Where today meets tomorrow: harnessing the power of AI (part I)
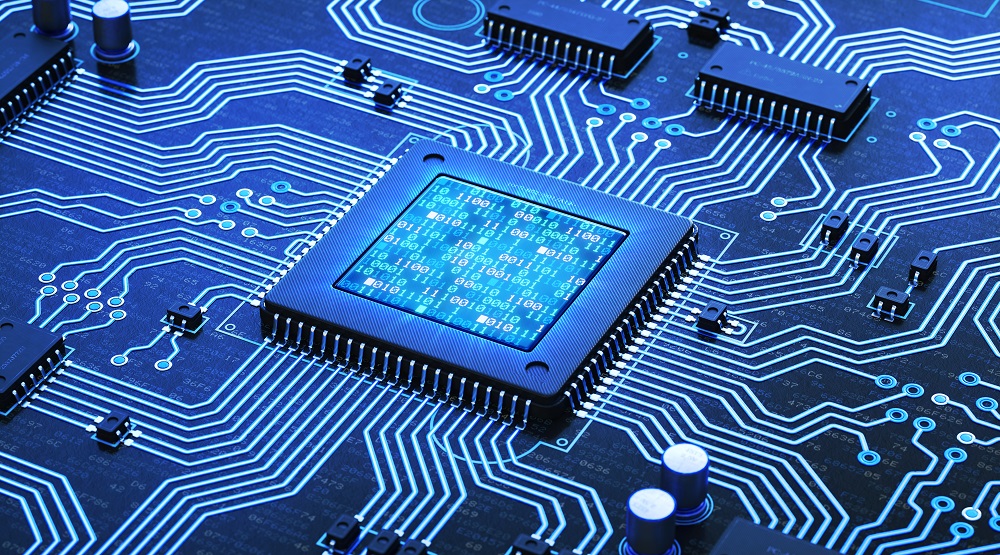
Semiconductors are at the core of every major disruption and innovation. Today, with an unprecedented level of investment, we are witnessing artificial intelligence (AI) become an engine for the growth of the semiconductor industry.
Manufacturing companies as well as software companies are embracing integrated circuit (IC) design as a new core competency. These players are driving the complete virtualization of the product development and manufacturing process — building the most comprehensive digital twin. They’re also in the best position to solve the big bottleneck of the practical application of AI: a sufficient amount of relevant and complete training data and a product development platform that allows to design and verify functionality and real-time performance of advanced, AI-driven features.
AI – a growth engine for semiconductors
It is mind-boggling to see how fast the semiconductor industry is advancing and how much investment and research goes into AI and machine learning (ML). Given the enormous investment both at the corporate level and in venture capital, there is little doubt in my mind that AI will be at the core of the next industrial revolution just as much as in the next “killer products”.
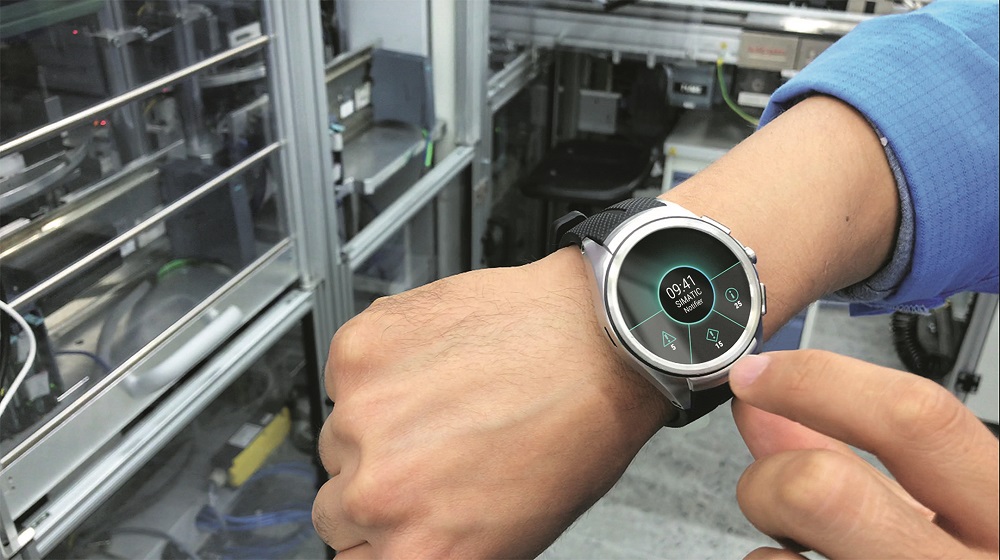
It has been this way for the past 40 years – whether it’s the Palm Pilot, the smartphone or the smart watch — they all started with limited capabilities and were fairly clunky. Thanks to Moore’s law, the next generation is twice as beautiful, sleek and powerful, and makes us want to open our wallets.
