AI Spectrum – Examining the Benefits of AI Powered Generative Design – Transcript
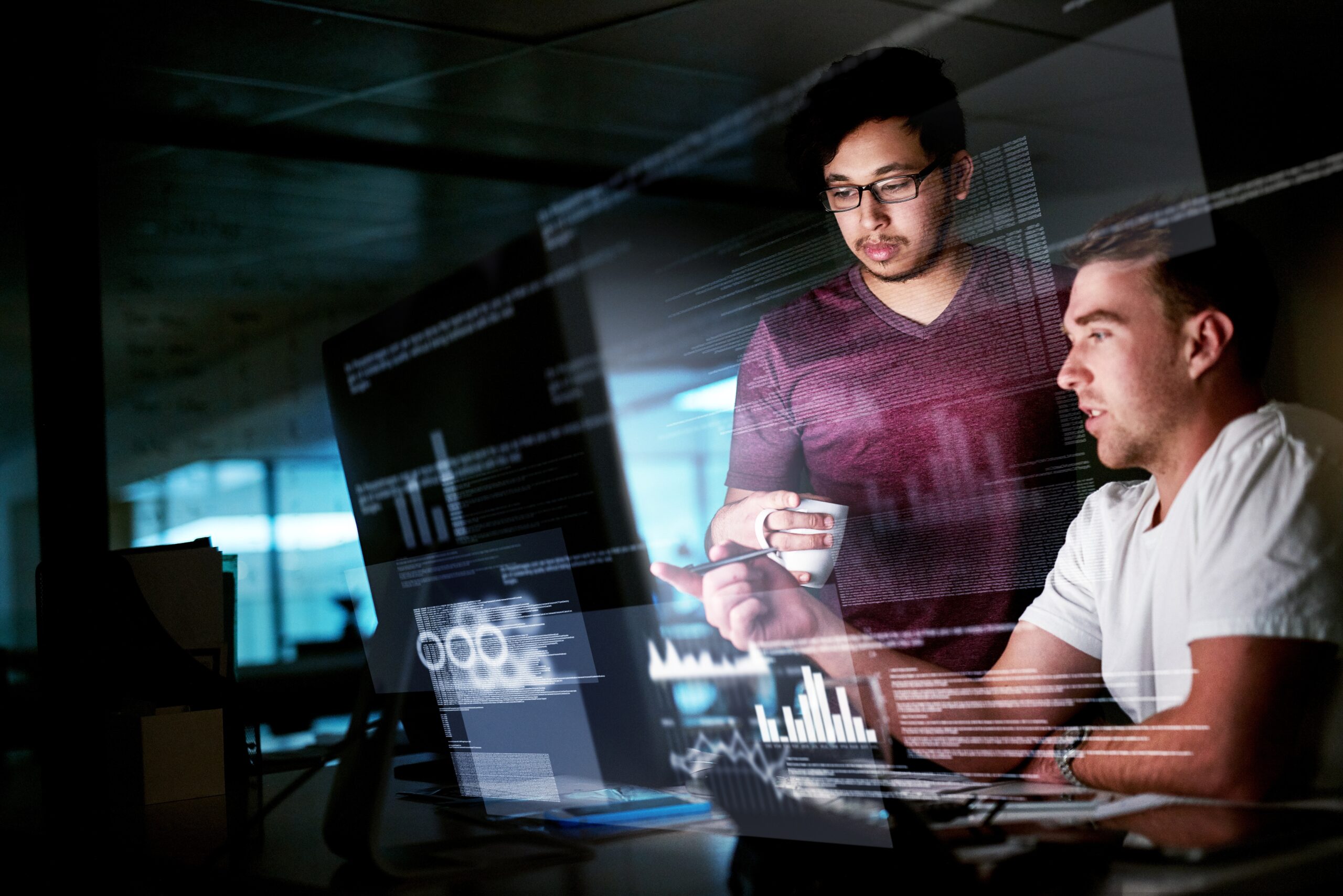
In a recent podcast, I spoke with Tod Parrella, Senior Product Manger for NX Design solutions, about the impact of AI on the design processes – specifically how it enables Generative Design for smarter solutions and wider design space exploration. You can listen to the complete episode here or keep reading for the full transcript of our talk.
