The Evolution of the Digital Twin in Industry with Tom Phillips of Siemens
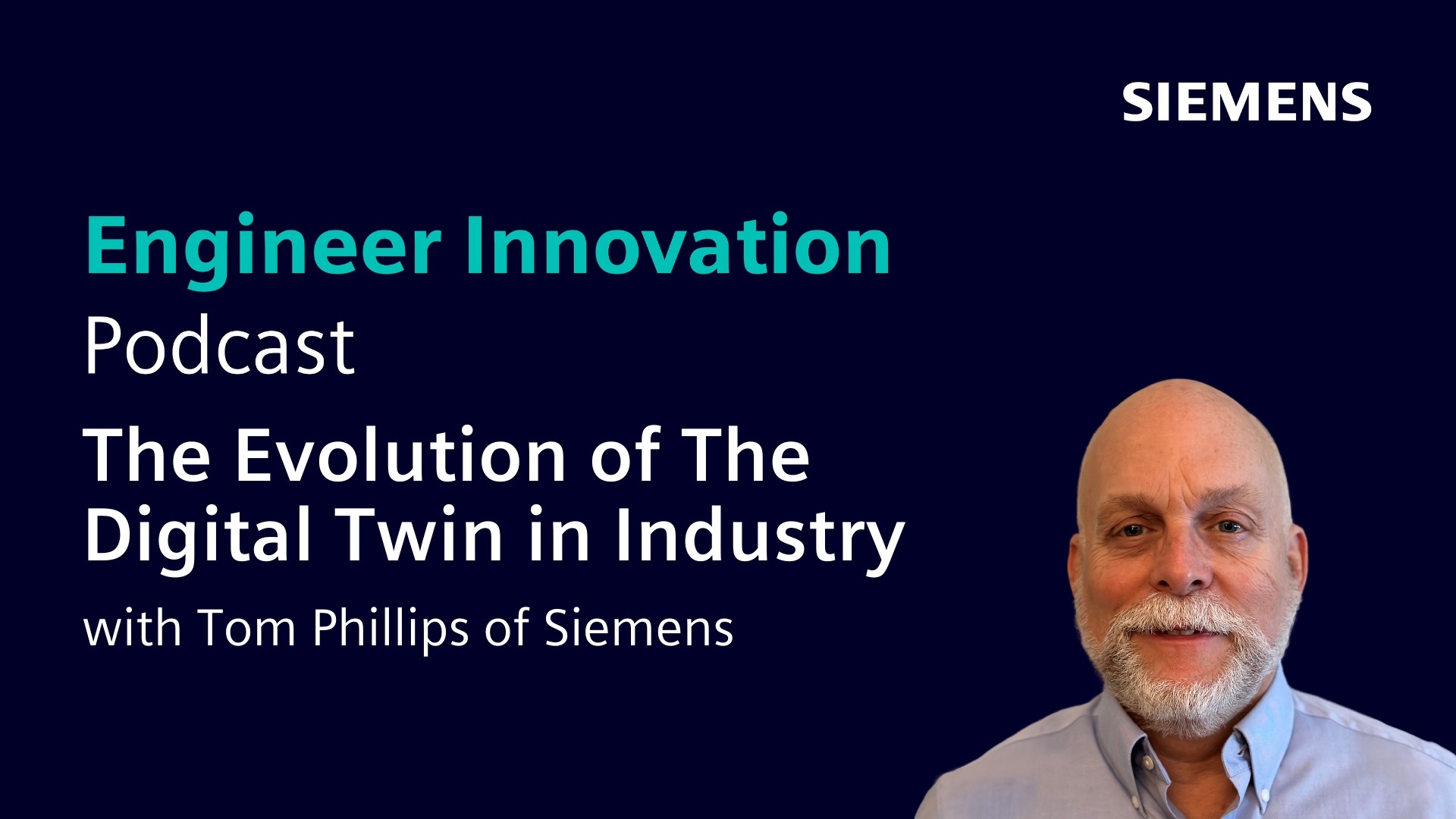
Listen to the Engineer Innovation podcast on
On today’s episode, we’re joined by Tom Phillips, Director, Simulation Portfolio Development of Siemens Digital Industries Software, who sheds light on the advanced applications and benefits of the digital twin in the industrial world. His insights are focused on the practical aspects and the future of digital twin technology in enhancing industrial operations.
Key Takeaways:
- How the digital twin enhances product and process understanding.
- The significance of the digital twin in predictive maintenance and condition monitoring.
