AI in the Digital Transformation Revolution: Industry perspective from Dale Tutt Part 1 – Transcript
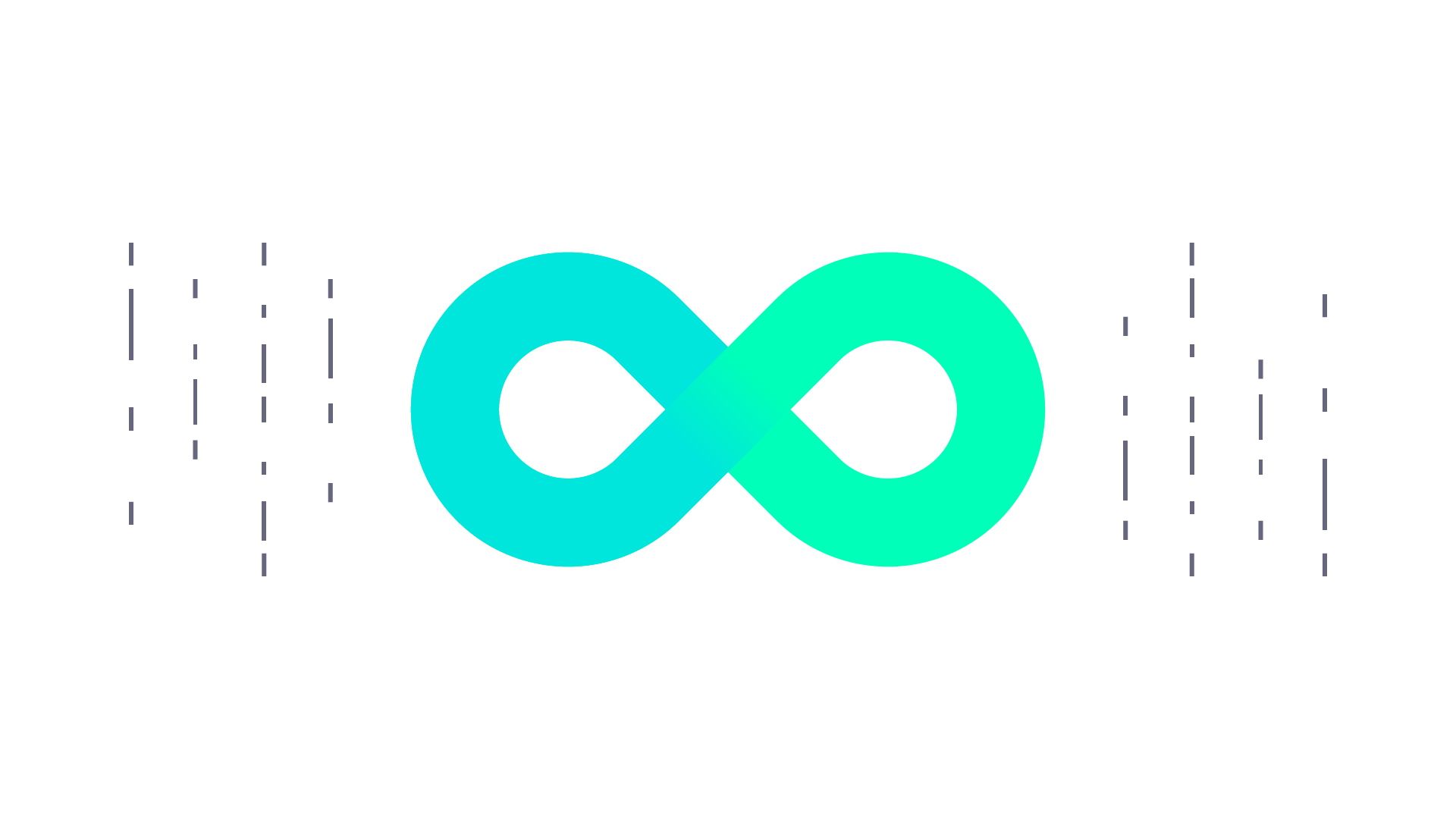
In a recent podcast, Justin Hodges talked with Dale Tutt, Vice President of Industry Strategy, for Siemens Digital Industries Software, about the impact of AI on the ongoing digital transformation taking place in industry. Check out part 1 here, or find the full transcript below.
Justin Hodges: The following is part 1 of a 2-part conversation between myself and Dale Tutt an experienced aerospace and defense professional for over 30 years. It’s a pleasure to hear from Dale’s first-hand experience as we discuss the symbiotic relationship of digital transformation and artificial intelligence. And now, dear friends, is Dale Tutt.
