How Simcenter Amesim helps you in fast battery aging identification from experimental data
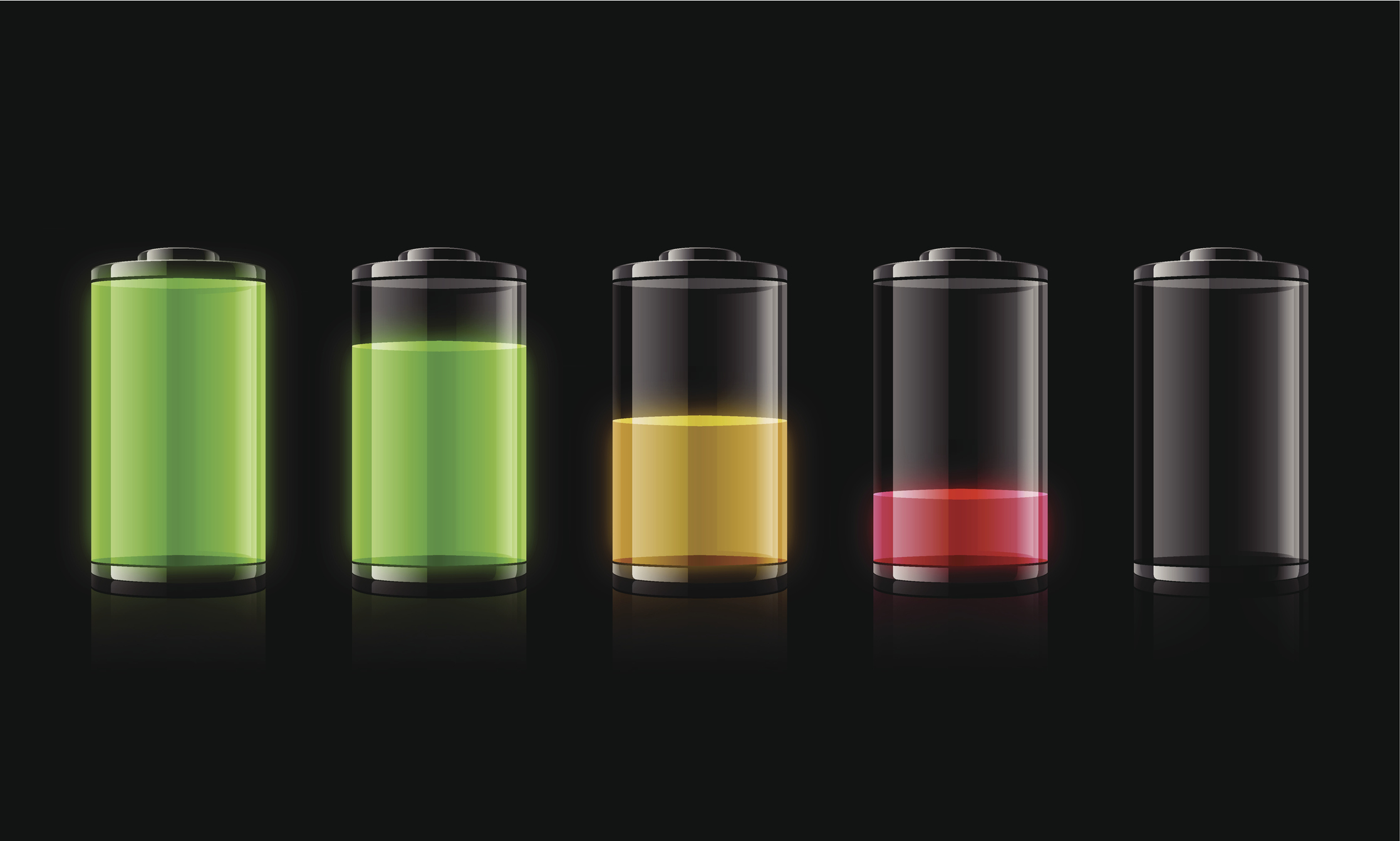
Introduction
Electric vehicles (EVs) have gained widespread popularity in recent years due to their environmentally friendly and cost-efficient nature. However, as with any battery-powered technology, battery aging is one of the most critical issues to be considered during battery pack design for electric vehicles. Battery capacity decreases during its usage, which results in a range reduction of the vehicle.
To predict the battery capacity loss over its lifespan, different modeling approaches can be found in the literature: the physical approach and the empirical approach. Both have their pros and cons, compared to the physical approach, the empirical approach does not require a full understanding of the aging mechanism. Only aging test data are enough to calibrate the empirical aging law.
