Today meets tomorrow: The opportunities and limitations facing AI and the digital twin (Part II)
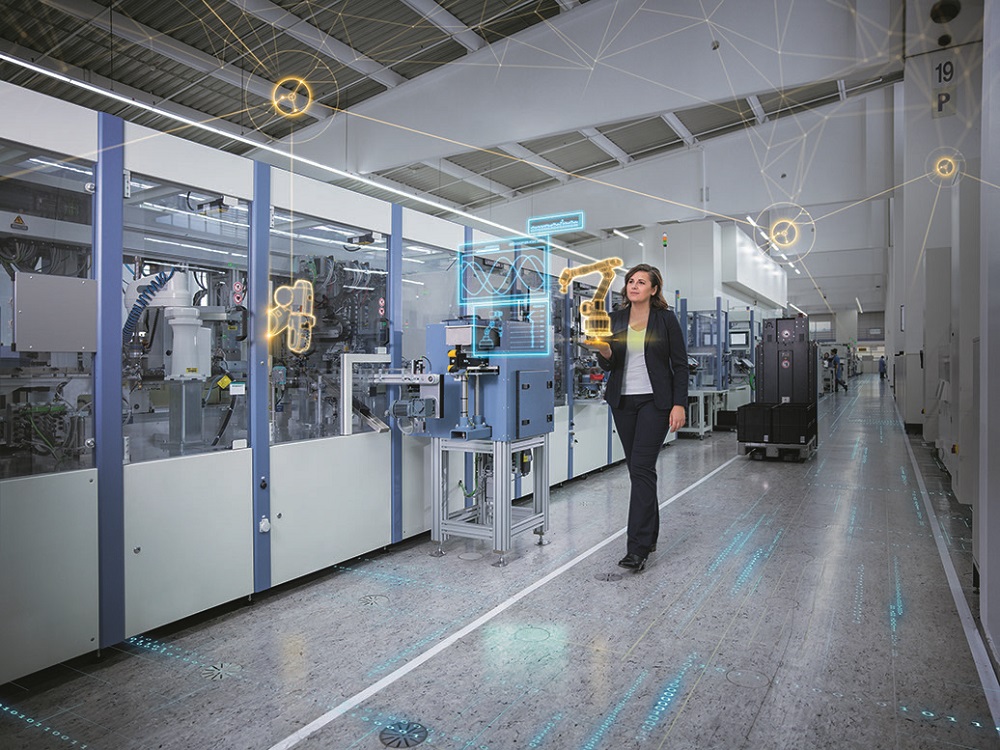
In our previous blog, we discussed the challenges of simulation and the digital twin as well as how artificial intelligence (AI) can bring real world data into the digital twin.
In this part, we will focus on the use of AI on the factory floor and the limitations engineers could face when using AI to implement a digital twin.
The potential uses for artificial intelligence are enormous, yet unexploited. While artificial intelligence can help build simulation models, many are using the technology to collect and analyze vast amounts of data. Financial institutions use its algorithms for fraud detection and manufacturers can review products for defections and use consistently-flowing data to build a more robust digital twin.
AI and the digital twin on the factory floor
Artificial intelligence has tremendous potential not only in designing products, but also when it comes to making production more efficient, flexible and reliable. As industry continues toward increased digitalization, data in production environments are the basis on which entire plants operate and systems generate.
AI can bring greater reliability and efficiency to the factory floor. For instance, unplanned downtime has always been a major problem that industrial manufacturers have faced. A digital twin can use AI-based analyses for predictive maintenance, which can reduce downtime by scheduling or alerting of maintenance needs before they become problematic.
Digital twins have been used to structure the planning and design of products and machinery, even production operations themselves. This flexible and more efficient approach have helped manufacturers produce more affordable, high-quality and customized products faster.
AI can now use these machines and processes to gather insights from these high volumes of data by themselves and optimize their processes during live operation. On top of that, neural processing units are now part of controllers for object recognition, visual/sensor-supported quality checks in production plants and image-guided robot systems are more flexible when reacting to unexpected situations. If a machine-learning algorithm spots quality defects, it can respond automatically during runtime.
