The Mechanical Side of Generative Design Part Two – Transcript – Talking Aerospace Today
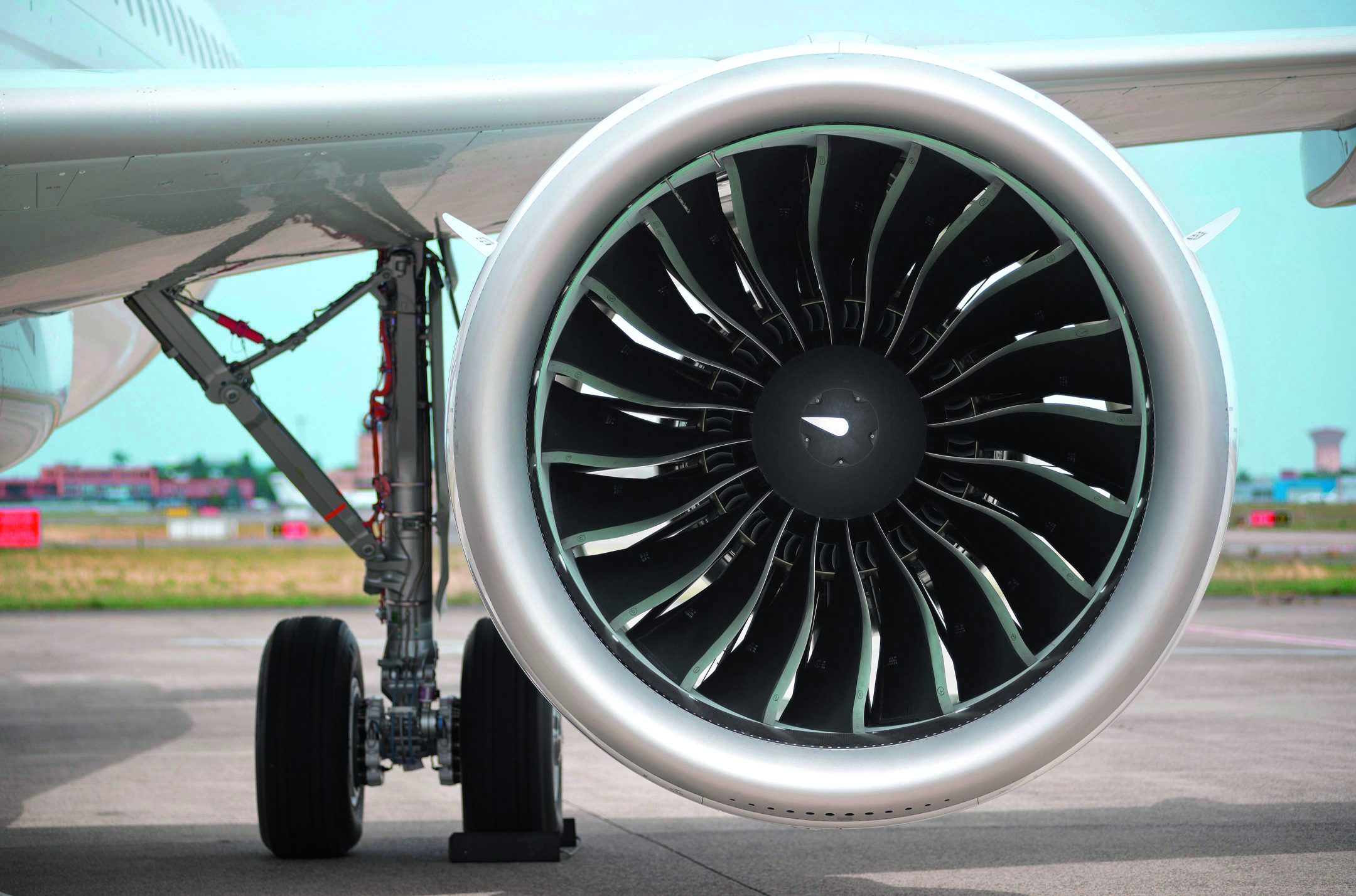
In this episode, Todd Tuthill and John O’Connor continue their conversation from the previous episode on generative design for mechanical engineering, discussing it’s present capabilities and where it could go in the future.
Patty Russo: Greetings and welcome to another episode of Talking Aerospace Today. I’m Patty Russo. Today’s discussion picks up on the conversation about generative design for the mechanical domain in aerospace and defense. Like our last discussion, today’s conversation continues with Todd Tuthill, VP for Aerospace and Defense here at Siemens Digital Industries Software, John O’Connor, Director of Aerospace Product and Management at Siemens, and Maddie Goetze, Content Writer for our A&D marketing team. With that, Maddie, the floor is yours.
