Today meets tomorrow: The opportunities and limitations facing AI and the digital twin (Part I)
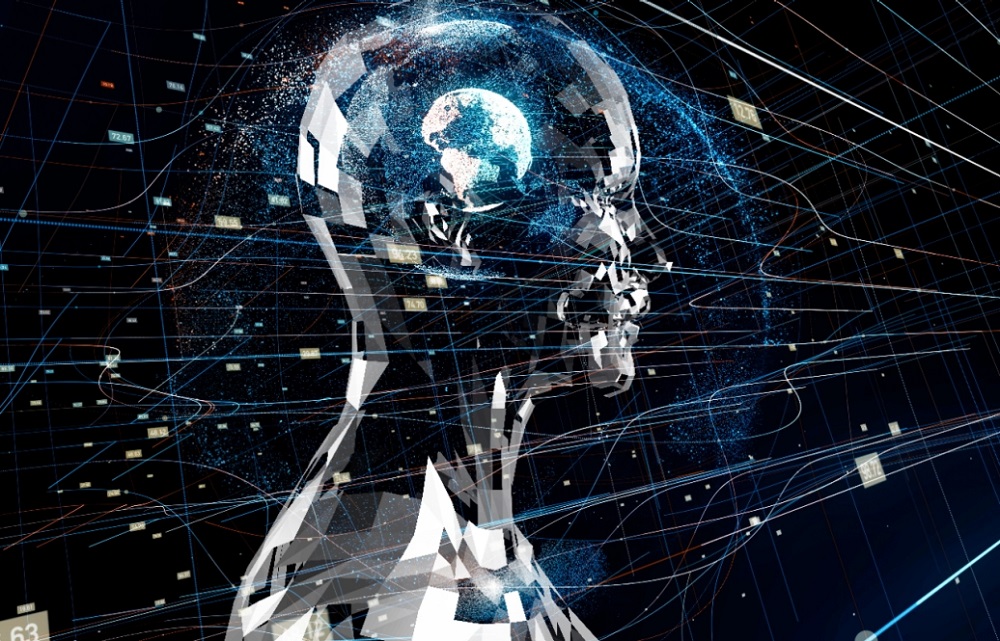
Artificial intelligence (AI) is at the forefront of many industry leaders’ minds as it’s positioned to be a major enabler and disrupter. However, its implementation is still in the early stages. How will designers and engineers of sophisticated, complex systems use artificial intelligence and how will the digital twin help advance its capabilities?
First, it’s worth noting how AI and the digital twin can work together today to aid designers.
AI Assisted Design
AI and machine learning (ML) can assist to create user interfaces based on expected tasks to increase user adoption and productivity. By changing design parameters and running AI algorithms, designers can evaluate possible best fits based on the results of the algorithms. They can also use data from older, existing designs to uncover latent design properties and then use these findings to improve the current model.
Generative design is an approach to find an optimal solution by systematic variation of parameters, structure or shape of a design. The evaluation is mostly done by simulations orchestrated by space exploration tools. AI-driven behavior models for well-known parts of the system, or parts that cannot be modeled mathematically but learned instead, speed up these evaluations.
AI can recommend next steps based on predictions of the previously completed design. This supports better design quality by guiding the designer or engineer to the next logical step without having to search for the step in the menu structure.
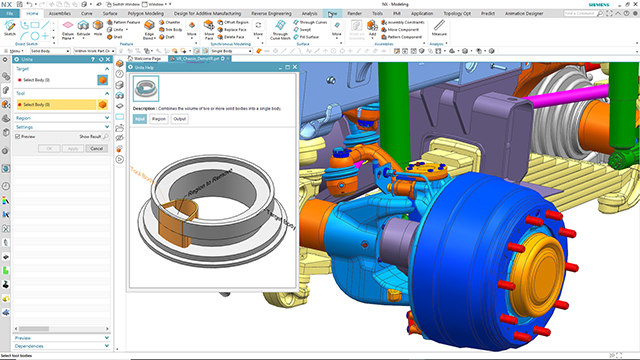
Challenges of simulation and the digital twin
Because a digital twin is a completely virtual representation of a physical product or process, speed and accuracy are key when it comes to creating it. The digital twin needs to represent all aspects of the system, ranging from CAD and software model simulation to flow dynamics and electrical circuit simulations. There are a number of engineering disciplines that come into play in development digital twins and the variety of model types required to create these virtual representations.
