Insuring AI on the factory floor – the transcript
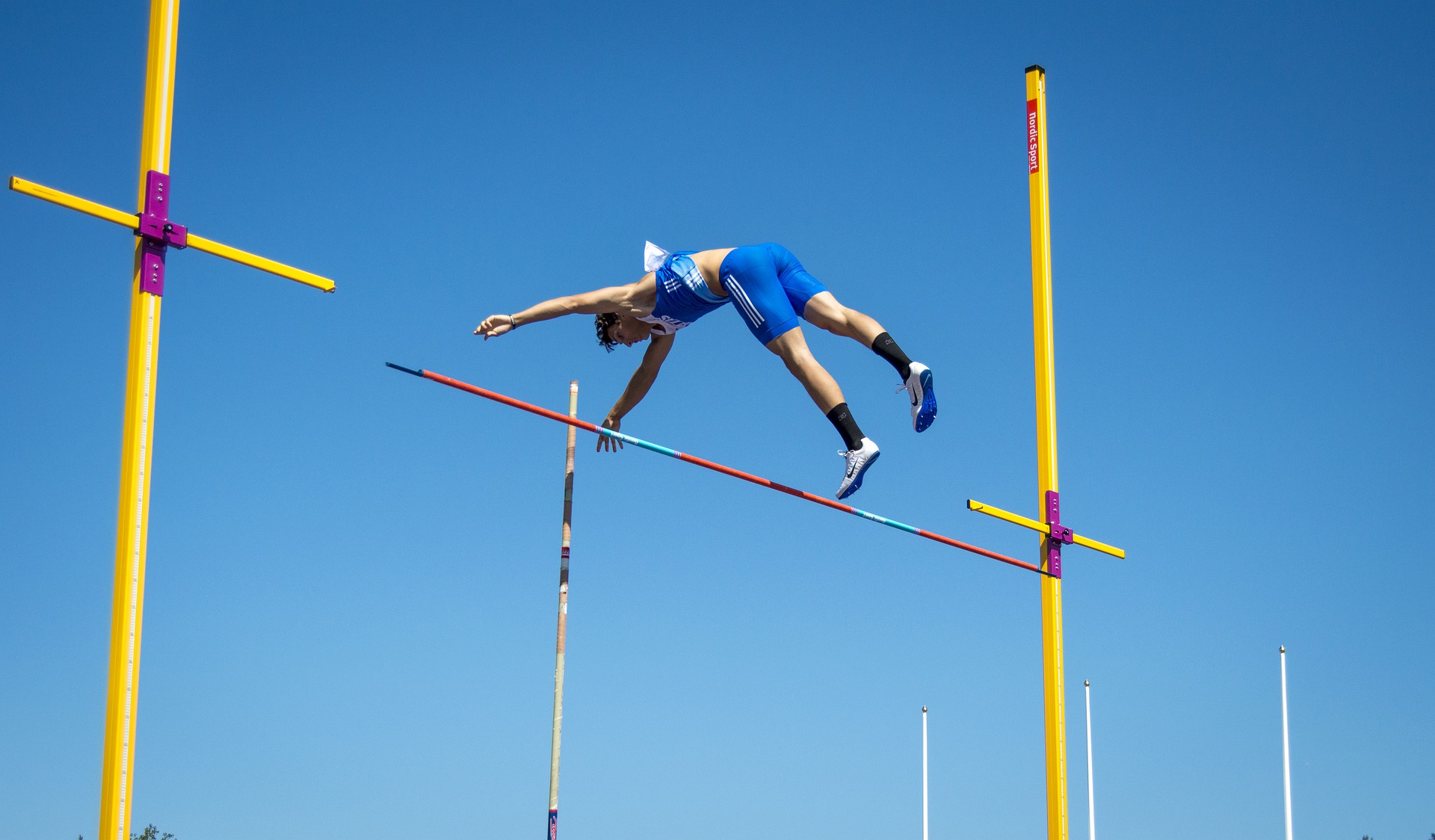
Previously, I wrote a blog about insuring AI. As a follow up, I then had a real interesting interview with our Siemens expert and a representative from a leading insurance company in this podcast. For those that would rather read than listen, here is the transcript from that podcast session.
[00:00:10] Thomas Dewey: Welcome to AI Spectrum, where we discuss an entire range of artificial intelligence topics. I am Thomas Dewey, your host, and today we explore an exciting and emerging concept: ensuring AI-based systems against failure. Joining us today are Michael Berger, who is head of Munich Re’s AI Insurance Unit; and Boris Scharinger, Senior Innovation Manager at Siemens Digital Industries. Gentlemen, thanks for joining us today.
[00:00:38] Boris Scharinger: Thanks for having us.