Edge computing can process data where it’s generated, whether that’s at the plant or the machine. One such location is the Siemens Electronics Works Amberg, Germany, which produces Programmable Logic Controllers (PLCs) and other industrial automation products for the world market. To ensure quality, the printed circuit boards (PCBs), a critical part of the controllers, are tested through x-ray during the manufacturing process. The problem with X-ray testing is that it requires long throughput time and hence is the factory’s bottleneck. An additional x-ray machine would result in capital investment of €500,000 and must be integrated into the manufacturing environment. Instead, AI experts trained an algorithm on an edge application at the plant to predict whether the soldered joints on the PLCs were free of faults; in other words, whether or not an end-of-line test is necessary. Thereby, test efforts for the x-ray machines were reduced by up to 30 percent in the first step. Closed-loop analytics are planned to be factored into production in the near future.
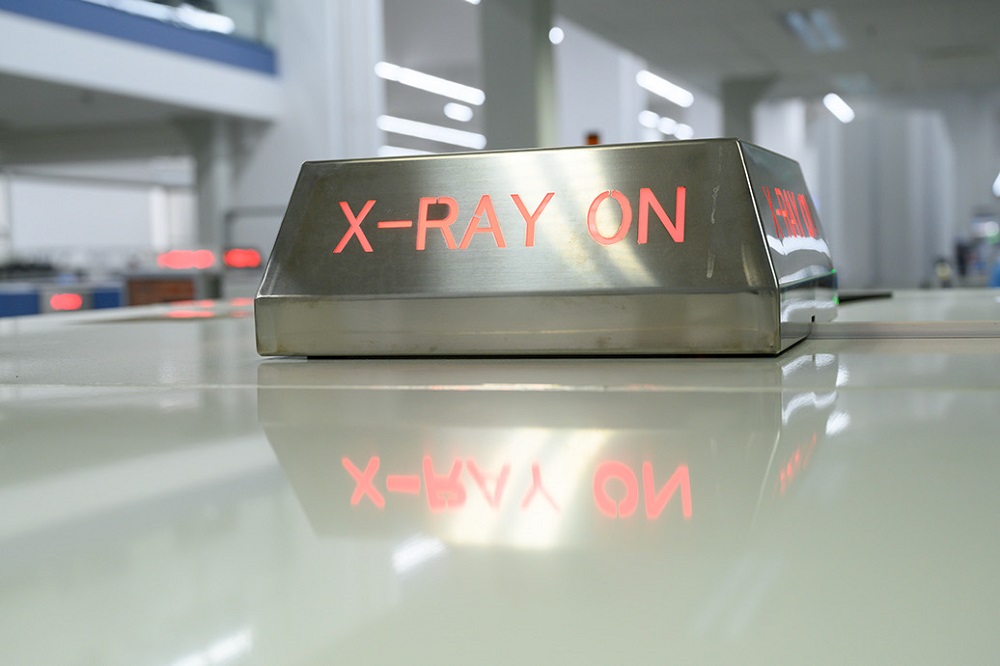
Limitations of AI in the digital twin
Regardless of its benefits, AI doesn’t come without its challenges. There are several shortcomings and limitations that engineers are likely to encounter when using AI techniques to implement a digital twin:
- AI technology needs trusted, ‘clean’ mass data for teaching the AI system with respect to the expected properties/behavior. This is often hard to get and to verify, especially for newly designed systems. On the other hand, the verification and certification of AI-based behavior in safety-critical applications, such as autonomous driving, is a challenge yet to be solved.
- A digital twin mirrors the properties/behavior of a concrete asset, while AI systems are essentially mirroring statistical properties/behavior. This can make AI twins problematic.
- AI technologies have no physical background. They can show deviations between real and expected behavior but cannot easily explain the physical reasons behind it.
- Finally, customers are always concerned with how they can protect IP and the data associated with those assets while data sharing.
There are two basic approaches to addressing the limitations when combining conventional AI techniques with physics models:
- Sequential approaches. Using AI to detect anomalies before a simulation is employed for detailed analysis. Conversely, simulations are used to teach AI before it is applied as fast surrogate model for simulations.
- Parallel approaches. AI and simulation models are combined. For example, by substituting well understood components by AI surrogates in a system simulation, using AI to calibrate simulation models, or using AI to automatically analyze complex simulation results.
Summary
There is massive potential for growth, efficiency and innovation when using artificial intelligence. The potential applications for AI are monumental across industrial manufacturing. This is merely the beginning of the opportunities this technology will provide.
Comments