AI-Enabled CAD: Enhancing Design Efficiency with Siemens’ NX
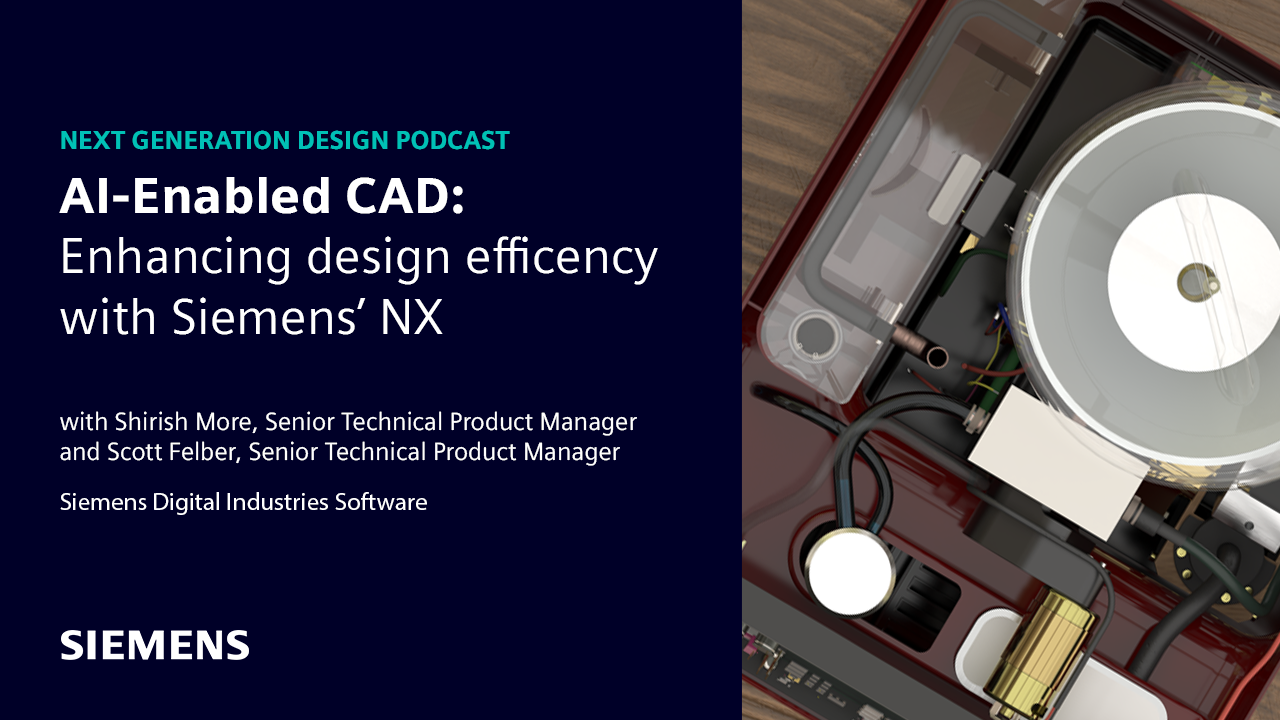
How are AI, LLM, and machine learning reshaping the future of CAD and mechanical design? What advancements can we expect in product development with the rise of AI-driven solutions?
In this episode of the Next Generation Design podcast, host Greg Arnot sits down with Shirish More, Senior Technical Product Manager for NX Architecture, AI, and PLM SaaS transformation, and Scott Felber, Senior Technical Product Manager from the NX Customer Success marketing team. They delve into how AI is revolutionizing the CAD landscape, transforming traditional design practices, and driving new levels of efficiency and innovation in engineering workflows.
Shirish and Scott discuss the evolving landscape of AI in mechanical design, from automating repetitive tasks to integrating advanced capabilities like generative design and real-time simulation. Discover how Siemens is leveraging AI and large language models to improve user interactions and deliver cutting-edge solutions within the NX suite, to empower designers to work smarter and bring products to market faster.
Find out how Siemens ensures data security while implementing AI, and how their approach allows companies to harness the power of AI without compromising intellectual property. Plus, hear about the impact of personalization in CAD software and how AI is creating a more intuitive, user-friendly design environment in NX CAD.
What you’ll learn in this episode of the Next Generation Design podcast:
- What makes AI, machine learning (ML), and deep learning different? (2:00)
- How does Siemens harness AI without compromising IP security? (4:45)
- How Siemens integrates AI into NX to enhance productivity and user experience (6:50)
- Will AI replace engineering jobs? (15:10)
- The future of AI generative design and conversational interfaces in CAD software (17:25)
- AI features within NX and how they will evolve (22:10)
- AI’s role in NX Molded Part Designer (27:45)
You can always listen to the Next Generation Design podcast right here, or wherever you do podcasts.
