How AI is accelerating design space exploration transcript – Part 1
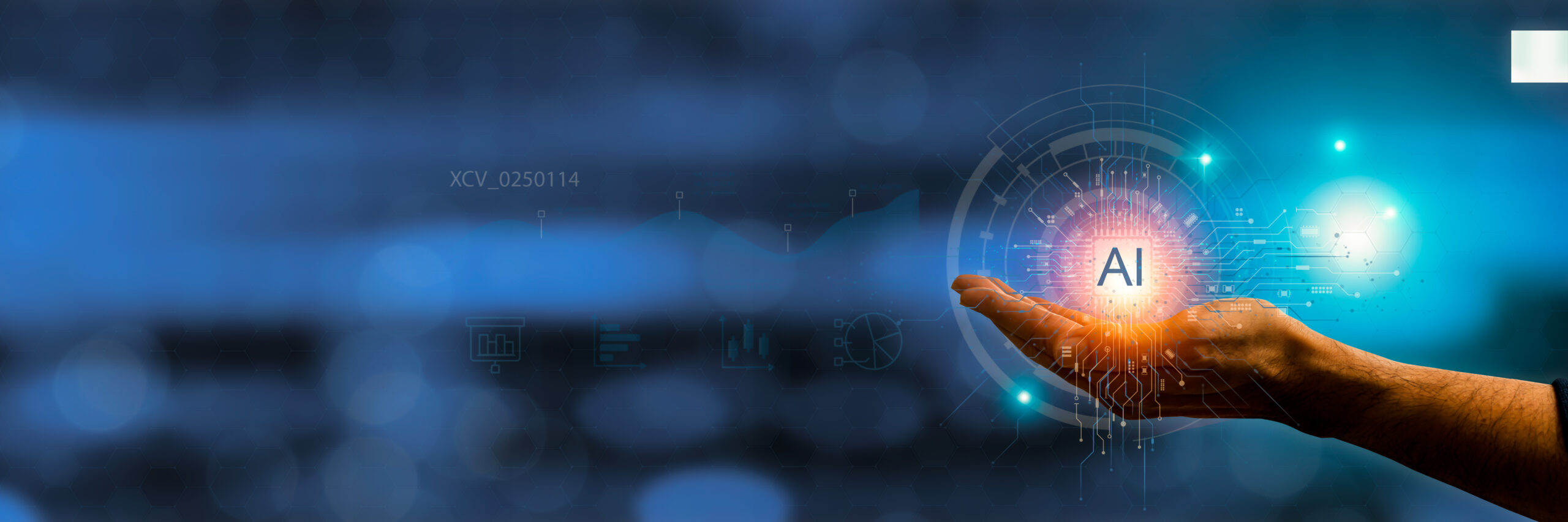
In a recent podcast Dr. Gabriel Amine-Eddine, Technical Product Manager for the HEEDS Design Exploration Team, talked about the ways AI is being used to accelerate the design space exploration process. Check out the transcript of that conversation and listen along to the episode below.
Spencer Acain: Hello and welcome to the AI Spectrum Podcast. I’m your host, Spencer Acain. In this series, we explore a wide range of AI topics from all across Siemens and how they’re applied to different technologies. Today I am joined by Gabriel Amine-Eddine, technical product manager for HEEDS Design Exploration Team. Welcome, Gabriel.
Gabriel Amine-Eddine: Thank you.
Spencer Acain: So, before we start on the main topic here, can you give us a little bit about your background and your work at Siemens?
Gabriel Amine-Eddine: So my background was originally in aerospace engineering, and I did further post-grad studies specializing in complexity science and that’s subsequently where I got my doctorate from. It was right at the beginning of my PhD when I first heard about concepts of evolutionary algorithms and applied artificial intelligence. I just didn’t really have an idea nor appreciation how that would catch up to me today. At the time I was investigating electrosprays and turbulent dispersed multi-phase flows working on computational fluid dynamics, and I kind of wanted to use some of those methods to tune and calibrate the methods and models that I was using at the time. I never quite got the chance to see that aspect through. But I faced during those times the challenges associated with automating the copious trials and experiments that naturally come along with the domain of CFD research. After that research, I joined CD-Adapco’s post-sales application support team, helping our now Simcenter STAR-CCM+ customers with their CFD applications and multi-physics problem solving. And it was during that experience I first heard about HEEDS, I got quite interested in it, and now here I am today talking about HEEDS and the new technology that we’ve been bringing to it.
Spencer Acain: Great. It sounds like you’ve had a pretty diverse background before coming to work on your current project with HEEDS. But for people who are unfamiliar, can you briefly explain what HEEDS is and how you’re using AI in it?
Gabriel Amine-Eddine: Of course, so HEEDS is a multidisciplinary design analysis and optimization software package that provides process automation and design space exploration to accelerate product development and reduced overall cost. Our customers, typically, designers and engineers, can use HEEDS to find innovative solutions that satisfy all performance requirements that they’ve been asked to design, and we do that faster. Fundamentally, HEEDS enables our customers to automate their CAD to CAE simulation tool chains in a manageable way, execute simulations on distributed resources such as local computers, clusters, cloud computing, perform design exploration and optimization as well as gain insights to their designs and discover also, why their designs perform the way they do, what’s the reason for their behavior and how and whereabouts they can improve their products to beat competition and gain that edge in the market. We’re using AI specifically alongside the design optimization component of HEEDS, otherwise known as SHERPA.
Spencer Acain: All right. So you’ve integrated AI with the SHERPA element. Can you expand on that a little bit? So was this something… Did you have AI in this tool before or are you getting it now? Is that a new element?
