Virtual Development and Demonstration of Advanced Driver Assistance Systems
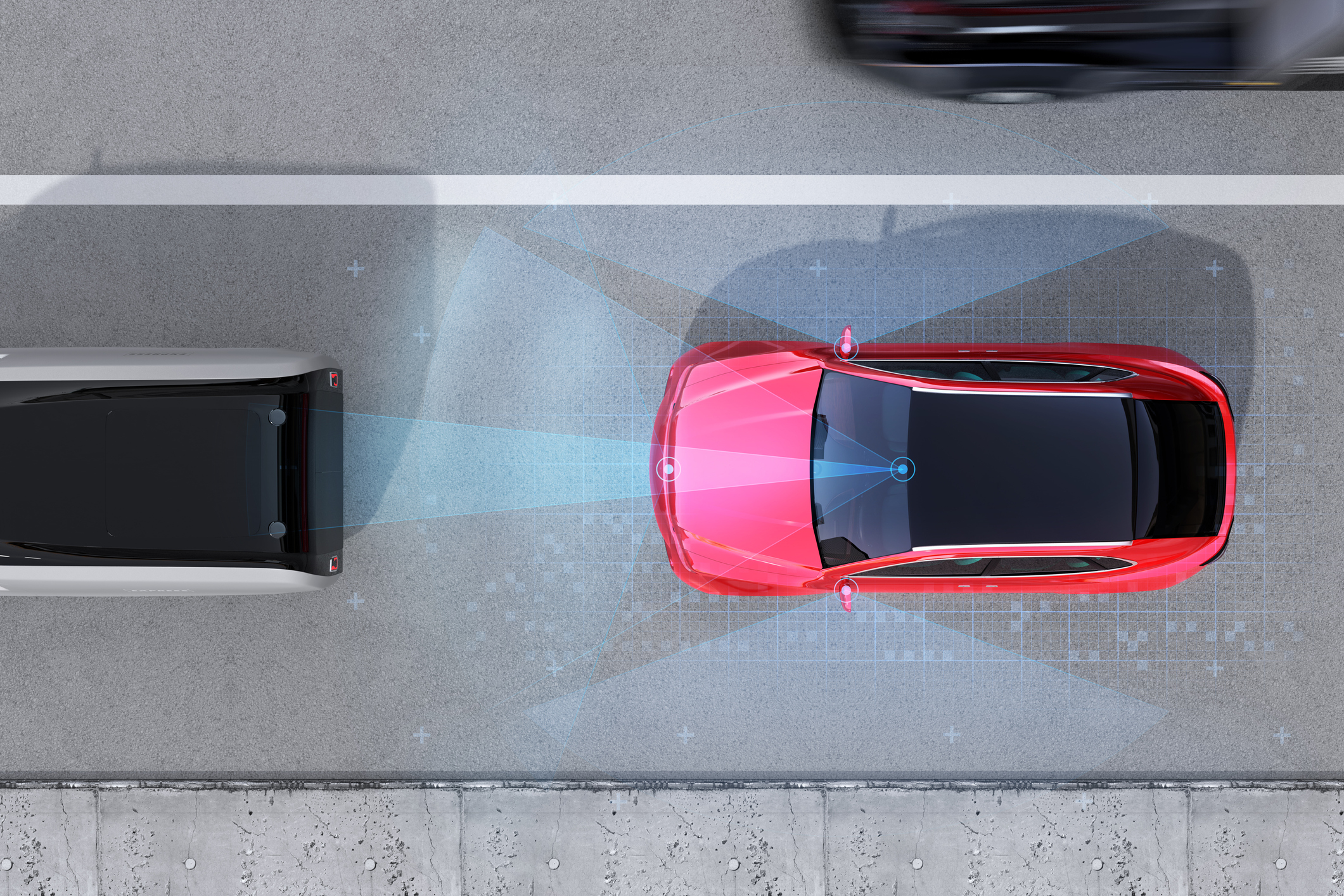
How long until truly autonomous vehicles are on the road? Technology is advancing in vehicles at a very fast rate and new features are constantly being added in an effort to enhance comfort, convenience and safety. Numerous manufacturers have their version of auto-pilot, lane departure assist, adaptive cruise control, automated parallel parking among others. These enhancements are known as Advanced Driver Assistance Systems (ADAS). These systems aid the driver while driving the vehicle, and control some of the behavior of the car directly. This helps minimizing human error and thus reduces injuries and fatalities.
