The future of CFD – Your 15 minutes free gaze into the crystal ball
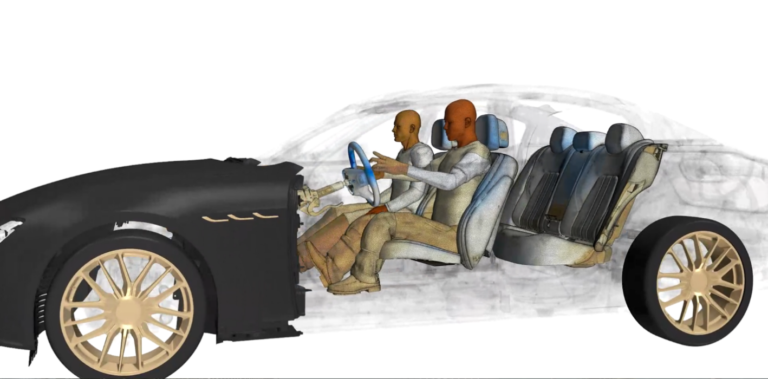
The following blog is based on a article originally published in NAFEMS’ special edition of Benchmark magazine to celebrate 100 years of CFD. We – as a software vendor were asked to provide our perspective on the future of CFD in the Vendor Viewpoint section. The blog at hand is limited to the Siemens perspective. For more insights into the future and the past of CFD please take a look at the complete edition.
We have partnered with NAFEMS to offer our readers a 50% discount on a year’s subscription. Read on to find out how to claim.
ChatGPT is turning the world (of coding) upside down while Computational Fluid Dynamics (CFD) codes are developing at a yet unseen pace. Machine Learning exercises are flooding the just freed-up GPUs from bitcoin mining while CFD codes start running productively on exactly those GPUs. A ship with thousands of cars is burning in the North Sea while the automotive industry is starting to embrace CFD to mitigate battery thermal runaway. Wildfires in southern Europe destroy forests at scale while a start-up company is in a hurry to built an innovative fire fighting aircraft. Oceans are getting warmer and warmer while engineers explore sub sea farming with the support of CFD simulation. Countries ban fossil-fuel based propulsion system while the race for alternatives at scale is in full swing. Europe is forging ahead to achieve a hydrogen based industry economy while making efficient use of such technology is far from trivial…
The world is changing at an ever increasing pace. At the same time the clock of climate change is ticking. It will require significant social and economic efforts to overcome the resulting challenges. However, if humankind manages to cope with climate change, it is also clear that innovative engineering will have played an essential role in this achievement. And as the (non)emission of CO2 and related climate gases is at its heart governed by the fluid mechanics and thermodynamics of reacting flows, it is ultimately also a major job for Computational Fluid Dynamics (CFD). The race is on and so the future of our species is more tightly coupled to the future of CFD than one could imagine.
But that being said – what will the future of CFD look like? We have dared to make a prediction.
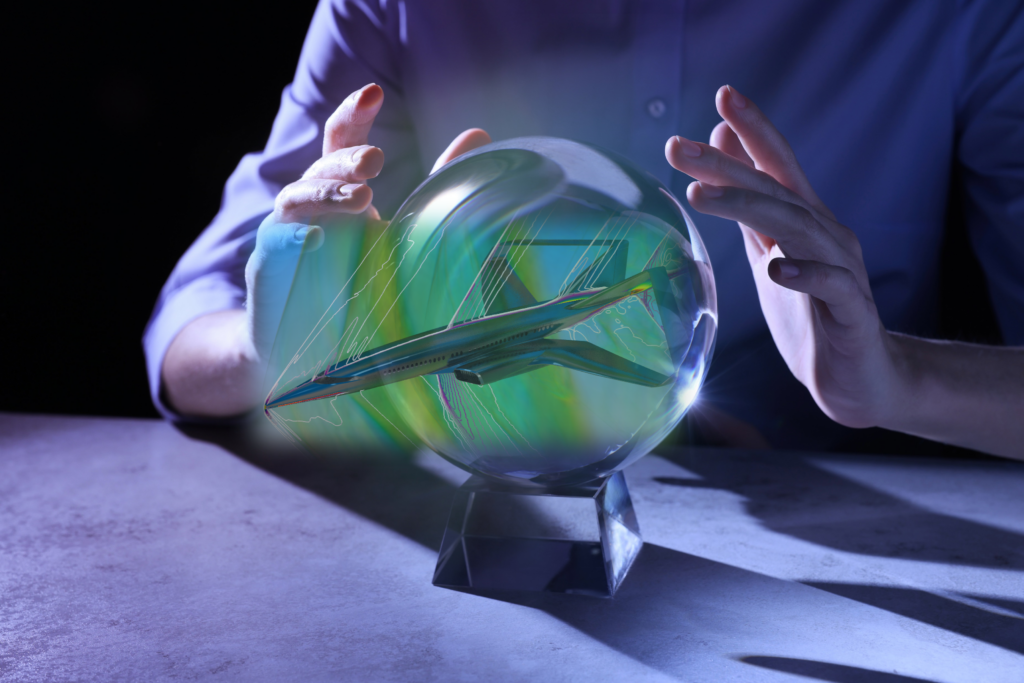
Prediction is very difficult, especially if it’s about the future!
