Adapting to a new era of AI with Justin Hodges and Remi Duquette (Series 2 Episode 4)
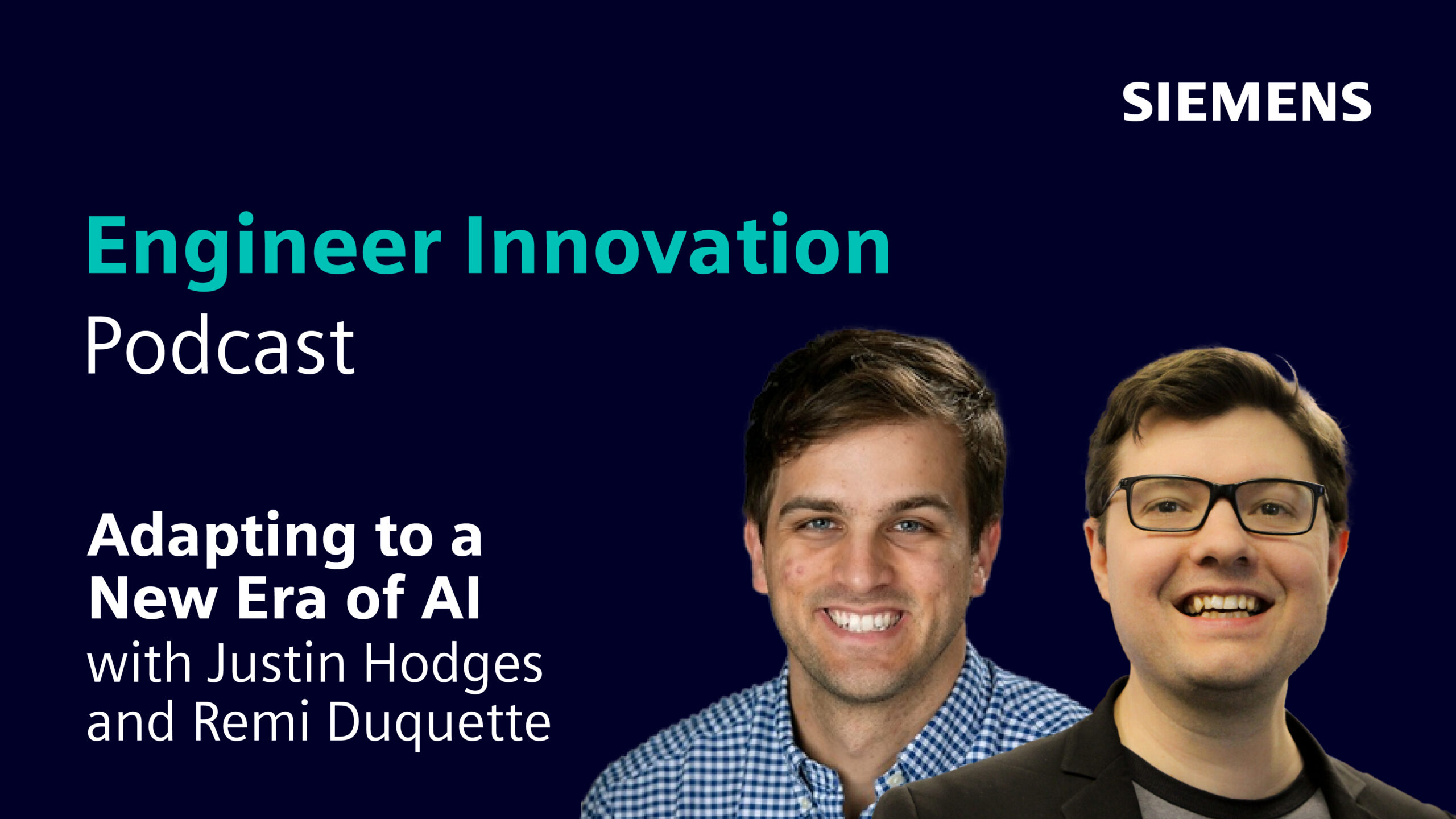
On today’s episode, we’re joined by Justin Hodges, Senior AI/ML Technical Specialist in Product Management at Siemens Digital Industries Software, and Remi Duquette, Vice-President of Innovation and Industrial AI at Maya HTT.
We talk about:
- How ChatGPT has changed public perception and understanding of AI.
- How both Justin and Remi found their way into AI, and their journeys so far.
- Whether AI is accessible for people without much prior experience.
- Some of the best examples of AI and ML today.
- The value of digital twins.
- The importance of data quality with AI.
- How AI and ML can help us explore innovation.
- Will AI replace engineers?
- How can organizations start implementing ML and AI in their workflows?
This episode of the Engineer Innovation podcast is brought to you by Siemens Digital Industries Software — bringing electronics, engineering and manufacturing together to build a better digital future.
If you enjoyed this episode, please leave a 5-star review to help get the word out about the show.
For more unique insights on all kinds of cutting-edge topics, tune into siemens.com/simcenter-podcast.
This podcast is available to watch here.
-
How ChatGPT has changed public perception and understanding of AI.
-
How both Justin and Remi found their way into AI, and their journeys so far.
-
Whether AI is accessible for people without much prior experience.
-
Some of the best examples of AI and ML today.
-
The value of digital twins.
-
The importance of data quality with AI.
-
How AI and ML can help us explore innovation.
-
Will AI replace engineers?
-
How can organizations start implementing ML and AI in their workflows?
Host 00:12
Hello, on today’s episode, we’re going to be talking about artificial intelligence and machine learning. And with me today are two specialists in AI and ML. We have Justin Hodges, who is a Senior Technical Specialist in AI and ML at Siemens, and Remi Duquette, who is a VP for industrial AI at Maya HDT. Hi, guys, how you doing?
