So much data, so much potential
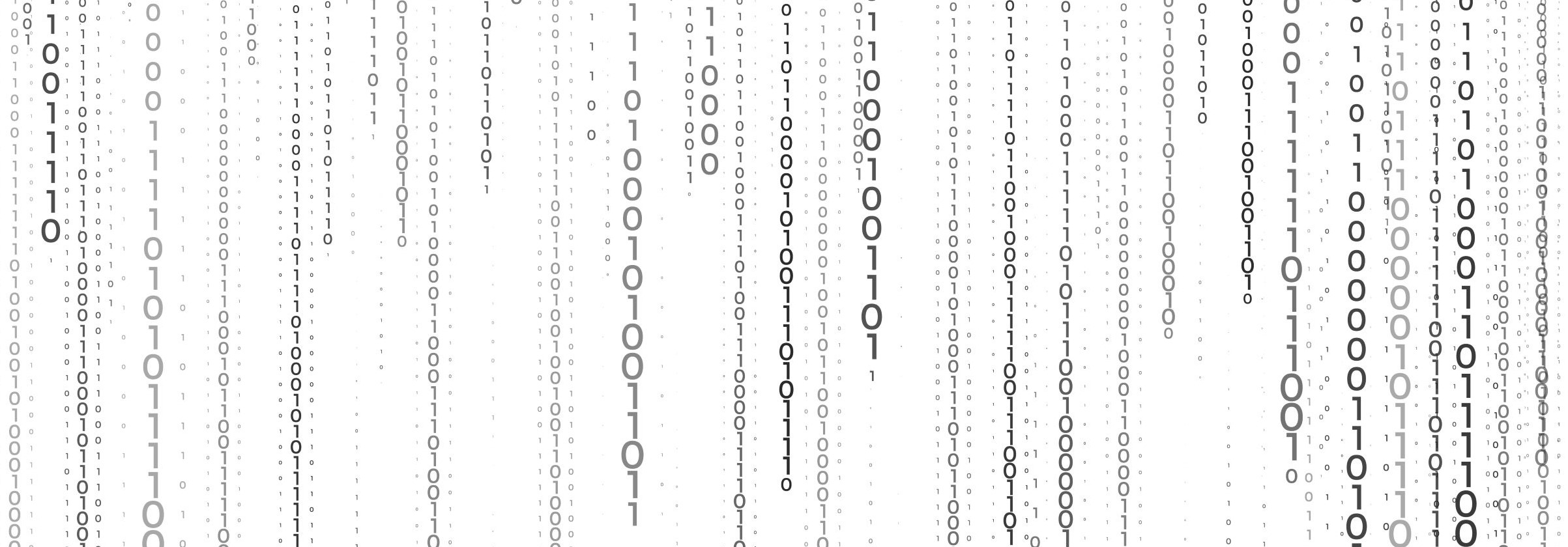
Part 2 of our 3-part blog series about Industry 4.0.
In our previous post about Industry 4.0 (i4.0), we discussed robots and machine-to-machine (M2M) connectivity. In this post, we will discuss sensors, the digital twin and predictions.
The modern manufacturing process produces a lot of data. Imagine yourself as a fly on the wall in the factory—there’s a lot happening, and everything that is happening produces data. Computers collect all the data from the manufacturing shop floor, including process data (what is happening, when, and by whom), material data (what material is used in the production process) and many other bits of information. As sensors have become common on industrial shop floors, even more data is now available. Sensors can measure everything—weight, temperature, humidity, air pressure, noise. Just name it, and they cover it.

The data collected from the sensors on the shop floor enriches the process data dramatically. We now know not only that we produced a specific snack from specific ingredients, with a specific weight on a specific date with specific quality; we also know the humidity, temperature, and many other factors that might influence the final product. This complete data set creates what is known as the digital twin of the process and product—a complete virtual representation of what and how a product was produced.
An enormous quantity of data is collected. I will discuss how this data is used in the next post, where I will cover big data and ML/AI.
Now, let’s talk about prediction.
In the past, when something went wrong, the machines were stopped, and a person was required to resolve the issue. He or she needed to investigate the root cause, and then fix it in order to restart production. This downtime led to significant financial losses. However, today, as rich data is collected about the manufacturing process, we can identify issues before they even take place.
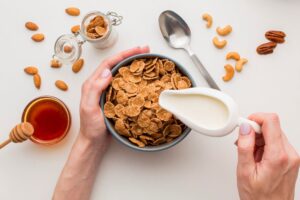
Imagine a machine that puts 700g of cereal in every box on a line. As there might be slight variations between flakes, you could imagine that 700.001g is also valid, but 702g is not. In the past, if there was an issue with the equipment that allocated the flakes, and a 702g pack was measured, the production was stopped. The operator had to come onto the floor to fix or replace the part.
Today, when measurements are collected on an ongoing basis from the various sensors and real-time results are calculated, an anomaly tendency can be measured before the issue itself occurs. It can predict that if the same curve is maintained (700.01g, 700.02g) it will soon lead to a problem. It can alert the operator before an issue occurs rather than after.
Stay tuned for the next post where we will cover big data and AI/ML.