Nils Bohr
It goes without saying that this is by no means a guaranteed roadmap for Siemens’ CFD products. It is my and my colleagues’ subjective extrapolation of the status-quo and trends one obtains in the CFD community, its research and surroundings.
What will the future of CFD look like over the next 20 years?
Will we still be using mesh‑based methods? How will meshes be created?
Meshfree CFD codes offer an appealing alternative approach to mesh-based methods for selected applications. When rapidly getting results is a priority over the highest accuracy, Smoothed Particle Hydrodynamics (SPH) is and will remain an efficient tool. However, there will not be a silver bullet CFD method. Even more than today, the future will consist of a blended, hybrid coexistence of mesh-based and meshless approaches. Automation of meshing and the generation of high-quality meshes with minimum user effort will continue to evolve.
Technologies like model-driven adaptive mesh refinement will further develop and allow us to meet required accuracy levels with minimum user intervention. The same is valid for particle-based SPH methods, where automated and intelligent resolution refinement will become an established methodology. AI-driven mesh generation may be another future topic. Clever approaches to change between mesh-based and mesh-free treatment might become a reality as CFD codes absorb all technologies into one piece of code.
And yet there will not be a one-size-fits-all CFD meshing solution but an automated, intelligent, and adaptive choice of the most suitable method for a given problem. In any case, any suitable approach and meshing technology will be faster than today’s solutions. Ensuring high fidelity while reducing engineering time for simulation set-up and hardware configuration and access are an important driver for the continuous investment in CAE solutions at Siemens. This will enable CFD engineers to model the complexity of today’s products with adequate meshing technology while staying integrated into a single CFD simulation environment.
How will turbulence be handled?
Like for meshing or meshfree approaches, no different from today, there will not be a single solution in turbulence modeling. Turbulence modeling will still be based mainly on a combination of statistical modeling Reynolds Average Navier-Stokes (RANS) and scale-resolving simulation (SRS), like large-eddy simulation (LES) or Detached-eddy simulations (DES). Some improvements in both methods will likely come from a combination of physical assumptions and machine learning (ML).
The major challenge will remain the same, accurately predict unsteady turbulent flows with the determination of separation. And as RANS alone will be unlikely to cope with this challenge in a fully predictive way, while LES remains rather computationally expensive, a difference compared to today will be the way the two approaches are combined: either in a segregated manner, where they are the most appropriate or in conjunction, where the local solution of the SRS models will inform, locally, the statistical model (e.g., via Machine Learning).
As engineers increasingly leverage such AI-based model choices in CFD simulation, they must have a sound understanding of those models and clear visibility of which model a simulation uses, when, and where. Despite the many benefits AI may bring, due to the nonlinear nature of turbulence, this field will require critical judgment through engineering or researcher experience and strict validation.
With continuously increasing computational power, high fidelity models, LES, or Direct Numerical Simulation (DNS) will further get into industry space where appropriate and dominate most of the research in turbulence. However, engineers will continue using the most efficient solution to answer the engineering question. Hence RANS will remain the state-of-the-art industrial CFD approach to go faster unless results prove it has hit its limits to solve a given turbulent flow problem.
Will the future of CFD mean running in real‑time?
Going faster is the precondition to exploring the possibilities to find innovative flow solutions and modeling the complexity of products with the required high fidelity for predictive engineering.
While real-time CFD is the ultimate ideal, overnight runs will still play their role in this high-fidelity design space exploration engineering for some time. Compared to today, such simulations’ sophistication level will increase as engineers create even more comprehensive multidisciplinary digital twins in response to more complex product requirements.
At the same time, as the industrial metaverse evolves, the need for rapid, real-time fluid dynamics predictions will become a new application class. AI and low-fidelity physics approaches will play a significant role in this ambitious goal, just like new hardware: Quantum computing would be the ultimate disrupter in that respect. Until then the continued exploration of intelligent speed-up technologies and leveraging next-generation CPU (Central Processing Unit) based HPC (High-Performance Computing), GPU (Graphics Processing Unit), ARM and low-latency cache technology, will be of paramount importance to get closer to real time fluid dynamics simulation.
Which is the ultimate method for the future of CFD?
In conclusion, neither for meshing/modeling nor for the general technology of fluid mechanics predictions will there be a single methodology to serve all needs. Like today, but on a more diversified range, there will be a spectrum of methods to choose the most appropriate compromise between required fidelity and response time. Methodologies may range from “traditional” high-fidelity CFD methods, through rapid meshfree simulations like SPH, to AI-supported fluid dynamics prediction. For simulations, AI may be a supportive force in modeling choices, but engineering expertise and judgment of CFD results will remain essential. However, with the continuous embedding of this breadth of CFD technologies into unified platforms/code environments, engineers can stay integrated while making the best possible simulation choices and going faster. Finite volume, SPH, Lattice Boltzmann, they will all survive and coexist in a more integrated fashion, each offering a valuable solution for a specific engineering challenge
What will be the big trends in CFD for the coming 20 years?