Maddie Goetze: So John, could you give us a little bit more detail with regard to what mechanical generative design can offer and where that will go in the future?
John O’Connor: Sure. So the key thing to be able to do a generative design is to be able to have these driving constraints on the design. So having really tight integrations between simulation tools and design tools like mechanical CAD modeling tools makes it so that you get the ability to be able to drive that desire because you really can’t have a fully generative design unless you’re combining some type of simulation engine into the design process. And then you want to be able to have that simulation engine driven to certain constraints based upon whatever the kind of governing physics are for the problem that you’re trying to solve. So if you create that environment and you have the environment, now you can begin to exploit a much wider range of design options. And this will eventually create over a series of learning cycles.
John O’Connor: It will create sort of the best design you can achieve within the constraints you applied. And as I mentioned before, those constraints are not just, you know, the physics of the problem, but they’re also the constraints regarding the manufacturability of the design, material choices, cost. Other types of factors come into play when you’re creating the model that you will use to drive that design via generative process.
Todd Tuthill: So John, if I could follow up there with regard to learning wrapped around the future of mechanical design and I think about a problem that we see with artificial intelligence in general and large language models and the aerospace industry and customers I talked to say, “Well, Todd, it’s our proprietary data. We’re not sure we want to give a company like Siemens or OpenAI or Microsoft Access to our proprietary data.” So John, do you see a time in the future where we’ll be able to take a mechanical design tool and a customer will be able to let it learn with their own proprietary data in their own particular way that keeps it separate and apart from their competitors and even from us? Do you see a time, and what might that look like you think with proprietary data links and customer data?
John O’Connor: Yeah, I mean that time is now actually, where we have customers that are employing a process by which they are able to use the previously developed sort of, you call, like, non-generative developed, you know, developed via human interaction to be able to feed a generative model that will then drive to a better design. And there are a variety of different areas where you see this, but the key thing really is being able to give people that engine that allows them to be able to do this while also harvesting that data that exists. Because you really can’t have any type of AI-driven generative process without that fundamental underlying data that you can then use to drive it.
John O’Connor: So companies have these immense resources, or these immense sets of data of previously developed design definitions, and so if they are able to convert that into a format that can be utilized by an AI generative driven type of engine, you can get really spectacular improvements in design quality and the functionality of the part by, really by a process that would allow you to arrive at a result that you couldn’t arrive at from a purely human sort of purely intuitive process. So the things that you know, these systems are great at are finding those hidden non-intuitive solutions that will get you to sort of a next generation level type of performance for a design.
Todd Tuthill: Yes, so we talked about time at the beginning, and I want you just to dream a little bit and think about time, and once we have these generative solutions that can learn from customer data. How much time do you think we can reduce one of these big design iterations for a large chunk of an aircraft to what can we take it from what it is today to what it is in the future? You know, just rough order magnitude, do you think?
John O’Connor: It’s a little bit of a moving target really because you’re suggesting like, well, how long would it have taken you to create something that it would have been really, really hard for someone to create on their own anyway. But assuming that someone would have had sufficient cycles to create that, you could be looking at an 80% reduction in the amount of time that it would take to do that. And it really ties directly into what does it take to develop a process by which you’re creating these next generation products? So these faster iterations allow you to be just that much more efficient than your competition. And so over a few of these cycles, you can be a full product generation, a full product cycle ahead of your competition and then effectively dominating the market from there on out.
Maddie Goetze: That’s really interesting and it’s really exciting to think about the future of generative design. So adding on that, John, what are some examples of mechanical generative design tools or capabilities that customers can use today?
John O’Connor: Yeah. So there are a wide variety of these. Obviously at Siemens, we have the industry-leading capability in this area, and that stems from a couple of things that are sort of basic CAD modeling capabilities and also things that have to do with integration with simulation engines. And I’ll talk about the simulation engines in just a second, but to talk about what it means to be able to create a practical utilizable general design. I talked about this evolution from it’s kind of inspirational conceptual topology that would be created when this area sort of began, and now we have the ability to create these really, truly manufacturable geometries that can produce an end result.