Remi Duquett 00:32
Hi, I’m doing well. Thanks.
Host 00:35
So I’m going to start off with a question I know is gonna irritate both of you, because we’ve talked a bit before about this, but that when I first interviewed Remi, about 18 months ago, I really struggled for some practical examples of AI and ML that everybody would understand in the real world. I think we did end up talking about things like automated driving, and Siri and Alexa, which are kind of a bit tedious. This year, I think the whole world of AI and ML has changed, from a public perspective, because of ChatGPT. Because I think before it was something that was kind of abstract. And now, everybody has a sandbox in which they can play with AI and ML, and understand it, and see some of the power of it. What are your views? Before we start talking about the serious stuff on ChatGPT?
Remi Duquett 01:21
Justin, you want to kick us off?
Justin Hodges 01:23
Sure. Somewhere, I heard the phrase, I don’t remember where, about it — that it’s really valuable to have someone that knows a medium amount about everything. And I think that’s really useful to help round out when we do all sorts of tasks, or in the case of engineering, all sorts of disciplines and sort of thinking. So I think it’s a huge enhancement. And I think that, you know, kind of like when cell phones were first introduced, and it was a novelty to have them. But now, literally, it’s everywhere, and you can’t imagine being without it. I think it’s kind of like that. Rising tide raises all ships. And I think that will be sort of how it proliferates into everything. Not exactly ChatGPT, but this style of an assistant.
Remi Duquett 02:03
Yeah, I mean, I concur. It’s kind of interesting that you mentioned ChatGPT, in the sense that even back in November last year, not even what, five months ago, it was not revealed really to the rest of the world. And all of a sudden, everybody has woken up to a very specific example of something they can use to augment themselves. And I think, you know, as we’ve done for the last five years now at Maya, it’s really to augment people, whether you’re an engineer or the operator or something that requires technical, specific tasks to be done in a better way. ML and Deep Learning in general, has helped a tremendous amount of specific cases. So we can talk about those specifics. But, yeah, it’s interesting that all of a sudden, everybody is talking about AI.
Host 02:52
So with ChatGPT, now, suddenly, everybody thinks they’re an expert in AI and ML, but at the start of this, I introduced you two as experts. Can you tell us a bit about your journey into AI and ML? Because I guess you both started off from an engineering background. And you’re basically early adopters of this technology. So Justin, how did you get involved in AI?
Justin Hodges 03:13
Sure, so I grew up academically and professionally in the world of turbomachinery, which may be typical C/CFD sort of background. And then, in 2017, I did an internship in Princeton that combined CFD to healthcare-related flows, like in lungs and machine learning. And then, I remember when I was there and I would have coffee or lunch with the interns, I quickly realized that everyone else there was just replacing basic cardiac CFD flow projects with machine learning models. And I thought, “Oh, this is going to cause a shift in paradigm thinking.” So from then, I made it my manifesto to make that my career one day. And so just progressively incorporating it into my dissertation, doing development or research-type projects, and then eventually, making that my full-time role. With my background being the traditional thermodynamics, fluid mechanics, turbulence, heat transfer, that sort of thing.
Host 04:10
And Remi, how about you?
Remi Duquett 04:12
Yeah, I mean, it’s maybe a slightly different way into ML. About, 2012, being based in Montreal, I was surrounded by what became one of the key AI towns in a world, where Dr. Yoshua Bengio and others became very famous for making neural networks actually be very practical and resolving all sorts of different problems, mostly on the machine vision side, but also in other aspects. And based on being surrounded here, by these new technologies that were evolving very rapidly, I kind of got interested in the topic and got my feet wet — taking some classes and other things to ramp up my knowledge of how neural networks can be leveraged in engineering. And eventually, that progressed into applying those in the real world, in real industrial applications, that we, you know, focus on here at Maya. That’s been my journey into machine learning, Deep Learning in general because I was surrounded by it, really.
Host 05:14
So both of you guys are already deeply immersed in the world of AI and ML, but lots of the people who are listening to this podcast will be perhaps taking their first steps. So to what extent is this technology available to regular engineers, including old guys like me, who are basically just old gnarly CFD guys? Is there a special set of skills that we’d require? Or is it something you can begin to just pick up today, would you say?
Justin Hodges 05:38
I think it’s something that’s pretty readily available compared to a lot of fields and how they matured in popularity over time. I think the open-source nature of the journals and conferences is immense. Something like 30% of papers in some of the major journals are open-sourced, in terms of code and everything. So it’s a great way to get exposure as a practitioner or someone who wants to practice. And then in terms of tips, I would say, in addition to the courses and things that people probably know about, there’s a website called Kaggle, that I would recommend. It’s where machine-learning competitions are hosted. And it’s a great resource to learn because you can go in, copy any dataset and code, and just break it and learn that way by doing, which is a really nice complement to the theoretical knowledge that’s out there on the internet in vast quantities.
Host 06:25
Because you only really learn by doing, don’t you? You can read as much as you like about this stuff, but actually, you have to get your hands dirty. And like you say, break a few things. What’s your view on this, Remi? Do you think you need to be a specialist in order to start using AI?
Remi Duquett 06:38
You don’t need to be a specialist, but you do need to have a good foundation in statistics and probability, and math in general. What I would say is, you know, clearly, as an engineer, we have all those bases covered. But if you’re not an engineer, then clearly, you’re going to have to ramp up a little bit, perhaps on probability and different measures that neural networks will provide. But, yeah, the learning by doing and trying out in Kaggle is a great place to start. You’re going to need some Python skills, because most of the frameworks are kind of written around Python, whether it’s PyTorch or TensorFlow, open-source components that you can leverage to build those models and train them based on the datasets that are referenced either in Kaggle, or other public datasets. There’s an immense grouping of different capabilities there that are readily available for anyone that wants to try it out and break something.
