How AI is accelerating design space exploration transcript – Part 2
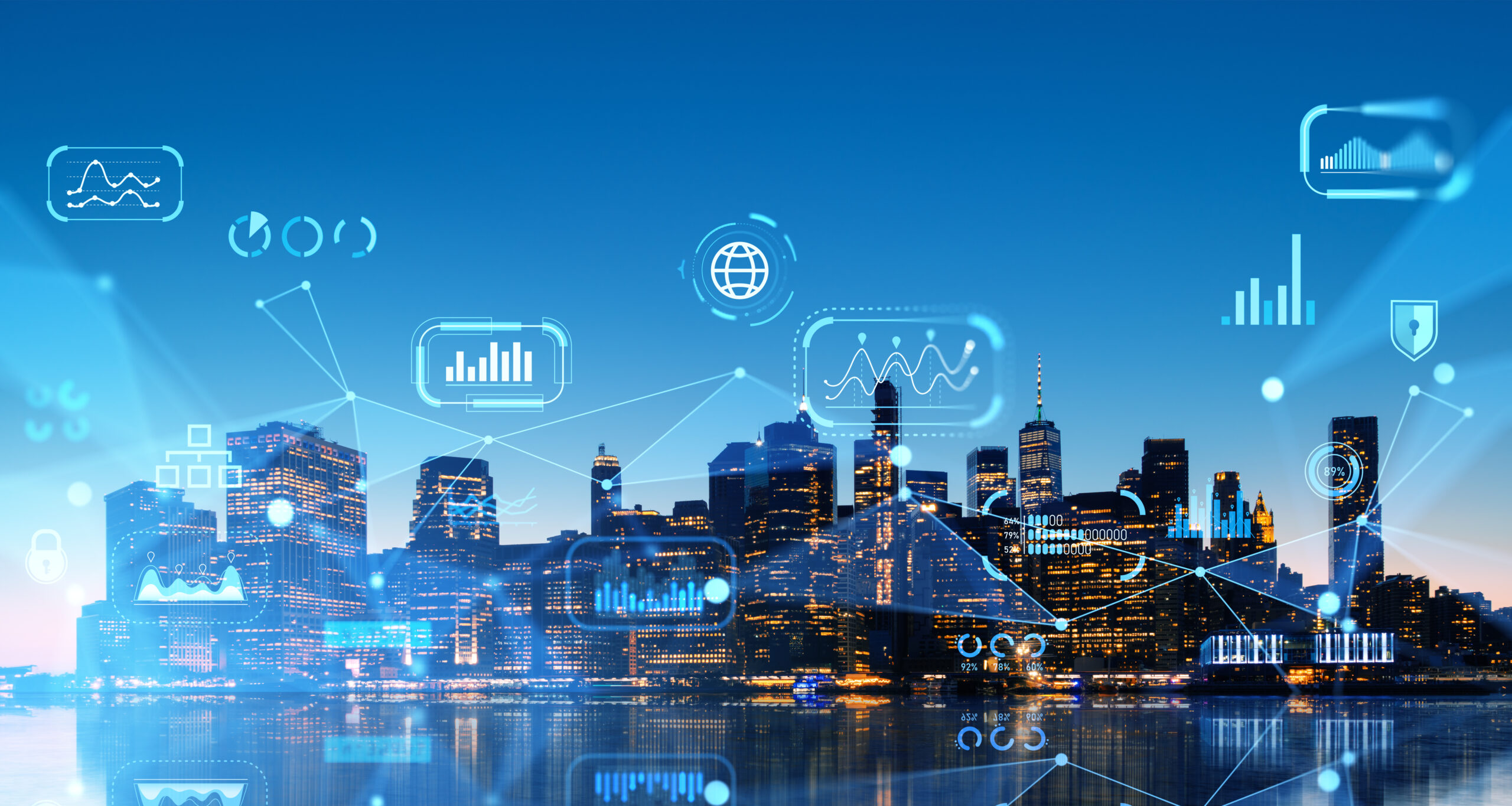
In a recent podcast, Dr. Gabriel Amine-Eddine, Technical Product Manager for the HEEDS Design Exploration Team, continued to explore the applications of AI in design space exploration while discussing some of the challenges he and his team faced in deploying AI solutions to that space. Check out a transcript of that talk here, and listen along here or at the link below.
Spencer Acain: Hello and welcome to the AI Spectrum podcast. I’m your host, Spencer Acain. In this series, we explore a wide range of AI topics from all across Siemens and how they’re applied to different technologies. Today, I am once again joined by Gabriel Amine-Eddine, technical product manager for HEEDS Design Exploration team. In the last episode, we discussed the ways that the HEEDS was using artificial intelligence to accelerate the design process. But can you tell me how you came up with this concept, because it’s an interesting, very novel idea, almost, because it’s like you have an AI that’s basically in here deciding, do I want to spend a lot of energy on this, do I not? And then it’s balancing, like you said, many, many different parameters to come to this correct conclusion. It’s an interesting way to approach the problem of designing something.