Spencer Acain: Hello and welcome to the AI Spectrum podcast. I’m your host, Spencer Acain, and in this series we talked to experts all across Siemens about a wide range of AI technology and how they apply to various products. Today I’m joined by Tod Parrella to discuss how AI is driving automation and engineering and design.
Spencer Acain: Tod has worked for 16 years in the NX product management organization as part of the team that sets the vision, strategy and road map for the investments Siemens makes in the NX portfolio of products. To that end, artificial intelligence is a key technology being explored within NX not only as a means of improving how customers interact with the software but also to enable generative design and engineering practices.
Spencer Acain: So, Tod, to start our conversation off, can you tell us more about generative design and engineering work, and why it’s important?
Tod Parrella: Sure. Well, the topic of generative design has come to the forefront of engineering a few years ago now because what it can do in terms of helping our engineering design community find the best possible outcomes of their next generation products. So, it what generative engineering does is it expands the design space of possibilities for our customers that allows them to explore many more variables of options you have. Oftentimes you’ll have weighted criteria when you’re designing a product, you have often have many conflicting or requirements and multidisciplinary requirements that you need to incorporate into that design for how your what materials you’re going to use, what manufacturing method are you going to use, whatever the system, performance requirements, all of those things are so many variables to be juggling, and so as customers are looking to you know, find that next iteration of and performance improvement, or that next innovation in their design that they bring to market. They’re finding that generative engineering and leveraging artificial intelligent algorithms can help them in that process. And really, it’s about exploring hundreds or thousands of iterations versus single digits or you know or a dozen iterations that they typically do today.
Spencer Acain: So you’ve mentioned this a little bit now that obviously your generative design and engineering capabilities are built with AI can you expand on that a little? How are you using AI to enable generative engineering practices?
Tod Parrella: Well, our key technology inside of Siemens is our AI engine that essentially leverages a suite of algorithms to explore the design space, and so the challenge is with some of these engineering and generative problems is that the design space can be quite broad. So typically what a user does without AI is choose a methodology to explore the space and that can be quite difficult because there’s sort of macro algorithms that look at the bigger picture. And then there’s, you know, sort of more localized micro algorithms that that, that focus in on a particular parameter or variable and that becomes a very complex problem for the engineering community to know which, quite frankly, which is the right tool to use leveraging AI that like we do inside of our AI engine. That AI engine, you know, figures out which is the best approach to take for you. And it figures that out based on exploring the design and then taking that feedback from a design and then making interpretations of how it might need to change this approach to zero in on some of the some of the key objectives that you’re looking to looking to me.
Spencer Acain: I see. So. It kind of sounds almost like you’re optimizing the problem of optimizing your design.
Tod Parrella: That’s probably it. Probably a good summary for sure.
Spencer Acain: So, we’ve been talking a lot about how AI can enable generative engineering and generative design now, but is this something you could do without AI? Is it something you been able to do in the past, or is it only possible now thanks to the advances in artificial intelligence?
Tod Parrella: Yeah. So, it really depends on to what degree of generative engineering you’re at. There is sort of a skill of ability and complexity in the world of generative engineering, and so the basic form of generative engineering is what we’ve seen out there in the market, which took a lot of, really caught a lot of interest because of the visual aspects of what topology optimization was doing. So, topology optimizations are just as a form of generative engineering. It’s one aspect of generative engineering. It leverages special algorithms to optimize the designs based on users that have inputs and constraints. That’s generative engineering. Is it using artificial intelligence per se? Yes and no. You know it’s using something specific. It’s using a method, a specific method to run through and look at an optimization on a set of problems. So that would be an example where you’re not leveraging some of the aspects of learning in that process, but you are still doing generative engineering. If you’re getting into the bigger scale and complexity of what’s possible through AI. Yes, you start incorporating capabilities to learn from your previous designs or learn from learn from designs that exist out in the world today and that just that’s sort of another level of what generative engineering can offer.