Host 06:41
So as this discipline, as it becomes more and more useful, do you think we’re going to see more of a role for data scientists in engineering? Or are engineers going to evolve towards being data scientists? Or don’t you think it really matters at all and kind of a standard engineering skill set will allow you to use this stuff productively? Remi, do you want to go first on that one?
Remi Duquett 07:49
Sure. When you look at engineers versus data scientists, I think it’s not one or the other, in my view. So I think there is a certain level of engineers that will push. I mean, as engineers, we all use tools, in a practical fashion, right? To deliver different models, whether it’s [inaudible] or neural networks. It’s really just another tool to leverage in our arsenal. But data scientists typically will go a little further than that, they’ll try new sets of hyperparameters in the model and try different types of models, perhaps, that engineers may not try just because they’re going to use the true invested and just perfect and apply them for their tasks. So maybe, it’s a bit of both, but I would say it will remain both for a long time anyway, until it’s maybe bread and butter for everyone to use these tools.
Host 08:39
Do you agree with that, Justin?
Justin Hodges 08:40
Yeah, both. There’s one thing my friends and I pass around whenever we’re frustrated about something, or how rapidly the field’s moving and trying to keep up, and it’s that, the line between a computer scientist and mechanical engineer, from the mechanical engineer side, is shrinking and becoming invisible. So to some extent, yes, I think our skill sets will have to adapt and accommodate that over time. I think a lot of people who did grad school in the last 10 years for mechanical engineering would say, they took very, very, very few hardcore actual programming classes. Even my mentors, that I look up in the highest esteem. Although they’re accomplished, they’d say in school they’d never took a single formal programming class. So it is going to probably be something like that to pick up. And then, the other jokey-type thing is, you know, when tractors came out, farmers didn’t go extinct, but they also still had to keep farming. That was just another tool. So whether it’s the tool integration for traditional ways of designing things, or some of this knowledge, I think it’ll be a both situation, for sure.
Host 09:45
So I don’t want to focus too much on obstacles. So maybe now we can just talk a bit about some use cases. So for you guys, what are the best examples of AI and ML that are usable by engineers today? So do you want to go first now, Justin?
Justin Hodges 10:01
There’s too many. We’ll start with the real relatable one to pretty much any audience. You can say the ChatGPT one, the Siri one. I think it was a few years ago, Google had, like, an investors call, or some marketing event where, you know, they would talk about new stuff they’re working on. And they had a Google assistant that could call restaurants, place reservations for them, and need no input the whole time. And so this is like years old now at this point. But the point was, at the time, that was shocking because you could not be involved at all, and still get things done. And I think that, some of the tasks that are very complicated, as an engineer, as original equipment manufacturer, obviously, automating some of them would be very, very good for freeing up your time. Look at electric vehicles, right? I mean, the race to redesign and create new things that are the first of the kind in the company’s history is just a huge burden for time and responsibility. And if you had services or systems or resources that are akin to ChatGPT assistants, user-augmented experiences that are AI-based, I think those are highly attractive, even if the fidelity of the designs are the same. But they have, like, this time savings of 30%. To me, that’s one of the only ways I see that companies can meet these ambitious goals of designing the same products in half the time.
Host 11:18
Okay, so that’s the first one. But I think the point there is, that the engineers are expensive and limited resources, aren’t they? And, you know, I’ve been doing computational fluid dynamics and engineering simulation for 30 years. And for that whole time, we’ve never been able to do as much as we want to do because we’ve always been limited by computing resources, by costs and, actually, by our own time. And, actually, so, I think what this is going to do, it’s going to free up engineers to spend more time doing the stuff that we want to do, which is innovating, understanding, and less time doing the kind of mechanical jobs that we all hate doing. Is that a fair assessment? Justin?
Justin Hodges 11:50
Yeah, to state it, I guess, in more plain terms in my original statement. You know, if you work for an airplane manufacturing company, you don’t want to spend your time coding, you want spend your time focusing on, like, why a plane crashed, if it crashed, right? And, so, automating all of the details technically to remain focused on applying your principal discipline is ideal, I would say. So, yeah. Yeah, you’ve got it right.
Host 12:12
So you gave us one example, Justin. Was there a second one you wanted to give us now?
Justin Hodges 12:15
Yeah, I think time savings. You know, you’ve heard digital twins reduce sort of models surrogates, it’s in this class of this sort of use case, I would say. I mean, useful for a lot of reasons, but one common thread I would say is time savings. Right? I mean, if I’m designing a component for … again, the airplane example, right? Like, you know, a key consideration, or ambition, is to find the most efficient type of design, right? That meets all my requirements. But now there are thousands of off-design, operating conditions possible where you even need to take that most performant design and make sure that it’s safe, in all of those other scenarios, which are just way too many to simulate or test. Right? So I think a really attractive idea is to take the work that you do, to come up with those most performant designs, and generate these AI-based models, that are basically surrogates, to simulation and allow you to have confidence and check off all those thousands of other design points that, you know, frankly, you just couldn’t simulate. You’d have to sort of make peace with not simulating all of them and do your judicious analytical thinking on which ones are most key to simulate. It’s a trade off, really. So I’d say that’s the second one. It’s hinged on time savings.
Host 13:23
Excellent. And Remi, have you got any examples that you’d like to tell us about?