Physical approach
Based on electrochemical model
Empirical approach
Based on equivalent circuit model coupled with an empirical aging law
Pros
Method gives insight into different degradation mechanisms
Pros
Only aging test data to calibrate the aging law needed
Cons
- A deep understanding of the aging mechanism is required to calibrate the aging law
- The degradation mechanisms are hard to fully comprehend
- Additional experimental test efforts are required
- Aging test data is needed
Cons
- Method has little connection with degradation mechanisms
- Aging test data is needed
Table 1: aging modeling approaches
While the calibration of the battery equivalent circuit model is relatively straightforward, the calibration of the empirical aging law comes with two challenges:
- Different batteries have different aging behavior. When a given empirical aging law works fine with one battery, it may not be suitable for others.
- The calibration of the empirical aging law from aging test data requires important engineering time and effort.
To overcome these challenges, Simcenter Amesim 2304 comes with the Battery Aging Identification Tool. This new feature allows users working on battery-powered systems to:
- Get a customized empirical aging law suitable for your battery
A generic aging law is used in this tool and its parameters are self-adaptable to new mechanisms. For example, low-temperature aging mechanisms are automatically considered if related aging test data is available. - Calibrate the empirical aging law
Step by step support use aging test data to calibrate the empirical aging law
The new Battery Aging Identification Tool
The new Battery Aging Identification Tool has a user-friendly and graphical workflow to quickly use the available data and convert it into empirical aging models.
- The tool takes battery experimental aging test data as input.
The aging test data corresponds to the battery capacity loss over time at different test conditions (e.g., different states of charge, different ambient temperatures, and different currents). You can get the aging test data by performing experimental tests on battery cells. - The tool provides you with a step-by-step guide through the identification process.
This process includes different steps such as the data import, the identification of the aging law, and the export of the aging law to the battery model. - The output of the tool is the identified aging law which is an expression in function of state of charge (SoC), C-rate and temperature. You can send the aging law directly from the tool to the battery model in Simcenter Amesim.
The generic empirical aging law identified by the tool is described by the equation below:
Where:
- Qloss is the relative capacity loss in [%].
- Bcoef is the aging prefactor B [%/year]. It depends on SoC, C-rate current and temperature.
- Zcoef is the aging exponent Z [null]. It depends on SoC, C-rate current and temperature.
- tyr is the aging time [year].
Bcoef and zcoefare polynomial expressions self-adaptable to the input aging test data. More detail about the generic empirical aging law can be found in [2].
Case study
The case study is about the aging law identification for a Li-ion NMC/C battery cell with 26 Ah of nominal capacity [2,3]. The case study includes two experimental test campaigns:
- Calibration test campaign: to collect datasets for aging law identification with the Battery Aging Identification Tool.
- Validation test campaign: to collect datasets for the validation of the identified aging law at PHEV (plug-in hybrid electric vehicle) conditions.
Calibration test campaign
To calibrate the empirical aging law, a calibration test campaign has been performed in the laboratory. The aging test campaign included both calendar and cycling aging tests as shown in Figure 2. Over 90 cells were tested over a year. At least two or three cells were tested for each condition. For each cycling test condition, the cell was cycled around a mean SoC in a +/-5% window. Using the test results from this aging test campaign, an empirical aging law was identified with the Battery Aging Identification Tool.
Validation test campaign
To validate the identified empirical aging law at realistic PHEV conditions, an aging test with repeated WLTC profiles at varying temperatures over a year has been performed on a module with 12 cells in series (12S1P) in Figure 3.
For this validation test, a scenario of daily use was chosen which includes 4 WLTC profiles, followed by a fast charging and a 4-hour rest period. A full cycle equivalent to one day use lasts 6 hours. The ambient temperature profile during the validation test reflects the temperature of the city of Nice (France) in 2015 with an acceleration factor of 4, thus, 24 hours correspond to a 6-hour test period. An offset of 5 °C was also added to accelerate aging.


The battery cell model with the empirical aging law is placed in the sketch in Figure 6 to simulate the battery aging during the validation test.
Figure 7 shows a comparison between the experimental and the simulated capacity losses. The simulated capacity loss shows good agreement with the experimental data.
Conclusion
To learn more details of this case study, please refer to the demo of “Identification example with the Battery Aging Identification Tool” in Simcenter Amesim [4].
Discover all the new features of the Systems 2304 release in this blog post.
Reference
- How to accurately identify the battery model parameters while saving engineering time, Siemens
- R. Mingant, M. Petit, S. Belaïd, and J. Bernard, “Data-driven model development to predict the aging of a Li-ion battery pack in electric vehicles representative conditions,” Journal of Energy Storage, vol. 39, p. 102592, Jul. 2021, doi: 10.1016/j.est.2021.102592.
- R. Mingant, M. Petit, A. Li, G. Autefage, “Battery Aging Identification Tool, a new Simcenter Amesim feature on electric storage library,” Simcenter Amesim User Conference, Lyon, France, 2023
- Identification example with the Battery Aging Identification Tool, Demo in Simcenter Amesim: qthelp://lmsimagine.lab/ame_dir/demo/Libraries/ESS/S08_Using_Battery_Aging_Tool.html