Spencer Acain: All right. So it sounds like it’s a bit of multifaceted there, both in the approach and in the technologies under the hood.
Tod Parrella: Yeah, absolutely. And you know we bring capabilities to the market that covers all aspects of that and really, it’s just dependent on you know what’s most suitable for our customers needs and typically customers evolve with their learning of how to use these technologies and how they can leverage them to increase, you know, expand and grow and scale the possibilities of what they can achieve with our solutions.
Spencer Acain: Well, it sounds like with generative design and engineering, you’re a lot of the burden of exploring the design space and creating designs has been shifted from the engineer to the system, the artificial intelligence under the hood. So how do you see the role of engineer changing with this new kind of AI driven design paradigm that we’re developing here?
Tod Parrella: Sure. Well, if you look at the role of engineers and designers today, and analysts, I would say you know, they’re typically key cogs in in that process of actually designing and validating each step of the stage of the design. So typically, you designers make products, they get analyzed and validated through simulation tools. Either designer level simulation tools or analysts level capabilities right where you have a multidisciplinary workflow happening. You also incorporate the manufacturing aspects of that through manufacturing engineers and so that’s a key part of the whole end to end workflow for developing products. Of the way that or you know the possibilities that artificial intelligence can bring to in the generative engineering approach can bring to design. It helps with automating a lot of that heavy lifting work, right? And a lot of that very specific and tedious work. And so through automation and by leveraging, you know, automation through these algorithms, the way that their roles change by moving towards a more generative approach to design is that they become more orchestrators.
Tod Parrella: In a sense, it frees them up to be able to think more of the real engineering problems and the real objectives or what you’re trying to achieve in your products, where you ensure that your setting and defining the correct set of requirements for all aspects of how the product needs to be developed so they become more orchestrators in defining those requirements and then they’re allowing automation and the algorithms to run through and drive and present the options back to you rather than having to go through all of those. All of those very manual steps that I mentioned in the beginning, which is essentially the current state of engineering design today.
Spencer Acain: Sounds kind of like you’re letting engineers get back to being engineers instead of just having to be pushing a lot of numbers and doing a lot of testing and stuff.
Tod Parrella: Yeah, for sure. I mean CAD technology, you know, our technology has revolutionized the way products are developed, right. But it also required people to become skilled experts and many, many aspects of various aspects of, you know, CAD and simulation software and technology and so, as we become smarter and present our tools as more engineering advisor based tools, we take that burden off of the engineer and like you said, open it up to them getting back to being more of what an engineer typically views themselves as. As you know, being their biggest priority, right? So yeah, the more that we can bring engineering intelligence into our tools along with automation, the more we’re really gonna help our customers and our companies really innovate further and faster.
Spencer Acain: So integrating AI in the design process has to be a big change for how things have been done traditionally, and I imagine it’s one that can’t happen in a vacuum. So how do you see the move to an AI driven generative engineering process changing the way that the product design process happens?
Tod Parrella: Yeah. So I mean, really what most companies are trying to achieve today are these closed loop workflows like so engineer and validate and manufacture in sort of a closed loop. And also I’d say at top of that adding in to getting data analytics out on the back end system performance side in the real world and then looping that bit back in and driving the new sets of requirements that you’re probably what you’re learning from the field. It’s again back into that loop of the design process. AI, you know, through the AI tools you can integrate, you know, multiple capabilities like you say it’s not only multi-objective but multi-disciplinary. So you have that ability to connect your design, your simulation and your manufacturing tools together into that process. And really again mentioning automation through that integration and automation, you’re able to drive that closed loop design process much more efficiently than what typically happens today. As I mentioned earlier, with multiples of those disciplines working in a more manual way.