Remi Duquett 13:27
Well, I can extend maybe the use of those reduced sort of models, or surrogate models. So as engineers, we typically will design and simulate and test and build the most optimum that we have, in the certain period of time that we’re given to do it, right? Now, typically, our job would pretty much stop there. In terms of, we would get feedback from the usage, perhaps of the product, if that’s the case. And get new boundary conditions or things that we would need to apply to any new conditions, environmental conditions, that we had not really simulated for based on new usage or novel usage of whatever we produce. But now, we have a tool that we could leverage in operation, right? So the engineer’s job is extended in that way, where we can now put those reducer models to run in real time with the apparatus that we’re deploying on the product we’ve created. And that sensory, real-time telemetry that you can gather around the use of the products can also be fed to that AI model and provide basic outlier detection or places or environments where, actually, the product was not tested in those environments. And kind of self-protect the environments and the usage of the product based on those, maybe, signals that you send back to the user, or whoever is using your product. So that is kind of extending now the reach also of what engineers can achieve. So that would be one practical thing that we’ve deployed, whether it’s on marine vessels, or in manufacturing of lithium batteries for electric cars, like, these kinds of places where, fortunately, or traditionally, we would not necessarily extend our engineering prowess into, and now we can actually make a difference in those operational environment, based on leveraging all those simulations because those simulations are very precious, right? I always use the term imbalance, and maybe for some of the listeners it might be a bit of a technical term, but when you look at data, you have a lot of good data. People are using your product, typically, roughly, in the range that you’ve designed it and simulated it for. But the real interesting components are the failure modes that the product will have. And if you’ve done your job right, actually, those things don’t happen that much. Because it was actually designed to avoid these failure modes. But now we may have new tools to leverage and create more of those failure types of data to learn from, where we can optimize and further gain a few margin points. Maybe it’s weights that we want to save, or higher performance in terms of temperature, distribution. Whatever it is, that from an engineering standpoint, makes the product better is perhaps where these new tools can help us.
Host 16:15
And I guess in the world today, so a big change is kind of the advent of digital twin as well, because of engineers, my generation, you know, validation was something that happened once at the end of development, and you might get some usage data. But now digital twins offer the prospect of getting that kind of real-time uses data, including failure, for any and all products continuously as well. And so I guess that’s a good way of feeding these algorithms. Would you agree?
Remi Duquett 16:41
Absolutely. Certainly, if leveraging real-time telemetry to then optimize in other areas that, as you said, in the past, you would have had one validation test or a couple of validation tests where you’ve invested in pristine environments, right? Your product, now you can extend it to the real lifecycle of the product and pull that information. Whether you use Siemens MindSphere, or other IoT type of data-gathering tools, you can feed that back to your engineering lifecycle and build amazing Amosun models for a system-level type of engineering or other types of engineering, where you can leverage that data.
Justin Hodges 17:18
That’s a good point, that was a really nice compliment, because my answer was pretty much “use it in design to prevent bad outcomes,” right? Then also, even though that may not always be completely possible to know for certain if you do have an anomaly, or an edge case where you’re operating outside of your safety envelope or something, use machine learning as the operator persona as well, so that if it does slip through and does happen, you do have these real-time ways of measuring with executable digital twins, or just anomaly detection methods, or like you said, with telemetry, so that the operator can be alerted before the situation gets worse, right. So that is nice to have these safety nets, like at several parts of the process of design and operation.
Host 18:01
I talked to some people who were using executable digital twins in the reaction wheels of military satellites. And one of the issues they have is, as usual, with an executable digital twin, you only have a limited number of sensors. But they’re also rather prone to cyber attacks as well. And so they need to be able to know that if they see a problem with one of their sensors, whether it’s because the satellite is falling out of the sky, or because there’s a sense of failure, or because it’s a cyber attack. And they use ML algorithms for telling the difference between those three events, which is really, really powerful, I think.
Remi Duquett 18:35
It is, yeah, for sure. These use cases of leveraging AI models to pinpoint operational inaccuracies, whether it’s sensor drift, or sensor failure of some sort, which have a very detailed signature, typically, when they happen, whether it’s a pressure sensor, or temperature sensor, or whatever it is, even a thermal-imaging camera, you could inject some pixels in there if you’re a cyber attacker. But in the end, these can also be detected relatively easily by AI models that would see that the patterns are changing from a data distribution standpoint, in ways that are, let’s say, unnatural.
Justin Hodges 19:13
Yeah, and I don’t know if it’s immediately something we appreciate how hard that would be to do as humans, right? If I look at an analog signal, obviously, it would be digital, but if it has the look of analog or time series, and there’s just, like you said, all sorts of spikes and things. I mean, that’s hard enough to interpret. But imagine having three dozen or even just 10 of those signals concurrently feeding you back information, right? There’s no real other means to, as a human, ocularly look at the information and then gather patterns between the two. They must have something that’s exceedingly obvious on failure. I mean, you could easily miss these things. So now there’s an inherent value, having this information that back to you as a result or classification or something from machine learning.
Host 19:54
On that point, the point of data quality because engineering data, whether it’s from simulations or from real-life field experiences from digital twin or from test is inherently dirty, isn’t it to some extent as well. So how well do machine learning algorithms tell the difference between dirty spurious data points and real-life events? Is that something they’re very good at doing?
Remi Duquett 20:18
I mean, it’s kind of interesting, because I always tell everybody that comes to us with a data set, assume your data set is dirty until proven clean and reliably clean. And it’s kind of interesting, from a data science perspective, a lot of people would take datasets offline and clean them with a million operations, and then build a model with the clean resulting data. And then once it goes into production or operations, a few of those transformations are not exactly done the right way or the same way. And then your AI model will go berserk. And it’s not because the model is wrong, it’s truly you’re not cleaning the data the same way that it was trained. So AI models can be very sensitive to data in, you know, sporadic things, or yeah, just noise in the data. So you have to be real careful on how you clean, we call them data pipelines between the raw data that you’re collecting and the actual AI inference that you ingest the data with and produce some outcome. So yeah, we always put big safeguards around data-cleaning process, because that can easily trip engineers, if you’re not careful, and applying too many transformations that will really be there in real time.