Accessibility of CFD
The offering and usage of cloud-based CFD solutions will massively increase. With the flexibility and scalability of SaaS business models, companies of all sizes and individuals already today have instant access to the exact amount of hardware and software needed at a given time for their specific CFD project. Pre- and post-processing will move away from workstations and into a web browser.
The recently released Simcenter Cloud HPC by Siemens allows you to run your CFD simulations on optimized hardware configured and managed by Siemens, using the underlying Amazon Web Services (AWS) infrastructure.
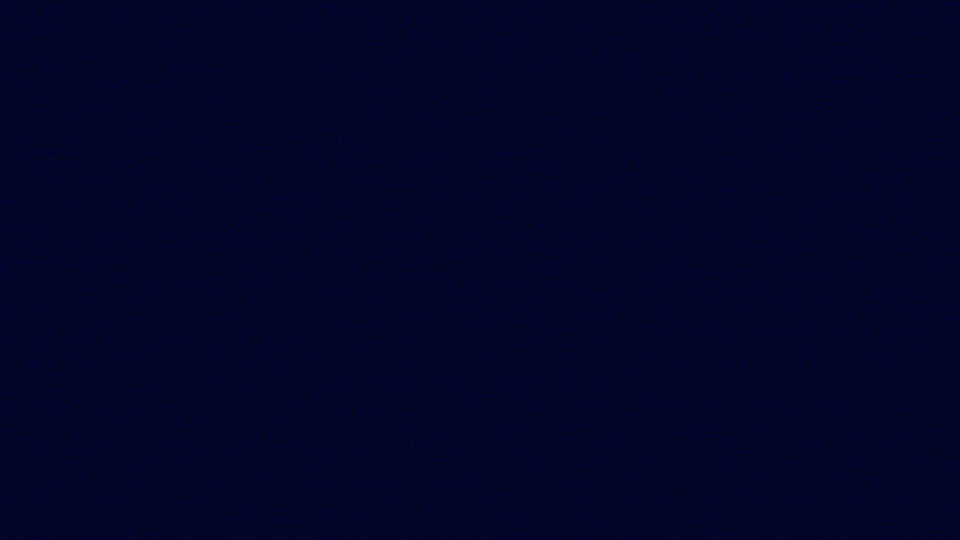
Moving a simulation to the cloud takes as little as two clicks of the mouse, without leaving the Simcenter STAR-CCM+ user interface, and jobs can be monitored through a web browser on any device. Pre-paid credits cover the cost of hardware, software, data transfer and storage. This results in a single pay-as-you-go charge for each simulation run which greatly simplifies budget management for businesses of all sizes. This trend will continue, cloud technology will further evolve, and such offerings will become an increasingly important strategy for companies needing flexible access to CFD.
Democratization of CFD
The barriers to high fidelity CFD will further decrease on all levels. Cloud-based offerings (see above) will grant anyone with some device and browser access to CFD software and the required hardware. Modern CFD solutions will leverage client-server technology with its decoupled backend (solver, physics) and front-end (GUI, pre- and post-processing, automation). Such architectures enable the independent creation of dedicated, tailormade app-like front ends for specific applications using low-code approach, or natural language processing front ends opening up CFD to new end-user bases. CFD experts will be able to deploy these app-like front ends by incorporating and maintaining best practices with minimum effort.
Novel input and output device technology (touch pads, Virtual Reality (VR), Augmented Reality (AR), ..) will make it easier to both setup and explore simulation results.
All these User Experience (UX) enhancements will further open up the usage of CFD to non-CFD analysts at a much broader scale and more regular basis. An increasing number of designers and application-focused (non-CFD expert) engineers will leverage predefined simulation methodologies. The industrial metaverse may add another whole new user class for fluid mechanics simulation, further extending the audience and user base for CFD simulation to an even younger generation on a broader educational level than just engineering or research.