- The role of the digital twin in improving manufacturing and operational efficiency.
- The role of simulation and analytics in refining the capabilities of the digital twin.
- Advice for beginners on starting the digital twin in operations, focusing on design phase and predictive modelling.
- Evolving from basic digital twin creation to automating models for enhanced usability across applications.
- The concept of reduced order models and executable digital twin for real-time applications in monitoring and maintenance.
This episode of the Engineer Innovation podcast is brought to you by Siemens Digital Industries Software — bringing electronics, engineering and manufacturing together to build a better digital future.
If you enjoyed this episode, please leave a 5-star review to help get the word out about the show.
For more unique insights on all kinds of cutting-edge topics, tune in to siemens.com/simcenter-podcast.
- How the digital twin enhances product and process understanding.
- The significance of the digital twin in predictive maintenance and condition monitoring.
- The role of the digital twin in improving manufacturing and operational efficiency.
- The role of simulation and analytics in refining the capabilities of the digital twin.
- Advice for beginners on starting the digital twin in operations, focusing on design phase and predictive modelling.
- Evolving from basic digital twin creation to automating models for enhanced usability across applications.
- The concept of reduced order models and executable digital twin for real-time applications in monitoring and maintenance.
Tom Phillips:
Augmented reality, virtual reality, all of the AI tools and machine learning, the compute power, all that data you mentioned being streamed out, boy, it’s going to be really exciting to see the directions that we take with that industrial metaverse and what it really opens up for possibilities.
Stephen Ferguson:
My name is Stephen Ferguson, and you are listening to the Engineer Innovation podcast. So, in today’s episode we’re talking about digital twin and, specifically, the executable digital twin. So the term digital twin was first coined in 2010, but I never heard it used in anger until about 2016 when I thought, “Hey, that’s a really cool idea. I wonder when it will actually happen.” And then by 2018, everyone was talking about digital twin. I remember hearing the word thrown about on mainstream TV and on the radio.
2018 was also the year that Gartner put the digital twin at the peak of inflated expectation on its hype curve, predicting the digital twin would reach the plateau of productivity, as they call it, in five to 10 years, all of which means that in 2023, we’d expect to be seeing the first credible examples of digital twins being spotted in the wild. My guest on this episode is executable digital twin evangelist, Tom Phillips, and together we conduct a health check on the current status of a digital twin, discussing what’s been achieved so far, what the obstacles are to further progress and where the digital twin is heading next. I hope you enjoy listening to this episode of the Engineer Innovation Podcast, and don’t forget to follow or subscribe. How are you doing, Tom?
Tom Phillips:
I’m doing great, Stephen. How are you?
Stephen Ferguson:
Good, thank you. So we’re going to talk today, I think largely about digital twin, and there’s been a lot of talk about digital twin. So back in 2018, the Gartner hype cycle put the digital twin at the peak of inflated expectations, which is a state of maximum hype, and after that new technology, dives into something they call the trough of disillusionment where interest wanes as early adopters failed to realise the promised potential technology, but hopefully the technology emerges into a plateau of productivity, which is mainstream adoption and everybody’s starting to use it productively. Where do you think we are on that journey? Are we still in the trough of disillusionment or are we starting to emerge into the plateau of productivity with digital twins?
Tom Phillips:
That’s a really great question, actually. And if I back up a little bit, right when the digital talk of the digital twin first started emerging, everybody was talking about the digital twin, but there was not a uniform definition of what it really is. So, if you were an FEA company, digital twin magical became an FEA model. If you were a CAD company, it became a CAD model. And the result was that a lot of these companies approached customers, and they tried to implement this in a digital transformation strategy, but it was all not connected and not well put together, and so, I think it led to a mismatch of expectations, where they weren’t getting the benefit out of the digital twin that they needed to. And so, this is where I really like the Siemens approach, though, because we take the view that the digital twin is not just an FEA model, it’s not just a CAD model.
It’s a comprehensive set of models that capture all the information related to a product or a process throughout the lifecycle. So it stems from design into production to out in field use, and so, we’re able to coordinate all that information and really allow people to get that business benefit out of it. Are we in the trough? There’s still a lot of expectations out there, but I think people are beginning to see that it’s not about a disconnected set of models and information. It’s about integrating that whole data stream and implementing it. And the other thing that leads to maximising the benefit of the digital twin is, it’s not just about the model. You’ve got to structure your organisation and have processes internally that allow collaboration between different groups and different functions. And I think, in the industry now, there’s a wide realisation that is the way it needs to be implemented. So, I do think we’re coming back out of it, and I think I’m biassed obviously, but I think Siemens has a very good approach.