Host 21:29
Do you have any comments on data quality, Justin? Is it something that concerns you greatly?
Justin Hodges 21:32
It’s kind of all around. I mean, if you think about physical test data, and you say, “My ambition is to make a machine learning model that can sit on top of my physical measurement data that’s being taken to assess the quality of it,” right? I mean, look how many examples that could mean. I could set up my equipment, have all my sensors from my tests, and I could do that improperly. And I could have a machine learning model catch that it was set up improperly, before I waste a test, or one of the components in the actual device, like the car or whatever I’m testing, could break. That could be a feedback, right? Or one of my sensors could break, and that could be a feedback. Or everything truly operates properly, but the conditions or the testing environment is now producing an anomaly in itself. That could be picked up as essentially this class of like data quality, like recognizing that the patterns are not healthy, normal patterns. So even in addition to what Remi said, in terms of, like, the importance of treating bad data, and things like that, I mean, if you talk about data quality, it’s also, even well-posed models can be used in this as, like, the end outcome. Detect healthy operation. So yeah, I think it’s key. I think all engineers have heard the phrase, “garbage in, garbage out.” So in this case, we spend a lot of the time making sure that we have good data come in and lesser amounts of time just probably picking the algorithm or the architecture of what we’re going to use and tuning it. And that’s just the nature of the beast, I think.
Host 21:58
Okay, thank you. So this podcast is called the engineer innovation podcast. So obviously, we’re interested in innovation, and creativity. And I think part of the myth of engineering is that engineering advances by these big eureka moments, when in reality, you know, they’re small, incremental nudges towards an eventual target. To what extent do you think machine learning and AI algorithms can help us explore and experience genuine creativity and innovation, how are they going to help us with that?
Remi Duquett 23:25
I mean, if you look at generative pre-trained transformers, like GPT, there are equivalents in generative design that will produce new geometries that are really, could not have been taught about from by engineers. And so those kinds of AI design tools will definitely trigger innovations in ways that are probably unpredictable today, right? The way that an engineer will look at a new structure that’s been put in front of them, that seems to do the job, and then the engineer will spark some idea. And to your point, I don’t think it’s eureka moments. It’s like fine-tuning these different things that will come out. And because we’re just going to get more inputs from generative type of algorithms and transformer kind of technologies that we have access now. I think that is really what’s going to transform us slowly to become better engineers and augment ourselves.
Host 24:22
Have you got an answer to that one, Justin, or still stumped?
Justin Hodges 24:27
No, there’s just too many things to dial down. I’ll come out first on incremental versus eureka. So, I would say there’s a gigantic inertia in the principal field of machine learning. And, you know, the example I like to cite is that in 2020, from the Stanford AI Outlook published like survey, there were about 100,000 papers published that year globally, that had something to do with machine learning or AI. Well, if you look at the Holy Grail of mechanical engineering literature, American Society of Mechanical Engineering, just as one popular one, right? They were created in 1870-something, I believe, and total, to date from them, till now they’ve published 250,000. So 100k, in a year 250k in a lot of years. I mean, you can see that there’s going to be a lot of small wins, and maybe even eureka moments because there’s so much inertia behind this, in terms of publication. And publications aren’t everything, but the point is, commercially, you’re seeing it everywhere in the news. Academically, you’ve been seeing it for a while. So, it’s kind of just coming in from all corners. And there are, I would say, some use cases that are absolute eureka moments, where machine learning can do it 100 times faster, right? And then it’s just an obvious thing, that that’s how it’ll be done for a long time. And then there are others where it’s very incremental, like you said. You know, for example, let’s just zoom in to computational fluid dynamics, right? Like, we know, there are a lot of equations and things in there that affect the answers and the accuracy that are like super empirical-based, right? Like, I may be doing a heat exchanger design in my CFD simulations, using some equation that was derived for like flow over flat plate. Because we have more unknowns than equations, we have to put in stuff and empirical stuff ends up finding its way in there, right? And then yeah, now machine learning can replace, in some cases, those parts of the empirical code that now are more accurate, and that’s an incremental advancement, but still an advancement, right? So yeah, part of the reason I was spinning my wheels when you asked the question, there’s so many examples. On some levels, it’s huge. On some levels, it’s minor, but I think there’s enough for us to, that it will keep happening. As far as creativity goes, there’s a ton of apps out there, nontechnical, right, for photoshop, for image generation for speech, for making music. I mean, just as a human, it’s really cool that you can be so creative in a different way. And that’s got to affect the rest of us that use computers for non-creative tasks, right? So, yeah, I think it’s an exciting time.
Host 26:53
It really is. So we’ve talked about replacing bits of algorithm, but what about the potential I’m hearing about replacing engineers? So in preparing for this, well, I decided to ask ChatGPT to prepare a list of interview questions. And I promise you, I haven’t used them all. But one of the ones that came up for was kind of what are the ethical problems of AI and ML, in regard to engineering? And I guess that means the potential for replacing each of us? Do you reckon that’s going to be a long-term effect of this, we’re going to see less use for engineers or more use for engineers?
