Thermal management for a deep learning machine
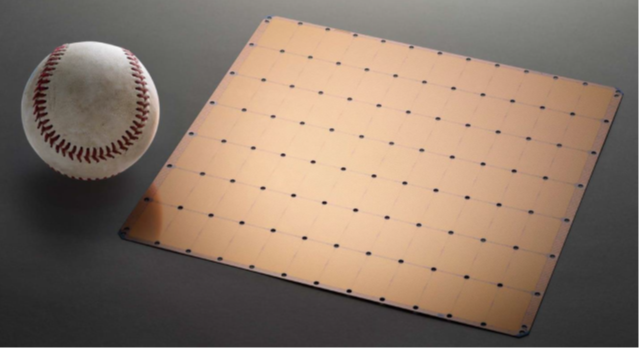
One of the world’s fastest AI chips. Should it be air or liquid cooled? Thermal simulation reveals the most feasible approach.
Artificial intelligence (AI) will bring deep language processing and the ability to see patterns in large amounts of data. It could bring benefits in research such as breakthroughs in treatments for cancer and brain injuries. Already, designers are shaping the new machine learning systems and the super-powerful chips that will drive AI.
Computer architects Cerebras pioneered the Wafer-Scale Engine AI deep learning chip. This is one single electronic chip that almost fills a 12” silicon wafer.
It contains:
- 1.2 trillion transistors on a 46,225 mm2 silicon wafer,
- 400,000 AI optimized cores,
- 18 GB of on-chip memory,
- 9 petabytes of memory bandwidth
- and uses the TSMC 16 nm process.
The chip is designed with 84 ICs on one wafer to obtain the low latency that is critical to achieving such massive processing power. Of course, each IC generates heat, making a red-hot 17.6 KW in total, so the WSE needs an equally powerful cooling system.
The challenges of cooling the WSE
Removing 17.6 kW of heat was not the only challenge. The cooling had to be completely even across the whole wafer because the WSE will only tolerate a small variance in temperature between the ICs. The cooling system had to ensure that the variation in temperature would never exceed 7°, a very narrow tolerance. There was a practical constraint too, the final design needed to fit into a standard 19” rack.
To devise a thermal management strategy for the WSE, Cerebras partnered with Electronic Cooling Systems (ECS) in Santa Clara, California who would conduct tests and design a suitable cooling system. ECS are experts in state-of-the-art simulation and measurements and thermal management for electronic systems. ECS;s remit was to cool the wafer to below 85° with an ambient temperature of 30° to prevent the heat from compromising the performance of the wafer.
Two possible cooling strategies
ECS considered air cooling and liquid cooling. Both appeared feasible.
First, ECS considered air cooling with fans. Would it be feasible, or would the power density dry out the vapor chamber in the heatsink base and result in a thermal runaway? ECS began with simulations to model an air-cooled solution with Simcenter Flotherm XT to see how the results would compare to the requirements.
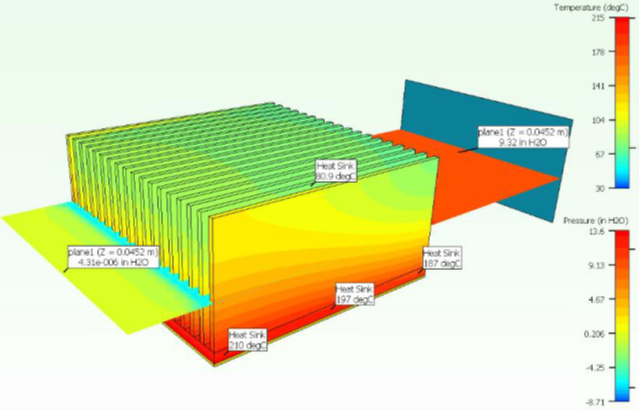
They also evaluated the alternative approach which used liquid cooling with a cold plate and a heat exchanger (HEX) to reject the heat. For electronics cooling applications it is usual to use a mix of 30% propylene glycol and water with corrosion inhibitors. This design used a HEX, a cold plate and a pump and needed to maintain a very low coolant pressure loss between the inlet and the outlet. ECS used Simcenter Flotherm to measure the 20 kW HEX test set-up and measured the airflow and performance.
Simulation leads to one final design
Quick simulations with Simcenter Flotherm soon answered their initial questions. Further modeling enabled ECS to see the pumping pressure and the pressure of the coolant to the microchannels. Finally, ECS modeled the cold plate for the final design that would maintain the very uniform temperature distribution across all the 84 IC sites.
Simcenter Flotherm XT provided useful detail about the effects of fans and heat sinks. With the second model using liquid cooling, simulations showed how the cooling would work. Importantly, simulations revealed that only one approach would lead to a feasible design.
In our on-demand webinar, Guy Wagner, Director at ECS discusses the simulations and shares the results that shaped the final ground-breaking design for the WSE.
Further resources
- Read our White Paper: “Thermal Management Solutions for Electronics”, to learn how thermal simulations can impacts your electronics design.
- Experience Simcenter Flotherm electronics cooling software for yourself to understand its functionalities and see the value it can deliver for you. Download the free trial here.
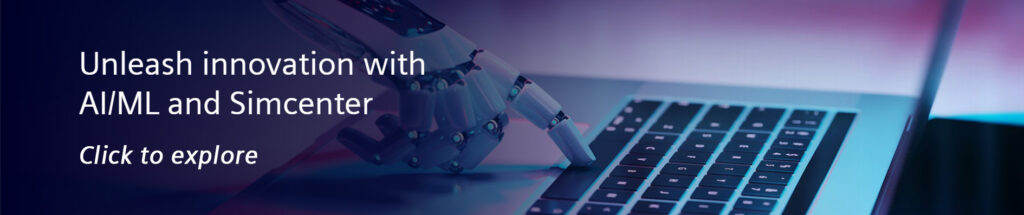