Read the summary or watch the video version of this episode: How is product design changing with AI in CAD? | The role of AI in NX CAD
Listen to or read the next episode of the Next Generation Design podcast: Designing Formula SAE Cars with Rensselaer Motorsport Releasing Tuesday, December 16, 2024
Listen to or read the previous episode of the Next Generation Design podcast: From Concept to Creation: The Importance of Openness in Siemens Software
At Siemens, what we are trying to do with AI is not only a way to copy what humans do, but to actually help them do more by automating tasks, making smarter decisions and providing insights that predict what might happen next.
Shirish More, Siemens Digital Industries Software
Connect with Greg Arnot
Connect with Scott Felber
Connect with Shirish More
Podcast transcript for AI-Enabled CAD: Enhancing Design Efficiency with Siemens’ NX
Shirish More: AI can analyze vast amounts of data in real time, and it can provide actionable insights, which businesses can then use to operate much more efficiently. So, with the current trend, it’s obvious that customers are going to start asking questions like, “Okay, how can we start leveraging AI?” With the recent advancements in computational power, data availability, and the algorithms that are improving every day, AI has become much more accessible and scalable that now, users or customers are saying, “Well, yeah, we need to start leveraging AI; otherwise we’re going to go out of business.”
Greg Arnot: You’re listening to another episode of the Next Generation Design podcast. I’m your host Greg Arnot. On today’s episode, I’m joined by Shirish More, Senior Technical Product Manager for NX Architecture, Artificial Intelligence, and product lifecycle management SaaS transformation, and Scott Felber, Senior Technical Product Manager from the NX Customer Success marketing team. Before we begin, let’s meet today’s guests. Shirish, Scott, welcome to the podcast.
Shirish More: Thanks, Greg.
Scott Felber: Thanks, Greg.
Greg Arnot: I think it may be fair to say that there is some confusion around what artificial intelligence actually is and how different people, possibly from different generations, might perceive or define it. Shirish, can you define what AI is from the Siemens perspective?
Shirish More: So, AI, or artificial intelligence, in simple terms, is when machines are designed to think, learn, and solve problems like humans. At Siemens, what we are trying to do with AI is not only a way to copy what humans do but to actually help them do more by automating tasks, making smarter decisions, and providing insights that predict what might happen next. At a high level, that’s what we are trying to do. We are not just trying to copy what humans do but help humans achieve mechanical product designing-related tasks.
Greg Arnot: Can you comment on the difference between concepts like AI, ML, and deep learning?
Shirish More: Yeah, people often confuse AI with terms like machine learning and deep learning. At a high level, machine learning is a type of AI where systems learn from data to make decisions or predictions without being specifically programmed for each task. Deep learning is more advanced than machine learning, wherein we use layered neural networks to recognize complex patterns and then make decisions. So, at a high level, AI is an umbrella or a big idea, and machine learning and deep learning are the techniques that make it happen.
Greg Arnot: Scott, why do you think AI is so popular right now?
Scott Felber: The biggest reason that I see AI is really popular right now, especially in the mechanical design CAD space, is because what we’re trying to do is eliminate the repetitive barriers that many design tasks involve. We’re trying to eliminate those tasks that can be predicted and known from your workflow, so you can concentrate and think more about the engineering you’re working on. So, to me, today, AI in our production release is all about increasing the productivity of designers.
Shirish More: What has happened is AI, in recent days, has also gained immense popularity due to its transformative impact on productivity and efficiencies across various industries. I joke a lot with customers—like, a couple of years back, we used to get an interaction going with them on AI, but they used to always say, “Well, yeah, we’re not there yet.” But now, since the introductions, or the way customers—or users, in fact—have started using smartphones, for example, or with the introduction of ChatGPT, all of a sudden they realize the potential of AI. They realize that AI now has the ability to automate repetitive tasks. They understand how AI can analyze vast amounts of data in real time, and it can provide actionable insights, which businesses can then use to operate much more efficiently. So, with the current trend, it’s obvious that customers are going to start asking questions like, “Okay, how can we start leveraging AI?” They have all this knowledge from all these years—how can they take advantage of that knowledge and other things. So, in summary, with the recent advancements in computational power, data availability, and the algorithms that are improving every day, AI has become much more accessible and scalable that now, users or customers are saying, “Well, yeah, we need to start leveraging AI; otherwise we’re going to go out of business.” So, I see immense potential going forward as to how AI can contribute towards mechanical product design tasks.
Greg Arnot: How does Siemens add value to today’s Large Language Models?
Scott Felber: To me, today’s Large Language Models in traditional AI are based on text, photos, videos, and stuff. What we’re doing at Siemens is we’re taking what we call the modalities of this data, and we’re including engineering data, manufacturing data, electronic design data, and also data from the shop floor that we can now help build that knowledge base, which they can then go ahead and learn from.
