Big pharma and the digital twin with John Perrigue (Season 2,Episode 6)
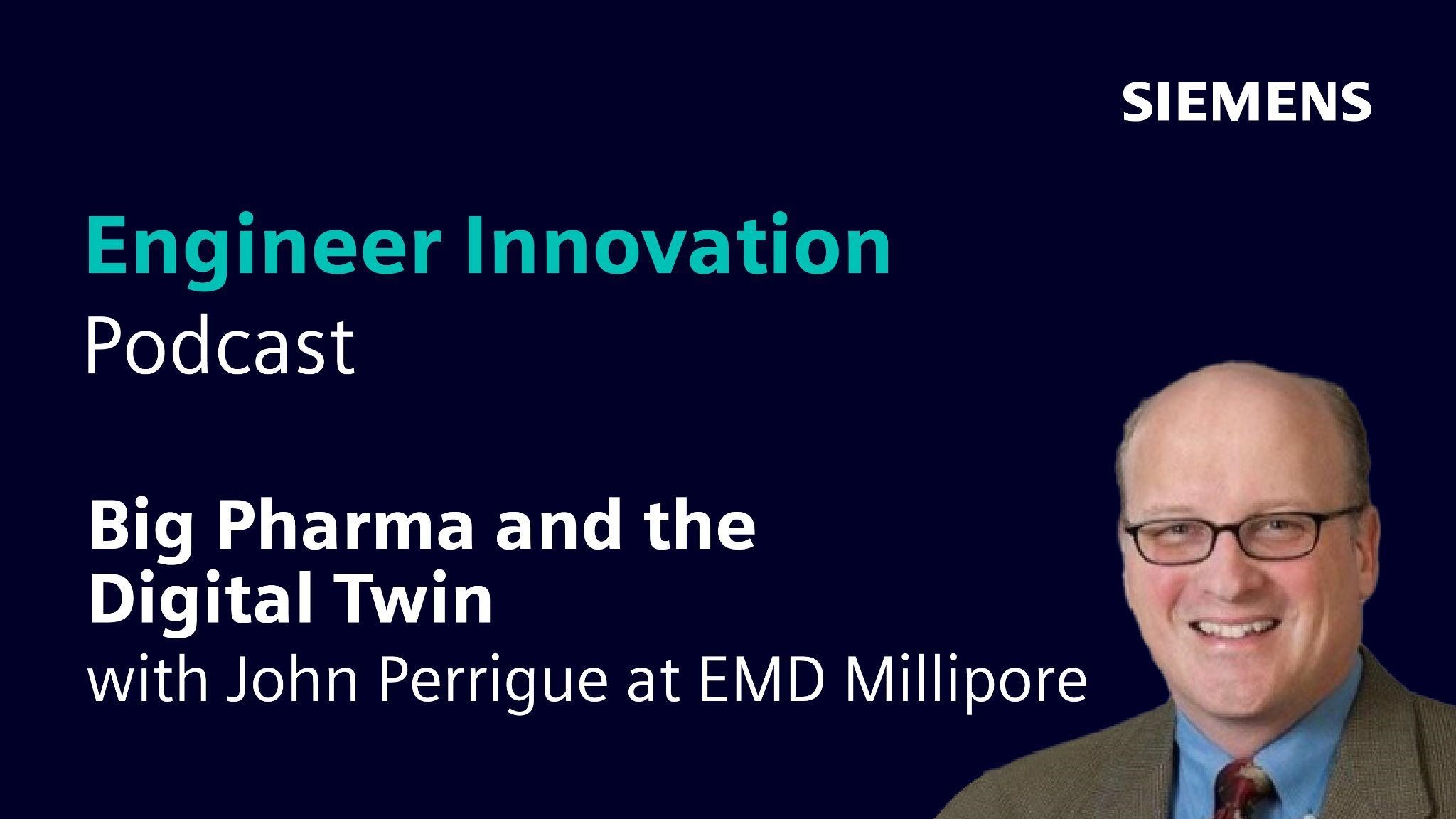
On today’s episode, we’re joined by John Perrigue, who is the Head of Digital Operations & Smart Manufacturing – Life Sciences at EMD Millipore and Senior Director at Johnson & Johnson, Digital Process Design. We talk about:
- How John first got involved with simulation and testing at Johnson & Johnson.
- How J&J uses digital twins and the benefits of this.
- How J&J’s simulation capacity grew and scaled over time.
- Tackling the human element involved in scaling.
