A physical or machine learning catalyst model? Battle in Simcenter Amesim
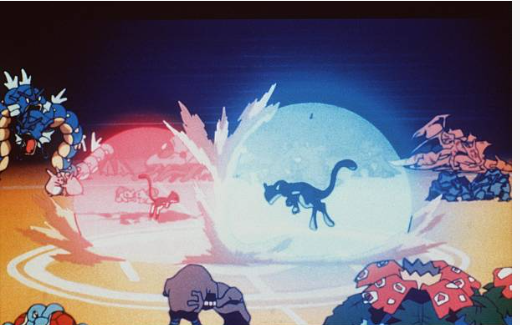
Do you know Simcenter Amesim Machine Learning catalyst model? Humm what is THAT? Let’s have a look:
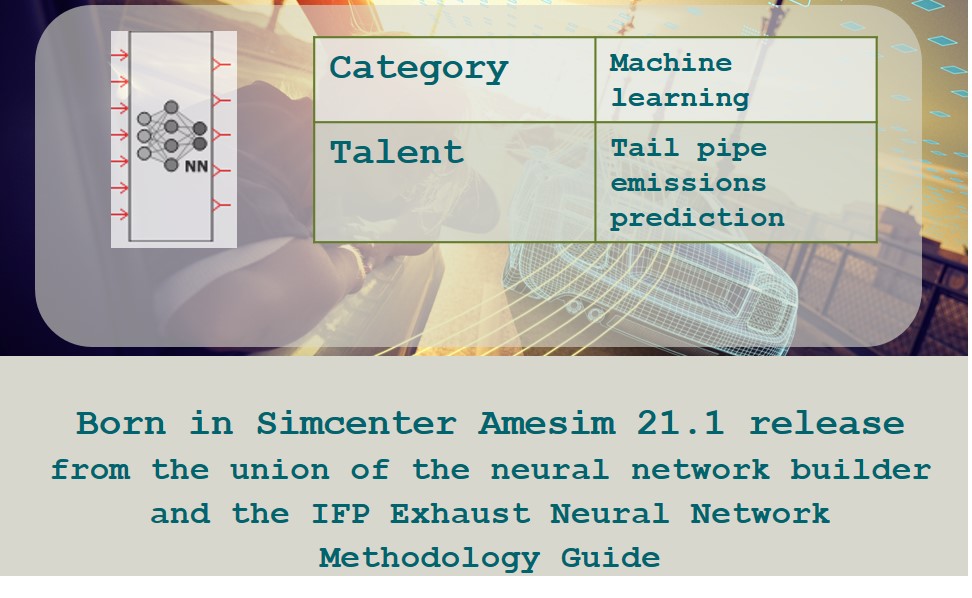
Tail- pipe emissions prediction is a useful talent to simulate vehicle exhaust emissions in real conditions. But… wait a second! There is already a catalyst model in Simcenter Amesim with the same talent, right? Let’s check…
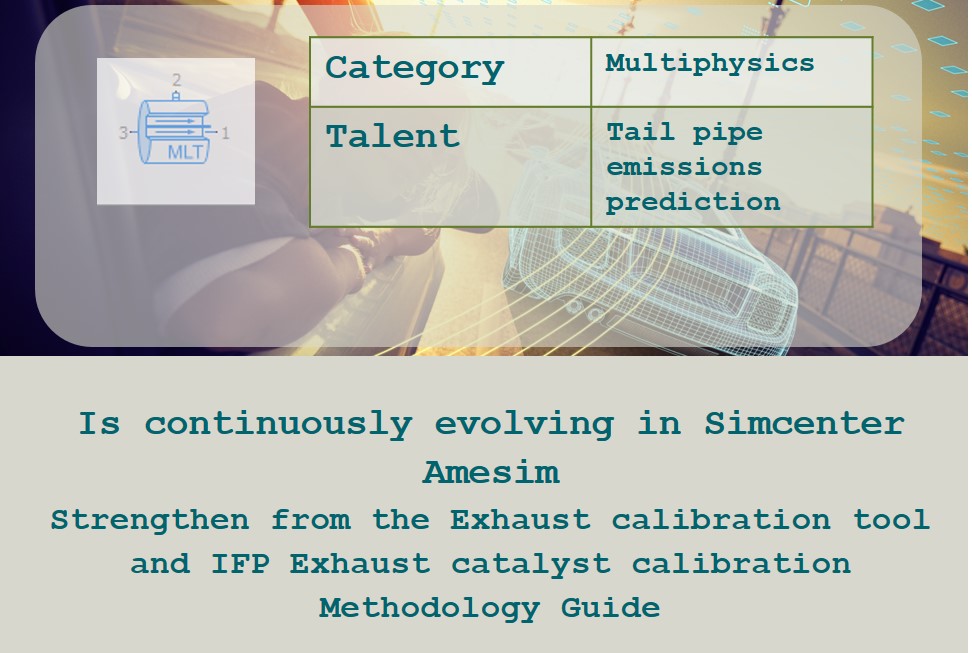
As far as Simcenter Amesim offers two catalyst challengers, how can model trainers choose the right one to serve their vehicle emissions simulation needs?