Shirish More: To what Scott is saying, the framework that we are putting together we are designing it to integrate seamlessly with our existing digital twin, IoT, and industrial automation solutions that we are already putting together. It adds significant value by adding AI capabilities—like large language models and conversational interfaces, leveraging those large language models. Once it becomes part of our product lifecycle, design tasks, and other processes, it’s just going to help improve the overall efficiency and productivity for the end users.
Greg Arnot: With AI, I know security is a big concern for our customers. How does Siemens keep customers’ IPs secure?
Shirish More: We are taking a multi-layered approach to protect our customers’ IP or intellectual property. We ensure that the AI models are trained within a secure environment, and any data exchange that happens between the customer’s location and our AI/ML services, the data is exchanged in an encrypted manner to prevent unauthorized access. Furthermore, we have traditionally implemented strict access controls, and we also make sure that we have audit trails to monitor and safeguard sensitive information—not only throughout the product lifecycle but as we start introducing AI components, we are making sure that these security-related approaches are true towards customers’ IP as well. So, what we are doing, Greg, is giving customers the option to perform data extraction and encryption on-site or on-premises and then use our secure mechanism to train customer-specific data models or machine learning models. We are putting all these frameworks together, which will allow our customers to leverage their own IP for training machine learning models and improving their productivity using the knowledge they have from all these years.
Scott Felber: On top of that, there’s one other big part about that security model. When the data is managed within our Teamcenter environment, that environment also has an extra protection layer that controls who can and can’t see the data. For instance, you may be a day-to-day designer, but we will protect that data stored in Teamcenter from, say, the research and development department so that those who don’t need access don’t get mixed in early on and start releasing things ahead of time. So, there’s really almost a secondary level of how we protect intellectual property—even within the company itself—not just from the outside world.
Greg Arnot: Can you tell our listeners about some of the advanced technologies that Siemens is planning to bring on board NX?
Shirish More: Siemens is continuously evolving NX by integrating advanced technologies that leverage AI and machine learning. We were the first ones to introduce adaptive UI or command prediction, and since then, we have introduced a number of capabilities that leverage advanced AI techniques. Some of the exciting developments going forward that we are going to work on include the integration of generative AI, which will allow engineers to explore a broader range of design possibilities. For example, it will automatically generate optimized designs or alternatives based on specific constraints and requirements. So, that’s one area where we are heavily focused. Additionally, we are looking into incorporating, as we discussed earlier, large language models to enhance user interaction, enabling engineers to ask questions, get assistance, and manipulate and refine designs using natural language. These innovations going forward are aimed at reducing the overall product development life cycles, improving overall product quality, and making the design process more intuitive and aligned with the future of engineering. That’s where we are heading, Greg, with what we are trying to bring inside NX when it comes to AI.
Greg Arnot: Shirish, before we talk more in detail about NX specifically, I’d like to talk about AI in CAD generally. What trends are we seeing now with AI in CAD, and how do you see AI shaping the future of CAD?
Shirish More: The future of CAD is very well poised now to be heavily influenced by AI, based on the questions that I’m getting from customers and users. The reason I say that is that making product design using NX or any other MCAD software needs to be more intuitive and efficient, and this process needs to be more efficient and collaborative. We anticipate a shift from traditional modeling to more of an AI-driven workflow, where the software—or, in this case, NX—not only assists but actively participates in the overall design process. So, I’m expecting that going forward, AI will enable real-time design suggestions, real-time simulation and validation, reducing the need for physical prototypes or iterations using labs and whatnot. AI is going to be really handy in these cases, where it can quickly parse through a lot of historical data. When I say “quickly,” it’s a relative term because compared to where we were a couple of years ago, now that we have the option of running high-compute machines and whatnot, it’s much easier for us to parse through large amounts of data, which will help us enable real-time simulation and validation. Additionally, AI is going to be much more integrated with the things we are introducing in NX—from selection to suggestions and recommendations. I expect AI to create a much more personalized design environment. What I mean is AI is going to enable users to have a much more personalized design experience, where the software adapts to the individual user’s needs, understands the user profile, preferences, and work habits, and, in turn, starts suggesting or guiding the designer, making the overall process much more enjoyable and efficient from the user’s perspective.
Scott Felber: It’s really about that real-time validation as someone is designing a part. To notice that you may start to be going down the wrong path to meet certain design criteria and notify the user along the way and say, “Hey, have you considered this, this, or this, based on the design you’re working on?” For instance, maybe you have two or three parts that could be combined into one for a design-for-manufacturability study, or maybe you’re planning to fasten the parts together one way, and it suggests, “It would be better if you fasten them together this way.” Things like that really assist the designer in quickly getting the design done. Because as we said, the biggest thing our customers are asking us for is to increase their speed to market to get their products done first. I think the single biggest thing with AI shaping the future of CAD is that today, we’re all talking about AI front and center. AI is doing things. It kind of goes back to the last question we just discussed. In the future, I see AI sinking into the background and just doing its thing unknown to the user; it just becomes second nature and becomes part of your daily tasks and workflow. You don’t even realize it’s AI; it’s just helping you design faster. That’s what I really think the future is going to look like.