Remi Duquett 27:24
That’s a very interesting question. Because I certainly in the medium timeframe, I think we will all see engineers being augmented and not reduced. I think our capabilities will just be better and better over time. By using and being augmented by these new technologies that are there, they’re just new, amazing tools that engineers will endorse and leverage to the nth degree in very creative ways, I’m sure. In terms of replacing engineers, I would say we’re definitely far out from that happening for multiple reasons. A lot of the tasks, even if they’re fairly complex, can be done extremely well with AI technologies. But when you look at the complexity of harnessing different things, and bits and pieces, and putting them in a fairly complex ecosystem, and environments, and engineers will make practical things happen by virtue of their trade and the way they’re trained. They’re just going to do it better for a long time. And before you can train an AI to be a really well-versed engineer that can replace us completely, I think we’re quite a way out. But I could be proven wrong, and that’s fine. If it augments engineering, and creates better engineers than us, then we’ll go and enjoy whatever they produce.
Host 28:46
I’m 51. I’ve been working in engineering for 30 years, I reckon I’ve got about another nine years left, do you think I’m going to make it? Or am I gonna get replaced by an algorithm?
Justin Hodges 28:55
I’ll ask a question back to you that I think reverberates my answer. Innovation is not new with AI. In the last however many years you’ve worked, right? So how many times have you seen a colleague have innovation, and then just say, “I’m totally done, now. I’m gonna go–” I don’t know, whatever you guys do in London, or in my case of Florida, go to the beach, right? No, they have so much more to do. They never just say, “I’m done. I will see you tomorrow. I’ll see you next week.” So I think kind of like Remi echoed, I mean, great. You can really be quick at getting certain things done. But now you have so much else to do that will help your overall end product quality, or they allow you to do other explorations to improve your design, whatever you’re making. So yeah, I think it’ll go back to the farmer-tractor example. Farmers are vastly more capable of output, but they’re not just sitting on their hands with triple the amount of vacation, right? So yeah, I don’t think you’ll be replaced.
Host 29:49
Yeah, I could do with more vacation, though, but that’s a relief, at least, Justin. So thank you for that. So we’re about out of time. So as a final question, we talked a bit about how individual engineers might get started in AI. But if there’s any organizations who are listening to this podcast, and they’re really convinced by Remi, and by Justin about the benefits of AI and ML, how would they go about getting a start and taking the first steps towards implementing AI and ML in their workflows? What are some really good first steps for those guys?
Justin Hodges 30:19
Well, we work really hard to enable our colleagues in our company to help with that transition. So if you’re already buying products in our portfolio, then it should be pretty seamless to just also inquire about your specific needs for machine learning. Part of what I’d spend a lot of time doing at work is, like I said, enabling colleagues on this disseminating information, materials, shared customers, our groups are very active in publishing to show from a thought leadership point of view, what is possible, if you don’t already know. So yeah, we try to have a support base model to help customers without onboarding. But if you take out the commercial side of that, and just say, general, it’s always good to read papers or newsletters that summarize advancements in your field, that happens a lot. So that can be really good to spur ideas you have of what is possible in general. And then, like I said, we’re equipped, then prepared to just make it very easy in a conversation to sort of explain what we have to offer to help you get there.
Host 31:17
How about you, Remi? Can Maya help with that journey, too?
Remi Duquett 31:21
Yeah, I mean, we’ve actually puts in place of program where we call it like, get AI ready, and parts of it is a lot of education topics around what are the pitfalls, where to focus attention, and what kind of tools are out there to help you. And as an engineer, if you look at even just the [inaudible] RunBuilder, as an engineer, you can wrap your head very quickly around how to leverage in reduced order models, using the Siemens tool to get them full set, and just understanding a little bit better what those new tools and avenues are for you, I think that would be a great way to start and get educated. As Justin mentioned, in terms of papers, and any documentation around models, look at all the opensource Python TensorFlow type of models that are out there publicly available with code, papers with code is, fortunately for us in the AI community, very prevalent. So you can look at any of those that can give you some hints, and as engineers we’ll keep tinkering and making them even better. So start from those baselines, and move on from there.
Host 32:24
Thank you, guys. That’s some really helpful first steps. Thank you for being excellent guests on the Engineer Innovation podcast, and thank you all for listening.
Remi Duquett 32:33
Thank you, Steven.
Justin Hodges 32:34
Thanks.
Chad Ghalamzan 32:36
Thank you for listening to the Engineer Innovation podcast, powered by SimCenter. If you liked this episode, please leave a five-star review. And be sure to subscribe so you never miss an episode.
