Making your historical NVH data work for you
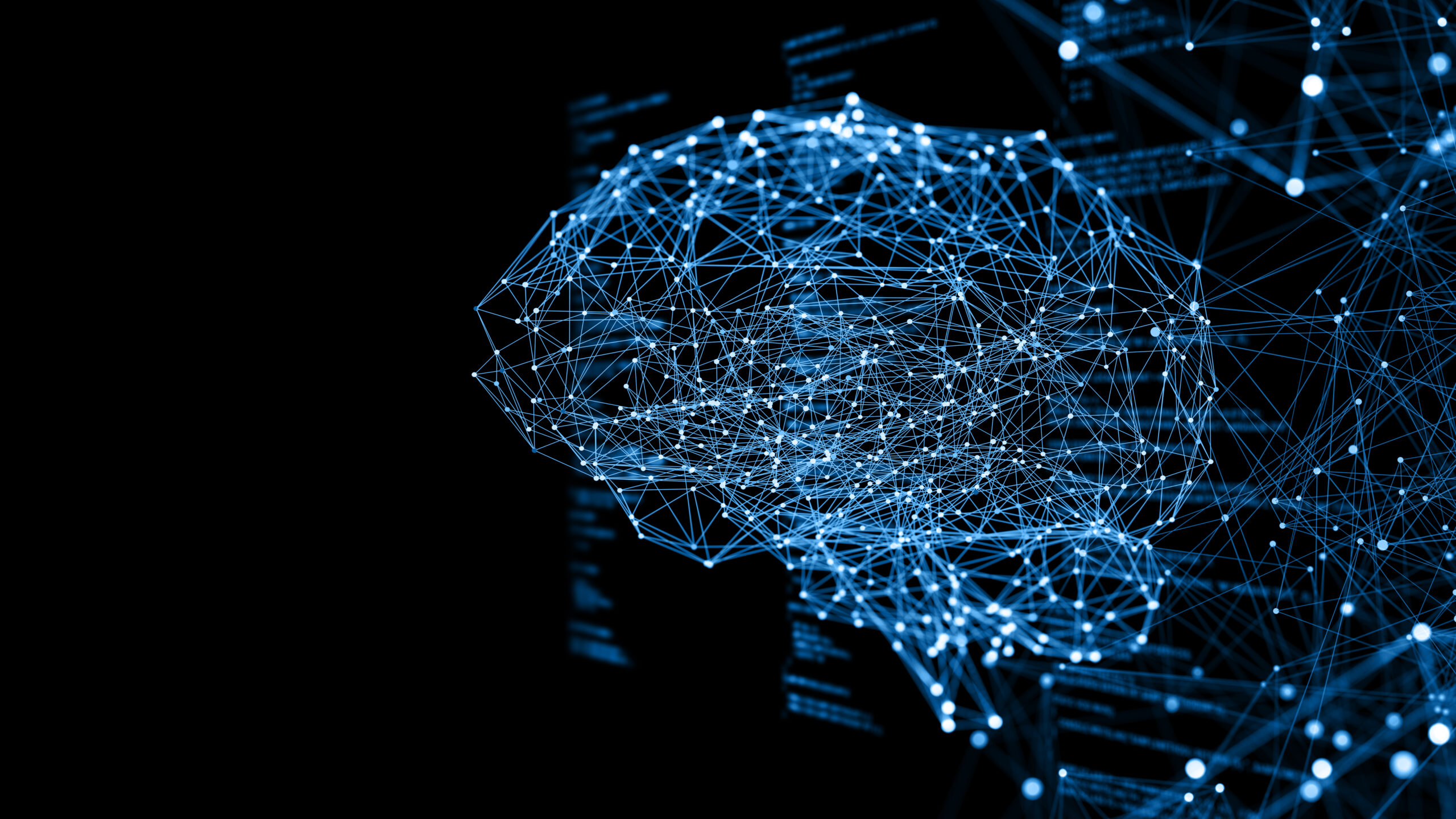
This article was co-written with Wouter Vandermeulen, senior project engineer for Simcenter Engineering Services.
As a child growing up in sunny southern California, I spent many afternoons reading in a lounge chair beside our backyard pool. Mysteriously, I would often find curious treasures hidden under my cushions: a handful of nuts from our nearby trees.
I decided to find the source of these nuts. I stood watch at my bedroom window, took time logs, and A/B tested whether different locations for the lounge chairs affected the number of nuts I would find under the cushion later.
