AI in the Digital Transformation Revolution: Industry perspective from Dale Tutt Part 2 – Transcript
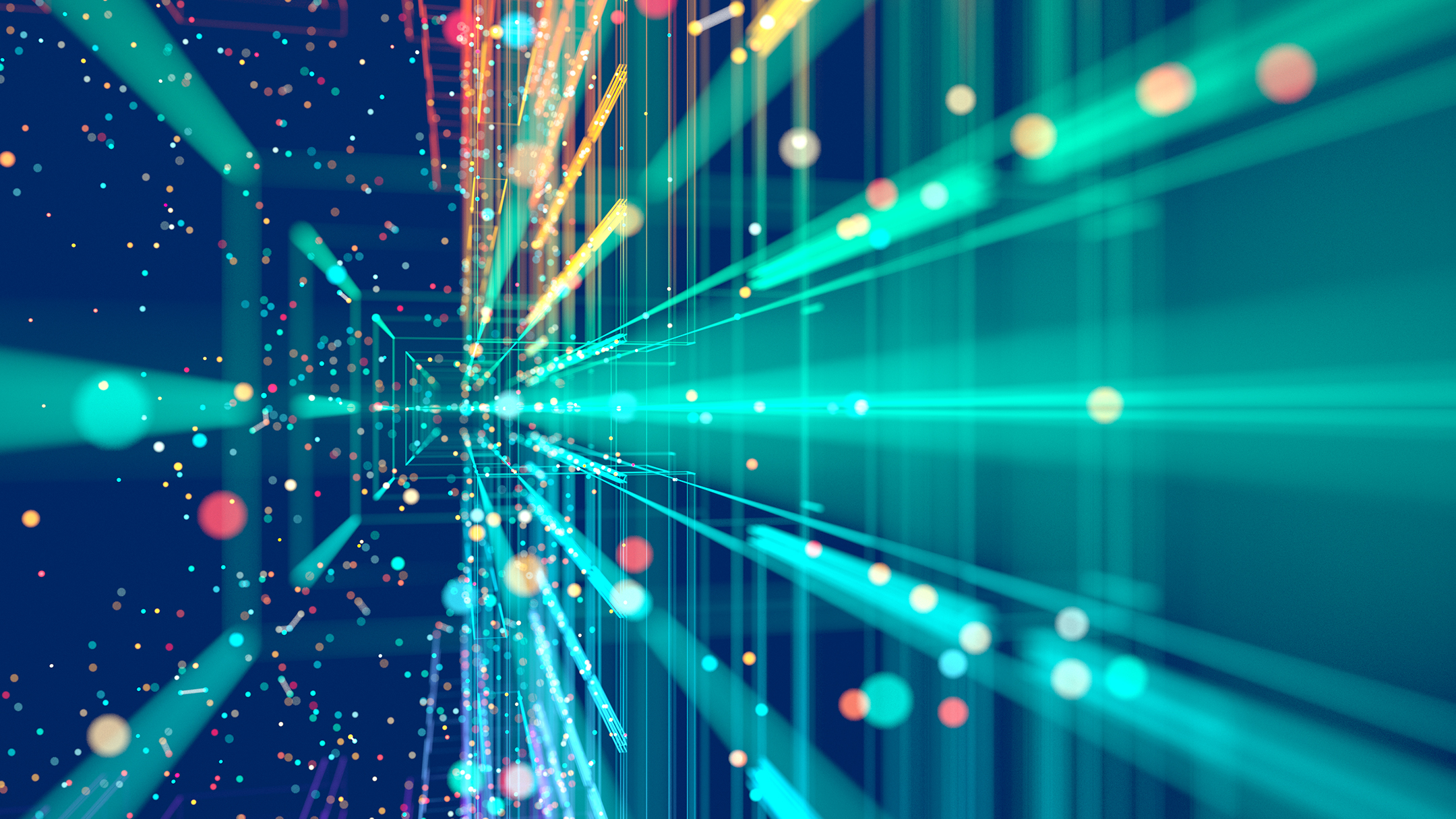
In a recent podcast, Justin Hodges continued a discussion with Dale Tutt, Vice President of Industry Strategy, for Siemens Digital Industries Software, on the many uses of AI in the digital transformation journey and where that will lead in the future. Check out a transcript below, or click here to listen to the full episode.