Stephen Ferguson:
Yes. Actually, Gartner predicted in 2018 that the technology was going to start to come to maturity in five years’ time, and we’re at 2023 and we’re at the beginning of that timescale, aren’t we? And we’re starting to see some real-life examples of digital twins. Could you kick us off with, do you have any examples of digital twins that you could use to explain to listeners actually what digital twin is and what it does and what sort of benefits it’s showing for the engineering community?
Tom Phillips:
Maybe I can take you back on a history lesson from my career a little bit. I’m going to focus on the simulation end of the world. By that what I mean is we make a virtual representation of a product or a process that’s physics based. So now I can do simulations that predict the performance of how that product is going to behave or how that process proceeds. And so, if I take you back, maybe I date myself a little bit too much here, but back in the ’80s, new PhD coming out of school, hired into a gas turbine manufacturer, and I was working on heat transfer with commercial engines at the time. And the director that hired me said, “Tom, I’ve got a new initiative that we’re doing. It’s going to be called physics-based heat transfer.” And I remember thinking, “Good God, what did they do before? Did they consult the Psychic Friends hotline or what did they do?”
But it ends up, it wasn’t predictive at all. What they would do is they would take test data, and they would tweak all the knobs, and they would match that data to the point where even a thermocouple that was bad that had pulled off and wasn’t measuring metals, measuring air temperature, they’d blindly go ahead and match that, and the result was they had a really bad reputation. They were not able to be predictive at all. And so, things have progressed, right? You got not only methodology. So, we were able to go back and implement a methodology, where we used textbook heat transfer correlations to actually make that predictive, and it was a physics-based heat transfer model in the end, and we got a good reputation as a result of that over time.
And I think there’s all kinds of examples out there. The automotive world and the aerospace world depend on virtual mod. And I think we’ve come so far now that most of the industry, there may be some laggards out there that aren’t doing any kind of simulation of modelling at all, but those are starting to be few and far between. I think most people are making good use. So, it’s everything from medical devices where we’re able to model the operation of an infusion pump, say for an ambulatory pump to provide insulin for patients, and that kind of thing. We’re able to go through and help model the energy consumption, the volume of liquid that gets pumped and delivered to a patient. There’s so many examples, Stephen. I think we’ve really come a long way over time, and there’s not too many people, I don’t think. Maybe I’m wrong, but I think most people buy that you can model a device or a process and get good results.
Stephen Ferguson:
So that’s interesting. So you started in 1980s, and so I guess, you are familiar with punch cards and those kinds of things because I know almost like a generation later, I left university in ’93 and went straight into industry. And by the time you were talking about you were using test data to almost tune and tweak and fiddle your simulation results. But actually, 10 years later, when I came in industry, we were starting. We were the first people to start to use simulation data and simulation results to guide design processes to start to be taken seriously, maybe not in the early ’90s, but by 2000 people were starting to begin to believe in simulation.
So, most of my career, it’s all been about doing simulation, validating that simulation with some test data. But then those simulation models, once the products you were designing went into development, it almost went into use. You forget about it after that as well. So whereas you used simulation data in early days to tweak your models, we used it to validate our models, but now with the digital twin, we have this opportunity to have this continuous data stream of real-life usage data that we can use to improve our models and to improve our products during their lifetime. Is that one of the big payoffs of all of this?
Tom Phillips:
Oh, I think it absolutely is. And if you look at the way the technology has evolved way back when, compute power, there’s probably more in my phone right now than there was in the computers I used at the time. So the compute power has really improved, and Moore’s law, everybody’s familiar with that. The compute power just continues to improve and improve and improve. The result is we’re able to have models that have more fidelity. We’re able to include models that have more enhanced physics, more fuller range of a multi-physics approach. But then, also you look at other technologies, right? You’ve got AI and machine learning, you’ve got the cloud, there’s edge compute devices, there’s all these things. So, that now allows us to take this digital twin, and what you mentioned is very important, this validated digital twin. And now I can take and produce a reduced order model using maybe neural net technology or other, it doesn’t have to be AI-specific, but I can take and produce a reduced order model that runs real time.