Dale Tutt: Hello, Justin. I’m Dale Tutt. I’m the vice president of industry strategy here at Siemens Digital Industry Software. In my role, my team’s role, we help develop strategies and solutions for the industries that we serve. And so I joined Siemens after 30 years in the aerospace and defense industry where I was designing and building new aircraft and new spaceships, working at companies like Cessna Aircraft Company and The Spaceship Company, which is part of Virgin Galactic, so I’ve had a lot of fun, but now I’ve been able to bring my experience working in industry to Siemens as we develop new solutions for our customers. So one of the things that we focus a lot at our team is what’s digital transformation look like for our customers. Our customers are struggling to develop new products faster, they’re trying to be more innovative, they have new startups that are always entering the marketplace that are threatening them, and so they’re looking for how they can go faster, whether you’re a startup with a new idea or with you’re existing company that is maybe feeling a little bit threatened by some of these startups.
And so we see also challenges with sustainability, that there’s new requirements for sustainability, and every company has this now at the front of their mind that we want to have more sustainable products and more sustainable processes. And another challenge our customers are facing is they’re sometimes finding it hard just to hire enough employees. There’s a shortage of engineers, there’s demand for more engineers in software and systems engineering, there’s demand for more technicians out in your manufacturing facility, and our customers are struggling to hire and fill some of these roles. And so they’re looking at business transformation, how do they go faster, how do they address sustainability, how do they address their workforce issues, and they’re looking at transforming their businesses. And they’re turning to digital transformation to help them transform their business.
And we’ve been very successful in how we’ve been able to give them connected systems and interoperability between different applications, and a seamless transition from engineering into manufacturing. But I think the next big thing on the horizon is how do we implement artificial intelligence and machine learning into our solutions, because this is now what’s going to truly help companies move from just connecting solutions to really automating their solutions and then going into generated design and really optimizing a lot of their products and their processes. So this is the next big transition that’s going to really transform how our customers work and the solutions that we provide for them.
Justin Hodges: I see it the same way. And what’s exciting is I think a lot of people have been doing or working in AI for decades would say every 10 years or so there’s a little surge in interest and it fades away again, but I’d say this time around it’s sticking. So you’ve seen already in the lifecycle of AI products out there that it’s gone from this early phase to actual being adopted and implemented and used, and it’s here to stay. So I think this time around, I think it’ll be quite exciting. And earlier we were talking about just this transformation and how far we’ve come in the simulation community, and you gave a really nice example about how our problem statements really shifted from considering a few designs to now many designs, from tens of designs to thousands of designs. Given your extensive industry background and expertise in this field, it’d be great to hear you speak a bit on that.
Dale Tutt: Absolutely. So one of my roles when I was at Cessna Aircraft Company, we were looking at new design concepts for new airplanes. And I think our experience was similar to a lot of other companies in this area where as we were looking at these new concepts, we would look at maybe 10 different airplane concepts. So we’d have a set of requirements for the airplane, and we’d look at different wing configurations and different tail configurations, different engines, but due to the constraints of time and the fact that we were doing a lot of this work manually and we were connecting, we were doing an aerodynamic analysis in one part of the company or one group, we were doing a structural analysis in another group, and then we were trying to connect the results manually, what we saw then is it was really hard to look at more than maybe 10 or 20 different options, different configurations of an airplane or new program over a period of three or four months.
So we were often constrained by time and just the number of people we had working on the problem. But nowadays, as we start to automate a lot of these processes, we’re now starting to see customers looking at tens of thousands of different configurations. So they set up their requirements for their new program, and now they’re looking at tens of thousands of options over the same period of time, maybe even just in a few weeks sometimes, and coming up with a much more optimal solution than what companies were able to do 10 years ago when they were just looking at maybe a dozen or two dozen different options. So a much different environment that we’re able to operate in now.
Justin Hodges: I think that’s a ripe problem for picking for generative AI. So the foreground here is generative AI comes from very, very complex models on large amounts of data, general objective being to produce high fidelity outputs from all of this complex and vast amounts of data. And like you said, now the considerations, all these permutations in our design space is increasing, digital transformation, so yes, now we’re trying to incorporate the physical data, historical information, bill of materials, digital data, so it’s the complexity in terms of our data’s increasing. So I think it is an opportunity with a lot of ROI for generative AI. But just to the point, what do you think some of the big win opportunities are for generative AI in your industry purview? Which is your experience being aerospace primarily, but you have a lot more of that in your focus now.
Dale Tutt: We’ve called it generative engineering for several years, we’ve seen some of those aspects today where we are being able to use some AI technology in our design of, say, our electrical engineering solutions, our electrical systems on an aircraft or a car where you’re really starting to optimize a system. You hear examples of some of the automotive manufacturers where they have a couple extra miles of wire even in a car, and that adds 50, 60 pounds to the weight of the car, and that has to now be moved around. And it’s the same thing if you’re in an airplane, you don’t want to have that extra weight. And so we’re seeing examples from multiple industries where they’ve been using generative systems or solutions to optimize, say, the electrical system or maybe to optimize the performance of some pneumatic components, their duct work.
So we’ve seen examples in both electrical and mechanical design over the last couple of years with how customers are able to optimize their solutions, but it’s usually being done more at a component level or maybe a subsystem or system level, not necessarily at the entire aircraft or car or consumer electronics product, whether it’s a new TV or the electronics in a new TV or anything else. So I think we’re seeing a move now where they want to optimize the entire product now instead of optimizing elements within that product.