Shirish More: To add to that, it has to be seamless. Nowadays, when we use smartphones, we hardly notice that there are so many AI aspects—from simple auto-completion to suggestions based on how I use things on my smartphone. So it has to be seamless. I see, going forward in CAD, that since we introduced selection prediction, users are now getting so used to those predictions that they don’t even realize NX is predicting what they should select, and they just go with the flow. So, I see, going forward, things are going to turn up in MCAD, especially as agents are actively monitoring how users are using the software to design their products, and in turn, doing things that users will start expecting AI to do. We’re going to reach a point where it’s going to be more of a collective intelligence thing, where the user is working with the system, and AI is learning, establishing the context, and then guiding the user in designing the product. I see a huge potential going forward for AI within NX, as well as in the overall MCAD industry.
Greg Arnot: As engineers with mechanical engineering backgrounds, what are you hoping to get from AI in CAD?
Scott Felber: To me, the single biggest thing is the elimination of redundant tasks—the stuff that makes our job boring every day. For example, if you’re blending parts, putting rounds and blends on parts at the end of a design, it’s very click-intensive. With selection prediction now, I pick a couple of edges, and it highlights all the other edges I’m probably going to want to blend. If it’s correct, I just do it, and I’ve saved myself tons and tons of clicks. That’s what I’m really looking forward to on day one. And then down the road, as we just talked about, it’s going to be that validation and the ability to help me with my design, so I don’t have to stop and look things up, maybe. It’ll say, “Hey, you may want to do this,” or “You may want to go ahead and do that instead.” That’s really what I’m looking forward to.
Shirish More: To add to what Scott said, I’m particularly excited about AI’s potential to reduce the time spent on routine tasks, allowing designers or engineers to focus on innovation and solving the real problem at hand is where AI is going to be really helpful. I’m also hoping that AI will continue to evolve to a point where it can offer real-time guidance and intelligent suggestions based on the context of a design. The reason I say this is a bracket, for example, whether it’s a reinforcement bracket or a bracket used for other purposes; knowing the context and then, based on that context, guiding the designer to accomplish a task is going to be a game-changer. That’s one area where, as a mechanical engineer, I think AI is going to be really useful. I’m also looking forward to having a more seamless integration between different stages of the product lifecycle—from design to simulation to manufacturing. Having the power to understand the context or recognize the intent, and then, based on the lifecycle of a product, suggest things and automate tasks that will speed up the overall design process and reduce time to market is where I think AI is going to come in really handy going forward, Greg.
Greg Arnot: A common refrain that’s often heard is that AI is going to replace our jobs. Do you think engineers have anything to worry about? Can AI really replace us?
Shirish More: It’s a valid concern—not only from when I talk to customers. It’s not a concern that whether AI will replace them; the concern is that are you touching information or content that you’re not supposed to? That’s the concern. But the reality is that the way we are putting the framework together, we have incorporated a number of options where the user gets a preview before committing to a change that’s suggested by AI. We are putting some architectural components in place that will allow the user to gain trust and confidence with an AI-driven assistant. So, in reality, what’s going to happen is AI is not here to replace engineers; it is here to support them and make engineers and designers much more efficient. Once they start realizing that AI is really handling repetitive, time-consuming tasks and allowing them to focus more on product development, creative, and complex aspects of their work, they will start adopting it. While AI can assist in generating design options or automating certain processes, it lacks the contextual understanding of the intent of the design. It’s always going to be a human who drives that aspect of their design. So, to be honest, I don’t see AI replacing engineers or designers. What I see going forward is AI helping humans or engineers become more productive, innovative, and efficient rather than replacing them.
Scott Felber: You touched a lot there on increased productivity for engineers. As we talk, not just at the engineering level, but as we go up levels in the organization—to engineering managers, VPs, and so on—what they really want is for their entire workforce to be more productive. So, what we’re really doing is enabling that increased workflow productivity so they can get more things done with the same amount of people or more things done in a shorter amount of time. There’s that balancing act at a management level, too, to help increase productivity, which is what all the executives we talk to day-to-day want for their people.
Greg Arnot: Scott, Shirish, I wonder if you can talk to me a bit about generative design– one of the earlier uses of AI in CAD software. What role do you see it playing going forward?