Today, the ability to combine the models from all the authoring tools in the digital twin flow is limited by the lack of standards. Even when there are standards, they do not completely specify the semantics required for these tools to all behave correctly when combined.
Engineers and designers can benefit from AI technologies to build digital twin models. They can compare “learned” behavior against simulation models. This helps in a myriad of ways including:
- Identifying parameter dependencies
- Calibrating the digital twin models
- Discovering actual issues in the simulation models themselves
On the one hand, model order reduction (MOR) models, being fast but not as accurate as standard simulation models, can speed up the learning by simulation for AI models. On the other hand, well trained AI models can substitute the simulation models where speed and memory footprint count, e.g. as virtual sensors on controllers, or when architectures are evaluated, as in generative design. Due to that acceleration, teams can explore many architectural implementations and verify the results in the same amount of time it would have taken for one original simulation run.
AI Brings Real World Data into the Digital Twin
Once the design has been released to manufacturing and is out in the real world, the next challenge is how to correctly parameterize and calibrate the digital twin based on the different states of the physical asset as well as capture the use cases that match up with the actual use of the physical device. It is essential that the digital twin accurately represents the current state of its physical counterpart.
Artificial intelligence helps with this challenge by using, sensing and interpreting the enormous quantities of data from real-world conditions to optimize parameters as well as predict behavior changes —such as those caused by aging. This ensures the virtual representation is adjusted throughout the physical asset’s lifetime and the digital twin is updated accordingly
Using data generated by digital twin simulations to further train AI models helps create a closed loop that produces feedback. This can then be used to enhance or optimize an existing digital twin.
This is possible at every step of the design and manufacturing and lifecycle process. For example, using parameterized CAD models to train neural networks can speed up the evaluation of parameter variations when applied to construction problems. Leveraging synthetic data generated from CAD tools can create pre-trained AI models that in turn can be adapted to real-world data through transfer learning. In addition, using environment scenarios captured and modified in simulation can improve AI-based control, such as for autonomous cars.
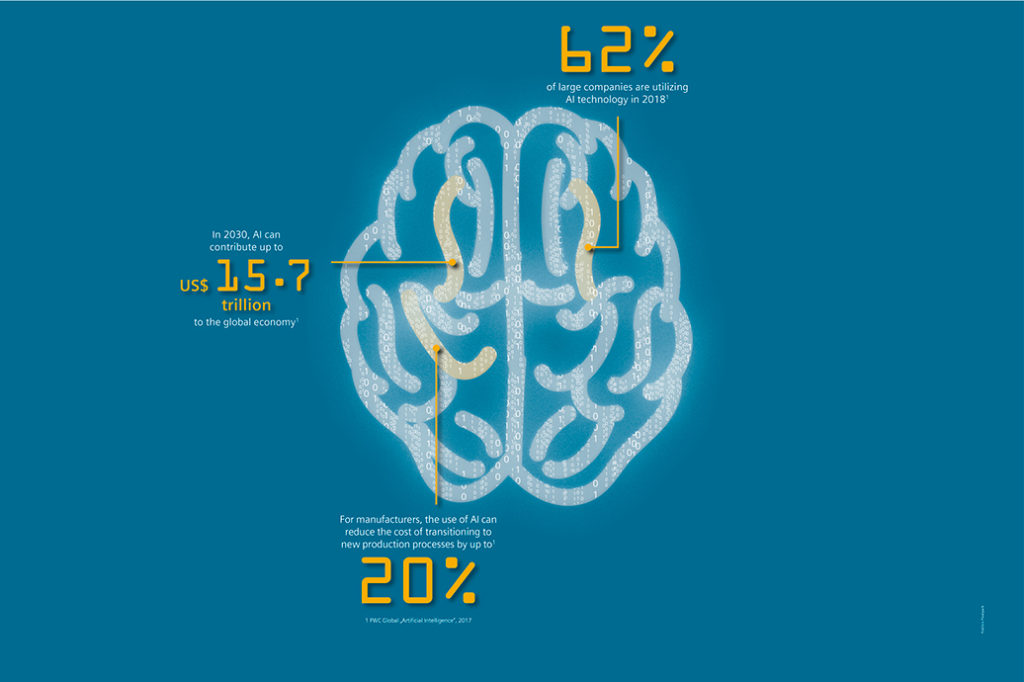
This concludes part I of our series. In part II we will address some of its uses on the factory floor and the limitations engineers could face when using AI to implement a digital twin.
Comments