In 2018, venture capital funding of fabless semiconductor companies in AI exceeded the level for the entire industry in 2002. Like a seismic force, companies are creating domain-specific AI and deep learning capabilities. By an informal analysis, there are around 40 companies specializing on vision applications and about the same number focused on cloud/HPC AI and AI applications on the edge, respectively.
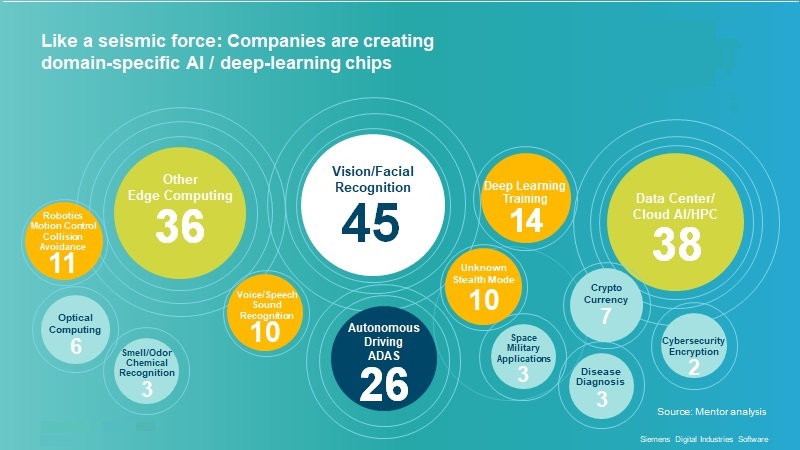
There’s reason to assume that we will continue to see more and more powerful, power-efficient and highly specialized chips for AI and ML applications, enabling more and more accurate applications with amazing real-time responsiveness.
The semiconductor playing field is shifting
At the same time, companies not known as “chip designers” or manufacturers are increasingly entering the scene: not only Google, Facebook, and Amazon, but also Ford, Lockheed Martin, Boeing and Northrup Grumman, just to name a few.
The simple reason for this focus is the potential for differentiation. For any manufacturer of a consumer or industrial product, having the fastest, most robust and most power-efficient hardware can give them several years of lead. For example: advanced driver assistance systems (ADAS), industrial vision applications, autonomous control and other disciplines.
Especially when it comes to advanced applications and AI, systems companies may have an advantage to overcome one of the big bottlenecks: the right data.
Availability of data is still a limiting factor for many AI applications
Oftentimes, we are led to believe there is an abundance (if not a deluge) of data. However, there is quite a number of highly-relevant industrial applications of AI and ML where that’s not the case at all.
Take autonomous driving as an example.
Akio Toyoda, Toyota’s president and grandson of the founder, has stated that to accomplish safety in autonomous vehicles, it will take an estimated 8.8 billion miles of testing, including simulation to complete verification of its driving functionality. To do this level of road testing requires driving to the moon and back 16,000 times.
The same is true for many scenarios of predictive maintenance. If a component breaks after an average lifetime of ten years, it takes a long time to gather enough data to predict its failure from the real world.
The digital twin: how to overcome the data bottleneck
Automakers, machine builders, aerospace and defense companies, and consumer product manufacturers have been virtualizing product development and manufacturing for many years. They have pursued the vision of a comprehensive digital twin of the product, its manufacturing process, and, with the rise of IoT, its operation.
As products grow more and more complex, a digital twin that can accurately represent the physical and mechanical product, the software driving its electronic components, and even the behavior of the IC processing the input from a multitude of sensors is becoming a necessity. It’s the tool that engineers will need to use to develop and verify tomorrow’s products. However, it is also capable to create the immensely large datasets needed to build and validate AI/ML-driven features that are robust enough for the real world.
This concludes part 1 of a summary of what I talked about at VLSID on January 7, 2020. In my next blog post, I’ll discuss how the digital twin of the product becomes part of the vision of a mobility digital enterprise and how AI will factor in to creating integrated mobility solutions that span all the way from the chip to the city.
Comments