Let’s engage a battle between these two catalyst models and see which one is the strongest. May the best one win!
Round 1 – Computation time
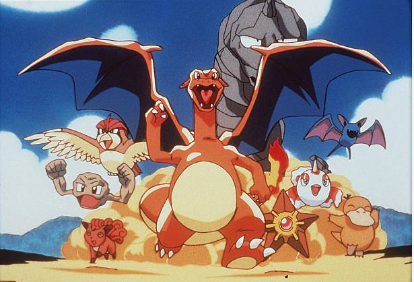
Simcenter Amesim physical catalyst model fully fits with system simulation and control development requirements. Indeed, it presents fast computation times and compatibility with fixed time step solvers. For instance, within the Subaru application case, presented at the 2020 CLEERS virtual workshop, the model rans 11 times faster than real time. But its rival, the Machine learning catalyst model demonstrated even faster simulation times! How is this possible?
Machine learning catalyst model doesn’t embed the same complexity than the physical one. Capturing the physics and thermal gradients implies a discretization of the monolith and the handling of species and chemical reactions. Hence, physical model includes much more explicit variables to solve; another argument in favor of the Machine learning catalyst model which wins on this specific point.
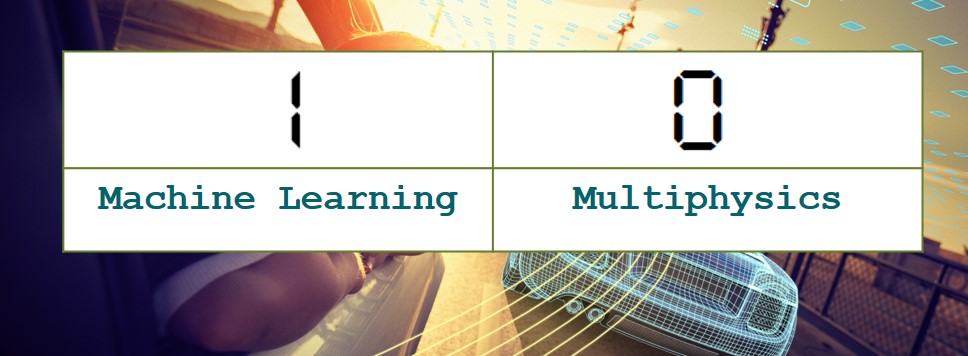
Round 2 – Data for model training/calibration
Machine learning catalyst model requires intensive training in order to deliver its full tail pipe emissions prediction talent. This training can be intuitively performed using Simcenter Amesim Neural Netwok builder interface.
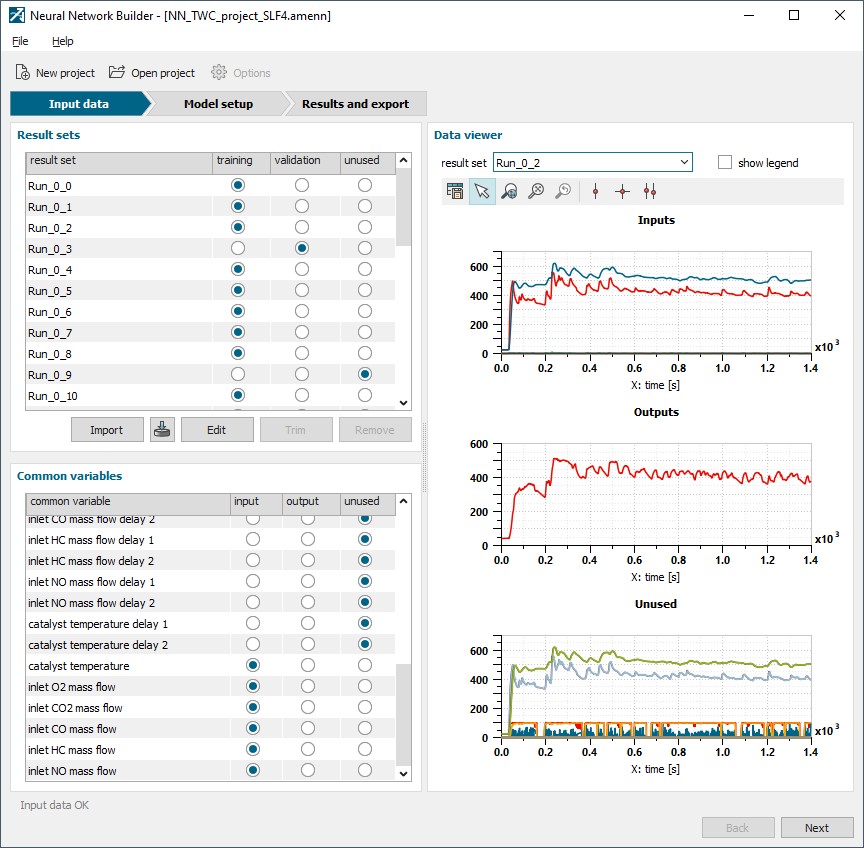
The more the Machine Learning catalyst model learns from training data, the best and brilliant is its emissions prediction power. The main drawback is evident: if only few test data are available the prediction level will be poor.