John O’Connor: And you can tune that end result depending upon the type of manufacturing process that you expect to have. But that geometry that you’re creating is probably always going to be in the context of a part that’s designed using traditional CAD modeling methods. So you need to be able to take the generative output, which is typically a faceted mesh definition of a shape. Very organic looking. Very much tuned to the pure physics of the problem. But then combine that with traditional parametric or Boolean modeling capabilities that allow you to be able to add in additional detail that would have been maybe just purely machined off the part. So, you know, when these additive types of products, for example the products very rarely are they purely an additive product.
John O’Connor: Often in their use, they’re manufactured through a combination of processes, both additive manufacturing and subtractive manufacturing, to be able to create the final part. And so where you have more prismatic geometries or like traditional CAD type of shapes, you need to be able to design those well and design those on the same platform that you’re using to create the faceted mesh design that’s been created by the generative process. So that’s one thing of being able to do that. And if you can’t do that and you don’t have a really tight integration there It’s not integration, just have a platform that understands both a traditional prismatic modeling approach as well as an additive modeling approach. Or I should say as well as a generative modeling approach.
John O’Connor: Then you’re going to have a lot of trouble in your design that adds extra time, extra cost, and potential for errors. All the kinds of things that slow down programs and make it so that they have a harder time meeting their ultimate schedule and getting the parts out the door that they need.
John O’Connor: So that’s the first part. The second part is making sure that your simulation capability that understands the physics of the problem employs a multiphysics solution, could be structural, thermal, you know, magnetic that could have all kinds of capabilities that you need to drive the design. Having that on the same platform that then can employ the types of optimization algorithms, genetic algorithms, other types of engineering, simulation, types of algorithms that would allow you to drive that design to the constraints that have been set up in that multiphysics model.
John O’Connor: So having a CAD system that is able to easily speak the language of generative and also of a traditional CAD design methodology, and then also having a system that has on that same platform the same types of multiphysics capability and CAD modeling capability that will drive that. So doing that all in one place, it saves a lot of time, avoids errors and makes for a much more sophisticated and much more efficient process.
Maddie Goetze: Todd, is there anything that you would like to add to that?
Todd Tuthill: Well, I guess as we kind of finish out the episode, the thing that I want to refer back to, I want to think back to two things John said because they were key. First off, we talked about time and John estimated we could save 80% of design time and I want you to think about the implications of that. We’ve been talking about digital transformation maturity for a while and to me, the key villain, the key problem that digital transformation maturity solves is workforce. What if you could multiply the impact of your workforce by five?
Todd Tuthill: That’s incredible, right? That’s incredible, and certainly I’m not saying that we’re going to multiply your entire workforce by five. We’re talking about generative design, but it’s a significant impact to your workforce and the ability to get so much more done with the existing staff you have, and we kind of talked about that in the opening as how does that affect current engineers? It lets them be creative. It lets them spend their time doing the things that we studied in college. The fun, exciting stuff, and it lets programs do so much more with the existing staff that they have.
Todd Tuthill: So that 80%, is so exciting as well as something John talked about, the connection to simulation, and this massive reduction in or massive improvement in multiplying the impact of staff and this connection into simulation is where we’re going to go next in this podcast. We’re going to talk about closed loop optimization and that’s where we take, you know, the connection to electronics, to mechanical, to software, put all the domains together and connect in a simulation in a closed loop fashion to really supercharge where we can go with this whole idea of digital transformation maturity.
Todd Tuthill: And as interesting and exciting as these previous podcasts have been, they’ve been, it’s been great having Tony on. It’s been great having John on talking about the single domains. The podcast we’re going to follow with will be all about optimization and really the multidisciplinary combination of that. And that’s what’s so exciting to me and just continues to show incredible benefits from really a full digital transformation of a company’s process and especially the digital transformation as informed and helped by artificial intelligence and machine learning. So it’s been fun. Maddie, thank you for being on the podcast. John, it’s been great to have you on today. Thank you for all your insights as well.
Maddie Goetze: Great. Goetze, Maddie (DI SW ST&MK ISME ISG GTM IM1)
Well, thank you, Todd and John for your insights in this discussion and thank you to our listeners for joining us. We hope this conversation helped you learn more about generative design and how it relates to mechanical design for aerospace. I’m Maddie Goetze and we look forward to having you join us for our next episode of Talking Aerospace Today.
Siemens Digital Industries Software helps organizations of all sizes digitally transform using software, hardware and services from the Siemens Xcelerator business platform. Siemens’ software and the comprehensive digital twin enable companies to optimize their design, engineering and manufacturing processes to turn today’s ideas into the sustainable products of the future. From chips to entire systems, from product to process, across all industries. Siemens Digital Industries Software – Accelerating transformation.