[00:00:40] Michael Berger: Thank you for having us, Tom.
[00:00:41] Thomas Dewey: Boris, can you briefly describe your role at Siemens, and perhaps give us some AI portfolio context, like what role does AI play in your business?
[00:00:51] Boris Scharinger: So, as you said, my role is Senior Innovation Manager in the Siemens Digital Industries Strategy Team. My areas of focus are artificial intelligence and high-performance computing – so, that’s what I care most about. And artificial Intelligence in our portfolio is important, it actually is a fairly broad range. It starts with very basic things: Spicing up existing products. If you imagine we have this wonderful engineering piece of software called NX, and AI supports users by providing a smart user interface. So, it helps increase user productivity by smart UI functions. That’s one area where AI plays a role in our portfolio. AI also speeds up our amazing and time-consuming simulation activities. We have simulation software for engineering purposes, computational fluid dynamics that is really making a big difference in speeding things up. And last but not least, there is a vast range of AI-related applications on the shop floor: visual quality inspection or predictive maintenance, things that happen either in discrete manufacturing or in the process industries all over the place.
[00:02:11] Thomas Dewey: Basically, it sounds like AI everywhere at Siemens. So, Michael, what about your role at Munich Re, and what is Insure AI all about?
[00:03:48] Michael Berger: I’m heading the Insure AI team at Munich Re. Perhaps to give some background, Munich Re is one of the leading global reinsurers and is strongly advancing its transformation towards new business models and my team is part of these transformation initiatives. Munich Re offers the insurance product, which we call aiSure. So, this insurance product covers AI performance risk. The idea is that if the AI does not perform as expected, AI users get a financial indemnification and this makes it easier to adopt AI services. So, our aiSure product makes the AI service more attractive, it creates a competitive advantage for the AI service provider. This helps the AI providers to acquire more clients and to grow faster because of the extra trust and credibility that aiSure creates. And we believe that Munich Re is solving a common challenge with aiSure, namely, using insurance to build trust into new technologies. In this case, the new technology is AI. Via our product, we incentivize the adoption of robust AI. So, in short, aiSure is about the following: in case the AI fails, the user receives a payout for the economic loss.
[00:03:40] Thomas Dewey: Well, we’ll dig into more of that a little bit later. But first, we wanted to find out, Michael, what inspired your personal interest in AI?
[00:03:48] Michael Berger: My fascination in AI comes from my interest in studies about decision making, especially in the presence of uncertainty. So, questions like how to and how should we make decisions under uncertainty? So, those questions always fascinated me. And nowadays, we have more and more data available to guide our decision-making. And of course, this poses the question, how should we utilize this data to do so? And this is the reason why I started studying data science and tried to understand the limitations of AI and machine learning. And this led to the idea to insure some of the risks related to AI.
[00:04:29] Thomas Dewey: All right, it sounds like a great path. So, what role does Munich Re want to play in the domain of AI in the industrial application space?
[00:04:40] Michael Berger: So, Munich Re offers services along the whole IoT value chain. On the one hand, Munich Re provides its own database services and analytics, for example, via our subsidiary Relayr. And on the other hand, Munich Re supports AI service providers via our aiSure product.
[00:04:59] Thomas Dewey: We hear a lot about AI in the insurance industry for better predictions and claim automation. But you approach AI from a different perspective. Can you tell us more about that?
[00:05:11] Michael Berger: Yes, sure. So, via our aiSure product, we’re really offering an insurance solution which covers this AI performance risk. We’re supporting and empowering AI service providers to promote robust AI applications, where we are taking over the performance risk of the AI. In case an AI system fails to deliver up to the promised specifications, then the users will receive a compensation payment. So, this is really what we try to do with aiSure.
[00:05:47] Thomas Dewey: So, that’s probably one of the products that our audience has never thought about. What would be a typical customer?
[00:05:55] Michael Berger: Our aiSure clients are companies who want to add trust as a feature to the AI service in order to increase sales. So, we have clients in a variety of verticals like in FinTech, legal tech, cyber threat mitigation, or manufacturing. We work with corporate clients, as well as with startup companies. For example, take one of our AI startup partners, Augury. Augury conducts machine health diagnostics based on machine learning models, trained on vibration data, temperature data, and other kinds of data. Munich Re provides insurance backing for a guaranteed diagnostics warranty. This means that in case a failure event would not be detected, we would pick up the repair and replacement costs for the monitored equipment. So, the users of the AI service get compensated in case of an AI failure. And again, this builds trust in the AI service of Augury and supports their sales.
[00:06:59] Thomas Dewey: Boris, getting over to you. This sounds a lot like the industrial-grade AI concept. What’s the concept behind that term?