- Do you need a Ph.D. to use Computational Fluid Dynamics (CFD)?
- Did Covid accelerate the use of simulation in the pharmaceutical industry?
- What is the endgame for automation optimization?
- The benefit to patients from this technology.
This episode of the Engineer Innovation podcast is brought to you by Siemens Digital Industries Software — bringing electronics, engineering and manufacturing together to build a better digital future.
If you enjoyed this episode, please leave a 5-star review to help get the word out about the show.
For more unique insights on all kinds of cutting-edge topics, tune into siemens.com/simcenter-podcast.
- How John first got involved with simulation and testing at Johnson & Johnson.
- How J&J uses digital twins and the benefits of this.
- How J&J’s simulation capacity grew and scaled over time.
- Tackling the human element involved in scaling.
- Do you need a Ph.D. to use Computational Fluid Dynamics (CFD)?
- Did Covid accelerate the use of simulation in the pharmaceutical industry?
- What is the endgame for automation optimization?
- The benefit to patients from this technology.
Thu, Jun 08, 2023 3:32PM • 27:45
SUMMARY KEYWORDS
simulation, cfd, process, siemens, twin, engineer, product, j&j, type, sense, talking, results, environment, digital, core, started, rescale, models, scale, manufacturing
SPEAKERS
Stephen Ferguson, John Perrigue
Stephen Ferguson 00:12
In today’s show, we’re talking about Big Pharma and Digital Twin, and exploring how engineering simulation and test are helping pharmaceutical companies to truly globalize production, bringing highly effective medicines to the communities that really need them at low cost. My guest today is John Perrigue, who was until recently Senior Director of Smart Factory and Digital Twin at one of the world’s largest pharmaceutical companies. How’re you doing today, John?
John Perrigue 00:35
Good, Stephen, how are you?
Stephen Ferguson 00:36
I’m doing really well. So I started off by using the words Big Pharma in the introduction, and I’m not sure that these days that sounds a bit pejorative because the words Big Pharma are often used in quite a negative sense, aren’t they? But the truth of it is, is the pharmaceutical industry really is a huge global business, isn’t it?
John Perrigue 00:54
It is, and I would say from the Johnson & Johnson perspective, it’s a multinational company and multi-product. So we’re medical device, various pharmaceuticals, because everybody thinks pharma in one sense, but it could be tablets in what they call small molecule or large molecule, vaccines, many, many things so you can subdivide pharma out. And the one interesting part about Johnson & Johnson till recently, the announcement is, with the divestiture of Kenvue or the consumer business, we are also working with a lot in the sense of our Consumer Products Division and Digital Twin as well. So it was a very interesting time at J&J because you saw a lot of things and a lot of different technologies and a lot of different needs for Digital Twin.
Stephen Ferguson 01:32
So when did you get, first get involved with kind of simulation and test at J&J, then?
John Perrigue 01:37
I was brought in, in roughly about 2014, to start looking at standardization around how manufacturing would work, primarily in our pharmaceutical consumer businesses and liquids and semi-solids manufacturing.
Stephen Ferguson 01:49
Just for the listeners, could you explain what semi-solids are?
John Perrigue 01:51
Semi-solids, that would be creams, ointments, gels, things that are highly viscous materials in viscosity, but not pourable, in many, many sense. But part of the overall types of products that people use every day. Standardization, though, however, was very challenging. Because when we looked at J&J, it’s multiple sites, multiple acquisitions, different products, and different equipment. And the struggle was, is how do you write a standardized procedure practice and best practices for sites to operate under, when you’re dealing with different scales and different types of motors, agitators, means of homogenization and so forth. So we stopped, and we rethought our whole position and said, “We need to think differently.” So we started to evaluate the use of Digital Twin and in particular, now, using computational fluid dynamics, discrete element modeling, and various other means to be able to translate a larger vessel at one site to a smaller vessel at another site or from lab scale to manufacturing production scale,
Stephen Ferguson 02:52
And to ensure consistency of quality of products, wherever it’s produced anywhere in the world. So the customers are always, or your consumers are always getting exactly the same product.
John Perrigue 03:01
Exactly. And also being able to deliver it quicker, too, right? When you think about how we could translate is, do we have to go through all the same procedural steps that we used to before in the sense of developing a, what they call a manufacturing batch record, or procedure or work instruction type of operation? So we could use that to predict from one location to the next location based upon the physical properties of the material, and that process stage with different types of equipment, different types of speeds, different types of shapes of the equipment as well, in the sense of impellers, or mixing type of equipment.