Justin Hodges: The following is part 2 of a 2-part conversation between myself and Dale Tutt an experienced aerospace and defense professional for over 30 years. It’s a pleasure to hear from Dale’s first-hand experience as we discuss the symbiotic relationship of digital transformation and artificial intelligence. And now, dear friends, is Dale Tutt.
Dale Tutt: We’ve talked a lot about engineering aspects of AI, and I think another aspect that a lot of people tend to maybe not think about sometimes is the manufacturing aspects and how does this change and transform your manufacturing capabilities. And I think about some of these process intensive industries that I’ve worked in or that I’ve seen some of our customers that are working in, and all of them are now looking at how do they reduce energy emissions and carbon output. And so certainly as you can now start to use your digital twin to design your production lines, but then taking the real data off of those production lines and figuring out how you can further optimize that and using AI and machine learning to make that more efficient, more effective.
Some of the other things that we hear a lot of our customers talking about is understanding the battery aging process. So there’s a lot of demand for batteries because of all the demand for electric cars. And a lot of our customers tell us, “Hey, one of the things that we are challenged with is what’s called the battery aging process.” And this is usually a process that might take three or four weeks, or longer, as you’re developing a new batch of material for your batteries. And so it’s very process intensive and you can use AI to start to optimize that process and bring down that production time, if you can bring that aging process down, you may not be able to bring it down to days, but maybe you know the results of your processing in three or four day, and so you don’t have to wait three or four weeks to find out that you had a bad batch.
And so I think this is one of those areas where AI can really help customers and the data analytics to really help customers produce batteries faster and get to good batches and good quality batches faster, especially to help address the demand for new batteries. Another area that I have some experience with is when you’re going through manufacturing composites, carbon fiber composites materials are used for airplanes, for windmill blades, even in automotive manufacturers are using these materials now, they’re very process intensive and you have to control a lot of parameters around the humidity, what’s the temperature, what’s the temperature of the oven, what’s the temperature of the part. And a lot of times there’s, I would just say, a bit of black art to this.
You have to be very creative that you have these parts, and especially in an aircraft, it might be very thick in one part and it might be very thin in another part, and yet the whole part has to go into the oven at the same time and it all heats up at different rates. And so sometimes there’s a lot of learning that you go through as you build the first couple of parts and you end up maybe have scrapping. So I think now as you start to look at the properties and the process of how those parts are cured, that if you can start to use AI to help optimize those processes, there’s the opportunity for companies to really increase their yield and the quality of the parts that are coming out, and maybe even the strength of the parts. So that’s just an example of a very process intensive industry that you have to think about, you have opportunities for how AI can help solve those problems.
Justin Hodges: A ton. And they’re really interrelated to the longstanding objectives by the group even before you talk about AI. To demystify a bit and nerd out some, you’re describing this complicated problem with all these parameters. In a straightforward way, you’re suffering from the curse of dimensionality, there’s too many things going on, I don’t have enough data to model the relationships. The relationships of things like humidity and then maybe material strength that comes out on the other end if you’re processing materials, well, it’s a very non-linear problem as well as a very high dimensional problem. And of course, that’s the tool you pull out of your tool box is machine learning. We know it’s very good at capturing these non-linear relationships, and that’s a message that I think is overlooked sometimes when we talk about AI in our industry is, look, we have the same mission, it’s just a different tool that can handle these sorts of problems with cursive dimensionality and a lot of non-linearity.