Gabriel Amine-Eddine: That’s a good question. I would say AI as a term, has been used in various ways over the years. Some might disagree, but arguably, AI is in some form an automated intelligence, and that could be any kind of advanced algorithm. So to some extent, you could therefore consider an optimization algorithm such as SHERPA a form of AI. But these days, if we’re talking about AI, we’ll be using models that typically have to learn their AI capabilities. They’re not going to be able to just work blindly. We have to train them on prior knowledge and that enables them to make predictions about the future and predictions about knowledge that may not necessarily have been obtained yet.And I would also add that I’ve always considered AI as an almost adjacent field to optimization because many of the data post-processing techniques and machine learning methods that we see in the data science realm, a popular field, even more so in recent years. The tools and methodologies used are equivalent to those that optimization experts and some of our HEEDS customer base have been using for the past 10, 15, 20 odd years. And that’s really where we are seeing an overlap and focus towards these days.
Spencer Acain: It sounds to me like moving into AI is almost a natural extension of where you were going with these algorithms In HEEDS and other design space exploration tools that you already have. So, can you tell me more about the tools you have now? The HEEDS AI Simulation Predictor that you’ve been recently implementing and what kind of benefits that offers to the people who are going to use it?
Gabriel Amine-Eddine: Of course, HEEDS AI Simulation Predictor essentially provides, alongside HEEDS, a dramatically accelerated capability to conduct design space exploration studies. And what that really means is, allowing engineering teams to simultaneously take advantage of fast AI-based predictive modeling, alongside direct digital twin-based optimization to improve their products and systems. We’ve seen a significant focus in recent years on creating reduced order models from simulations, making simulations run faster and augmenting simulations with AI to have that speed-up, because we always want to do things at reduced cost in shorter timeframes, but also, meet those demands on quality and requirements. And those are the pressures that our customers today are under for their engineering challenges. But the main problem is, the more and more that you try to make simulations and models run faster, which usually involves reducing the complexity of them, you face the trade-off moving further and further away from that idealized digital twin concept. Because that digital twin concept is really a mirror of what you have in reality and what you can physically test, you want to have a complete digital representation that you can explore with ease, without the costs and burdens of running experiments, trials, which obviously would’ve been the case before we had the compute power that we have these days. And so the fundamental challenge is, how can we maintain the level of fidelity, of accuracy, that complex real industrial simulations need, but also make them run faster. And that’s when we utilize the HEEDS AI Simulation Predictor to provide that capability.
Spencer Acain: I see. So how much of an uplift are we talking about here? Obviously, you’re implementing these AI solutions as a way to make things run faster, but how big of a benefit are you actually getting out of this?
Gabriel Amine-Eddine: On average, we are seeing about a 40% improvement in speed-up. So for example lets say you are performing a design optimization study, running computationally expensive CFD simulations, and your study is expected to take 10 days to complete different designs, instead of your study taking 10 days, that study will complete in 6. Those 4 days of time savings is particularly significant when you’re pressed for project deadlines. Instead of running 4 days of CFD simulations, you are instead relying on informed AI predictions that take less than a second to compute. This provides an opportunity to get the same optimization results back faster, with no loss in design performance, but also the opportunity to evaluate more designs given a fixed time-frame. And to add to that, if you can evaluate more designs, and perform more exploration of a design space, you’ve generally got more opportunity to find even better designs than you would’ve done originally. And that is quite the potential engineering edge that you can gain.
Spencer Acain: I see. And that’s an AI driven element in that. So is this something you could have done at all without AI before or is this something you can only do now thanks to recent advances in artificial intelligence and machine learning and everything?
Gabriel Amine-Eddine: Correct, yes. There are challenges that are involved in pushing optimization algorithms to be at their absolute top performance, and there’s always going to be a continuous focus for us in that field. But being able to augment the optimization methodologies with AI, we can effectively turbo boost the algorithm and the decision logic that is involved in the choice as to whether or not you are relying on a prediction that is fast or whether you want to gain the quality and the fidelity that a digital twin simulation offers. And that’s really the benefit, being able to use them simultaneously and being able to decide when and whereabouts in your timeline of a design exploration study to use and leverage AI.
Spencer Acain: I see. So it’s like you have an AI model in there that’s deciding, do I want to run a really costly big simulation that might take me 12 hours, two days to run, or do I just want to call this other AI model and inference this and say, “I’ll do it in two seconds,” but it may be a little less accurate. And that’s the kind of trade-off, it’s balancing a little bit there too.
Gabriel Amine-Eddine: Exactly. It’s a bit of a fine balancing act.
Spencer Acain: Okay.
Gabriel Amine-Eddine: And don’t forget that these AI models, they still fundamentally have to be trained on data. And that means training on the expensive digital twin simulations, but as soon as they’ve been trained, they are ready to start making predictions, start making decisions, informed decisions to that matter.
Spencer Acain: I see. Well, that sounds like it’d be a huge asset to, well, basically any design team who’s going to be needing to run these type of complex simulations and stuff. But that is all the time we have for this episode. Once again, I have been your host, Spencer Acain, joined by Gabriel Amine-Eddine on the AI Spectrum Podcast. Tune in next time as we continue to explore the exciting world of AI.
Siemens Digital Industries Software helps organizations of all sizes digitally transform using software, hardware and services from the Siemens Xcelerator business platform. Siemens’ software and the comprehensive digital twin enable companies to optimize their design, engineering and manufacturing processes to turn today’s ideas into the sustainable products of the future. From chips to entire systems, from product to process, across all industries. Siemens Digital Industries Software – Accelerating transformation.