Stephen Ferguson 03:35
Back in 2015, when you first started on this journey, what was your simulation capability like at J&J?
John Perrigue 03:41
Very beginnings in this type of physics-based modeling, it was myself and one engineer that we started with, to do an evaluation to see proof of principles: would it work? Our first challenge is not necessarily the software, the software has been around for some time, it was really around the availability of, we’ll call the infrastructure, the IT components in the sense of access to digital core, computer core and core types, as well as the system and infrastructure as well that support it. We made a decision to go to an off-prem or an off-premises type cloud environment, to allow us that flexibility to look at different suppliers, different vendors of core and to be able to host our software from Siemens on that off-prem environment.
Stephen Ferguson 04:24
Who’d you end up with, if you’re allowed to say that?
John Perrigue 04:26
Sure, it’s a partner that has done a lot of work with Siemens and others, it’s a company called Rescale, based in San Francisco, California.
Stephen Ferguson 04:34
Right from the off, then, you are not using local workstations, but you are going and doing your simulation on the cloud, which I guess is a big part of the journey that you then subsequently went on?
John Perrigue 04:43
Yes, the thing about using the cloud is you could do a lot of your pre-work, what we call pre-processing and post-processing, on a standard local machine. So we set up that capability to do all of our beginnings of modeling and establish what we required in the sense of geometry, materials and process steps and perameters. And then we can also look at the size of the mesh, the number of cores that we’re looking at. And then we would basically then spin up a virtual desktop on Rescale, using a Linux server, Linux attributes, and we would build the virtual machine, move our pre-processing files up to the cloud, and then establish the number of cores and types of cores that we needed, and the associated runtimes that we’re looking for, to get the results. And then we would download them back and then do our post-processing for results and study the outcomes of the model.
Stephen Ferguson 05:30
So you started in 2015, how did your use of simulation scale over the next few years?
John Perrigue 05:35
That’s a great question. In 2015, we started with maybe two or three projects, proof of principle, because the thing about industry — and I think pharma somewhat lags our partners in aerospace, as well as in automotive, in some cases petrochemical — is breaking that standard ways of thinking, because when we would go to our r&d partners or operational partners, there is a understanding that they could determine and solve some problems quicker than you could be a Digital Twin. So we convinced a few colleagues that I know, that let us try to do something in parallel and prove that the models would work. And we were able not only to prove that the models work to these first pilot projects, but also the predictions that we were getting were happening faster than they could get in a, say, empirical or physical environment. And we were actually starting to guide and direct them to solutions and reducing their design of experiments. That case in point would be is, if we could run 1000 design of experiment and get results, whereas in a week, they might be able to run one, maybe two, and the factors were significantly greater, the cost was significantly lower, too, because if you think about it, if you had to run 1000 experiments to try to pinpoint down to an unknown situation and you’re only doing two a week, it’s a long time and a lot of money.
Stephen Ferguson 06:51
So you prove the concept then, and you’ve got sort of corporate buy-in for your processes, which allows you to begin this great scale-up. And so how did the scaling up go, after that point?
John Perrigue 07:02
To give you an idea of core hours. And we started in 2015, and core hours that were utilized for the models we did, we were probably less than 10,000 hours in 2015. By the end of 2016, we had exceeded over 100,000, going to a quarter of a million core hours. The change that happened for everybody was Covid, we were gaining traction every year, growing, like I say in a linear approach, it was growing kind of nonlinear. But in 2020, when the Covid outbreak happened, and all the sites and offices started to close, our team started to expand. It was myself and three individuals now, so a total of four. We went from roughly half a million core hours at the beginning of Covid, 2020, and at the end of 2021, we had exceeded 2 million core hours. We had roughly burned a million and a half core hours of designs for pharmaceutical, for Covid vaccine, for other vaccine, for Janssen, as well as in the consumer products, was just exploding because people were still buying, we still needed to produce. But we couldn’t do the same thing as we did before, ’cause line times were limited due to capacity and demand, as most organizations were experiencing. Plus, with shortages, we needed to be able to adapt our processes and predict if we needed to change from one technology to another technology, how could you do that rapidly to understand the performance of your, not only of your product, but also the performance of the operation in one environment to the next environment? So it was some very busy days, and some very long days between 2020 and 2022.
Stephen Ferguson 08:37
But it’s a good job that you had started that journey only a few years before because you’re in the right place at the right time to make a huge difference, weren’t you as well? We talk about exponential growth. But if you look at the growth of your core hours, and you plot it on the graph, you really were growing exponentially during that time as well, which is amazing.