Scott Felber: People complicate it, but to me, it’s a very simple concept at a high level. In generative design, I give the system the desired inputs I want—whether that’s where things need to attach, a certain volume it has to fit within, or what kind of loads and stresses will be on it. Then, I let it go ahead and do the work and say, “This is the design I would generate based on that set of inputs. Here are the outputs I can give you.” That’s it, to me, in its simplest form. A lot of people want to complicate it and talk more about it, but to me, that’s it.
Shirish More: For me, the purpose of generative design is to explore and optimize designs that might not be immediately obvious to a designer. There are solutions that might solve a problem, but day in and day out, designers are taking traditional approaches. Whereas from an AI perspective, we normally see solutions that designers might not have thought about. So, generative design, for me, is a framework that allows engineers to consider a broader spectrum of possibilities, leading to innovative, lightweight, cost-effective solutions, keeping the requirements and specifications in mind. We have seen numerous times how the generative design approach is valuable in industries where performance, material efficiency, or other input criteria are critical. Hence, generative design is really powerful, and leveraging AI for generative design use cases is going to be an awesome thing going forward, Greg. We have introduced it in a number of use cases inside NX, but I see a lot of opportunities, especially in sustainability. Nowadays, I’m getting requirements like, “Can you come up with a solution that keeps sustainability scores in mind?” That’s just one example where I see generative design playing an important role going forward.
Greg Arnot: That’s a fascinating spin on generative design. As AI advances, do you think there will ever be something like a conversational interface for product design? For example, if I were to say something like, “Change the display properties of all the fasteners in my design to green,” or if I were working on an injection-molded part, I could say, “Change all of the boss diameters to 30 mil for me.”
Scott Felber: 100%. We’re almost there today with doing similar things. It’s just a matter of time before you can say, “Change all the bosses to 30 mil,” and just talk to it. We already have voice commands within NX. You can talk to NX in a conversational manner today to create your designs. You can take standard sets of commands and give it more natural language, or you can do it command by command. So, I think the future is already here today on that one, Greg.
Shirish More: Absolutely. The idea of having a conversation with software or an engineering solution that supports you in coming up with a product design is just going to be there. It’s something that we have actively worked on for a couple of years, as Scott referred to. But going forward, this idea of integrating large language models and AI-driven natural language processing is just going to make it feasible for engineers to interact more in engineering terms rather than coming up with a training course that says, “Well, you need to click on this command,” or “You need to understand this process.” I think going forward, this whole idea of integrating MCAD solutions with large language models is going to make it feasible for engineers and designers to interact with their software using conversational commands. Imagine, going forward, being able to make design adjustments, as Scott said, or run simulations or generate reports on my design data that I have loaded in my session, for example, just by speaking or typing a command. It’s going to be a game-changer. How many times have I gotten a requirement that says, “Can you identify the 10 heaviest components in this assembly?” For me to perform that task now, I need to know, “Okay, first, load the assembly, generate a report, export that information to Excel, then apply the filter,” so many commands. But now imagine I can just say, “Alright, highlight the 10 heaviest components,” and we can execute that just based on a conversation. It’s going to be a game-changer, Greg. So, to answer your question, absolutely. The idea of having a bidirectional conversation either in form of text or a voice assistant through our MCAD softwares or NX itself, or going forward with VR and AR, it’s just going to be a game changer.
Greg Arnot: Well, that’s fantastic to hear. It’s amazing to think of the possibilities. Scott and Shirish, I’d like to talk more about NX specifically. What innovations are being seen with AI in NX? What features existed before and how are they evolving over time?
Shirish More: When you look at the traditional way in which users used MCAD and NX, users tend to follow set standards or practices. Now, with AI, what has really happened is it has given us an opportunity to look at different workstreams.
We have four key workstreams that we support today. So, the way AI capabilities work is that there are certain features we make available as part of the standard or premium module, and then there are certain capabilities that we make available for our users through value-based licensing. Most of these add-ons fall under those four key workstreams: personalization, prediction, smart human-computer interaction, and AI services– if a customer has a need to train a model using their own data, then we do have options for customers to use those as well. Adaptive UI or personalization, for example, is the ability for us to understand how users are using our software. We have modules like command prediction. The reason it falls under personalization is that this particular AI capability tracks how users are using NX and in what context. It’s an example of personalization or an adaptive UI, and it’s an add-on that we make available through our value-based licensing.
Greg Arnot: And do you see personalization being an integral part of the NX workflow going forward?
Shirish More: Personalization is going to be a huge workstream, where, compared to where we were before and where we are now, we can guide the designer. Not only can we assist in selecting particular entities, but if you are a sheet metal designer versus an injection molding designer, or if you are designing an injection-molded part, for example, we can now start suggesting things and guide the designer in making sure they are working with their design using the right best practices. Prediction and smart human-computer interaction, which we never had before, but now, with the ability to have a voice assistant coupled with large language models and a conversational interface is going to be a game-changer. That’s where we are heading. You’ve already seen that we have introduced many capabilities that predict things, from selection to grouping components of similar shapes, which help users navigate through large assemblies. We have capabilities to start predicting materials based on how the user has used NX or things they might have designed in the past. So, there are a number of opportunities.