Spencer Acain: So, we’ve talked a lot about how AI is kind of underpinning all of this new technology and the new ways that engineers can explore design space and design new products. But at the same time, this also means that engineers are going to have to start placing more of their trust in the algorithms and artificial intelligence systems that are underpinning the new these new generative design processes. So, what are you doing to kind of build that confidence in the solver in the system within the engineering community has who tends to be kind of hard headed about that kind of stuff and like to really understand what’s happening under the hood?
Tod Parrella: Yeah, that’s a good question and it’s not an easy challenge to overcome, of course. You know the real summary is you’ve got to build your AI technology on proven, you know, proven real world good design essentially. So when you talk about using machine learning, you know you could learn from many bad examples. So, the key aspect of that our engines that have been developed are based on proven real-world successes in the field. And so, in learning from that is key. So I don’t know. Yeah, as I mentioned, you know, we’re very confident in improving our technology through many, many use cases over many, many years that the technology is proving to give better performance and better outcomes in your products, and that’s the important aspect. It goes back to sort of the drop test simulation I mentioned to you before there. You know, there are people that used to see that and still question whether it was real, right? So, to your point, it’s a hard thing to overcome for engineers who really wanna see it first to believe it right?
Tod Parrella: But I think overtime and through over increasing confidence of our technology having been built on that proven infrastructure. It’s really the only way to get past that. Of course you have to have you have to have those real results, right? Because there are there are numerous experiences. thankfully I’m not aware of anything within Siemens within our technology, but there are numerous experiences out there in the market in the world that have given wrong results, right and that you lose the trust and confidence of your community. If that happens, right? and that also underscores the reason why engineers are still critical to even these advancements and technology in what we’re delivering to the market because at the end of the day. It’s still the engineer who is to validate and sign off on those on those designs, right? A computer is not validating and signing off on those designs, and so the engineering community, the engineering world is still critical to everything that we developed in the world today. And I foresee that to remain the case indefinitely.
Spencer Acain: So building on that idea of needing some early adopters for new technology, what sectors or industries are you seeing the fastest growth of generative design and engineering practices in?
Tod Parrella: We see the adoption of generative design quite heavily in the aerospace and the power generation markets for sure and I think the reason is primarily that they have some of the most difficult engineering challenge is to eke out performance in both capability as well as optimization of fuel usage quite frankly, right. So, if you take a jet engine, right, you’re always trying to be more powerful and more efficient, right, which includes using less fuel, to fly people across the world. So some of those aerospace and energy and utilities and power generation type workflows tend to be at the forefront of a lot of these technologies. Because of, you know what they’re trying to eke. So if they if they can get 10% savings or 5% savings, you know that’s that could be significant over time. Secondarily is obviously what we see in the automotive market. Again, anything in terms of transportation in general, where you’re you know you’re using fuel, right? And of course, with that fuel is changing, you know, energy sources are changing over time, but any, any place where you have to have to generate an energy, you use an energy source obviously is going to be most directly impacted by the possibilities of what we could deliver there and, in other cases, you might also look at things in the medical field and what we’re able to do there, I’m not saying that they’re you know, they’re definitely not the highest adopter of generative engineering, but improving patient outcomes can be something very interesting for how to leverage artificial intelligence and generative engineering for how to improve the bio mechanics of what we do in terms of implants and prosthetics into the user into the patient. So that’s just a sort of a really off there arbitrary, you know, sort of a side example, but the point is that there could be numerous upside benefits to leveraging this technology across a wide swath of industries. All of the nine industries that we go to market here at Siemens can be benefactors, some may be, you know, more leading adopters than others. And as I mentioned, who I believe that the leaders are. But all of them could really look at the application of this in a variety of ways and not just necessarily at the product design level, but it could be on the manufacturing tooling side, ways to generate more efficient tooling and manufacturing processes, and leveraging generative engineering for that. So the scope of possibilities in where you could apply generative engineering I think is really still in its infancy, we often look at it at the end product or the end design, but it could be leveraged quite frankly in numerous areas of design processes and engineering processes in our customers across a wide range of industries that we go to market in.