John Perrigue 08:55
Yeah, we shot off of our chart, exceeded our own expectations. And in reality, we exceeded many things and we learned a lot about how Digital Twin can really help support a business or an operation. But also we got a lot more astute as well, as looking at co-linearity or linearity or non-convergence of some of the processes, be able to start to say, when and how we set up our own DOEs because we would see things before they would happen based upon prior studies. And we would know how to set up the next model saying, not to do that, and became more educated, which is a sense of now there’s conversations around CFD, Digital Twin with a combination of machine learning and artificial intelligence. So the more data that you have behind it to use, the better that AI and ML can become to help you predict how a operation will run with a more complete data set. So I think that was the next conversation I’ve been hearing and the next evolution and I think the elements of having Digital Twin and running these scenarios and running the, we’ll call the “extensive limits,” to understand when the system is in control and when it’s out of control. And having that as a data set for AI/ML type of learning is going to be the next evolution for CFD.
Stephen Ferguson 10:07
We’ll talk about the future in a moment, but I just want to concentrate on the scale-up for a few more minutes. So we’ve talked about how you could use cloud computing to scale up your computed resources, yeah? That’s no problem at all. But surely, there’s also a human element there as well. If you’re scaling up exponentially in terms of the simulations you’re doing, you can’t scale the number of engineers exponentially as well, can you? How did you go about tackling that human element and making sure you had competent people to run those simulations?
John Perrigue 10:34
That’s a great question. And actually, during 2020, in particular, one of our internal customers, our consumer division, asked, “we wanted to do this ourselves.” So one of my Regional Vice Presidents in Asia Pacific that I was working with at the time, on a number of projects that his teams are doing in the consumer, Asia Pacific sites and manufacturing, said he wanted his team to start to learn how to do this. So they could broaden scale as you were mentioning, however, CFD is a very challenging topic. And the software applications are somewhat if, unless you use them every day, it can become very difficult to remember all the steps in the procedure and process. So we embarked upon a design in working with the Siemens engineering group to build basically a user interface or a UI. And this UI was constructed in the means of, when we look at pharmaceutical and consumer products, specifically in the liquids and semi-solids area, again, is we’re either dealing with Newtonian or non-Newtonian types of liquids. So the Siemens Simcenter application has tens of thousands of equations in it, but really, we’re only using a certain subset. So we wanted to make sure that the UI that we’re developing would target the new users that, as we started to democratize the tool, that they would be able to make decisions based upon information that they understood, not Lagrangian, not Eulerian type of mathematics, but Newtonian, non-Newtonian. So, do I have something that’s fluid viscosity? Or do I have something that’s more viscous, or I have a powder in a liquid type of environment? So I’m trying to understand that type of interface. So those were the types of questions we went through with the Siemens engineering team. And we basically built what I’ll call this user interface to ask a user, what site were they working from? What vessel were they selecting? What type of way and that process step? What was it? Was it Newtonian? Was it non-Newtonian? Were you heating and cooling? Were are you using homogenization? In the sense of, homogenizers is when the process of making a lotion or an emulsion, so changing the form to basically make it a cream in the process. So all these questions we laid out, we also looked at the manufacturing network in J&J, is roughly about 30 to 35 sites, it changes based upon acquisitions and things happening, but 30 to 35 sites and over 150 different mixing vessels, as well as different permutations beyond that, in the sense of the internal components and the external components that are used in the process. So we built geometries, we built raw material, linked into the raw materials that are used in these products, we had the operating parameters. And basically, the end result was that any engineer would be able to go in and utilize this tool, select the equipment, select the product they were trying to make, what step it was in, which is the raw material phases, and then basically set up the simulation to run their own simulations themselves to create these manufacturing batch records or constructions that we were talking about earlier, themselves, and that they could scale that and do this. So as we built that, because we had a lot of experience, it only took us about six to eight months to deliver this, it was very, very fast. So we started in early Q1 2021. And we finished in Q4 2021. And rolled this out to the organization, the one thing we did do is, we made sure that we had the operators on board. So we identified what we’ll call a key group of super users. And when we were doing the beta testing, we would have them test along with us. We’re training them on CFD, we are training them on the user interface, but we’re also training them on the democratization of the tool and the process. And when we rolled out, we had about 20 people we trained at the end of that six-month period to do this.
Stephen Ferguson 14:17
So how about things like your nuts and bolts things? Like mesh sizes, mesh shapes, turbulence models? Did they have to worry about all those things? Or did you hide all those things from them?