One of the first challenges is that the design, development, testing and validation of ADAS functionality is complex and requires a lot of time, money and effort to complete. This is where virtual verification and validation come into play; to be able to virtually evaluate the vehicle behavior and performance across a range of scenarios at a drastically reduced timeframe and cost.
The second challenge is that new vehicle designs equipped with advanced driver assistance systems need to be validated with respect to human perceptions of comfort and risk. Therefore, human-in-the-loop simulations are used to evaluate a wide range of scenarios in driving simulators.
ENABLE-S3 project: virtual testing & verification – accelerated application of highly automated and autonomous systems
The European Commission is funding R&D to support progress in this technology field, amongst others with the EC ECSEL/H2020 research project “ENABLE-S3”. This project was a collaboration of a large consortium with more than 70 partners that focused on improving verification and validation methods of autonomous driving functions for ground, air and marine vehicles.
In the ENABLE-S3 project, Siemens Digital Industries Software has worked out solutions to address both aforementioned challenges. Firstly, by delivering a simulation platform as a virtual verification and validation environment that is capable of modeling software, hardware and driver/human-in-the-loop. Secondly, by enabling the driving simulator (See the previous blogpost) to offer a highly realistic driving experience to the human driver by using Simcenter Prescan, Simcenter Amesim and Simcenter 3D to model the environment, the vehicle and its components.
To show our capability, let’s dive into three Use Cases that have been evaluated in the ENABLE-S3 project: 1) Adaptive Cruise Control (ACC) on highway, 2) Intersection Crossing and 3) Valet Parking. They are executed on the virtual demonstration environment in Simcenter Prescan shown in Figure 1. Within this virtual environment, the vehicle and its components are modelled with Simcenter 3D and Simcenter Amesim. This offers realistic vehicle dynamics behavior in Simcenter 3D with an accurate representation of active and adaptive components in Simcenter Amesim.
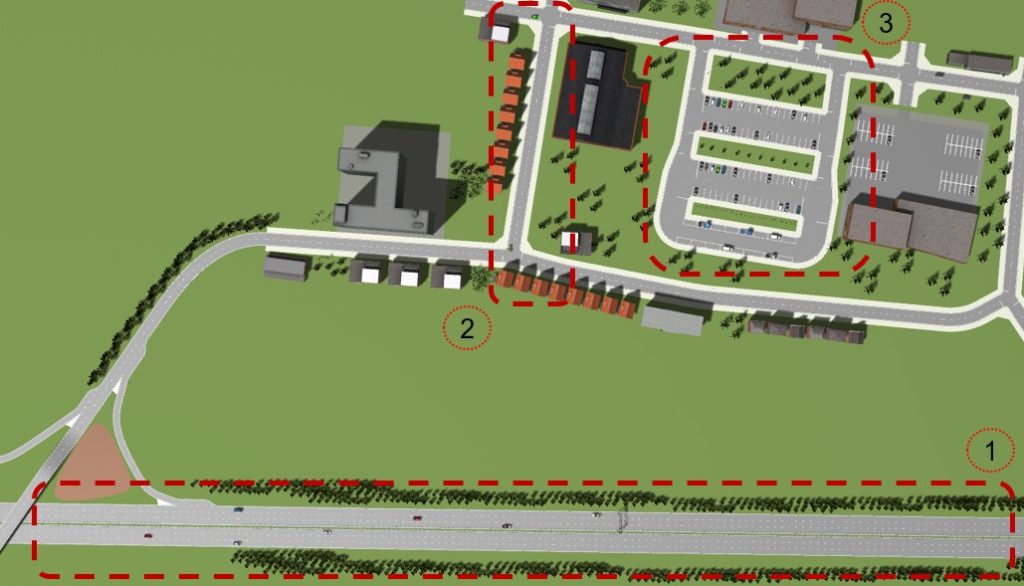
Adaptive Cruise Control (ACC) on highway
The first automated driving functionality (ADF) investigated and used on the simulator for demonstration is Adaptive Cruise Control (ACC), which is a Level 1 ADF according the SAE definition on vehicle autonomy. This system keeps the vehicle at a constant velocity set by the driver (cruise control mode). When a slower leading car is approached, the system automatically adapts the velocity and keep a predefined distance to avoid a rear-end