstrated astonishing performance either.
As such, the numerous stand-alone silod GPU solvers that are currently popping up on the market pose several big risks:
- They might force you to migrate existing models to conform with the standalone GPU solver
- For companies that relied on CFD runs on CPUs (which most of us CFD engineers are) a standalone GPU solver poses the need for you to re-evaluate results to ensure consistency with your legacy database and gain trust and confidence
- They make it hard on you to seamlessly switch between CPU and GPU as the cluster load/ project / business case requires
This is why it is key for us to provide our users with a seamless GPU experience, offering the flexibility to easily switch from one type of hardware to another and leveraging code abstraction methods that will guarantee CPU and GPU will be fed with the exact same physics model implementations.
As for all other solvers in Simcenter STAR-CCM+, a prominent level of consistency between CPU and GPU solutions is therefore guaranteed. The GPU-native coupled flow and energy solver maintains a unified codebase with the CPU-based solver, ensuring equivalent flow solutions.
Continuing our journey on the High Lift CRM, the results consistency can be appreciated by observing the convergence behavior of the drag coefficient and the surface distribution of skin friction on the wing and nacelle.
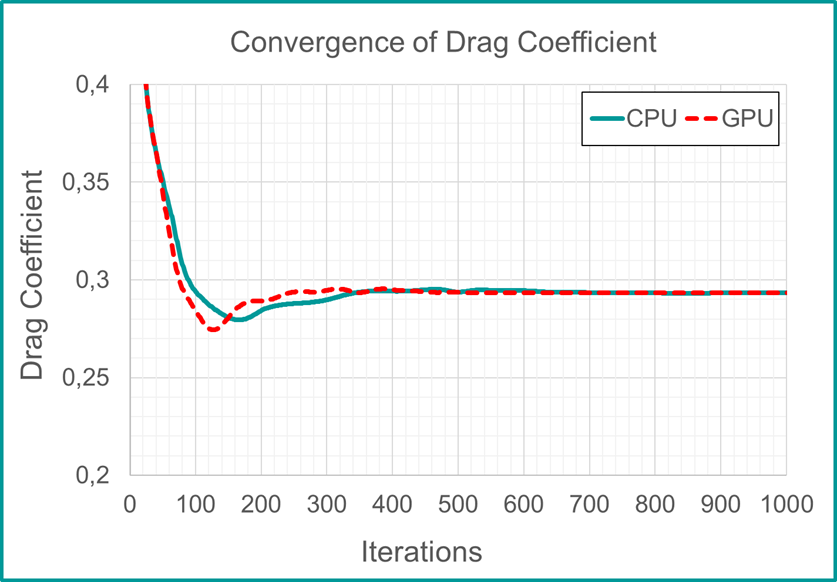
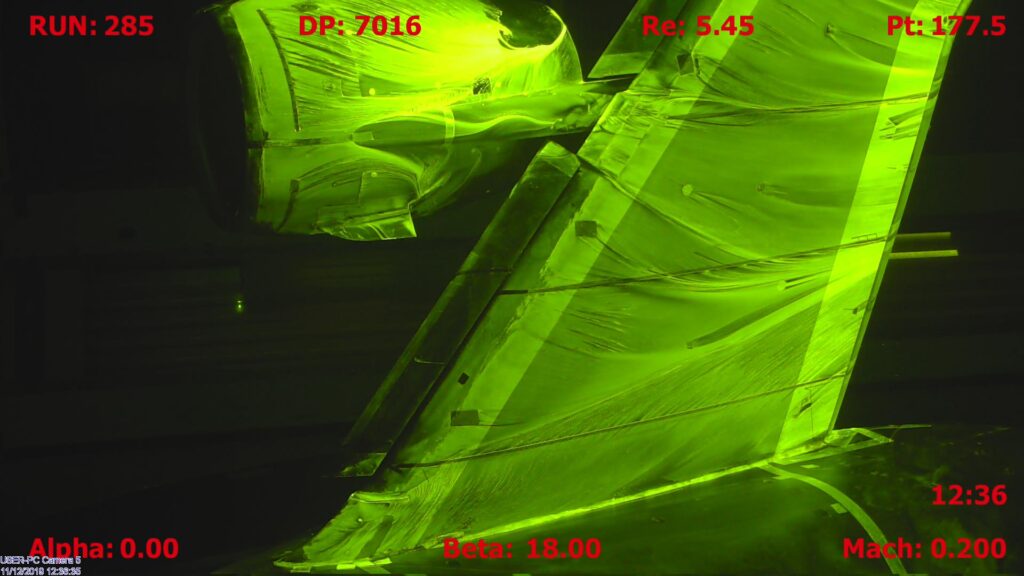

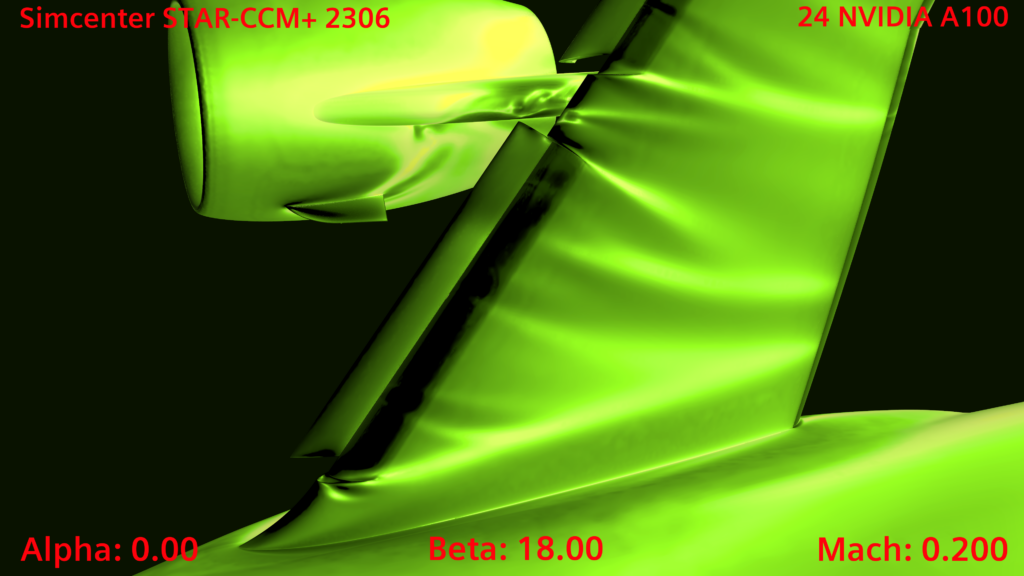
Comparison of the skin friction distribution between experimental test, CPU and GPU solutions, for the configuration at angle of attack of 18° (source: High Lift Prediction Workshop 4 – Wind Tunnel Data (nasa.gov))
Flying from cruise to high lift – a sweep study
Building upon our product pillars, accuracy continues to be a core priority for Simcenter STAR-CCM+.
During our flight on the High Lift CRM, we looked at how we perform, comparing the GPU results to test data. For this purpose, we performed a sweep study on the full spectrum of angles of attack, with a total of 25 simulations ranging from 2° to 21.5°. The results confirm excellent accuracy of the solutions with a high-level of predictability of forces and moment coefficients at all angles of attack.