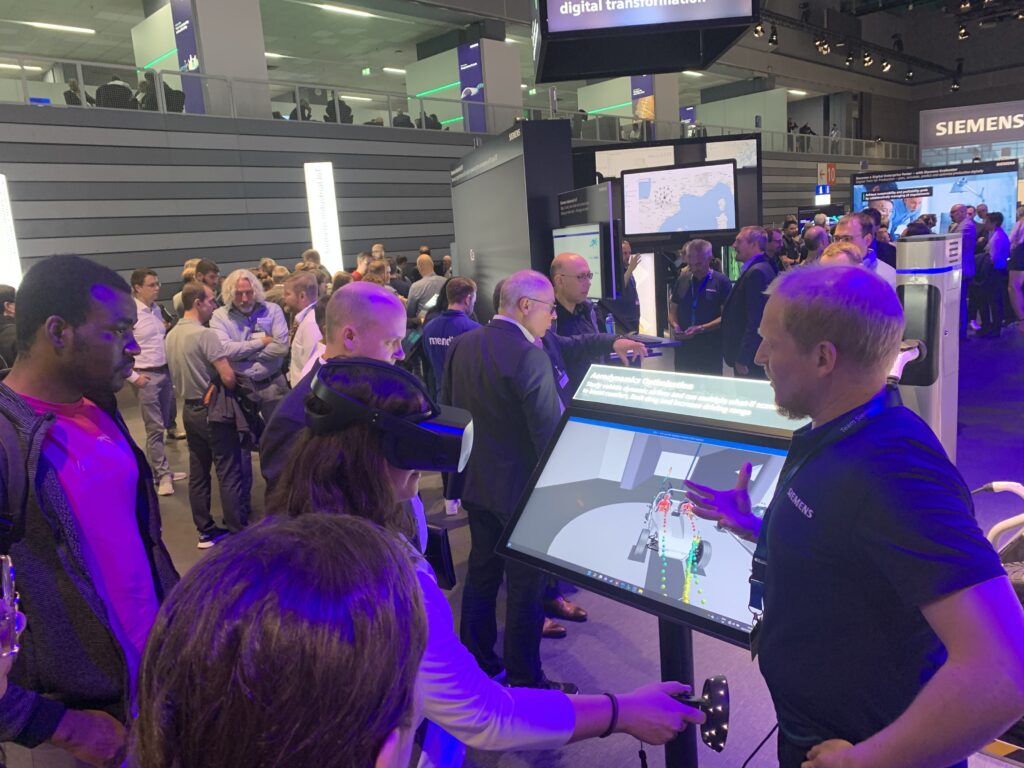
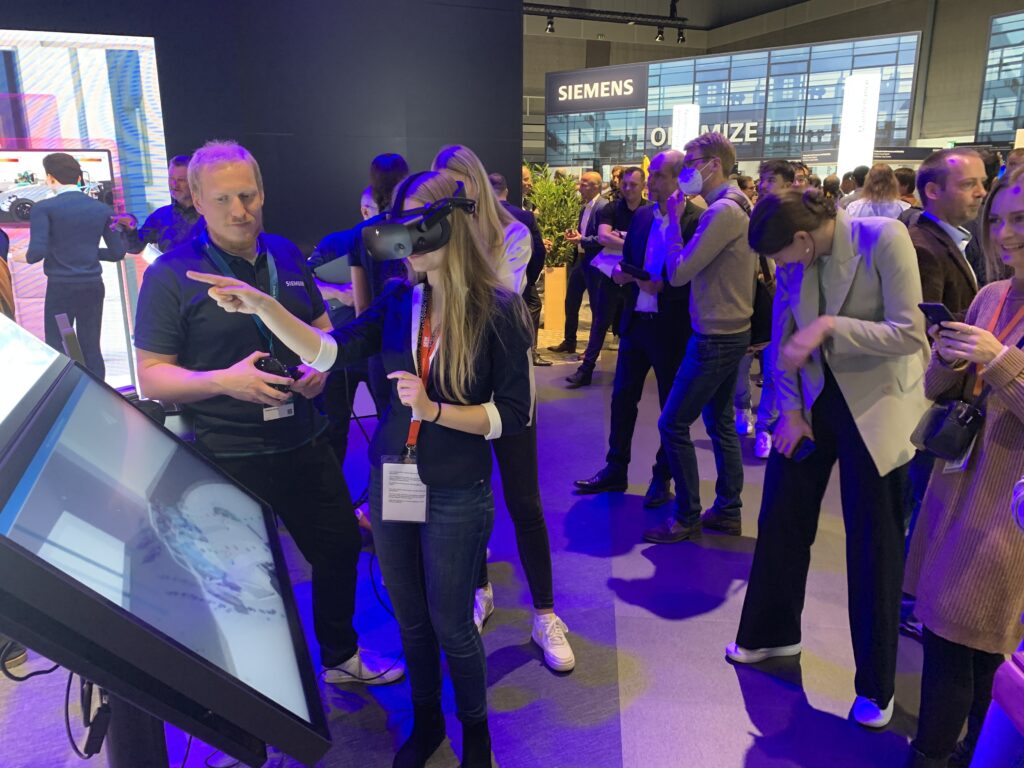
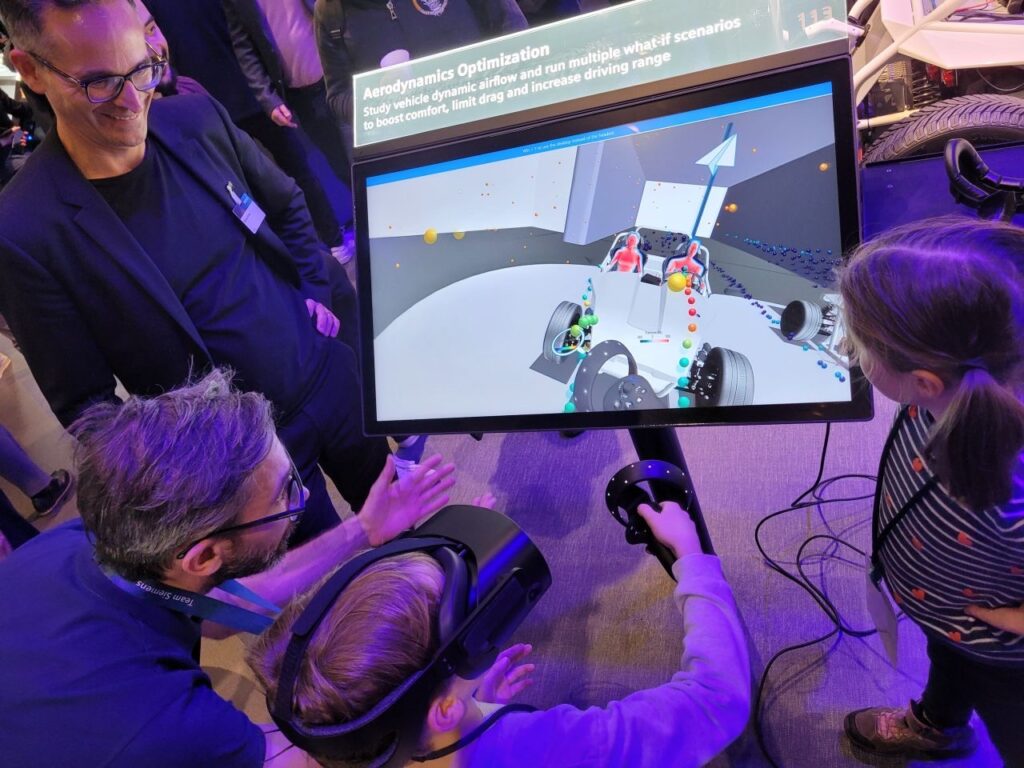
The future of CFD and Digital twins
A Digital twins offer the ability to replicate the real world realistically. They are a vital technology for the metaverse where the real and digital worlds will merge almost seamlessly. Not only in the industrial metaverse can we expect that CFD plays a significant role in the creation of holistic digital twins. With the increasing breadth of simulation (not just CFD) methodologies, the Digital twin of a product will consist of a range of models to represent the product in various stages of fidelity, from real-time-ready representations for the metaverse to very accurate representatives for close-to-production and validation purposes.
As such, managing digital twins with their instances of different levels of realism through Product Lifecycle Management (PLM) solutions becomes increasingly important. Engineers can leverage adequate model fidelity for a given engineering challenge- and this may even be a process supported by AI. The overall fidelity of a digital twin will further increase by modeling the complexity of today’s products across engineering disciplines in an integrated manner. CFD will be further embedded into a seamless ecosystem of multidisciplinary and multiscale simulations. Thanks to increasing computing power and novel simulation methodologies, real-time digital twins will become a significant game changer in unforeseen ways – even for comparably complex fluid dynamics problems.
Machine learning and artificial intelligence augmenting CFD
AI has and will continue to transform technologies in every industry. Applying AI to CFD applications will become an increasingly strategic asset to companies, as it can help reduce costs and create new differentiated values. AI-driven innovative solutions offer substantial benefits to CFD engineers, designers, and analysts.
AI embedded into graphical user interfaces is already starting to simplify setup procedures and mitigate errors. AI-assisted model preparation, like CAD/geometry preparation through intelligent part recognition and model setup through natural language processing, is becoming the norm because it significantly speeds up and simplifies the CFD setup procedure.
It can reduce computational, design program, and operational costs by offering the ability to assess more designs per simulation at a faster turnaround time. Furthermore, AI allows to reduce the process and program development turnaround time with ML-based surrogate models and intelligent AI-driven workflows to expedite turnaround time. It can enhance the accuracy of simulations by flagging anomalies and providing knowledgebase workflow assistance in the CFD process. This includes CAD, physics modeling, mesh settings, and post-processing.