So, now a second of simulation time is a second clock time, and now that enables me, and in addition, I can run that on the edge. So now I can hook that to a manufacturing device, and I can provide sensor input to that model, do a physics-based calculation, pass back that information back to a PLC or some other kind of device, and now I can interact physically with a device, and that we call an executable digital twin. But this technology evolution has really allowed us now to maximise the benefit of the digital twin overall. And when we come into that executable digital twin, I think it’s analogous to where we were back in the ’80s. People are now realising that, yes, you can model things real time. You can use that physics-based information together with AI, which ChatGPT has been all over the news, but we’re able to pass that information back to machines, and now we can minimise energy consumption. We can maximise quality. We can do all kinds of things. It’s open to the imagination right now.
Stephen Ferguson:
Yeah. So, when we first started this podcast, the big themes you wanted to tackle were digital twins, machine learning and AI, the metaverse, cloud computing. And we naively thought those are all separate topics, but almost every single conversation we have these days involves elements of some of those things or all of those things. So lots of those technologies really come together in a digital twin, I guess, don’t they?
Tom Phillips:
Yeah, they absolutely do. And it’s all evolving and it’s changing. As we get more compute power, we get more ability to run on the cloud, analyse data. It’s not just about that interaction real time either, we can take data and put it out on the cloud and train generative models or train AI algorithms to get better and better over time. We can do more in-depth simulations that maybe can’t run real time, or you don’t need to run real time, but provide information and close that loop and field usage back to the next-gen design. All of that technology comes together, and it really does enable a maximisation of that digital twin to impact business value.
Stephen Ferguson:
Yeah. So we talked about how some of the value of this is being able to measure the real-life operation of a product in the field, but what’s happened before is we’ve always been limited by a finite number of sensors. Sensors are expensive. Data transfer used to be expensive, and so, you can only put a certain number of sensors on the product, and you can’t always put those sensors where you want them to be as well, for various physics reasons or cost reasons or whatever. Does a digital twin give us more visibility on what’s actually happening in the product than just looking at your limited number of sensors?
Tom Phillips:
Yeah. And that’s a great point as well. So, there’s so many avenues of this, but what you’re talking about really falls under what we call virtual sensors. Again, I can take data, it might be limited, but I can take data from a device or from a product, feed it into a physics-based model, and then calculate all kinds of quantities. So as an example, you might measure screen at one location. When I’m able to feed that into a structural model and display, and even with augmented reality, it allows you real time. You can hold an iPad up and look at a structure as it’s operating and see a stress field superimposed on the object. And you can imagine the value that has to an engineer trying to develop a new product or somebody trying to troubleshoot a problem in the field. Now I’m able to take what was a limited set of data and just greatly expand it to give tremendous additional insight into what’s going on with the behaviour.
Stephen Ferguson:
And it also offers you some robustness against sensor failure because if you have one of your sensors fail, then you might be able to reconstruct data that you’re missing using digital twin. Or, for example, in terms of security as well, so cybersecurity, somebody could attack one of your sensors using a virus or something. Perhaps, you can use the digital twin to reconstruct that data or to tell you where your spurious data readings, which say that your satellite is falling out the sky, whether they’re real data readings or whether that they’re being as a result of, perhaps, some cyber attack or some sort of sensor failure. So there’s a whole data security element to this too, I think.
Tom Phillips:
Yeah. Like I said, the applications of this, I think are limited by the imagination right now. There is that redundancy on the safety features if you need that to be able to double-check that, “Hey, yeah, something’s going wrong.” You can also click that same thing, Stephen, and now it’s like condition monitoring. If I’m monitoring and I’m getting sensor input, but that greatly disagrees with what my model is predicting, chances are something’s not right with that operation. And so, now I can flag somebody right away through say a Mendix App, or I can get a message right to their phone that says, “Hey, something’s wrong. You need to go look at it.” So it goes into security, it goes into the operational effectiveness, or making sure you’ve got reliability and uptime maximised. There’s so many applications. This is fun right now being on this end of the curve.
Stephen Ferguson:
And it also doesn’t have to be you sitting at your desk waiting for a red light to go off. You can train AI and machine learning to spot when things are going wrong. And I guess, that’s also one of the other applications, isn’t it? It is early warning of when things might go wrong or might fail before they’ve actually failed.
Tom Phillips:
Yeah. And you could get the AI algorithm to even head it off. So, through a PLC, it might interact back with a manufacturing process so that it maximises the quality or avoids a quality issue coming off. One example of that, we had a food manufacturer that, I don’t think I’m allowed to say the name, but it was a popular snack, and that’s coming out of the oven, but you need the right density, you need the right oven temperature, you need the right feed rates in order to optimise the eating experience on the backend. So in order to optimise the quality of this snack food, we’re able to use this physics-based approach and together with other methods too. They’re also able to take camera images and with AI, together with physical-based models, look and maximise now that feed rate and the density, the temperature of the oven so that they maximise the quality of all the snack food production coming out. You can imagine how much waste they’re able to avoid and all that kind of thing. It’s pretty incredible.