Gabriel Amine-Eddine: So, originally, this technology, if we go to the deeper levels of it, accuracy aware AI, what is that? And that is a good question in itself, because accuracy aware AI, or more commonly known as uncertainty aware, there’s subtle differences between accuracy awareness and uncertainty awareness, even though they’re applied to the same concept. But uncertainty awareness has existed for quite some time. There are models creating models, polynomial chaos expansion. They provide not just a singular prediction, they actually provide a level of uncertainty. So, you can imagine a dartboard, if you’re firing darts in the center, you fire one dart after one dart, after one dart, you’re never going to get exactly the same position as before. You’d be pretty good if you were able to do that. But if you did it 1,000 times, you’re going to get some scatter. And if you do that enough, that scatter relates to a distribution. And that distribution is going to have a mean and a standard deviation or a variance. And that variance is used to actually quantify the uncertainty. Is a way of saying that I want to be able to make a prediction as to how accurate I’m going to be, because you can only really be accurate or accuracy aware if you’ve got something to compare against. But if you were to say, well, these are all of the possible options that might occur, you can be aware of how accurate the model is going to be. And that’s translated to the different models that are state of the art these days. Bayesian Neural Networks are one such model. And those kinds of models have been used by some of our customers originally, in particular Siemens Energy, to learn from data that they’ve collected from HEEDS Design Exploration studies for different product families. And they were using these models to do purely surrogate-based optimization. So, there’s no digital twin concept within that, but you’re performing optimization on the surrogate model itself, one non-certainty where AI models themselves. But the challenge with that is that when you start, if you’re not updating the knowledge that these models contain, as you push more and more the boundaries of what the model is able to predict, it can only make predictions on data that it is trained upon. As soon as you ask it to make a prediction that is nowhere near any data points that it originally trained upon, it’s going to make an extrapolation.
And if you don’t have a characterization as to how uncertain or how accurate that prediction is going to be, you’re shooting in the dark. So, these models effectively provide an awareness and they can actually respond to… When you ask them to make a prediction within the field of data that is previously seen, you could expect to have a very low uncertainty or a high accuracy, one is the inverse of the other. Whereas if you ask it for a prediction in no man’s land, so to speak, nowhere near any data points, the uncertainty is going to be quite large because it doesn’t know whether or not there’s going to be… It has no reference point effectively, or it’s far away from the nearest reference point. So, that’s what we really mean by accuracy aware. Siemens Energy were the first to utilize the concept of uncertainty awareness for their product design, their turbine blade designs. And this originally started off as a collaboration between Siemens technology, the DAI division, the HEEDS team of sponsors, and we’ve evolved the technology through different readiness levels, and now we’re bringing it to the market for all of our customers to benefit from.
Spencer Acain: All right. That sounds really interesting. So, what you’re saying is that the model itself is aware of its own accuracy and that it can provide a good level of information on that to the people who are using it so that they can know how good or bad the result is. And in a sense that almost it’s building a level of trust in the model. Because in my mind, you’d really have to have a lot of faith in this AI algorithm to come up with the right designs and to know that it’s selecting the right type of simulation, the full one, the AI one, that it’s going in the right design direction, so to speak, in its capabilities. Is that accurate? Is that how you see it too?
Gabriel Amine-Eddine: Yeah, absolutely. Because fundamentally, you need to trust your digital twin simulations to provide you the right answers-
Spencer Acain: Absolutely.
Gabriel Amine-Eddine: … bottom line. And despite my background is from the computational perspective, but even then, I would consider the experiment as the ground truth. That is what we’ve got as a reference. But going back to the CE simulation, you’re going back to AI models, we want to make sure that they are trustworthy, so that if we pass them to a colleague, our colleague can say, “Yeah, we trust it. It’s going to give us the right answers.’ And we want to be able to know whether or not the model is going to give the right answers at the end of the day. So, a model that is able to tell you not only just a singular prediction, but also variance is always very informative. And the funny thing is, when I first encountered these models, it was quite interesting to see where every time you ask these models for a prediction, they’re actually going to give you a different answer. You might be thinking, what is the actual value of that? But going back to the dartboard example or one of those circus games, circus stall games where you’re trying to fire a cork at a target to knock over some things, you’re going to have a number of different trials. And that’s what builds up a picture as to how well the model is going to be suited when it’s been asked to make predictions in context to what it’s been trained to actually effectively simulate. Because even CE simulations, 20, 30 years ago, they were considered surrogate models, meta models to some extent. We’ve really improved the capabilities of a lot of simulation tools these days.
Spencer Acain: All right. I mean, it sounds like that’s an important step too, that you’ve really thought about this even during the conceptual design of these tools, the ability to, like you said, just hand it to a colleague and then that person knows that this is good, this is fine, this is usable as is, I don’t need to fact check this. But that is all the time we have for this episode. Once again, I have been your host, Spencer Acain, joined by Gabriel Amine-Eddine on the AI Spectrum Podcast. Tune in next time as we continue to explore the exciting world of AI.
Siemens Digital Industries Software helps organizations of all sizes digitally transform using software, hardware and services from the Siemens Xcelerator business platform. Siemens’ software and the comprehensive digital twin enable companies to optimize their design, engineering and manufacturing processes to turn today’s ideas into the sustainable products of the future. From chips to entire systems, from product to process, across all industries. Siemens Digital Industries Software – Accelerating transformation.