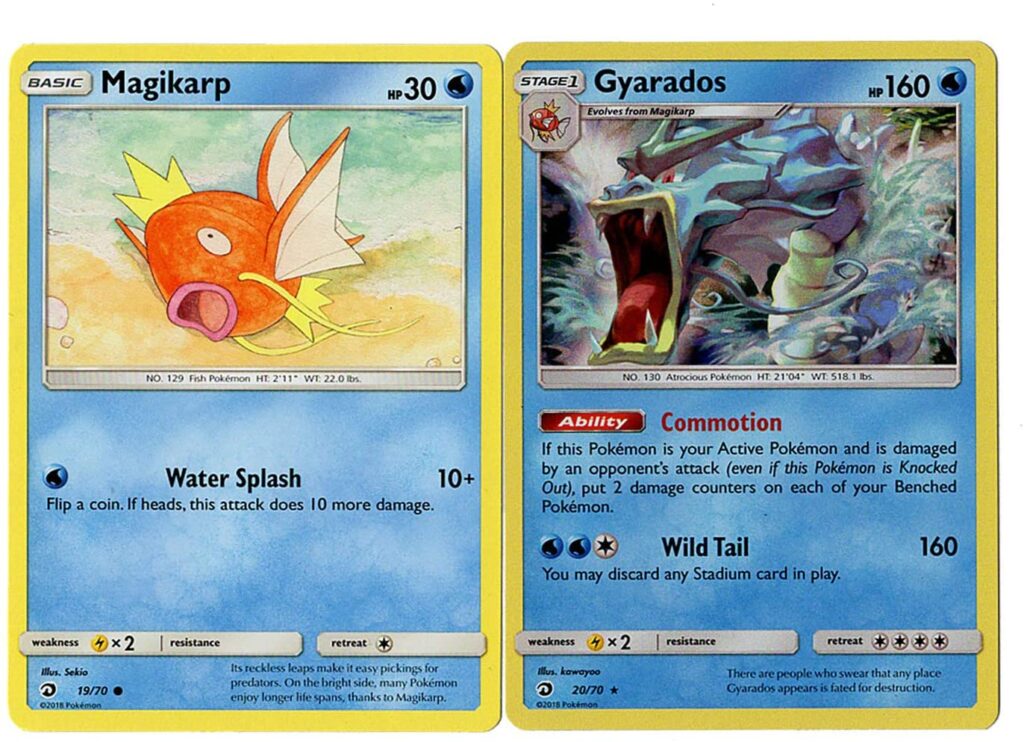
Machine learning model needs training data to reveal its real talent!
On the other hand, the physical catalyst model also requires some training using test data; but not for the same reasons. Indeed, Machine learning model is trained to mathematically predict the whole catalyst behavior, while physical catalyst model is represented by physical and chemical equations where only some specific parameters require a tuning (training) to adapt the model to the studied application case.
