Understanding uncertainty with AI
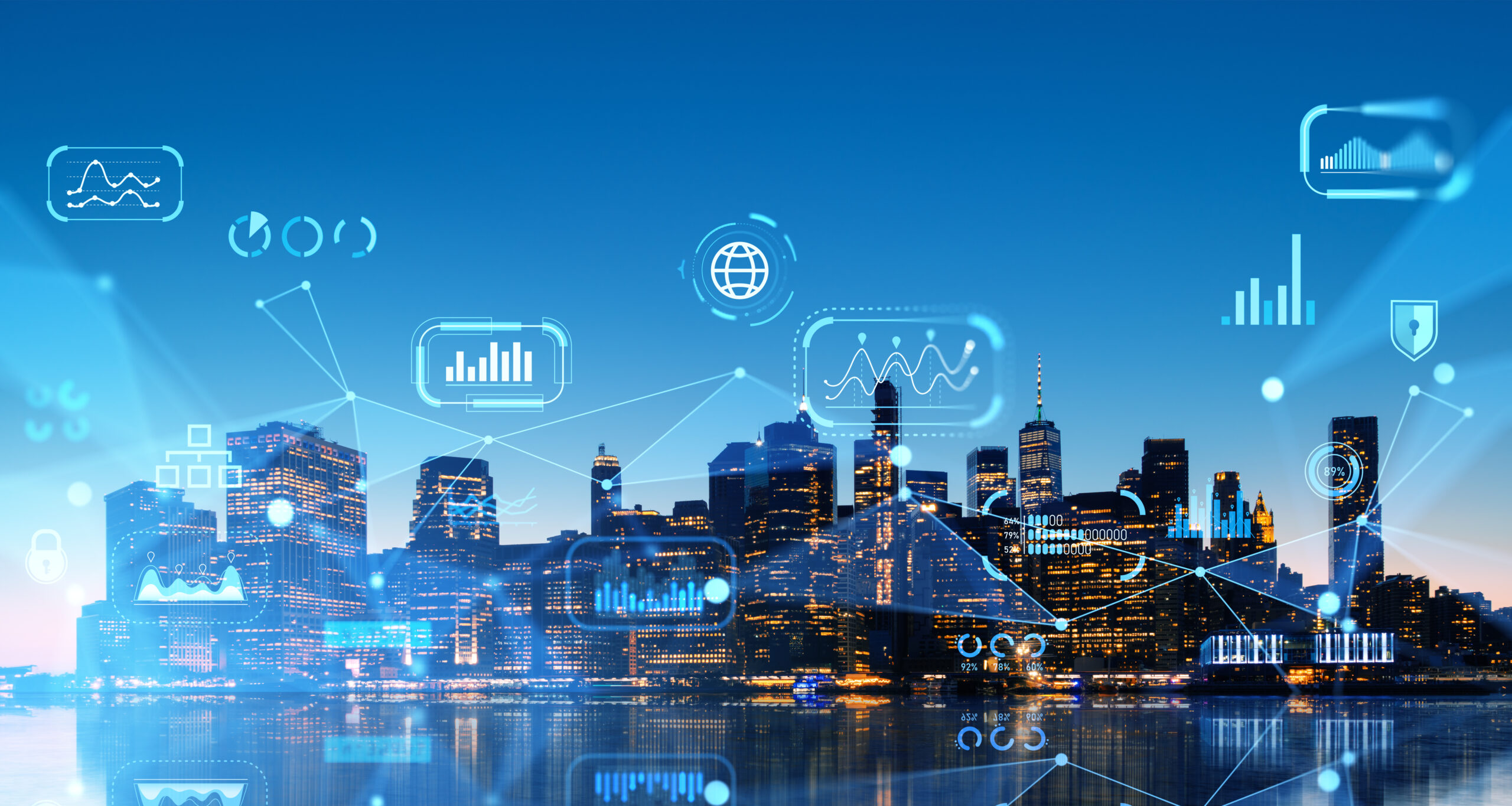
Finding the optimal design for a product is no easy task, especially as technology continues to advance and products grow increasingly complex. As powerful and intelligent as computers and software have become, it’s only natural to turn to them to assist in the process with advanced design space exploration tools that can leverage the power of Artificial Intelligence and Machine Learning. To rely on these complex, AI-driven tools however, it is vital to understand their accuracy and capabilities as well.
In a recent podcast, Dr. Gabriel Amine-Eddine, Technical Product Manager for the HEEDS Design Exploration Team, shed some light on the process that led to the development of modern design space exploration tools and the AI elements they rely on. Additionally, he highlighted how it is vital for the AI underpinning these tools to be robust and reliable especially when millions of dollars or even people’s lives may be on the line. Keep reading for some of the details of that conversation or find the full episode here.
AI helps achieve an optimal result
When it comes to developing new products and achieving the optimal design, it comes down to a matter of numbers, not just running a certain number of simulations to map out a design space either, but in the number of parameters being considered in the optimization process. The fewer assumptions that are made when assessing a design, which is to say the fewer parameters that are hell constant, the more closely the simulated part will align with physical world. This means less testing and validation of physical prototypes is needed, smaller fudge factors are requried to account for fixed parameters and, in the worst case, it prevents compounding assumptions from delivering a product that simulates well but fails to function in the real world.
While conceptually simple, actually optimizing a model with hundreds or thousands of variables is no simple task, especially when neither time nor budgets are unlimited. This is where AI-augmented design space exploration tools, like HEEDS, come into play to better manage the countless variables and arrive at a solution in a timely manner. However, it’s also vital that designers are able to get an understanding of the strengths and shortcomings of the results these AI tools provide, leading to the implementation of today’s accuracy-aware AI models.
Understanding uncertainty
To understand how good or bad an AI prediction is, it has to be compared to a known-good baseline value or assed against a known range results can fall in. Gabriel compares it to the spread of darts on a dart board, no matter how accurate the person playing is, there will always be some spread with all of the darts clustering within a certain area. While it’s impossible to exactly predict where a given dart will land relative to its target, using this method it is possible to quantify the uncertainty in where the dart will land, effectively measuring how good or bad of a throw it was.
This same method can be applied to the results produced by AI to create uncertainty aware models that can provide an estimate of how good or bad an inference it has provided. However, to make the next step to accuracy awareness, more data is needed. By combining this uncertainty awareness with ground truth data, usually gathered from experiments, the AI model can become accuracy aware with the ability to compare its results against good samples to numerically express how good or bad of an inference was generated.
Being able to quantify uncertainty and judge accuracy is especially important in design where the AI models will need to create new designs which are, necessarily, outside the realm of their training data. By extrapolating new designs from past data the AI can create novel designs but, without being able to assess the quality of the final results, it would be difficult to trust these designs, especially for critical applications which is where accuracy aware AI will play a vital role.
AI has a lot to offer in the world of design, and relying on it will help to speed up traditionally slow processes or even arrive at novel, new approaches. However, to truly rely on it, AI-generated results must be accurate, trustworthy and verifiable which is why building in uncertainty and accuracy awareness will be a critical step in adopting AI into the design process. Elevating AI from untested novelty to trusted colleague won’t happen overnight but, in the end, it will be worth it for the countless benefits it will bring.
Siemens Digital Industries Software helps organizations of all sizes digitally transform using software, hardware and services from the Siemens Xcelerator business platform. Siemens’ software and the comprehensive digital twin enable companies to optimize their design, engineering and manufacturing processes to turn today’s ideas into the sustainable products of the future. From chips to entire systems, from product to process, across all industries. Siemens Digital Industries Software – Accelerating transformation.