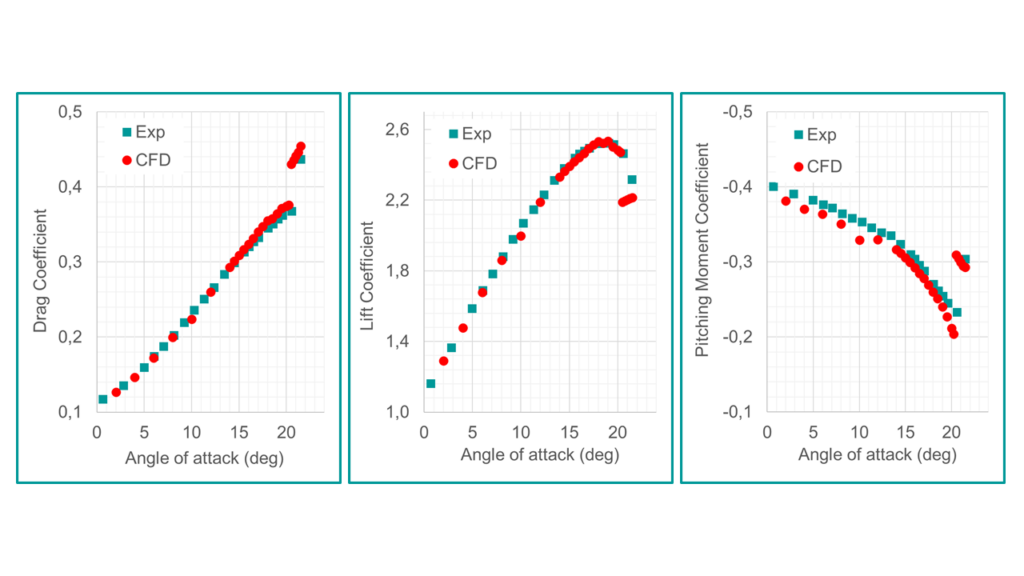
And the most exciting news is that the full study, with a mesh of 110M cells, can now be completed in 19 hours running on 24 NVIDIA A100, whereas a whole week would be required on CPU with 576 cores! These numbers, reveal an enormous potential to increase productivity at lower costs, something particularly attractive when creating digital aerospace databases from 100s of simulations.
Taking off on a budget!
While speed, consistency and accuracy of results are key aspects for CFD engineers, the primary advantage of migrating flow simulations to GPU hardware lies in enabling users to adopt sustainable practices and utilize cutting-edge technologies to achieve substantial cost savings, whilst minimizing environmental footprint.
Focusing on the GPU-native coupled flow and energy solver in Simcenter STAR-CCM+, a comparative study in collaboration with NVIDIA was conducted with two industrial test cases, comparing the performance of one GPU node with 8 NVIDIA A100 cards to one node of a popular CPU-only configuration with 128 cores.
Estimates based on the two test cases show the GPU-node energy consumption at about 31-36% of the CPU-only reference node.
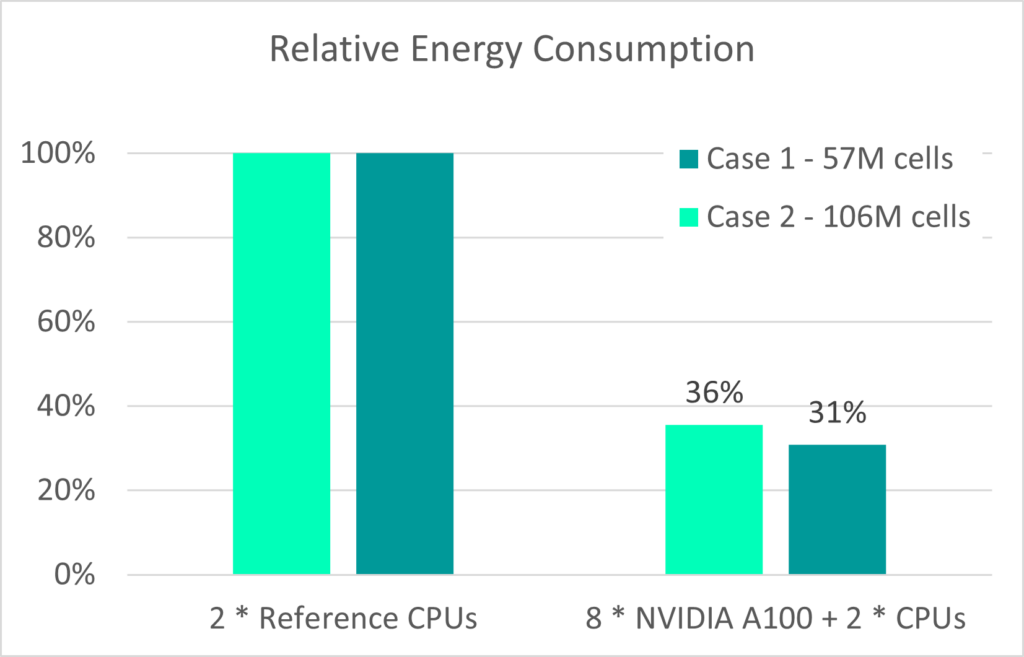
A strong cost reduction is also achieved when employing HPC cloud services. With a cost estimate from a popular cloud provider, running both test cases on an NVIDIA A100 node offers cost reductions of 44-51%, compared to the reference CPU-only node.