Scott Felber: The biggest one I want to mention, along with personalization and standardization moving forward, and the ability to take a customer’s intellectual property and their design workflows and train NX to align with their workflows. So, when they hire new or junior engineers, they have some of that built-in knowledge right away without having to learn it all themselves. The company can move forward along their best practices, and when they find something new, they can retrain the model and say, “Hey, we need to start working in this method,” and retrain the system, getting that information out quickly—especially if you have a large staff of engineers and designers.
Greg Arnot: Moving beyond the pure software product, what AI services are we offering?
Shirish More: There are two ways in which we offer our AI services. The first one is generic AI models, which are offered with NX. When a customer is using NX, we deploy the AI models on-prem, which are generic AI models. These are models that are trained using repetitive tasks. For example, based on our training documentation, we know these are the things that users, day in and day out, perform. When you apply a blend, you’re typically trying to get rid of sharp corners or sharp edges. This is just an example of a generic AI model, and customers like it because it makes the process much more efficient. Now, there are different types of AI services. This one is more of an out-of-the-box service with NX—it’s a generic AI service. But then, there are customer-specific AI services, where a particular customer, for example, from the automotive or aerospace, has their own best practices and data. One of the services we offer as part of our AI services is the extraction of information using associated metadata from a customer’s location. When I say “location,” I mean that the data might come from different enterprise software, and we use that information to train a customer-specific AI model, which they can then deploy on the user’s machine. So, in summary, we have generic AI services offering which we train with out-of-the-box with NX data and documentation and it’s deployed with NX. Then, we also have customer-specific AI models offered as AI services. These can be implemented by the customer, with a service that runs on their premises, extracts information, learns from it, and then they can deploy that on-prem as well. Those are some of the AI services, Greg, that we have in place going forward, and we are going to incorporate those for additional use cases as well.
Greg Arnot: How accurate are the results that our AI features are providing?
Scott Felber: The whole thing comes down to trust. The results are accurate based on the data—it’s a case of “whatever you put in is what you get out.” It does make good decisions based on the data it has, but if data is missing in the input process, it can’t figure that out. However, I don’t doubt the accuracy of the results. I’ve talked to a lot of customers at the last two Realize Live events this year, and I will say they are very excited about what we do today in production. They’re also excited when they hear about some of the futures we did talk about a little as to where we are going, and they can see where we’re going without it being said. Customers are excited to embrace it because of the productivity gains. That’s the feedback I got from customers at Realize Live this year.
Shirish More: With AI, keep in mind that, as I explained, we are trying to make machines make decisions the same way humans do, and the input parameter, in this case, is the context in which the user is invoking a particular AI capability. So, we can’t say that it’s always going to get all the selections, for example, 100% right, as if a human would have selected them manually. But it gets there. We do provide users the ability to improve the sensitivity of predictions, for example. So, yes, from an accuracy perspective, it’s very close to becoming 100%, but it will never be 100% because of the nature of the way AI leverages some of the input parameters and, in this case, the context. If the context keeps changing—if I do it on a simple block, oh yeah, you’re going to get it 100% right. But if you try to apply a similar logic to a more complicated part, it might get it right, but not always. So, it’s always going to be around 90%, depending on the use case and the context in which you are invoking a particular AI capability. From an accuracy perspective, I’ll say it’s never 100%, but we’re close to 100%.
Scott Felber: If you want to see some of that selection prediction and similar selection component stuff, there is a blog that’s going to be out there that we’re working on—a big one that covers a lot of that selection.
Greg Arnot: Thanks for that recommendation, Scott. For our listeners, we’ll put the link to that blog for you in the show notes. You’ve mentioned quite a few of the AI capabilities in NX already. I want to bring up another AI-enabled feature– NX Molded Part Designer – that was released relatively recently within the NX product suite. What details can you share about the module?
Scott Felber: What we started to do is capture best practices around molded part design. It’s not just about how to create the best snaps, the best features, or things like that, but there are also built-in validation steps that we’ve incorporated. For instance, we can predict draft angles based on the material you’re using to make that part. There are other things we can do to ensure it meets all your design standards with quick validation checks. If it doesn’t meet those validation checks, it will give you a hint to tell you what you can do to correct that—whether that’s adding a different command or going back to the original feature. So, when you send those parts out for a quote the first time, it’s not going to come back with a bunch of change requests and iterations from your suppliers. To create parts that are manufacturable from day one is the key with Molded Part Designer.