And another layer here, I love the manufacturing example, I think that’s a good one as a little case study on how much this industry and all this space is transforming this digitalization effort. You can really be granular about it and say, “Well, we should apply these machine learning class of methods to help people when they produce materials so they can understand things in the full lifecycle of whatever they’re creating.” So in a concrete way of saying it, let’s say I’m producing steel and I want to know things like, well, what if I do this type of a kneeling process to form the steel versus another? Well, it would be great to know the impact not just in the moment when you’re manufacturing things, but with this data-driven techniques 20 years later when the consumer purchases the materials and builds whatever they’re building, how does the life of the product behave based on these decisions you make very early on in the process.
And so I think it’s no surprise we’re seeing these data-driven methods, like you said, data analytics, these things that cover from design to manufacturing to operation, and wanting these tools to have coverage over all of these aspects. Obviously in that, that’s really looking like big data, right?
Dale Tutt: Yep.
Justin Hodges: But in the end, it’s an age-old struggle. We have the same sort of fight and sort of transition of new technologies into society. AI is probably a current flavor that’s easy to relate to, but I’m guessing you have some other stuff in your experience where some emerging technology came and there was this process to have society adapt to it, trust it, use it, anything come to mind?
Dale Tutt: Yeah, I think it’s some of these technologies that I’ve looked at over the years, I’m trying to think how many years ago that we started looking at augmented reality out in the shop floor, and at the time we looked at it, but it was hard to implement. This technology was challenging at the time, and our processes didn’t supported a lot of doing it a little different way. And so now you’re starting to see a change, what wasn’t possible seven, eight years ago now is starting to become more mainstream. And so we’ve seen this technology pop up once a while and it shows a lot of promise, but then I think the reality hits everyone like, okay, well, it’s a little harder to implement than we thought.
And so that’s where I loved the example that you used at the beginning about every decade something pops up, but I think this is where we’re starting to see it’s going to really get traction and take hold. I think the best applications of AI is people may not even realize that it’s in there, it’s just it’s changing how they’re working and they’re becoming more efficient, and it’s making the tools more usable, and they’re just like, “Wow, I just love this.” I think that’s when you’re going to see AI being really, really successful.
Justin Hodges: I’d say there’s a generational paradigm shift on what software should look like, because in my generation versus my daughter’s generation, there’s just going to be a different expectation on how intuitive, easy to use, plug and play the software is. So I think we’re seeing a lot of that with these AI apps that are percolating everywhere where you can just do crazy things and then that sort of thing, but the concerns for trustworthiness and things like that for AI are valid. The points that I usually bring up are, well, if they become more explainable and more trustworthy, our researchers can continue to shed some insight on why the machine learning models may give a certain suggestion versus another, do things to reduce bias and make sure things are fair, and there’s no harmful stereotypes perpetuated in the design or in the model predictions.
But I think it’s been an interesting case study as well in 2023 for ChatGPT, I think it’s amazing how much everyone has pretty much educated themselves on how to use it, what’s a good response, what’s a bad response, and I think that user education is going to be another key role here in terms of safely leveraging AI in our applications. Dale, can you think of another example in your time of a technology like AI, but not AI from past, where it was emerging, it was this type of trending topic that got all the attention? Obviously there were skeptics, but that process of it going from just a highly popular topic that was emerging to something trusted and in the tool belt of a mechanical engineer, aerospace engineer.
Dale Tutt: Absolutely. I go back to simulation and how it’s being used now to really define products and being used now today to really help drive decision making as companies are developing new airplane products or new cars, it doesn’t matter what we’re talking about. But in the early days, 20 some years ago, a little over 20 years ago when we were developing a lot of simulations, we were going through a process where we used desktop simulations, aerodynamic analysis coupled with flight control simulations, and we were developing systems doing that. And then we’d take them to an iron bird and we’d test them on the iron bird, and then we’d eventually, when we get to the airplane, we’d start flying.