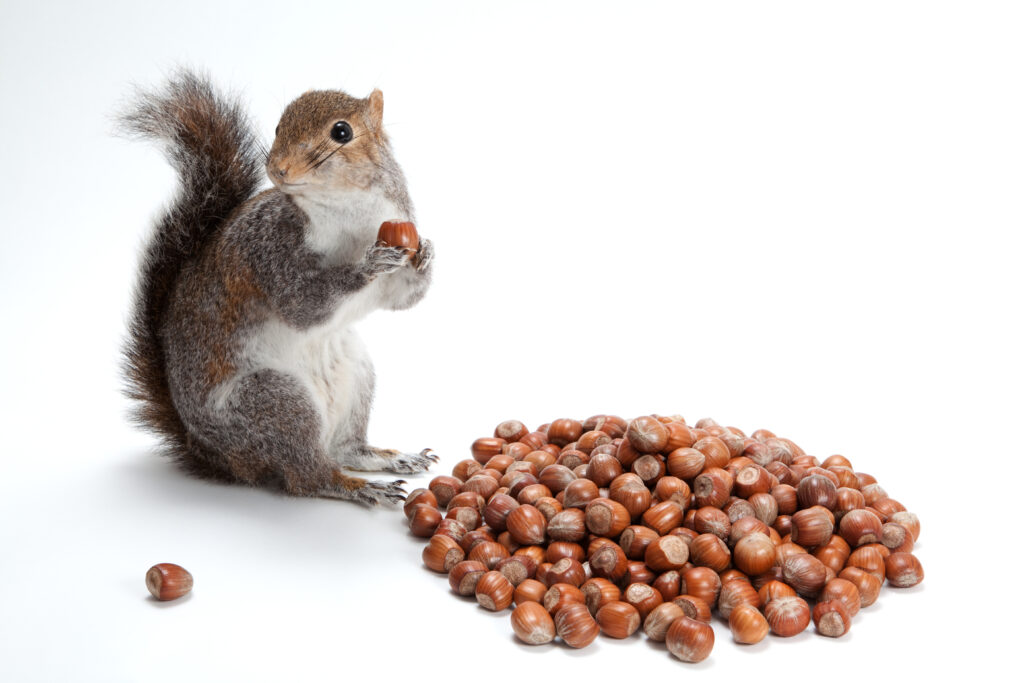
After days of observation, I watched a fat, brown squirrel run across our backyard, carefully lift a lounge chair cushion and deposit a handful of nuts there. For weeks, I watched this squirrel laboriously collect nuts from our yard, then return to the pool to hide them in the lounge chairs.
There was a running joke in my family that the squirrel had forgotten where it had placed its nuts, as we would find them under the cushions months later, dutifully waiting for their owner to return. I pitied the poor squirrel, knowing how much time and effort it had wasted collecting those nuts, only to forget about them and start a new stash all over again later.
As it turns out, we humans are not so different from my backyard squirrel.
Forgotten automotive NVH data
In the automotive engineering world, engineers painstakingly collect test data in order to develop a new vehicle. OEMs perform an extremely high amount of NVH testing, for instance, to assess the road noise inside the car when driving over a specific type of road surface. This noise is the result of a variety of design decisions made by the OEM, including type of car, tires, suspension, and more.
After the development of a new vehicle is finished, these test results go onto the (data) shelves and, like the nuts in our story, are rarely revisited. However, these resource-intensive test results are a hidden treasure. They are the conclusion of a complete development campaign combining design decision and in-house expertise. What if, using machine learning, you could dig through all that NVH data and retrieve your OEM road noise DNA, then reuse it for any future vehicle?
This is exactly what the engineers in our Simcenter Engineering and Consulting services group have done for their own database.
The promise of machine learning technologies
Combining automotive test data with machine learning technologies creates limitless possibilities. The size of the NVH data (for hundreds of vehicles at OEMs) and the consistent method of testing each vehicle make it an ideal metric to apply machine learning.
The automotive industry is already exploring the potential of machine learning. Volkswagen has developed a generative design process that uses AI to keep its engineering requirements but reduces material and weight. Audi has applied machine learning to its production process to optimize quality inspections by recognizing and marking tiny cracks in sheet metal parts. Machine learning is also being applied to vehicle entertainment systems at Mercedes-Benz to “revolutionize” the cockpit by learning what the user likes.
How can I apply machine learning at my company?
For engineers looking to apply machine learning to their automotive test data, the most straightforward method is clustering. Clustering allows you to determine whether the new measurements match with previous measurements. It also helps to determine whether the current measurement is an outlier. If so, it tells you if you need to check whether the test setup is functional, or if a sensor is broken or incorrectly installed.
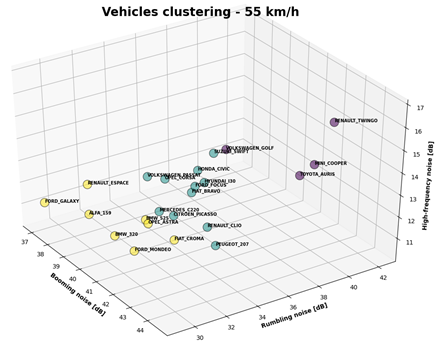
Additionally, using this method can enable you to predict the NVH results based on the vehicle description, similar to defining the vehicle at the start of the development (ie the car is an SUV, has this wheelbase, this suspension, etc). With this prediction, you can begin to finetune your new design based on your own OEM knowledge. You can start looking for solutions if your vehicle is not reaching target performance and use historical testing knowledge to aid in this search.