[00:07:09] Boris Scharinger: the“Industrial-grade AI” term expresses our Siemens Digital Industries’ ambition to facilitate and deliver AI that meets highest standards in terms of robustness and transparency, and respectively, explainability. Just imagine you are using an AI component, for example, in one of our generative product design software packages, and there’s a certification instance that asks you to prove that the constructive part that you’ve developed using that module is more stable than your previous design meets certain error conditions. And your response would be, “Well, you know, I don’t know why, but AI said so.” It’s clear to everyone that diligence in product design can’t be based on such a statement. So industrial-grade AI is really about maturing AI to a degree that we feel comfortable with putting it on a shop floor or putting it in an important part of our product design workflows. And from our perspective, diligence in the development process is a core ingredient to arrive at industrial-grade AI. And – I think that’s important for our audience – it’s not just something that refers to the runtime environment, It is also about the development process. Both the development process and the operations of the AI solution must be tightened.
And then, there’s the second ingredient: To bake industrial-grade AI, if you will, which is partnership. Because good AI solutions require that AI experts, domain experts, and automation engineers, or design engineers join forces together; otherwise, AI will stay mediocre. That’s what we want to promote and to work at Siemens: embracing excellence from different parties, building bridges, bringing people together. And there are a couple of examples of how we do this: facilitating shared data pools across companies to get better training data and derive better AI models from it; facilitate collaborative labeling for industrial use cases, and so on.
[00:09:49] Thomas Dewey: That sounds like a pretty difficult challenge to solve. What kind of challenges do you see for companies adopting industrial-grade AI?
[00:09:59] Boris Scharinger: Well, it’s starting with a cultural thing. So, if you look at data scientists, data science is a pretty new art form. And the generation of data scientists that we see in practice, it’s a young generation. This young generation has little exposure so far to complex software projects, where software engineering, very tight processes, methodology, requirements engineering, requirements tracking, validation, and verification concepts – all of that plays a major role. And Tom, probably you know it by heart from your EDA background, these projects are super huge and tightly managed to produce quality.
Data science projects are lacking methodology, lacking tight processes so far, lacking formal specification. We need to work and build bridges between the kind of mature art form of software engineering and the very young art form of data science to help data scientists who work more in an explorative way to understand the needs for tight processes and methodology. So, quality becomes manageable in a sense.
[00:11:24] Thomas Dewey: Michael, do you agree with Boris’s assessment of the challenges for adoption?
[00:13:00] Michael Berger: I agree. I would also say that robustness of AI is really crucial. Building robustness into the design, that’s a key factor. And do you know what does robustness means? Robustness really means stability in performance. And achieving stability in performance is important because then we can put trust in the model that it consistently makes good decisions, even under small input variations. So, of course, this raises the question of how can we actually create robust AI models? I think, in the past, we had a business problem, and then we would gather data to solve it. And nowadays, we have a lot of big data already available, even before we even ask a question. So, I guess we are poking at the data and try to find some interesting stuff. We try out some models, and then we use whatever works on average. However, I think this leads to non-robust model designs.
I think now, academic research on mathematical and computational statistics is really catching up with those practical issues. I think research informs us more and more about robust model designs and ways how to estimate also the uncertainty in the predictions that the model gives. I believe that this research provides a strong basis for the design and the implementation of more robust AI applications. As an example of this research, take conformal inference and the work which is done by Emmanuel Candès at Stanford, as well as the work done at Berkeley. I think this work allows us, under certain specified conditions, to really design applications where we can trust the prediction performance with a high degree of certainty. And I think this is super exciting stuff. In my mind, this will really also lead a new wave of more robust applications. And at Munich Re, we want, via our aiSure product, to support such robustness claims of machine learning models and we also want to make it easier for decision-makers at companies to decide about the AI use case.
[00:13:45] Thomas Dewey: So, we all know that insurance companies safeguard risks, but they really don’t like them a lot. How do you assess the risk?
[00:15:32] Michael Berger: I would say we actually like risk quite a lot. At the end, it’s the core of our business model. For us, taking risk is ultimately a statistical question which is based on a profound understanding of the risk. For aiSure, we ask ourselves whether we can really estimate how robust a machine learning system is. So, in other words, can we estimate probability distributions or probability bounds for the predictions that the machine learning model is making? Our job is then to understand the robustness of the machine learning model in the respective application. Based on this understanding, we then estimate the probability of falling short, relative to the given promise, based on a suitable statistically valid testing regime. And to do this in the right way, we draw on statistical and mathematical expertise as well as domain experts within the Munich Re group. This is really the basis of our aiSure product.
[00:14:54] Thomas Dewey: So, when you’re taking a look at a customer solution, what qualities do you find really make a good AI solution within that company?