The previous process had been, well, you’d do some modeling and then you’d start flying the airplane and you’d figure out and you’d go through fly-fix-fly approach. So we were trying to go through a process of simulation into the hardware and the loop simulation, then into the flight test. And as we were doing all of this work, we were working on a new airplane program and we found an issue with the airplane that was not going to meet the requirements for the roll control system. And so the pilot forces are going to be too high in certain flight conditions. So I just remember at the time our bosses said, “Well, this is all new. Do you trust it enough for me to change the direction of the program right now?” And we were like, oh, everyone’s ring in our hands and we didn’t know, but we at least as a company went on a parallel path. We did invest in creating a parallel path.
And it turned out when we got into flight test, the simulation, the hardware-in-the-loop simulation was exactly right, and so we had a plan in place, so we’d at least been able to use our simulation to do that risk mitigation. Now, fast-forward three or four more years later, we’re working on another new airplane program, and when our team found an issue on the airplane, everybody said, “Okay, we’re going to go fix it.” Because we had gone through the process of validating the simulation and demonstrating that it was giving us the results that we expected, that we weren’t just putting a lot of fudge factors in the simulation to make it work, that we could actually rely on that to make predictions about how the airplane was going to fly. So I think that as anytime you have a new technology coming on board like this, people are going to question, well, am I getting the right answer?
There are some examples when people have gone out to ChatGPT and they’ve asked a question and they get something that is not the answer they expected. And it may have been that they asked the question wrong, it doesn’t matter, they didn’t get the answer that they expected, and so then that calls it into question. So that’s where I think using some examples from the past, we’ve gone through this before with new technology on how we have to learn to validate it, learn to trust it so that you’re making critical decisions with it. And I think that’s going to be the path that we’re on to help address some of these questions of trustworthiness.
Justin Hodges: That was a great response, but I’m not going to lie, when you said fly-fix-fly, I felt jarred and thought people used to do things in that way, wow. But I was going to ask what’s some advice you would give to AI enthusiasts and practitioners to learn at a high level abstraction of, okay, when you went through that process with simulation with new technology, what was a key thing to do? But I think you pretty much answered it right in the end there, validate the methods along the way.
And that’s something I definitely preach in my time working in different projects and teams for AI and simulation work, is that we should form the problem in a way that’s familiar, i.e., based off of physics principles, conservation laws, simulation practice and methodologies, and then use it with bumper rails to give directional help but not use it in a blind black box way. And I think that I can say a lot of people in our industry are trying to make the box semi-transparent rather than black box. So I would definitely agree that that’s some expertise there to glean from simulation. Won’t ask how long ago that was, because I think that’s mean to the podcast guests to do that with AI. So great answer. Thanks.
Dale Tutt: Well, thank you.
Justin Hodges: Well, again, thanks for the chat. It was great to have you on the podcast. We can close the episode here, but I’ll give you the chance to say some parting words or an outro if you like, Dale.
Dale Tutt: Well, thanks, Justin. As we started the show, we talked about the power of digital transformation, how it’s helping companies with their business transformation, whether they’re trying to go faster or just they’re trying to address some of their workforce concerns or new sustainability requirements. And so I am excited about the power of AI and as it starts to embed in a lot of the solutions that we can provide how it’s going to really accelerate the digital transformation for a lot of our customers and really help them achieve their business goals, their business objectives so much faster than they have in the past. So I think we have exciting times ahead, it’s an exciting new technology, and I think it’s going to really change how people work, which I think at the end of the day is one of the things that we really want to help people with, so let’s help them out with that. So thank you for having me.
Justin Hodges: Well said. Totally agree. Thanks again, Dale. And with that, we’ll close the episode. Thanks for listening.
Dale Tutt: Thanks a lot. Bye-bye.
Siemens Digital Industries Software helps organizations of all sizes digitally transform using software, hardware and services from the Siemens Xcelerator business platform. Siemens’ software and the comprehensive digital twin enable companies to optimize their design, engineering and manufacturing processes to turn today’s ideas into the sustainable products of the future. From chips to entire systems, from product to process, across all industries. Siemens Digital Industries Software – Accelerating transformation.