Stephen Ferguson:
I think everybody who’s listening to the podcast right now is trying to guess which baked snack food you can’t talk about as well. So we’ll all have to have our own guesses. In the first episode of this season of the podcast, we talked to Ian McGann about massive engineering data analytics, which was about how you use all of this constant stream of data that you get from digital twins. So we’ve talked for years about big data. This is on a whole another scale, isn’t it? Because it’s constant, it’s real time. I think 80% of the data in the world is never processed, but we have to draw youthful conclusions and use that data to make decisions or make improvements. So how are we going to do that? Is that machine learning and AI again?
Tom Phillips:
I think it is, but there’s a lot of aspects to what you’re talking about there too. If we’re taking data on the edge and we’ve got models that interpret that data on the edge real time, and we’re able to make decisions, we may or may not store all of that data. We can be selective about what we store, so we maximise the value of what is storage, so that’s one aspect. But then we have whole infrastructures of things like our inside cub and that kind of thing that allow this data to come out and then selectively be used and analysed in different ways, and that can be a physics-based approach. So now, again, things that maybe don’t lend itself to a real-time approach, but I can go through and analyse that data very effectively and use it in a broader approach.
But I do think you’re right, the ability to produce reduced order models allow you to speed up going through large amounts of data that can be based on neural net approaches and allow you to cycle through a lot of data that you might not have been able to use. So, I think it goes two ways. It allows you to be selective about the data you do store, but then it just opens the avenue up for all kinds of simulations, not just physics-based kind of things, but maybe cost or quality studies, or all kinds of things that we just haven’t done before as an engineering community.
Stephen Ferguson:
Yeah. So that’s really interesting. And again, in another episode of this season of the podcast, we talked to Virginie Maillard about the industrial metaverse as well. At the moment, quite often, digital twins seem like an abstract concept, but the industrial metaverse is a way, I think you mentioned this earlier as well, for engineers and consumers and everyone to experience the living, breathing digital twins and see it and feel it and almost taste it themselves. Would you agree with that?
Tom Phillips:
I do agree with that. And I’m getting toward that long in the tooth end of my career, but I’m really anxious to see where all these technologies lead because I keep saying it, but the potential is really open. You just need somebody imaginative to come in and start making use of these technologies because we’re going to do it in new ways that people haven’t thought of before.
Stephen Ferguson:
So if anyone’s listening to this and they’d like to know how they can take the first steps towards the plateau of productivity for digital twins and start using digital twins in their day-to-day operations, how would you recommend they take those first steps?
Tom Phillips:
I like that question too because it’s an important one, and again, I’m coming at this from a simulation end of the world, so keep that in mind. But I think with the digital twin, typically, you would start in a design avenue. You’re going to make a model of something that’s predictive. It could be symptom simulation, it could be all kinds of different physics, but you’re going to make a representation and you’re going to validate it. And Siemens has the whole Simcenter Suite, it has the ability to construct that digital twin, those models, and then validate it with test equipment, test hardware that we have that interacts. But I think as customers evolve the use of digital twins, there’s a limited number of analysts that are out there, but a lot of problems that could benefit from a physical-based approach. You need the ability, then, to take those models and automate them.
And this is the journey because it’s all useful as you move through taking advantage of the digital twins. If I automate something, say for a non-expert, I can automate it with an analyst methodology in mind. So I can automate that expert method, but I can put a GUI on the front end that a designer or somebody, you just ask them questions about a device, and they can fill it in from a designer standpoint and then run a physics-based simulation and get a result, so that’s maybe a next evolution along the way. But then that opens up the automation is not just for a non-expert right now. I can automate with a tool like HEEDS, as an example, to do design space exploration. So now I can run hundreds of runs, maybe out on the cloud using distributed computing, whatever it is, but I can run that, gain tremendous insight about a design or performance as an expert, and that’s not limited to one stream.
I can couple in CAD together with stress, thermal and make that a logic base on a tree that gets automated and is incorporated into my design exploration, so now I can look at all kinds of trade-off. So you’ve got the digital twin that progresses into an automation that progresses into tools that can be used by both non-expert and expert alike. Now you’ve got the reduced order models, so now I take that same digital twin and I produce a reduced order model, maybe again from neural net technologies, but now I can run that real time and now I can make it interact with a device, that executable digital twin. So this same digital twin, I progress and evolve to the point where now I’m interacting with a device real time and an asset real time to control what’s going on or inform people.