[00:15:04] Michael Berger: We think it’s really about the people. So, we want the tech team to be experienced and down-to-earth. So, not focusing on buzzwords, but really being technical and being rooted in mathematics and statistics. And we really want that good data science practices are followed, especially robust and statistically valid testing regimes are used. This is key for us.
[00:15:27] Thomas Dewey: So, Boris, this should sound a little bit familiar to you. Do you think that the Siemens Digital Industries’ industrial-grade AI would actually pass Munich Re’s checks?
[00:15:38] Boris Scharinger: That’s a great question, Tom. I think, to be honest, it’s a journey. We are ahead, certainly, in building industrial-grade runtime environments. For example, if we take our AI accelerator hardware, the TM NPU. It’s an AI accelerator chip, embedded in industrial casing, and it is fan-less, and it’s extremely robust; no problems with dust in a production environment, that is industrial-grade from the bottom to the top. But the challenge is certainly in the development processes of the AI components themselves. What we do is: we go two-fold. We push the topics robustness, explainability, and so on by research and training of developers. We do some research projects with the University of Cologne, on requirements engineering for machine learning based solutions, for example.
But we also pull quality by our ML ops command and control center. So, we have a unit within Siemens Digital Industries that’s really specialized in providing 24/7 operations of AI shop floor solutions. That command and control center is staffed with data analysts and data scientists. They are specialists in detecting data drift and responding to it that may be communicating with the customer that may be retraining the model and recalibrating the model. And we provide these services even for third-party models. So for example, for a startup that has a great algorithm, but maybe it’s not yet prepared to provide these types of monitoring and operations services 24/7, even globally. Whatever our MLOps Center operates, of course, needs to go through some diligence checks, so it is clear to us what the model does, what we monitor, and how we could timely respond to exceptional situations.
[00:17:49] Thomas Dewey: Like you said, it sounds like a journey, and Siemens is learning, and we’re learning together with insurance companies. And I’m sure we’ll come up with a solution that will meet everybody’s needs. But let’s turn to have a look at the future. So, Michael, where do you see AI going in the next three to five years?
[00:20:19] Michael Berger: We already see that AI is taking over more and more simple decisions, so this means simple tasks which can be done at scale. I think that in the future, more focus will also be put on robustness, rather than just increasing the average performance. And I would expect to see that more and more statistical theories will also be informing accurate design and will be informing robust design. For example, we might be moving away from models which give only point out predictions without including much information about the uncertainty. So, I think we are moving towards models which give interval predictions where we can believe that the true value, the true outcome will lie within this interval of the model with a certain probability. In addition, I would also expect that we see more applications which are not pure prediction tasks, but rather model causal relationships. And I think that there’s really a lot of potential in these areas. But I also believe that insurance like Munich Re will be actively involved in the opportunities and the risks of AI, and will also play a strong role in supporting the wide adoption of robust AI applications.
[00:19:28] Thomas Dewey: Boris, the same question for you, where do you see AI going in the midterm?
[00:19:34] Boris Scharinger: That’s an interesting question. And I guess my answer is slightly less algorithmic than Michael’s. I believe and hope that we will see AI mature and scale, of course, as Michael indicated. In the industrial space, it’s really a challenge, for us and for others, to create AI solutions that scale like a product. In most areas, we see AI solutions scaling like a project, and I need several individual projects for several different customers, even if the problem that needs to be solved is a very similar one. For example, in the B2C space, we see a lot of web services that use AI to translate a text document, and that translation AI is the same for anyone who uses it across many users and customers. So, I hope that in the industrial space, we will see things scaling too.
I also believe that we will approach what some people call the machine economy – so, AI helping machines to interact commercially with each other. We see now a lot of use cases where AI is built into a machine for predictive maintenance, but any commercial transaction that follows because there is a predictive maintenance being scheduled, for example, that is done independently. And the combination of things like blockchain and AI, different AI technologies, maybe knowledge graphs, and so on, soon puts us into a position where also the commercial aspects of a machine transaction will be handled and supported by AI. So, just imagine machines brokering their predictive maintenance provider by themselves, including the payment. Or maybe the insurance claim towards aiSure will be handled by the machines interacting digitally with the systems and some AI support. So, that’s where I see the future in midterm.
[00:21:46] Thomas Dewey: It sounds exciting, and I hope you guys can accomplish those things. So, Michael and Boris, thanks for shining a light on this exciting topic – industrial-grade AI. It’s something I’d never really thought about, so I learned a lot. I wish you both well in your efforts going forward. Thanks for talking.
[00:22:04] Boris Scharinger: Thank you, Tom. It has been a pleasure. And thanks, Michael, also for joining us.
[00:22:07] Michael Berger: Thank you, Tom. Thank you, Boris.