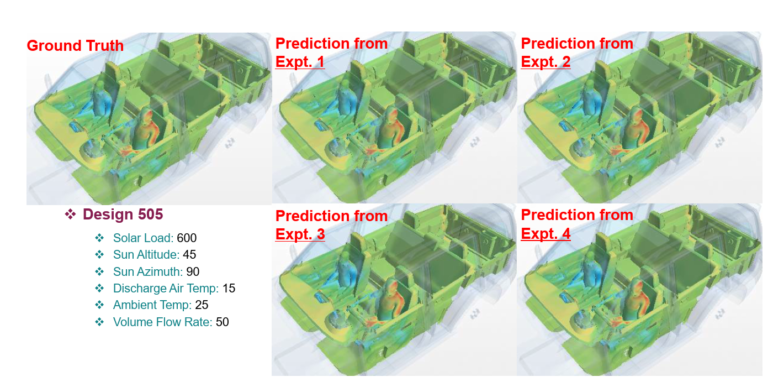
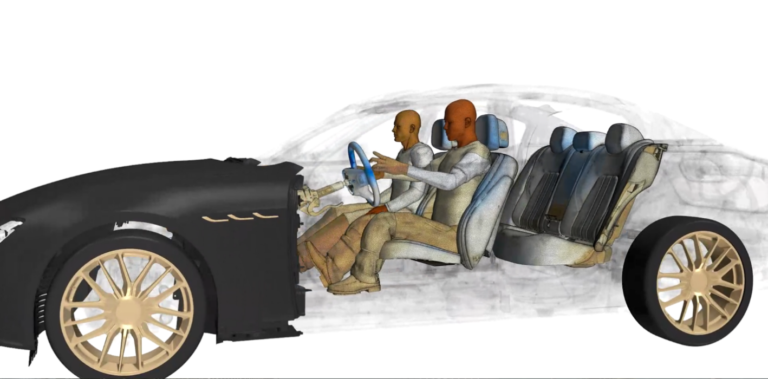
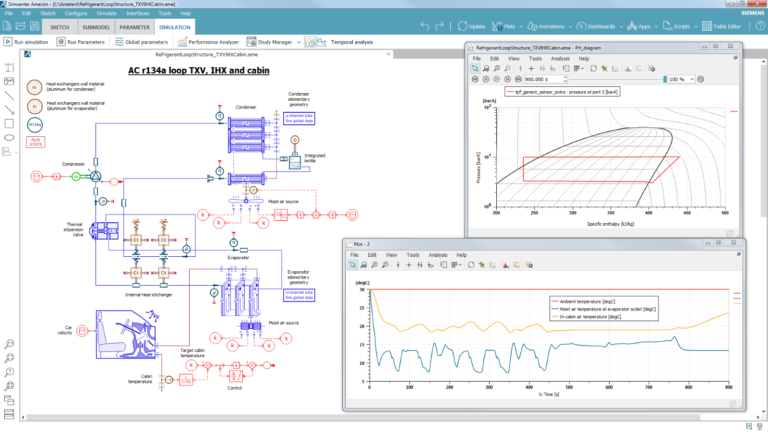
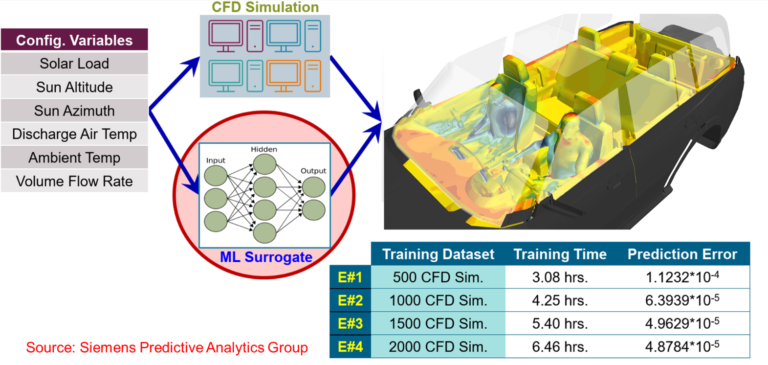
In conjunction with CFD, AI will improve product performance and efficiency by creating an ecosystem to simulate, predict, and seamlessly optimize products. Providing knowledgebase workflow assistance, AI will further lower the barrier of setting up meaningful CFD simulations faster.
But despite those benefits potentially offered by AI/ML, well-educated engineers will remain a critical factor in method development and assessment. In that sense, considering AI as the solution to every fluid dynamics problem is naïve. The nonlinear nature of fluid mechanics, well captured by Navier-stokes equations and established turbulence models, will pose a challenge to AI. On top of assessing data quality, appropriate data selection and validation to train AI and machine learning algorithms require engineering and AI knowledge. Delivering AI capabilities in CFD designs and simulations requires talent in machine learning, deep learning techniques and CFD skills.
Hence for the successful adoption of AI in CFD, it will be of paramount importance that software vendors deliver integrated solutions and consulting services for CFD and AI, something that Siemens is already doing and continues to invest heavily.
General‑purpose CFD codes vs. application‑specific CFD codes?
There is no one-size fits all solution, but the trend of functional integration to cope with specific applications in general-purpose tools will continue. For that, general-purpose solvers will continue to absorb simulation technology into one CFD framework or offer seamless open interfaces for co-simulation with specific standalone solvers. Ultimately, application-specific technology in general purpose tools will become more and more directly accessible thanks to the increasing ability to create low-code apps for tailored application-specific front-ends.
What will be the (development) challenges for CFD in the coming 20 years?
Methods of CFD
From a development perspective, ensuring a sustainable CFD framework is necessary to keep a code alive for a maximum period. Modern architecture is a precondition, just like project-oriented programming. The demands of handling code complexity rise as more multidisciplinary simulation technologies and solver capabilities get absorbed into unified code platforms offered on the cloud. All this requires proper development tools and strategies, product management skills, and continuous intelligent investments into software development.
A new challenge results from setups with the help of AI/ML as automated modeling choices pose a risk of becoming opaque to the end user. Hence, modeling approach changes on the fly need to be reported, allowing engineers to assess AI choices and, ultimately CFD results. This re-emphasizes the fundamental importance of engineering and CFD know-how despite or because of AI progress.
Another challenge comes with the amount of data that is being created as more and more simulations can be run in the same amount of time. Being capable to handle and investigate these vast amounts of datasets stemming from a wide range of sources, is of paramount importance to not limit the value of CFD for making the best possible engineering decisions. Hence both PLM and data postprocessing systems must be prepared for big data management and to support engineers in their analysis. Parallelization of pre- and postprocessing is hence equally important to the parallelization of the calculations themselves.