It could be condition monitoring, predictive maintenance, maybe I keep track of the click, click stress that occurs on a device and I calculate cumulative fatigue damage. And when it gets to a point where, “Hey, we’re close to the fatigue life,” I notify somebody, so they can come out and replace a device, again, maybe on a manufacturing line and avoid downtime. I can now also look at operational efficiency, and that’s both the product and the manufacturing line. We’re just starting to really hit the benefit of that digital twin. So, I’m hoping that gets people out of the trough you were talking about and on their way to realising that peak of value.
Stephen Ferguson:
I think from talking to you today, we can finally start to see that plateau of productivity appearing on the horizon, and I’m sure this year and next year and the year after, we’re going to see more and more examples of this being used in real life, delivering lots of benefits and solving lots of problems that we’ve not been able to solve before. So I want to say thank you to you, Tom, for being an excellent guest, and thank you for everybody who listens to the Engineer Innovation podcast.
Tom Phillips:
Thanks for having me. It was a fun conversation.
Speaker 4:
This episode of the Engineer Innovation podcast is powered by Simcenter. Turn product complexity into a competitive advantage with Simcenter solutions that empower your engineering teams to push the boundaries, solve the toughest problems and bring innovations to market faster.
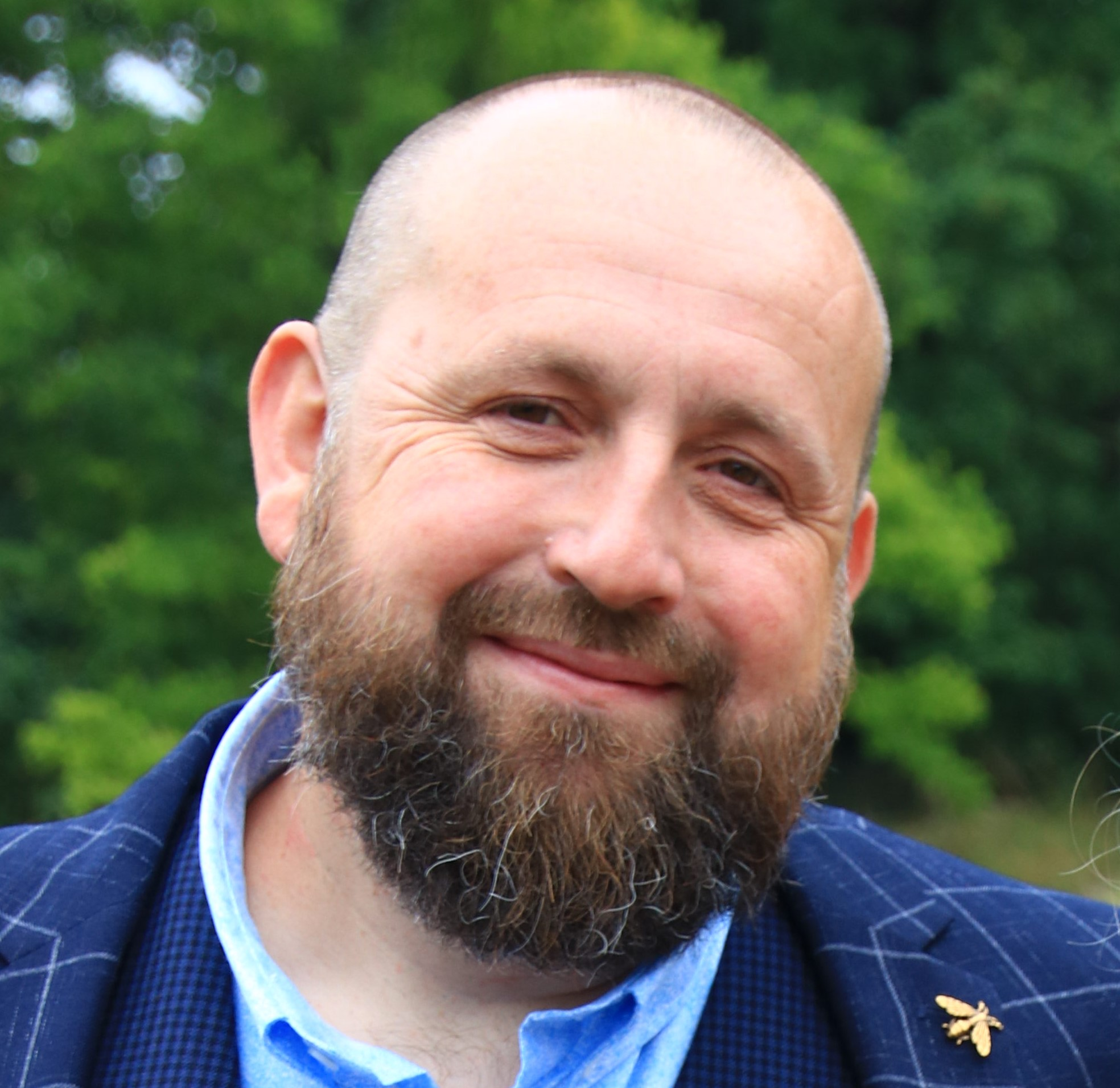
Stephen Ferguson – Host
Stephen Ferguson is a fluid-dynamicist with more than 30 years of experience in applying advanced simulation to the most challenging problems that engineering has to offer for companies such as WS Atkins, BMW and CD-adapco and Siemens.