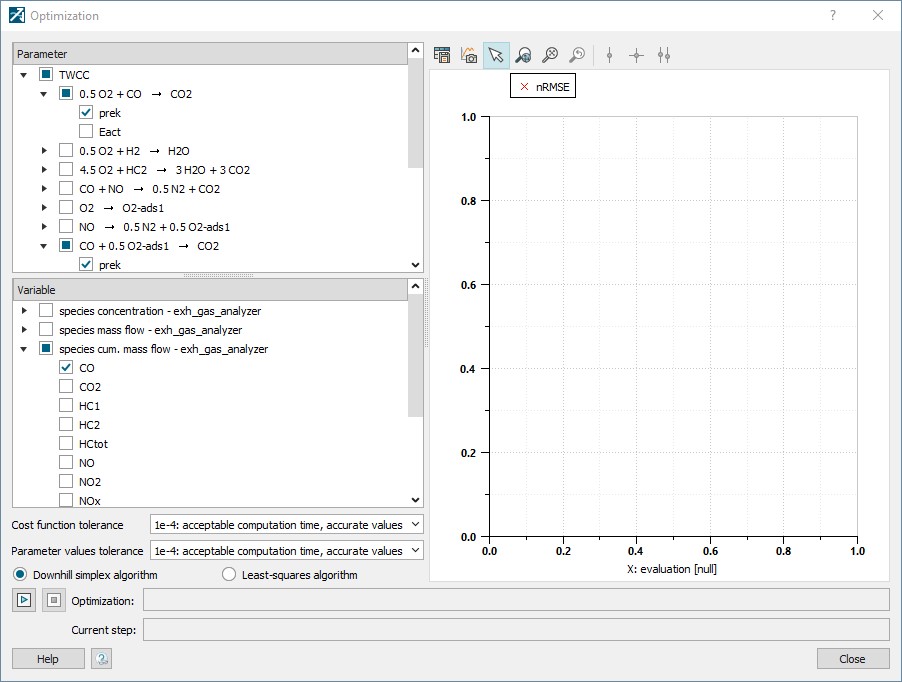
A conclusion? If only few test data are available for model calibration, the physical catalyst model is more suitable for tail pipe emissions prediction and wins against the Machine Learning catalyst model.
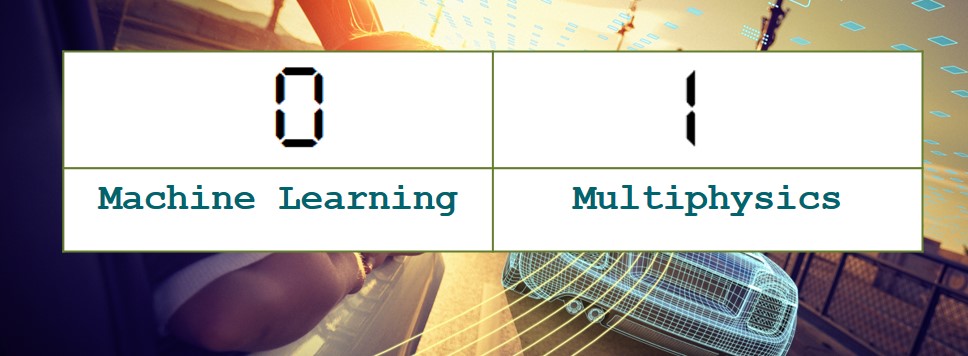
Round 3 – Model extrapolation capacity
The Multiphysics nature of the Physical catalyst model makes it robust to changes in the initial and boundary conditions which is not offered by the Machine Learning model. You can see the impact of changing the external heat exchange of the monolith for instance or even see the influence of geometrical parameters like the component size! Of course, it is also possible to change some parameters in the Machine learning catalyst model like inlet gas composition or inlet temperature within the limit of data used for the training stage. Nevertheless, physical model is more suitable than Machine learning model when used outside calibration/training range thanks to its physical content. Here there is a clear winner!
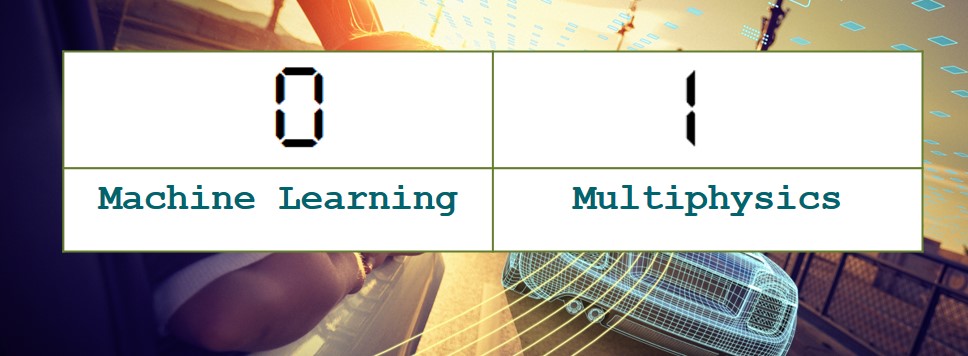
Take care! Machine learning model has not yet said its last word… it will continue growing!