Shirish More: Molded Part Designer is a classic example of collective intelligence. I get this question a lot: “Okay, what are you doing?” As I hinted earlier, there are a number of examples where we track the way users are using our software. In this case, we know that if the user is inside the Molded Part Designer, they are either working on an injection-molded part or a pressure die-casting part, for example. Based on the context and how they might have designed similar parts in the past, we learn from their actions. We’ve trained an ML model that goes based on the best practices as well. So, depending on the command that he is in—like Scott hinted on material prediction or draft angle prediction—we use the current design’s context or input parameters, feed that to a machine learning algorithm, and it comes back with suggestions. It means both for his initial design creation and once he starts validating his design. We look at the failures, and there is continuous learning and feedback where the designer is refining the design, and we learn from their actions. We have already trained the ML model using best practices, we start guiding the designer in designing the molded part right the first time. What we are trying to do at a high level is reduce the number of design iterations by getting the design right the first time. Once the design is ready, the simulation guy might come back saying, “These are some of the things that you might have to tweak,” or the manufacturing engineer might come, and that just adds to the overall design cycle. With Molded Part Designer, we are incorporating AI techniques to speed up the overall design process and get it right the first time, rather than relying on iterative design, which can be time-consuming.
Greg Arnot: Very impressive. I love the angle of an algorithm that learns from empirical data and just keeps on improving. And ultimately, reduces iterations.
Scott, Shirish, let’s look toward the future a bit. How do you see AI shaping the future of design?
Scott Felber: Going into the future of all the things we talked about, it’s going to be better designs done in a shorter amount of time, with higher quality, and being able to be manufactured from day one. The elimination of human error is something we hope to achieve as we’re doing the design. To me, that’s the thing—it’s going to increase speed to market, which will lead to better innovative solutions because you’ll have more time to think about the things you want to add to the parts. That’s a big one for me.
Shirish More: Yeah, I see AI making more data-driven decisions with greater confidence. It’s always going to be about trust and the ability for us to parse through large amounts of data. If there is customer IP, it stays with the customer. But my point here is that the way AI is going to shape the future of design is by making data-driven decisions with greater confidence. AI will also enhance collaboration by integrating real-time feedback, performing predictive analytics, and bringing that information into the design process, allowing for continuous optimization of design processes. As AI continues to evolve, we can expect it to take on more complex roles, such as generative AI, which will innovate designs going forward. It’s going to suggest things that we might not have even thought about, which is a good thing, and it’s going to improve overall design processes and ultimately push the boundaries of what’s possible in the overall product development processes.
Greg Arnot: Do you think NX X and SaaS– or software as a service– will enable further AI capabilities and development?
Shirish More: It’s not just NX X, but anytime we talk about cloud components, the beauty is that with cloud components—like AI services—the reason I say it’s important is because, first, we do know how the users are using NX X, for example. With that information, we can tailor the experience, personalize it, and start predicting things in a much better way compared to traditional NX, which is on-prem, where we really have no insight into how users are using our product or in what context. But with SaaS, it becomes much easier by providing a scalable, flexible, and connected environment. If you recall, I introduced two new terms: collective intelligence and community learning. These are possible only with SaaS offerings or cloud components because now we have better insight into how a particular customer or how designers as a whole are using our products. That’s the beauty of SaaS components or cloud components is that once we introduce cloud components, it will help AI by allowing us to learn from how users are using it. Based on this collective or community intelligence, we can start making overall processes more efficient.
Greg Arnot: So, before we go, what can you tell us about the future of AI in NX?
Shirish More: Well, I can’t reveal the entire roadmap or all the details, but I can share that the future of AI in NX is incredibly promising. With the partnerships we’ve established with some of the big AI players or vendors, we are focused on integrating AI even deeper into the design processes, making NX much more intelligent and responsive to user needs. Going forward, with the things we are incorporating, users can trust an AI solution that’s embedded inside of NX. This includes expanding our use of generative AI to offer even more sophisticated design suggestions, as well as enhancing real-time simulation capabilities. We’ll continue to improve user interaction through natural language processing, conversational interfaces, continuous monitoring, and continuous learning. Our goal is to make NX X not just a tool for design but a tool that incorporates collective intelligence—empowering our designers to push the limits of innovation and efficiency. We’re also going to work on other advanced technologies, so keep an eye out for these exciting developments as we continue to evolve NX with AI at its core and foundation.
Scott Felber: As we do more and more of it, it’s just going to become more naturally baked in, and you’re not even going to notice it’s doing it. It’s going to be just like, “It just does this, and it just does that.” All of us who have used CAD for a long time get used to certain workflows and don’t even realize why it’s doing what it’s doing. That’s really what the future holds for AI in the mechanical design space—making it part of your everyday tasks, where you don’t even question, “Is this AI?” or “Is that not AI?” No, it’s just NX.