Guy de Carufel 32:48
Looking to connect with a likeminded community of thinkers, doers, and change-makers? Are you a technical user, process leader, Deployment Manager or partner in the aerospace defense, energy or transportation industry? Join us from June 12 to 15 in Las Vegas, Nevada, for Realize Live Americas or from July 10 to 12 in Munich, Germany, for Realize Live Europe. You’ll have access to breakout sessions, research orientation to workshops, demos, hands-on training, and much more. Learn more at events.sw.siemens.com
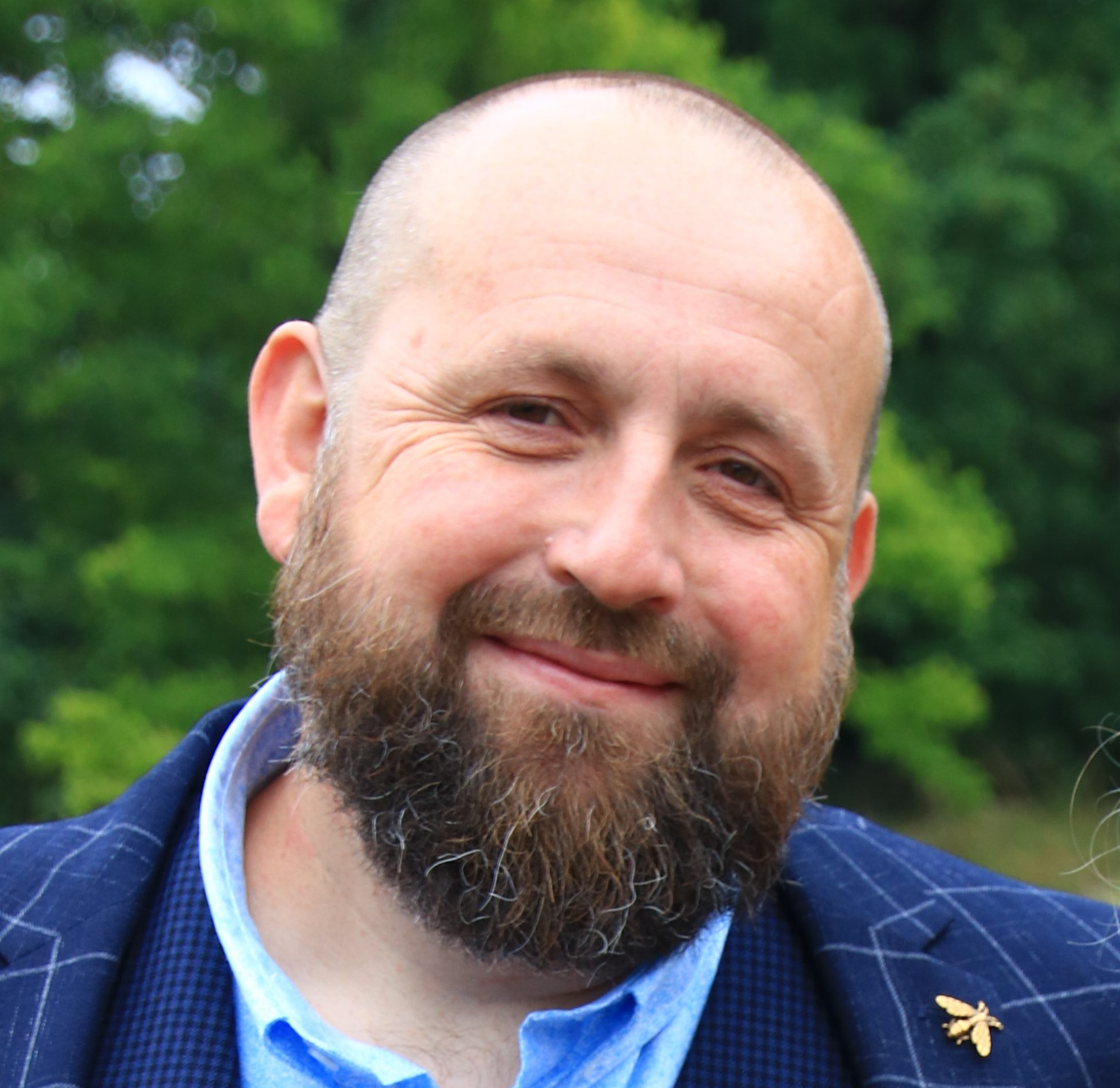
Stephen Ferguson – Host
Stephen Ferguson is a fluid-dynamicist with more than 30 years of experience in applying advanced simulation to the most challenging problems that engineering has to offer for companies such as WS Atkins, BMW and CD-adapco and Siemens. Stephen’s experience of AI and ML is limited to late night experiments trying to convince ChatGPT to do something genuinely useful.
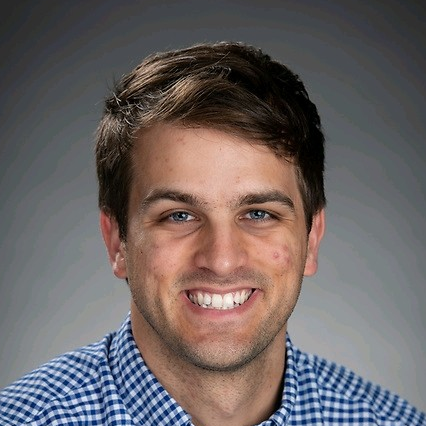
Justin Hodges – Guest
Senior AI/ML Technical Specialist, Product Management at Siemens Digital Industries Software. He has a bachelor’s, master’s, and Ph.D. in Mechanical Engineering specializing in Thermofluids and a passion for AI and ML.
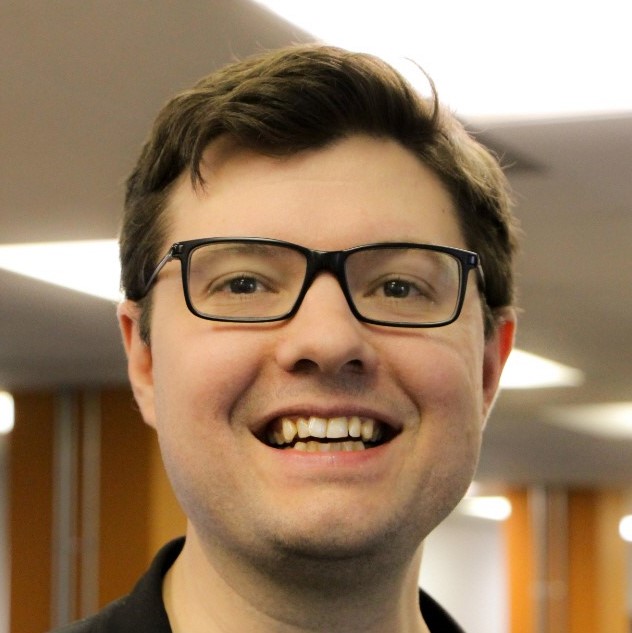
Remi Duquette – Guest
Vice-President, Innovation & Industrial AI at Maya HTT. With 20 years of experience building practical, effective solutions, Remi now plays a key role in heading Maya HTT’s industrial IoT and AI (machine learning, deep learning) solutions strategy and innovation.