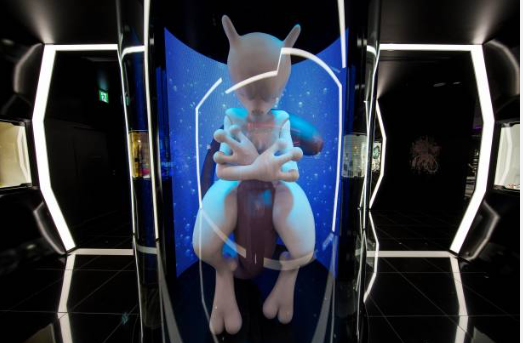
Round 4 – Model trainer* skills
*Model user
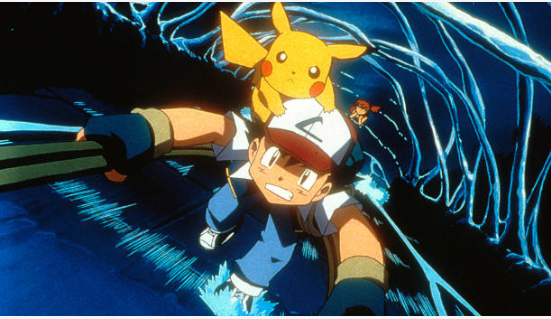
Simcenter Amesim offers the exhaust calibration tool and calibration methodology guide to ease physical catalyst model development. These solutions are described in previous blogs about catalyst reaction model development workflow and whether only experts in chemistry can develop a catalyst model.
No need to be a chemical expert to successfully develop a physical catalyst model in Simcenter Amesim! just some knowledge detailed in the catalyst model calibration documents are enough to follow the model development workflow.
On the other hand, Machine learning catalyst model doesn’t require any knowledge about chemistry… At all! Even a Machine learning expertise is not required thanks to the easy-to-use and intuitive Simcenter Amesim Neural Network Builder interface.
It’s a model accessible to all!
You just must take care about the consistency of the test data you use to feed the workflow. Bad data lead to… imagine what? Bad results.
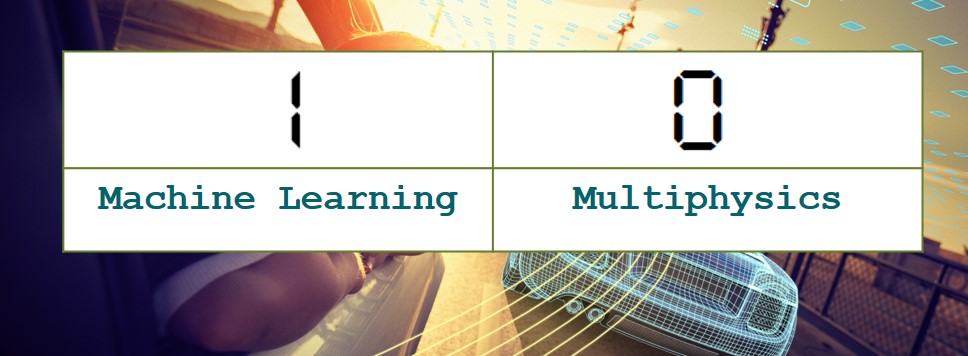
What if we stop this battle here?
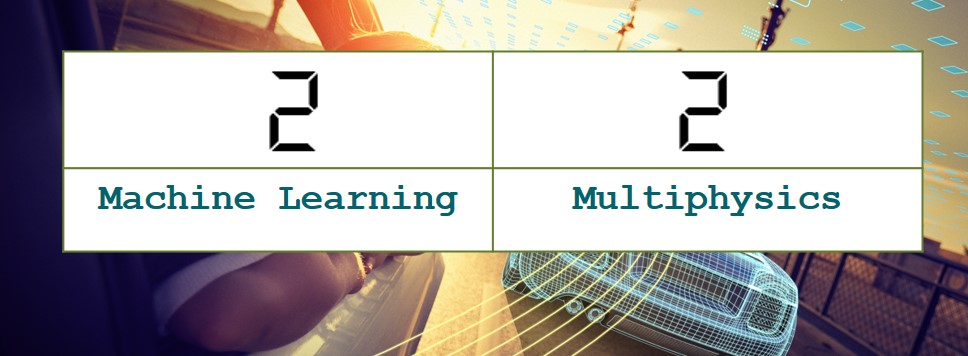
Okay, neither of the challenger wins the fight. Each model has some key strengths and limitations and you finally need to choose Physical or Machine Learning catalyst model according to your use case.
Beyond this trivial debate, it’s possible to make strength through unity, right?

About the author:
Sana Loussaief, Product Manager for Simcenter Amesim Internal Combustion Engine