Justin Hodges: I think that’s a really good key phrase and excerpt to have in there, generative engineering for years. I think it’s illuminating for people to see the jump between generative AI, generative engineering, how there’s some opportunities there. And that’s already, like you said, some area of interest for a while now, even before generative AI had become so popular. And what a great example, 50 to 60 pounds extra, that’s not insignificant whatsoever.
Dale Tutt: It’s not trivial.
Justin Hodges: How would you know that anyway from not having someone on a podcast with firsthand experience? So thanks for sharing. That’s really cool. And then, of course, we’ve laid the foreground for this high fidelity use case for generative AI, but what do you think about the mundane stuff? That’s really what a lot of people are attracted to talking about is automate the mundane, free up people to focus on their actual job descriptions and things like that. What are some mundane things you see out there?
Dale Tutt: And we’ve seen some of this already, for example, where you’re seeing predictive commands, where it starts to track how a designer is working today, and they know that once you’ve added certain features, then the next feature that’s added, sometimes to a part, say, if you’re doing 3-D design, then it starts to predict some of those things. And sometimes people look at that and they go, “Well, so what?” But when you think about the number of operations that a user might perform in a given day, if you can save 10 or 15 seconds per time and you do that 100 times a day, that’s actually freed up a lot of their time, and it’s just in the background. And in addition to that, I think we see a lot of engineers spending time connecting requirements and making trace links to all of the other elements in their design.
So they may be tracing to elements of their simulation or their tracing to elements of their CAD, their 3-D design or 3-D geometry. They’re even making trace links down into their verification plans. And so the more you can start to automate that through the learning that you can do with AI, that you can start predicting that… A lot of times people will look at this and say, “If you just are automating a particular use case, that might work in that scenario, but what about this scenario or what about my industry?” Well, I think the beauty of AI is that over time the system is actually learning from how the people are working and it’s starting to make recommendations and automating those types of tasks based on how the user’s actually working customized to their product or to their industry. And so I think that’s the kind of things that are going to happen in the background that are going to really change how people work.
10 or 15 years ago, maybe not even that long ago, five to 10 years ago, my engineering teams, as we were developing a new design, they were spending probably 60 to 70% of their time creating documents, creating drawings, making the data conversions from the CAD system into the analysis tools. So they’re spending a lot of time, I would just say, managing data. And if you can start to automate all those processes that just consume people’s time, now you’re going to start to allow them to focus on actually solving the complex problems instead of managing data, and imagine the productivity and actually just the job satisfaction that these people will have if they’re spending 100% of their time on solving problems instead of 30% of their time, and so I think that’s the great opportunity. And sometimes I think people look at all the big things that we can do with AI, but there’s a lot of little tasks that everybody does every single day that I think this will really change how they work.
Justin Hodges: I think there’s a lot of low hanging fruit. It’s almost like just the basics from this massive snowball and advancements in generative AI, I think just even the basics can go a very far distance for us in this other industries like engineering simulation, aerospace. And it’s great to see they’re one-to-one, because you’re basically calling out this very generic obvious use case for large language models of allow people to conversationally work with their same software to do these external API calls, AKA orchestrate workflows just from conversation. And everyone across tons of industries, mainstream LLM usage, that’s one of the key focus areas, and I think that’s industry firsthand experience you’ve said is the same. Obviously there’s the use case for support documentation, boilerplate code generation, document search, these are all also very useful things. So it’s good to see firsthand industry experience and needs of what’s out there versus what are the primary areas of focus for these developments made by companies like OpenAI.
Dale Tutt: Absolutely. And I’ll just chime in on that a little bit, I talked about some mundane cases on the design side of engineering, some of these products, but what you said there about code generation and the design of some of these electronic systems and the software, there’s a lot of repetitive tasks even in those processes. And the more you can start to automate that, the more productive you can make all of that work. Verifying the software, it’s very time-consuming sometimes. And so, again, the more you can use AI to help with the auto generation and then the verification, again, a huge benefit for a lot of these customers, especially as we start to see more trends towards software-defined vehicles in multiple industries, not just automotive, but you’re going to see it more and more in aerospace and heavy equipment and other industries as well. So pretty exciting stuff.
Justin Hodges: Thanks for the nice discussion Dale. I think we’ve laid out the foundation pretty well so far. We can end here for part 1 of the podcast. On part 2 we will pick up where we left on and move to discussing activity in certain industries and some of the next big steps for AI within this mass adoption of digitalization. So, for now thanks, we’ll talk soon.
Siemens Digital Industries Software helps organizations of all sizes digitally transform using software, hardware and services from the Siemens Xcelerator business platform. Siemens’ software and the comprehensive digital twin enable companies to optimize their design, engineering and manufacturing processes to turn today’s ideas into the sustainable products of the future. From chips to entire systems, from product to process, across all industries. Siemens Digital Industries Software – Accelerating transformation.