John Perrigue 14:27
We had a number of those things. We would allow a little bit of selection of number of core but, mesh sizes and calculations we pre-determined based upon the types of studies that we’ve done before, as the size of the mesh, the fineness of the mesh, and in some cases, we even limited the amount of cores based upon the selection. So you could do that, the UI, by the logic that the user was making in the sense of the predetermined questions and radio buttons that we developed, it would predetermine that calculation. So it was a partnership between our team, Siemens and Rescale because Rescale was actually setting up the virtual desktop that these simulations will be running on. So we would pre-determine with Rescale’s help what type of machine the user would end up having in the virtual environment, to run that simulation at that point in time.
Stephen Ferguson 15:14
And because you’d pre-selected lots of those things, it makes the results more comparable, doesn’t it? Across different users or different locations, you can have confidence that when you’re comparing two different designs, which have been simulated by two different engineers, that you can compare those results, I guess, that’s really important, I think.
John Perrigue 15:32
Exactly, I could set up my design and run it. And then you could come back a day later and do the same problem. And our results should be comparable. And in many cases, we did test, in all cases we did test that during our pilot and beta phase to make sure that everything was going properly. And we’ll say the differences between my results and your results were small standards of deviation difference. And that’s really probably only due to, we’ll call rounding, you know, mathematical rounding conditions, not based upon the hardware conditions.
Stephen Ferguson 16:03
I think that’s an incredible story. It’s honestly one of the best stories of democratization of CFD. And I guess the profile of these people, you didn’t have to hire 20 experienced CFD users with PhDs, because, like, when I started in CFD engineering 30 years ago, yeah, everybody else apart from me had a PhD, and you had to have a PhD to be a CFD guy. And I was kind of the exception,
time when regular engineers, who didn’t have to be specialists, could use CFD. And I guess at J&J, that time has already arrived, hasn’t it?
John Perrigue 16:34
It has, and I’m not gonna say they’re simpler problems that are being solved, makes it easier. But they’re basically problems that, you need to have a good scientific background. So, as you mentioned, you know, we didn’t just take any person, a lot of times, these were the process engineers, the ones that really understood what was happening before, but really started to understand the capabilities and the results and interpret the data at the end or interpret the output, if it’s an animation file, or whatever the case may be, to truly understand what’s occurring within the process. So that’s not impossible. So basically, your one course of fluid dynamics in your college degree was sufficient enough, basically, to understand the principles of what’s happening and what you should be expecting to see, based upon observation in reality, right, versus virtual.
Stephen Ferguson 17:20
And those guys are using CFD as it’s supposed to be used as well. So all of the meshing all of the selecting models. Now, those are obstacles that we have to get through in order to get some good simulation results. And ultimately, what they’re doing is they’re interpreting the results, and using them to make decisions in production.
John Perrigue 17:38
Exactly. And from lab to… when we talk about laboratory to full production scale. So they’re moving directly from maybe a scale, when you think about a lab, maybe it’s a one-liter beaker, on a bench with data and test results coming from the research and development teams. And being able to take those same parameters and say, “Now, I’m going to do that in 1,000-liter, or I’m going to do that in a 5,000-liter vessel.” The end result is that you need to have that same output parameters in each of those process steps. But you can use those as your prediction endpoints and then find how your system needs to operate. So the results are still the same. The physics is the physics, is how you can end up delivering that with the types of equipment and what the equipment’s able to produce. Or in some cases, we found out that equipment wasn’t able to produce that. So we wouldn’t be able to select the right technology, we’ll say the right piece of equipment, that could achieve those results before ever running a batch.
Stephen Ferguson 18:30
So I guess one of the side effects of the Covid pandemic, which was awful for lots of reasons, was that it forced pharmaceutical companies to accelerate their development. So that whole, you know, having some sort of vaccine or some sort of cure that works at lab scale. And then turning that into, in fact, billions of doses by the end of the pandemic, was a massive challenge wasn’t it, as well? Probably the biggest kind of industrial scaling-up challenge that the world’s ever faced. So I guess simulation played a key part in allowing you to do that.