CFD in a multidisciplinary engineering world
The demand for higher fidelity in the digital twin results in multiscale and multidisciplinary modeling requirements. Models need to become more accurate, more detailed, and interconnected.
Quantum, Molecular, Micro, meso, and macro scales need to be considered, and yet at the same time, those complex models should run faster. This will be a continuous challenge asking for clever techniques like, e.g., hybrid multiphase modeling.
Computer hardware/resource
Generally, the introduction of new hardware architectures and technologies may require the adjustment of CFD algorithms. A light version of this transition is the current porting of solvers from CPU to GPU. As supercomputers may consist of more and more heterogeneous architectures, combing multicore GPU and CPU, with shared memory, hierarchical networks etc. modern CFD codes will need to be prepared for a multitude of architectures, with algorithms and architectures that are leveraging the best of each world.
But this is minor compared to what development teams will be facing when the disruption from von Neumann to Quantum Computing (QC) occurs. In principle, quantum computers are tailormade for simulation in many respects. The potential is enormous. However, introducing QC will mark a true disruption for any CFD code as it will require a completely new algorithm implementation. In QC, nothing will be portable from traditional von Neumann architectures. In other words, QC requires a complete code rewrite of every current CFD software. To add to the challenge, while powerful and applicable in many CFD simulation areas, QC is not useful at all for other CFD-related tasks, while traditional von Neumann architecture is. So, the only efficient solution is a hybrid solution. This, in return, will require developers that could cope with either architecture. To date, the classic path to CFD code development is mostly that someone with sound physics understanding comes in and learns the programming part. And while object-oriented programming is comparatively easy to learn, this will no longer be true for QC algorithm implementation. As there is no compiler assistance in QC, it requires developers that can write QC-able code requiring deep knowledge of such algorithms.
Another aspect that will become more and more important is power consumption of computing hardware. As the trend to leverage massive compute power for CFD continues, cost associated to energy consumption becomes a significant factor. Hence, new – energy efficient technologies like reduced instruction set computers (RISC) play an increasing role in the positioning of time-to-solution vs. energy-to-solution to achieve optimum cost-to-solution.
Engineers / human resource
Educating future engineers is a crucial challenge. All other challenges can be overcome or minimized if the educational system provides industry and research with the next generation of CFD engineers. But those engineers can no longer be purely CFD analysts in the traditional sense. Novel manufacturing technologies (like AM and 3D printing) and closer connectivity between CAE-and -CAD departments are necessary for companies to stay competitive. Hence the profile of CFD engineers needs to include some understanding of manufacturing and design processes, just like designers need to start embracing simulation technologies. As AI penetrates the fluid dynamics engineering domain, the future CFD engineer needs a thorough understanding to make the right decisions on the most efficient technology for a given problem. The progress of CFD simulation heavily relies on this factor.
Generally, it is a society and industry challenge to ensure (CFD) engineering and computer science is an (even more) attractive career path for the next generations. Talented and well-educated engineers are crucial for humanity to cope with the challenges of climate change and its consequences.
Despite all democratization, it is of fundamental importance that engineers have a sound education. Despite all new technologies, like, e.g., AI, for those engineers that make critical design decisions based on CFD simulation, understanding the fundamentals of fluid mechanics and physics will remain critical.
Which single one technology will have the biggest impact on CFD in the next 20 years?
It is difficult to name just one. It will be a mix and the synergetic interaction of various technologies.
Cloud technology and the integration of CFD into the industrial metaverse will change how CFD is used and leveraged. Related further democratization and increased accessibility will significantly impact how CFD software evolves and is used to solve engineering problems. Along with this, we will see a growing infrastructure for real-time co-creation and collaboration in the engineering design space. This will further spark innovation through yet unseen abilities of cross-pollination between international and interdisciplinary teams.
AI and ML will be another game-changer, as discussed above.
Additive manufacturing (AM) has just opened a new way of bringing product designs to life that are no longer constrained by traditional manufacturing methods. Leveraging CFD-based topology optimization, engineers can explore the possibilities for designs of uncompromised high-performance flow solutions. While their production had been entirely unthinkable in the past, AM and 3D printing are opening the door for this new engineering era. AM and topology optimization make the flow the actual product designer, putting the traditional design, simulate, refine loop upside down.
The ultimate disruption will, however, result from the successful introduction of Quantum Computing into the (CFD) simulation space. Going faster by orders of magnitude with (CFD) simulation will change the pace of innovation in yet unseen ways.
How will we ensure that simulation predictions are fit for purpose?
CFD verification and validation
Certification and verification processes for CFD simulation tools are well established. They will remain a critical ingredient to the progress of CFD, its reliability and trust in digital twins, and its establishment in novel areas. While predictive simulation will continuously reduce the need for expensive measurements and prototyping, it will continue to require rigorous CFD methods and best practices validation through experiment.
Compared to “traditional” CFD simulation, AI / ML-based fluid dynamics prediction will require significant validation. Due to the lack of the abstraction level, i.e., a governing equation, analytical description, etc. AI and ML need engineers’ judgment to probe for critical areas outside the training range in certification processes.
