Connecting the virtual world with the real world to save millions on water distribution
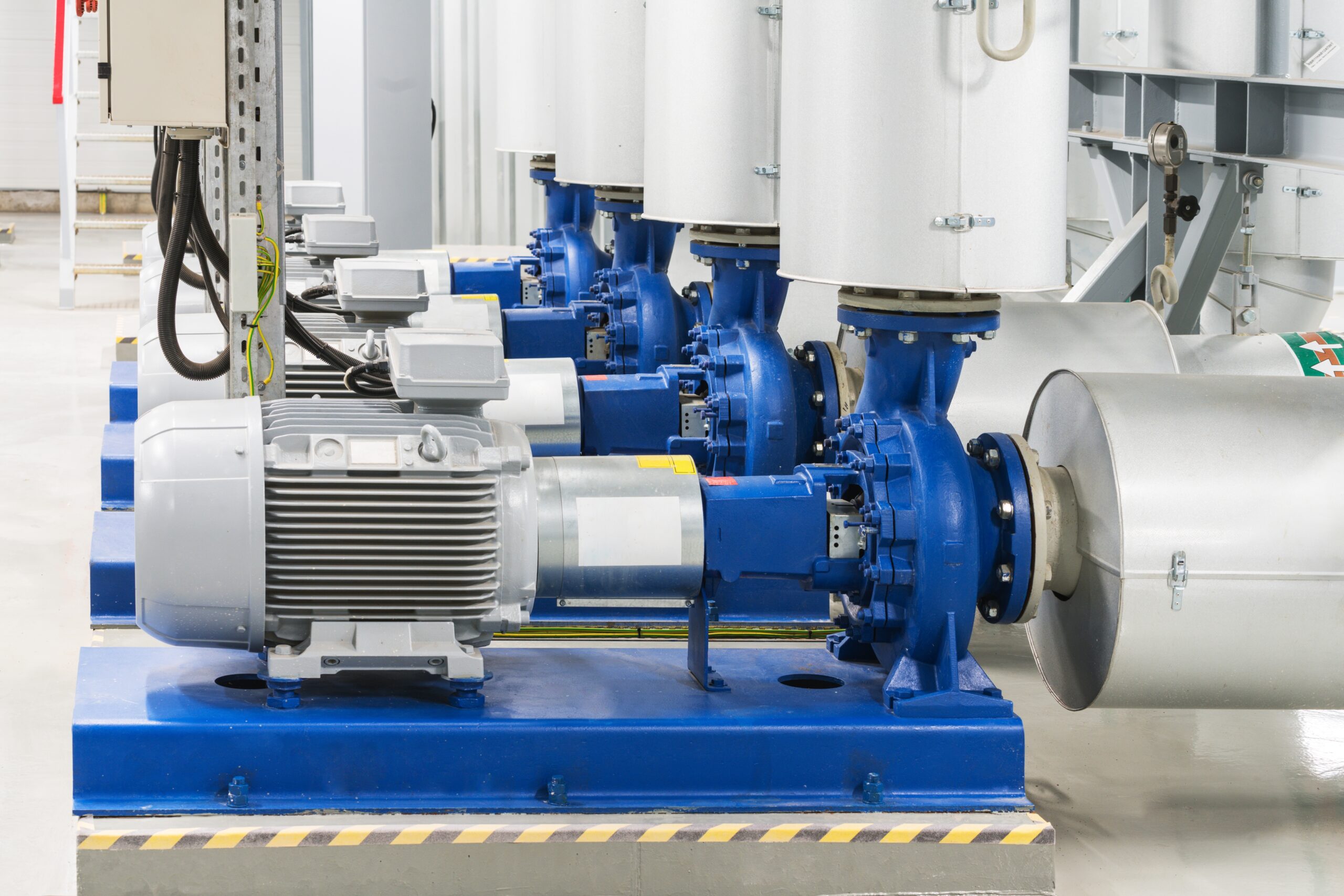
Using executable digital twins to perfectly match supply and demand, reduce energy consumption and increase throughput based on connected physics-based models.
Introduction
Water distribution networks are an essential part of our modern infrastructure, providing access to clean and safe drinking water to communities around the world. As our population grows, urbanization expands and water resources are impacted by climate change, the future of water distribution networks is becoming increasingly important.
To meet future needs, the water industry embraces more and more digitalization, including the use of virtual models to help improve the efficiency, reliability, and resilience of water distribution networks. These technologies allow for real-time monitoring of sensor data, detection of leaks, and improved management of water resources, leading to more efficient and sustainable water distribution.
Going one step further is to fully embed and connect the simulation model or digital twin to the real physical asset, in our case a network of pumps and reservoirs in a large water distribution network.
What’s the use case?
The observant reader noticed that we mention ‘executable digital twins’ in the sub-title, and not ‘digital twin’. Although closely related, we define a few criteria to distinguish an executable digital twin (xDT) from a digital twin:
- It’s self-contained, which means that the model inside is packaged and deployed in a standalone, executable form. This is an important aspect, because an xDT is distributed as a virtual extension of the physical asset, and therefore it should be useable not just by simulation engineers but by any user of the system.
- It can run anywhere, for example in the cloud and on PLC Edge devices, but also on laptops or PC’s like traditional digital twins
- It runs in real-time, or at least in sync with the physical asset it’s connected to.
An xDT brings value to many use cases along the product lifecycle, whether it’s in-design, in-production, in-operation or in-service.
The use case described here is a water distribution network in operation, where the challenge of finding the right balance between energy reduction versus ensuring reliable water supply is being addressed with an xDT. This approach shifts the efficiency of the system to the next level, where ensuring sufficient supply can take energy prices, reservoir levels, pump efficiencies and weather forecasts into account.