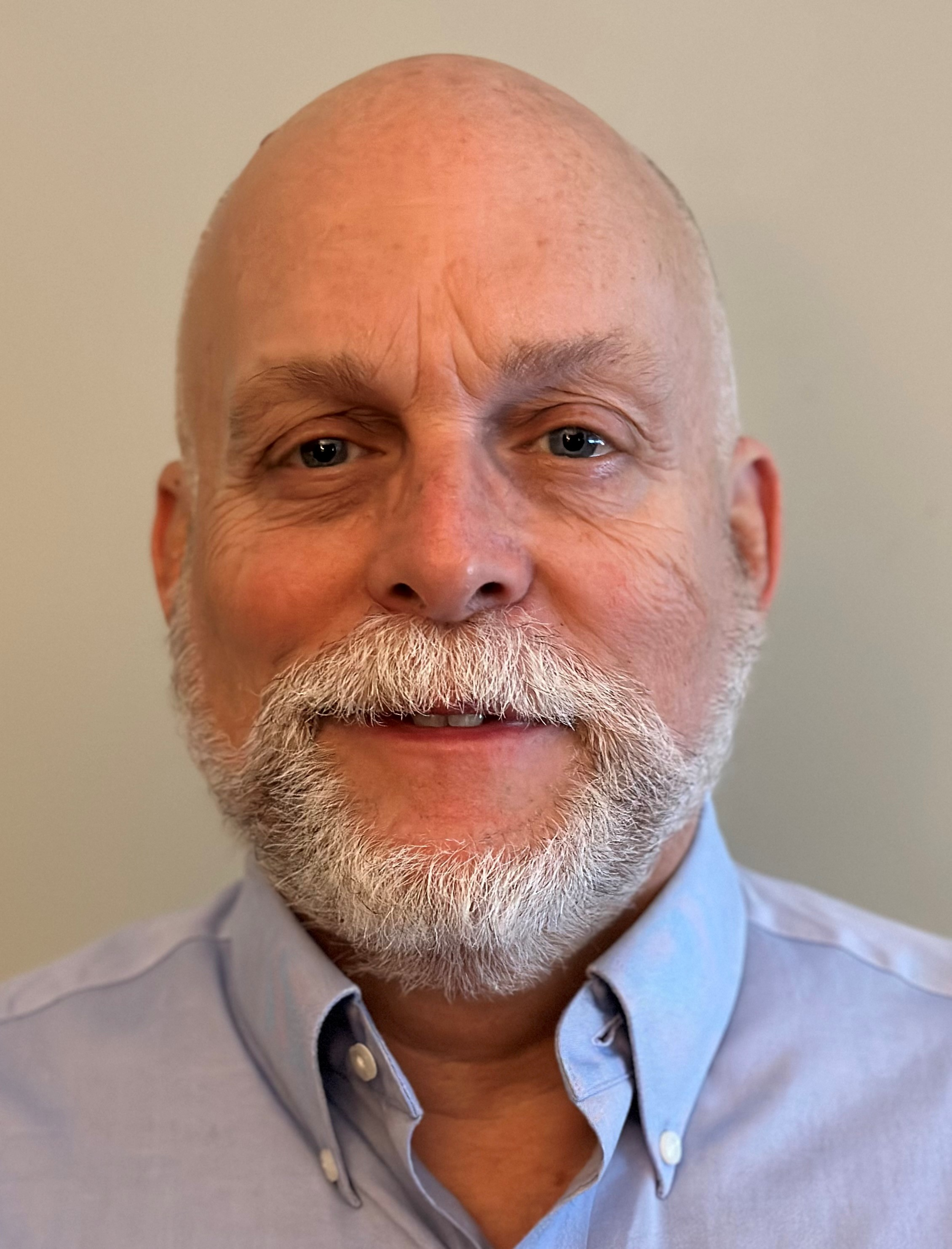
Tom Phillips
Tom Phillips with a Ph.D. in Mechanical Engineering has over 25 years of diverse industry experience, he has a demonstrated passion in helping companies improve engineering process. He especially enjoys helping companies proactively use simulation to innovate and develop products better, faster and cheaper with higher customer satisfaction.