Take a listen to the previous episode of the Engineer Innovation Podcast. Series 2, Episode 3: Experiencing Digital Twins in the Industrial Metaverse.
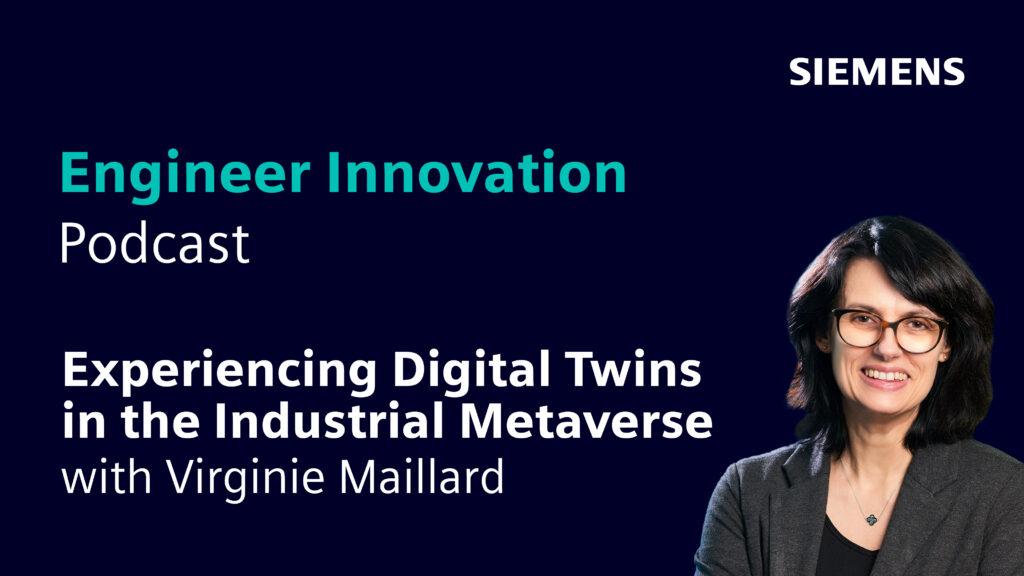
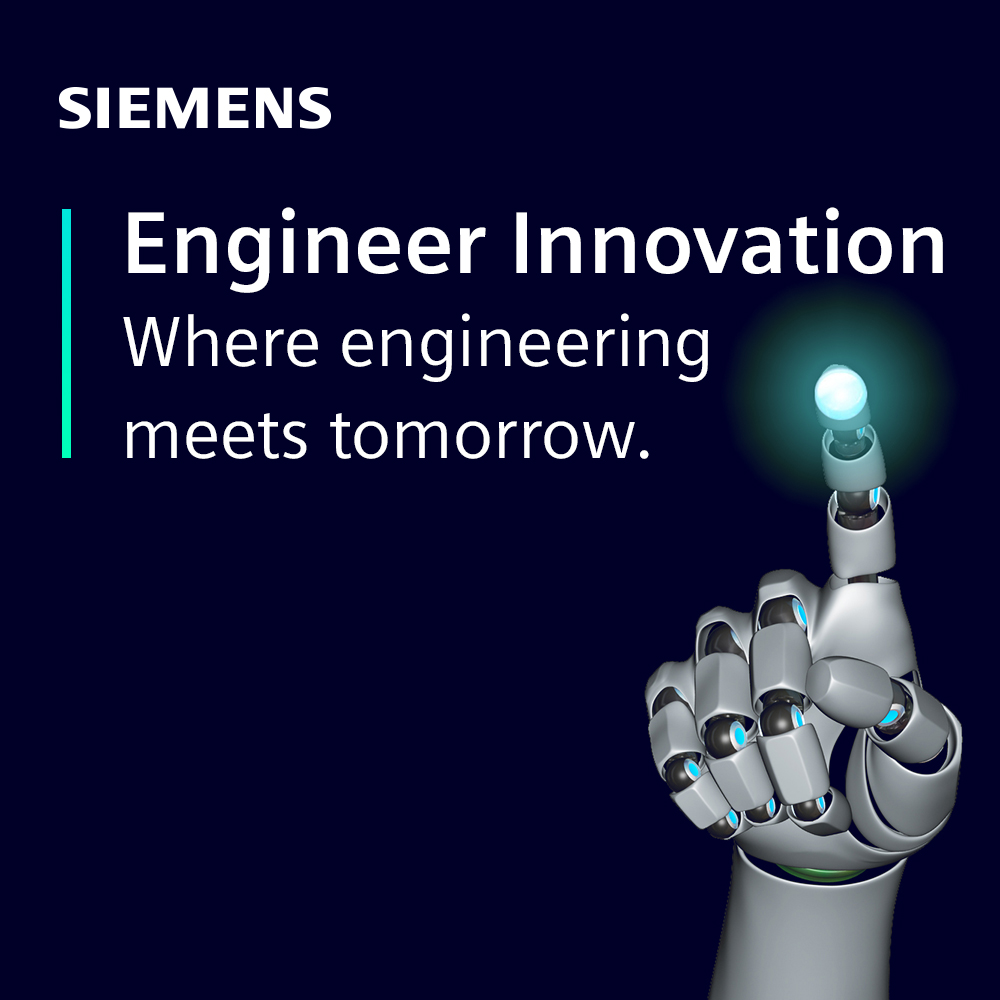
Engineer Innovation Podcast
A podcast series for engineers by engineers, Engineer Innovation focuses on how simulation and testing can help you drive innovation into your products and deliver the products of tomorrow, today.