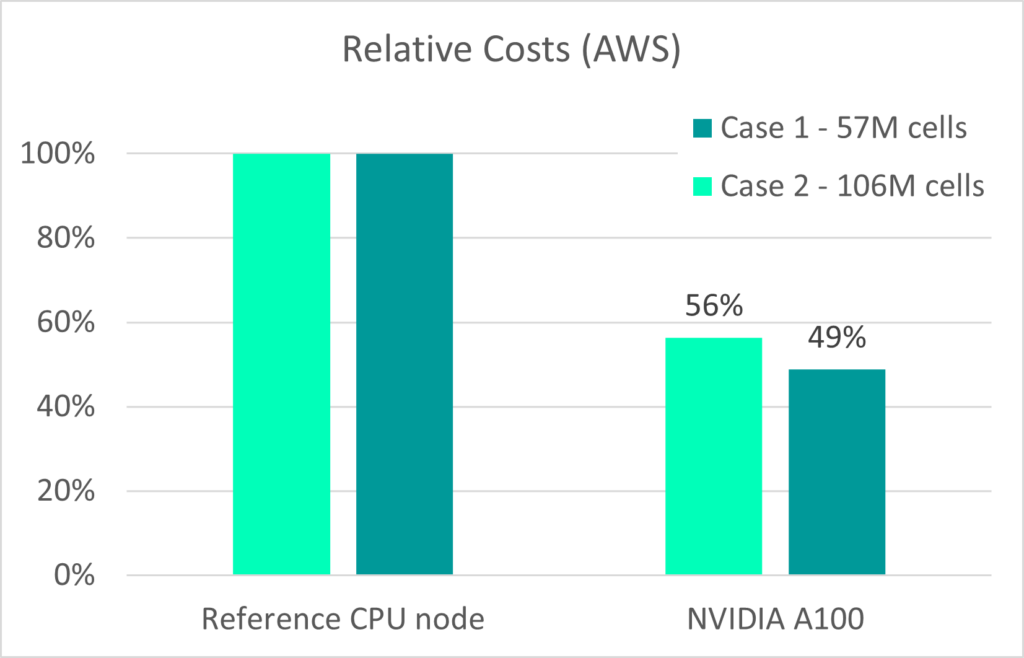
Finally, the compute time is sped up by as much as 17x using a node with NVIDIA A100s or up to 23x using nodes with NVIDIA H100s, compared to a reference CPU-only node.
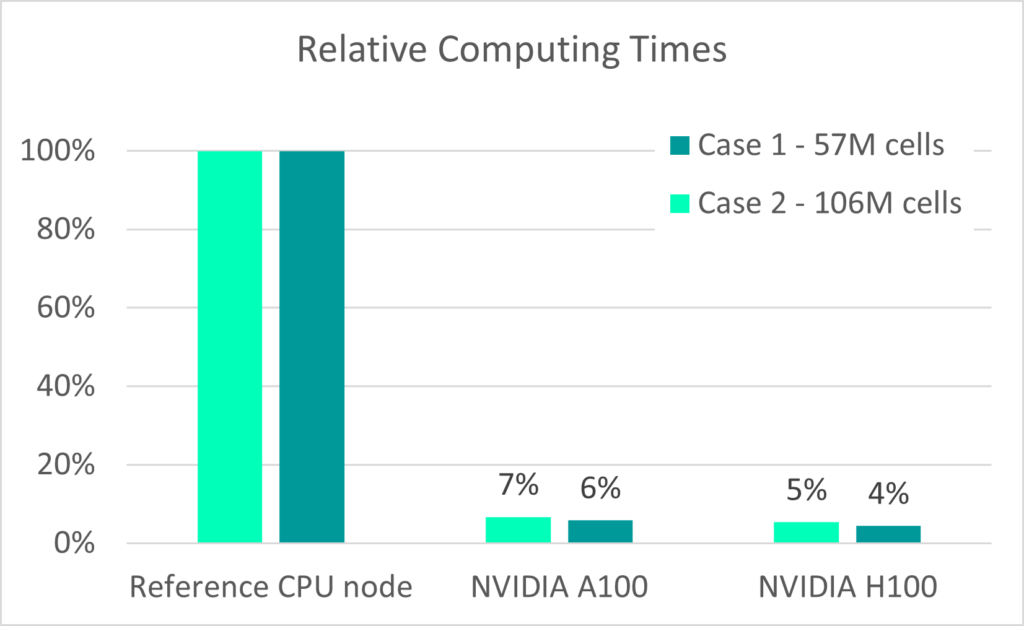
High-fidelity wall-modeled LES with the coupled flow and energy solver
Is this everything for this journey? Well, we have not landed yet.