Take a listen to a previous episode of the Engineer Innovation Podcast: Engineer Innovation: ChatGPT in the loop: Bridging humans to system simulations on Apple Podcasts
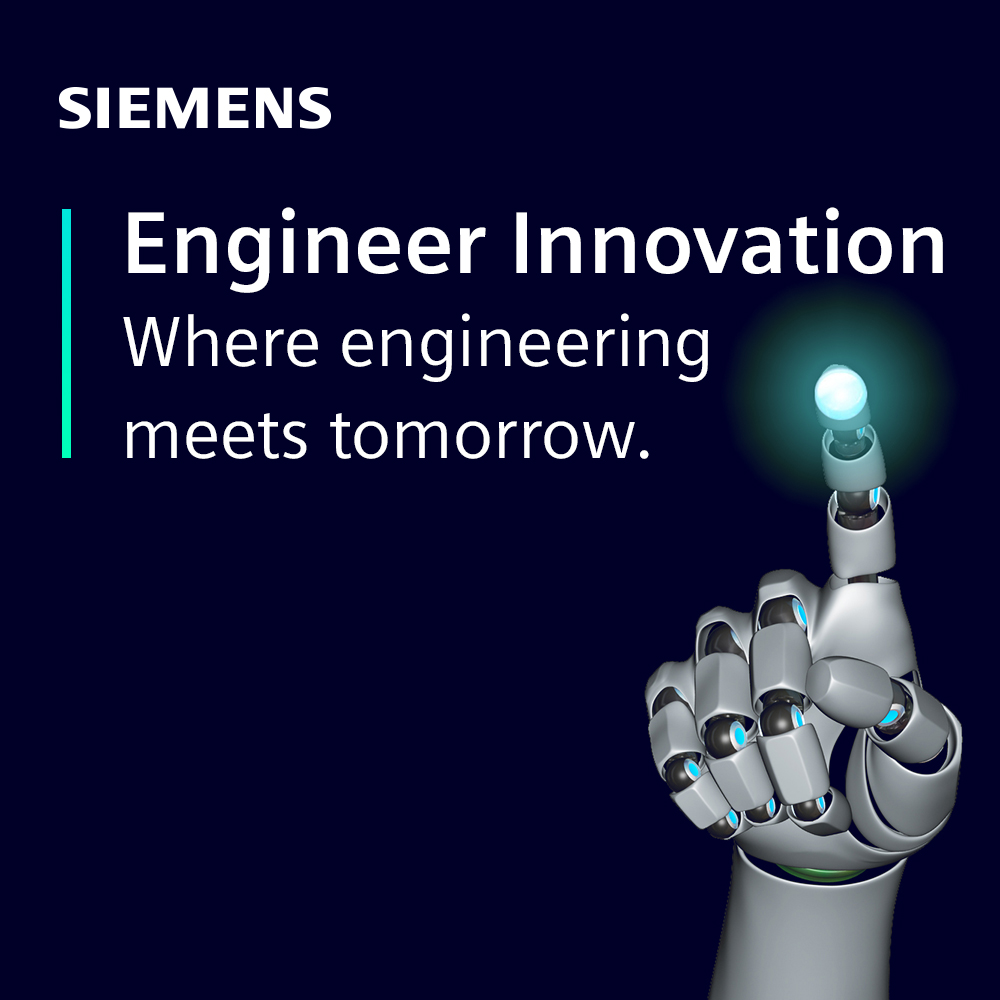
Engineer Innovation Podcast
A podcast series for engineers by engineers, Engineer Innovation focuses on how simulation and testing can help you drive innovation into your products and deliver the products of tomorrow, today.