collision. The actions taken by the ACC control algorithm are based upon the information given by two scanning sensors: the long range radar (LRR) and the short range radar (SRR). These sensors continuously evaluate the presence of a lead car. In the case a slower car gets inside the sensor measuring range, the control procedure computes the relative distance and speed between the two vehicles. The brake system is then triggered appropriately to slow down, in order to maintain a safe constant distance.
In the demo case, a straight highway piloting scenario is considered. In this scenario, the ego vehicle (which means, the vehicle with the autonomous driving algorithms under consideration) is cruising at approximately 100 km/h and approaching a lead vehicle. To avoid the collision, the ego vehicle slows down to the same speed as the lead vehicle as is shown in Figure 2. After 41 seconds, the ego vehicle decides to overtake the lead vehicle. From this moment, the ACC increases the velocity of the ego vehicle to the previously defined setpoint.
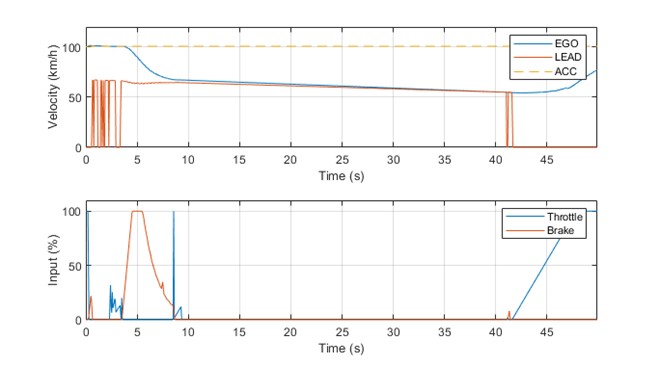
Intersection Crossing
The second ADF implemented on the simulator for demonstration is an autonomous intersection crossing, which is a Level-2 ADF. In this case, a cross-road scenario is chosen and it is assumed that two vehicles are approaching the intersection, both with a predefined trajectory. The autonomous intersection crossing functionality comprises of a lateral controller for being able to make turns and longitudinal controller for velocity adjustment. For the collision avoidance at the intersection, an algorithm is adopted and implemented that assumes that both vehicles are following a predefined trajectory, which makes the problem solvable by only changing longitudinal velocity. Therefore, the algorithm forces the ego vehicle to either brake or accelerate in order to avoid a collision. What will happen is that one vehicle will try to exit the intersection as fast as possible and the other will try to slow down as much as possible to avoid a collision between both vehicles.
The autonomous intersection crossing controller is demonstrated in the second scenario. The developed control algorithm is validated by having the ego vehicle approaching a cross-road together with one other road user. At a certain moment, their trajectories are overlapping and a collision might occur. Both vehicles are making a left turn and there is no communication between the road users. In this scenario, the ego vehicle arrives first at the intersection. It decides to slow down and give the priority to the other vehicle, because there is a chance of collision. After the road user leaves the intersection, the ego vehicle accelerates and makes a left turn. The velocities and control inputs are shown in Figure 3.