In addition to the GPU-native coupled flow and energy solver, Simcenter STAR-CCM+ 2306 comes with a fully GPU-native wall-modeled LES (WMLES) model, turning the deployment of WMLES simulation into a more viable option for aerospace aerodynamicists (and not only 😊). We have investigated the aerodynamic simulation of a business jet in a side-slip configuration, for which the computation of 1s of physical time can be achieved in 6 hours on 24 NVIDIA A100, with a computational grid of about 130M cells.
More GPU highlights in Simcenter STAR-CCM+ 2306
If this story has sparked your interest, I would highly suggest you try it yourself. Simcenter STAR-CCM+ 2306 will soon be released and will land on your desktops with a bouquet of sensational features. Including a whole lot more on GPU-native solver capabilities:
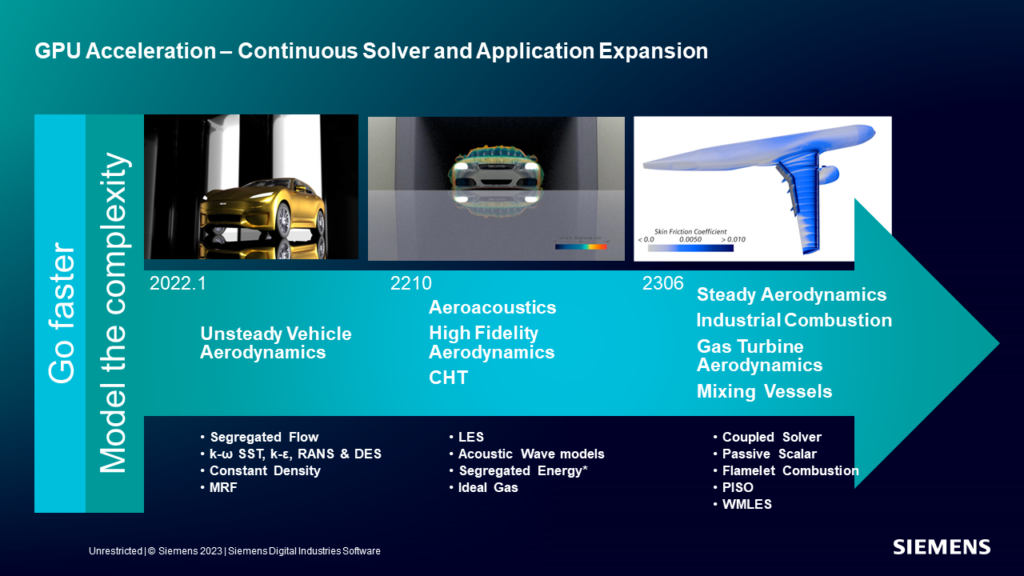
As for me, although our virtual flight has come to an end, I still feel that thrill resonating within me…time to access the GPU cluster and launch some high-fidelity aerodynamics simulations.
Thanks to Liam McManus, Chris Nelson, and Stephan Gross for their contribution to this work.