If we look at where the energy is consumed, research from McKinsey shows that industrial processes account for 42 percent of power consumption in the European Union, and pumps consume the highest share of energy at 30 percent. Hence, being able to reduce energy consumption will have a direct and heavy impact on operational costs.
To visualize this, the British Hydro Research Association once stated that:
“The plant had installed 50MW worth of cooling water pump power when 30MW was more than sufficient. This would be around £3,400 per hour in excess electricity just to move too much cooling water around the plant”
D.S. Miller, British Hydro Research Association
In our case, the system of a water distribution network contains 31 pumping stations, made up of 84 pumps which transport the water between around 20 reservoirs in the area. Depending on expected demand for the next 24 hours, weather forecasts, energy prices and reservoir levels the stations decide to start or stop pumping water using forecasts and operator experiences.
The system is mostly managed by individual operators at each pumping station using historic data and experience, not as a whole system resulting in pumps often being enabled in a reactive manner at full speed to meet spikes in demand. This leads to both over-filling of specific reservoirs, and the system balance being upset further leading to responses from other pumping stations to compensate. This is an intrinsically unstable system.
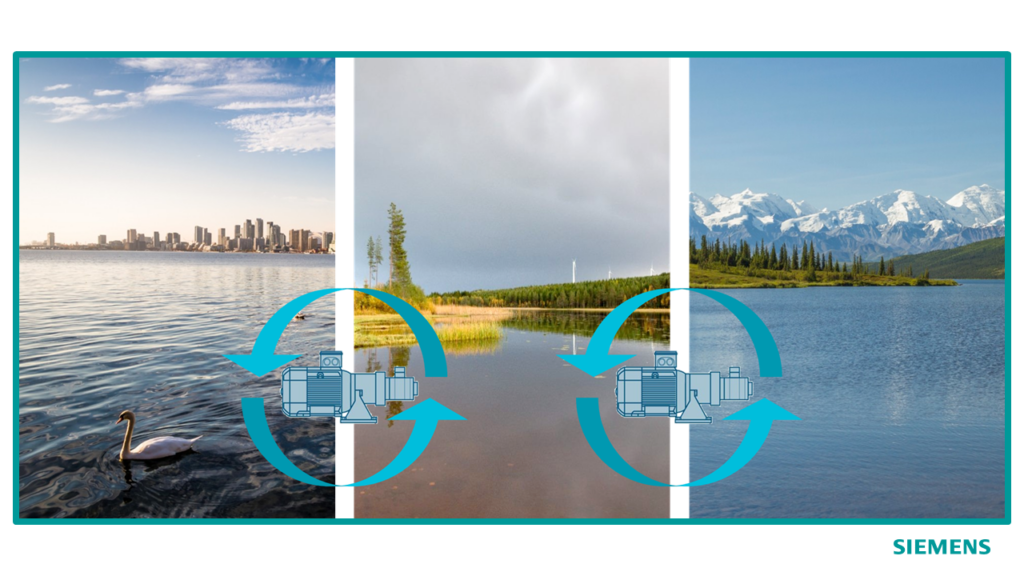
The role of the xDT in a water distribution system
With the use of an xDT, we want to drastically improve the efficiency of this system. It helps us to better operate the system as a whole and take more process parameters into account to make better decisions during operation.
There are several ingredients that go into building the xDT:
- The data from the real asset, in this case including sensor data from the flow meters and rpm sensors of the pumps.
- The virtual, physics-based model of the water distribution network will be used to predict the behavior of the system. In this case we created a full-fidelity, real-time capable model built in Simcenter Flomaster, which is ideally suited for simulation of large thermo-fluid systems.
- HEEDS, connected to the Simcenter Flomaster model. This is a powerful design space exploration and optimization tool, automating the optimization process to quickly evaluate performance trade-offs.
- The Programmable Logic Controller controls the actuation of the pumps. This includes a human-machine interface (HMI) well-known to the operators to control the pump station and interact with the xDT.
- Siemens Insights Hub is the industrial IoT platform, capable of storing data and interfacing with data, the model and the physical pumping station.
These different components are connected according to the following flowchart:
Benefits and conclusion
Now that we’ve set up the system and made the interfaces, we can have a look at the information it gives us and what difference it makes. Roughly said, there are 4 main benefits that are delivered by the xDT:
- We can better tailor the pumping scenarios for the entire system. This will avoid over-filling and over-pumping which led to cash losses in the past.
- The xDT becomes the centralized, data-driven management system that is repeatable and allows to collect and save knowledge and experience. This will improve predictability and operation efficiency compared to the past which relied only on operator experience and forecasting.
- We can better understand how the different parts of the system interact with each other, leading to more stability and demand matching in the full system. With this, we now avoid single stations upsetting the balance and response of the system.
- With the improved predictability of the system, we can now operate the pumps at their best efficiency points, instead of having to operate at full speed to meet the required demand.
Ultimately these benefits translated into double-digit savings on energy consumption, which is a yearly saving of a ton of money. Next to that, it turned out to be a powerful tool to improve operational understanding, control, forecasting and what-if scenario testing.
More xDT
The executable digital twin is an emerging technology with an unlimited number of use cases. It leverages the power of combining several essential elements in the product lifecycle. Siemens is uniquely positioned by offering all ingredients to be able to connect the real world with the virtual world, ranging from physics-based CAE software, an industrial IoT platform, industrial automation controllers or PLC’s and if required Engineering and Consultancy Services to support the deployment.