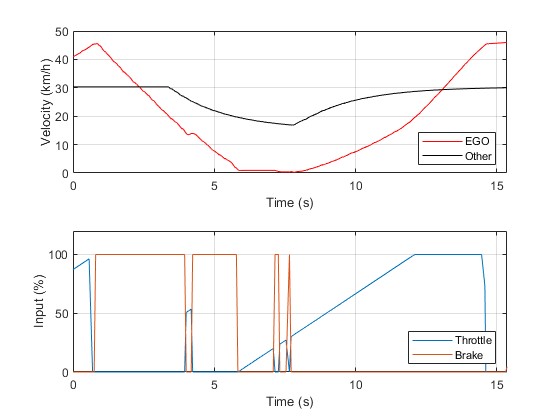
Valet Parking
In this case, the path planning and path following algorithms for reverse parking are implemented which is a Level-4 ADF according to SAE definition. This work is based on previous research on valet parking. The path planning uses a so-called “Hybrid A*” algorithm to find the shorted feasible path from the current position to the selected destination. This planning is first performed before the start of the maneuver. In case the path cannot be followed, e.g. in case of an unexpected obstacle, the vehicle is automatically stopped and the path is recomputed. Once the path is available, then a path following controller is used in real-time to move the vehicle.
The final scenario in the demonstrator is a reverse parking maneuver that requires a reverse turn of 90 degrees. The vehicle is driving to a position near an empty parking spot. The middle of the parking spot is used as the goal of the path planning algorithm. The obtained path is shown in blue in figure 4. Once the path is available, the path following controller is started. This selects the reverse gear and drives the vehicle to the selected parking spot. Because the planned path is computed with a more simplified model, this path is not followed perfectly, as the actual vehicle has a more complex behavior compared to the simplified model. This is replicated in the virtual environment with the use of the high-fidelity vehicle model in Simcenter 3D that uses the steer wheel as input.
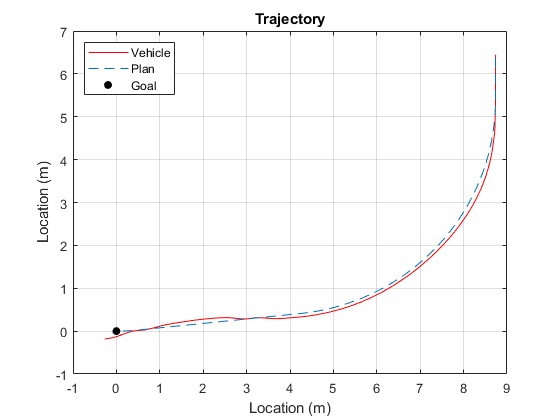
Conclusions
A virtual demonstration environment has been achieved, which allows the virtual validation of an ADAS for different variants of vehicle, environment models, sensor models, decision making algorithms or driver behaviors. The inherent flexibility and scalability of the platform allows engineers to test many scenarios, available models (vehicle, sensor or controller), and weather and traffic conditions. This virtual design, development and testing approach helps to increase trust and confidence in the developed algorithms and technologies, and ultimately to increase the safety both for the test operators, road actors and infrastructure. With this technology, it becomes feasible to virtually test scenarios, which are difficult and often impossible or too expensive to test in real life, such as certain weather conditions, and fault/failure of components. Three Use Cases have been presented that show that realistic vehicle active safety and autonomous driving scenarios can be covered virtually.
The platform will be used in the future to perform a variety of research activities, including development of human machine interfaces for future autonomous vehicles, human perception in autonomous vehicles, driver training for autonomous vehicles, model-based testing and validation.
Acknowledgements
Siemens would like to acknowledge the ENABLE-S3 project that has received funding from the ECSEL Joint Undertaking under grant agreement No. 692455. This joint undertaking receives support from the European Union’s HORIZON 2020 research and innovation programme and Spain, Portugal, Poland, Ireland, Belgium, France, Netherlands, United Kingdom, Slovakia, Norway.
Comments
Comments are closed.
fsdfsdfsdsdassadsad