Greg Arnot: Scott, Shirish, I’d like to thank you for joining us. It’s been a pleasure speaking with you both and hearing your thoughts on the role artificial intelligence plays in computer aided design. Thank you so much again to Shirish and Scott, we hope to speak with you again soon. Thanks also to our listeners for tuning in to today’s episode. Join us next time for more discussions about the latest in design innovation and software applications. If you haven’t already, please consider subscribing to our podcast on your favorite platform. And if you’re enjoying the content, please leave us a review. I’m your host, Greg Arnot, and this has been Next Generation Design.
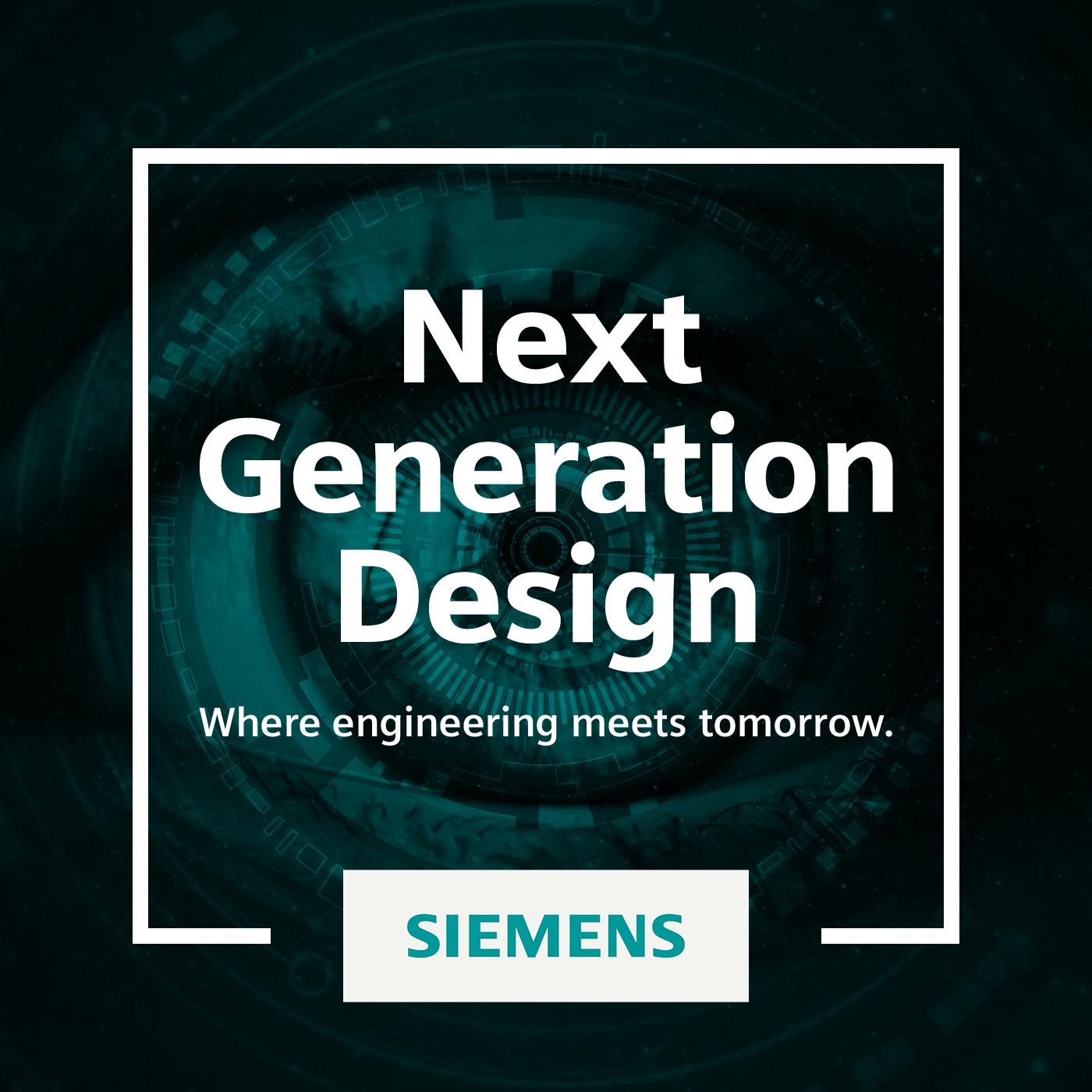
Next Generation Design Podcast
As product engineering tools continue to morph and expand at speeds human expertise may not be able to endure, Revolutionary design technologies that span beyond industry borders, will prove their necessity for companies looking to take over their markets in the future. What will the future of design technologies and machinery look like? What will your digitalization story be? Where engineering meets tomorrow.