Spencer Acain: All right. Well, thank you for giving me that kind of off the cuff answer there. It was very informative actually. It almost sounds like the industries that are kind of adopting this soonest are the ones where even a small gain in efficiency or in performance could be a potentially large impact on like cost or business or operating conditions.
Tod Parrella: Absolutely.
Spencer Acain: So, we’ve talked a lot about how AI is being used to enable generative design and engineering, but is there anything else interesting that you’re working on that you like to share with us
Tod Parrella: Sure, and actually I think a lot of you know to answer this question, some of this, you can actually already see available in the market from in our products today and that’s our focus towards not just leveraging AI and machine learning technologies for generative engineering, but how can we apply it towards the daily productivity of our thousands of engineering users across the world? Right? Because not everybody uses a generative engineering approach, right? There’s many, many industries and workflows that really aren’t necessarily applicable to that, right? So, leveraging AI and machine learning towards how we could help improve our engineers daily use of our tools. You know all of our customers could get great benefit from that. And so, you’ve seen us incorporating this technology into our command prediction tools, our selection prediction tools. These are all of the way that you interact with our system with NX and SIM center and our other products. So where we’ve incorporated machine learning algorithms into NX as sort of a I guess you’d say a service or a foundational service that’s available in all of our products to leverage so that we, as I mentioned earlier, we’re leveraging and learning from our users daily interaction with the products and the applications of this are enormous because many of our customers are leveraging this technology to learn from sort of the expert and the knowledge base in those companies for how good designs are designed and produced in our tools. And by putting that machine learning capability on to those users desktops. It’s an amazingly powerful way to help with the ever evolving problem of trying to capture the knowledge of a wide swath of experience engineers that at some point, won’t be in the engineering workforce right and will need to be disseminating that information to the next set of engineers coming on board. And so, we’ve been applying, as I mentioned machine learning into a variety of capabilities and NX that can learn from your usage. The way you interact with the features and functions, the way that you select and design products where incorporating it into the way that you we’re designing, we’ve developed these designer level simulation capabilities. We’re incorporating machine learning into there to help educate that next set of user who’s not as familiar with this tool on how to essentially use the tool. So, the more we learn from past usages, we can start serving that up as sort of advisors or guiders, you could say to recommend to that new user, for example. Oh, I see you’re trying to do this typically, you would pick these types of things to constrain the part, or to build that functional feature, and the best possible way. So that’s really one of the areas where we see on our side incredible value and the possibilities of what artificial intelligence and machine learning can bring to our community.
Tod Parrella: We have, you know, command predict we have voice, you know, voice command prediction, right? So where you can actually leverage the use of our tools just through your voice, right, which is not just a powerful capability for maybe physically impaired people, but also just possibly maybe the way that you’ll want to interact with your CAD software in the future like Jarvis from Ironman, right, where you just you’re just talking to the system and telling it what you would like to do. And so we have actually various capabilities already available in the product of Voice Command assistant as I mentioned and some of the prediction tools there. And that to me is probably the most exciting. Several things that we’ve been working on recently that I think maybe, the community is not aware of it might find great interesting as well.
Spencer Acain: Well, that sounds all sounds just really exciting. Great, futuristic stuff that you’re working on to an extent, but I think that’s about all the time we have for this episode. So once again, I am your host, Spencer Acain, and this is the AI Spectrum podcast. I’d like to thank Todd Parella for joining me here.
Tod Parrella: I think Spencer, it’s great to speak with you and I appreciate the time as well.
Spencer Acain: Great. And that’s all for this episode.
Siemens Digital Industries Software helps organizations of all sizes digitally transform using software, hardware and services from the Siemens Xcelerator business platform. Siemens’ software and the comprehensive digital twin enable companies to optimize their design, engineering and manufacturing processes to turn today’s ideas into the sustainable products of the future. From chips to entire systems, from product to process, across all industries. Siemens Digital Industries Software – Accelerating transformation.