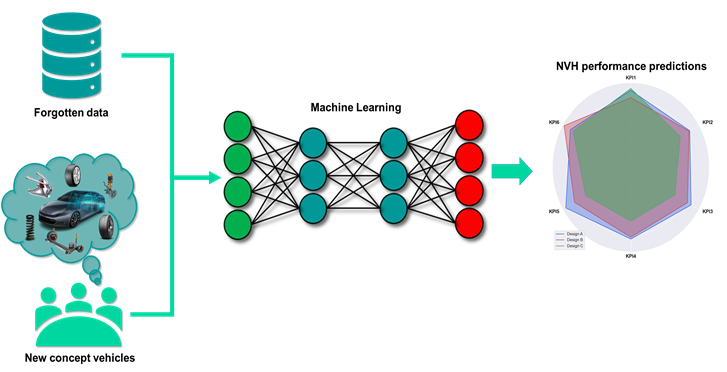
Finally, this method helps to pinpoint interesting benchmarking vehicles for target setting. When development for a new vehicle begins, the targets for NVH performance (and targets for other areas) need to come from a source. A standard solution is to look at competitor vehicles and record the NVH results. With our machine learning approach, you describe the competitor vehicle and evaluate what the ideal NVH performance would be if you were to build it yourself. If the competitor NVH results are much better than the results from your own experiments, you know that this car is worth investigating more closely.
Why would we need machine learning for this?
If a vehicle is described by a few parameters with only a few NVH results, a human could easily determine this relationship by hand or with some fancy spreadsheets. Unfortunately, new vehicle development is rarely, if ever, this straightforward. Imagine trying to apply the human-only method to 50-100 vehicle parameters with multiple NVH results for tens to hundreds of cars. Your average human’s eyes would eventually glaze over.
While machine learning in the automotive industry is still in its early stages, its potential remains huge. By applying machine learning to automotive NVH data, companies can regain valuable engineering time from tedious, time-consuming evaluations and allow them to focus on making better cars.
The best part about machine learning: It gets better every time.
Automate vehicle NVH validation using a data-driven approach
Alessandro Lepore, Business Development Manager at Simcenter, presented a webinar designed for NVH engineering communities, from experts to analysts, who seek smart solutions to speed up NVH data validation. He explained how to leverage historical NVH data by using artificial intelligence and machine learning techniques to deploy automated processes. Check out how data-driven NVH analysis enables you to shorten the development cycle and increase overall vehicle and process efficiency.
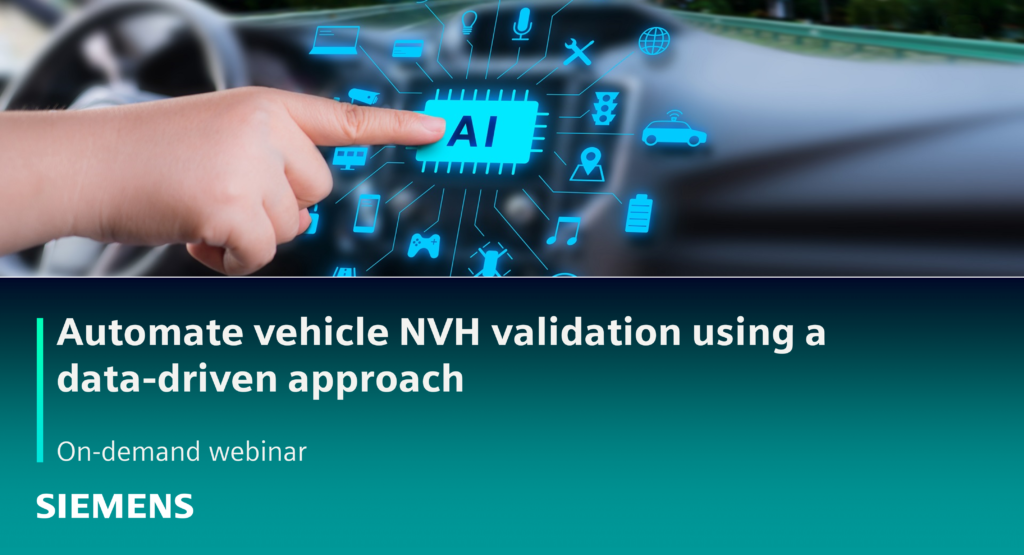
To learn more about Simcenter Engineering’s AI and NVH solutions, check out these related resources:
- Driving performance engineering using artificial intelligence in automotive: On-demand webinar
- How artificial neural networks aid in mechatronic system development
To get in touch with Simcenter Engineering, email us at engineeringservices.sisw@siemens.com.