What will be the application areas where CFD might contribute most in 20 years?
Whatever we humans do in our daily lives, from a technology standpoint, the (non)emission of CO2 and related climate gases is at its heart governed by the fluid mechanics and thermodynamics of reacting flows. In almost any industry, it will come down to solving a thermodynamics and flow problem, be it on the reduction, the complete mitigation, or even the proactive reduction of already emitted CO2. And that implies the extensive usage of CFD to do this on relevant time scales and within economic constraints.
Whether it is energy production, transport, infrastructure, or production, fluid dynamics simulations at unthinkable scales will be required to find sustainable solutions as fast as possible. And hence there is no single application or industry where CFD will have the most significant impact;
it will shape a more resource-efficient way of living across the board. And even if humanity will not manage to stop climate change and must face an adoption to new environmental situations, fluid mechanics will be of paramount importance.
What’s the future of CFD in the next 50 years?
Suppose it’s not happening in 20 years. In that case, it may occur in 50: Any yet not foreseeable hardware technology disruption, like quantum computing, that will lift parallel computing onto a new level would mean massive implications on CFD and its impact on engineering.
What about a 100 years?
Undoubtedly, the Navier-Stokes equation is a brilliant piece of math and physics. Given its impact on the way we can predict the motion of fluids and based on that engineer products, it is an outstanding success. But deep down there we all know that it remains an approximation. Describing the motion of fluids as a continuum is after all a non-analytical description of reality and hence from a theoretical standpoint poses the risk that we miss fundamental elements that result from the complex interaction of billions of molecules.

In all honesty, we often forget about this approximation, which however is both an obvious and impactful one. No doubt, his was the historical achievement of Navier: merging the pragmatic hydrodynamics engineering world with the theoretical fluid dynamics through the introduction of a viscosity concept. An ingenious idea. But the fundamentally underlying continuum assumption for fluids forced us to introduce concepts like turbulence and viscosity to overcome the deficiencies in the Navier-Stokes equation and make it of some practical usage.
Now, obviously with sufficient computational power one could solver Newton’s equation for each and every molecule and provided we know the intermolecular forces predict the bulk motion. Today’s Computational chemistry codes do exactly that or even go beyond length and time scales where quantum effects become relevant. But even within the next 100 years it might be a bit naïve to expect this a feasible solution for external aerodynamics of a car.
But, what if some day in the coming 100 years someone comes along, with something that fits in between, that closes a gap between molecular dynamics and continuum descriptions, something that absorbs Navier-Stokes into a more generalized, more complete, and more powerful description of fluids putting the description of turbulence on analytic grounds rather than modeling attempts…
We will never be able to predict the future, but until then we can predict the flow!
Made up by myself
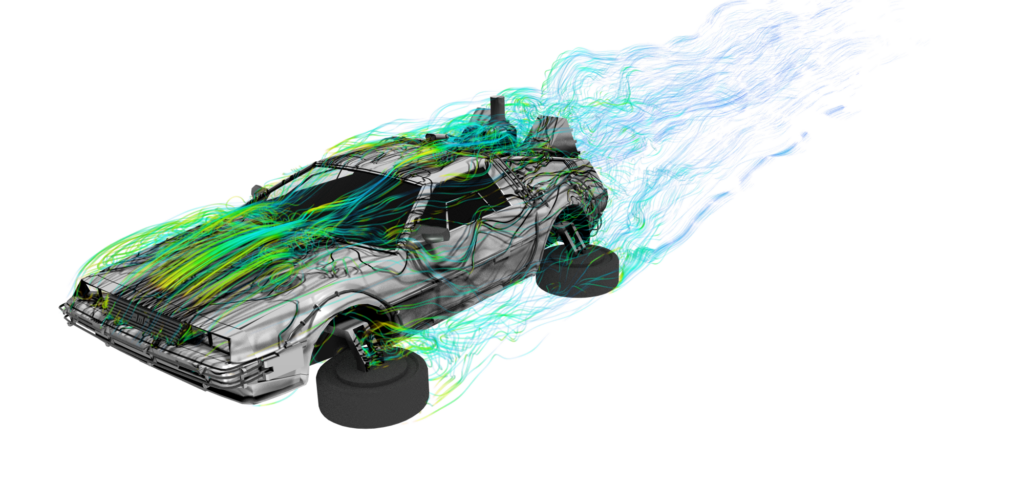
Acknowledgement
I would like to thank all the people that helped to gaze into the crystal ball for the future of CFD through great and insightful conversations, comments and their publications: Patrick Niven, Boris Marovic, Jens Prager, Bastian Thorwald, Samir Muzaferija, Christina Kothlow, Justin Hodges, Sylvain Lardeau, Ravi Shankar and Jean-Claude Ercolanelli.
Exclusively for our Siemens blog readers, NAFEMS is offering 50% off a 1-year subscription to benchmark magazine: sign up at https://www.nafems.org/publications/benchmark-magazine/subscribe/1year/ and use the code SIEMENSCFD at the checkout.