John Perrigue 19:02
Yeah, it did. The whole entire industry has been talking about Industry 4.0, for some time, trying to figure out how Simulation Digital Twin fits in. And I think, as you noted, it definitely accelerated that, “hey, we’ve got those modeling teams over there, they’ve been working, we see some good things out of them, we really need that that kick into gear, now.” So, I think Covid did transform many organizations that were using simulation, maybe in the infancy stage, or in the growth stage to say, we needed to bring that into our more day-to-day operations. So that is the next phase for many companies, was, we were working with peers across health authority and regulatory bodies, is to continue to drive to certainty of the models that we’re producing, and utilizing those models and those simulations to be a prediction for how a product will perform, in the sense of when a company comes in and gets an inspection from a health authority, using data as part of the means to fill in with empirical testing to close that loop and that design, but not having to do everything in the physical world.
Stephen Ferguson 20:04
Which is incredible. That’s a huge step forward.
John Perrigue 20:07
I think many companies are definitely building up their internal operations to become more resilient. It’s not really agility, it’s more resiliency, right? In the sense of being able to manage complexity, and being able to do that readily at hand. So in my role at J&J, that was the case, we were talking prior to the call a little bit, you know, my new role now with a different firm and helping build their smart manufacturing operations, is starting to consider those same things because they’re at a building stage now, versus when I left J&J. And I’m now on that same journey, again, which I enjoy. I love building. That’s the engineer. And a lot of us whenever you get the bell, then change and transform. It’s an exciting time.
Stephen Ferguson 20:46
So what do you think the endpoint of your new journey is, then? Because we talked a bit earlier in the interview about… you’ve obviously proved that you can democratize the simulation of really complicated multi-phase problems. And in doing that, you’ve taken the big first step, I think, in automation, as well. So you’ve got engineers, pushing the buttons and making choices. But once you’ve stuck all those things behind an interface, then you can start to automate some of those calculations and use them to do thousands of simulations. Now, one big issue is, of course, lots of your simulations are transient, and so there’s a lot of computer power involved. What do you reckon the end game is for in terms of kind of automation optimization? Machine learning, you mentioned earlier? Are we heading in that direction do you think?
John Perrigue 21:32
I believe we are, and I think it’s gonna get more and more to a combination of, as you’re calling, automation. So maybe it’s the automation of the model, but it’s really linking into automation, and moving simulation closer to the actual operation, in the sense of what we’ll call a reduced order model. And using sensors, real-time at the location with edge computing, simulation, all working together at one point in time to create what we call, eventually the Intelligent Twin, because simulation is going to be giving direct real feedback to the operator at the time, based upon sensors and results that are measuring the process. And eventually, I can envision that simulation will be used to be the prediction of where things will go or what to avoid. And really bringing that whole operation in a closed-loop real-time environment. I think we’re getting very, very close to that. I’ve seen some applications where it’s almost there. It’s very, very near. But I think on critical operations, critical applications, it’s the next evolution.
Stephen Ferguson 22:33
And so you talked about the start of your journey, how verification and validation and convincing your corporation that what you were doing was the right thing and giving people trust in your simulations. I guess with the Digital Twin, we’re going to have that validation and verification data, a constant stream of it in real-time, so we can update our predictions, we can improve our predictions, and that’s going to be a game changer as well, I think.
John Perrigue 22:56
Yeah, exactly. And I think the work that’s going on with external consortiums or engineering forums like ASME, American Society of Mechanical Engineering, you have ICH, all working in this environment to meet the demand, even the health authorities are starting to move in the direction of database design and prediction, it’s not only industry pushing its regulatory authorities to that are accepting that this is going to be the wave of the future. But the one thing we always have to ensure, as you’re noting earlier, is quality and accuracy and repeatability. Because of repeatability, in the sense of models, you want to be able to come back and run it a year from now and ensure and have that uncertainty removed from your process as well, that any models that you’re running are continually learning and also being very accurate in what they’re doing. Because at the end of the day, it’s all about patients and customers that are using these products.
Stephen Ferguson 23:46
Yeah, so we’ve talked a lot about drugs. And I just wanted to finish off by talking about the benefits to patients. So real-life human beings like me who struggle with a constant stream of ailments, especially as you get older, what is the benefit to patients of all the work that you’ve been doing?
John Perrigue 24:02
I’ll say first is, is that it really reduces the time to be able to deliver products to the market. If we think about a typical pharmaceutical lifecycle. Some people may know the background, you could have 10, what we’ll call 10 molecules that you’re looking at, in reality 10 molecules at the beginning of the early phases, pre what they call “phase one,” which is laboratory testing, these types of environments, out of those 10, you may end up with one product, but if you could use simulation to not only predict things during the design phase, in the r&d phase, but in the manufacturing phase, to shorten or understand how things will perform. You’re able to also deliver that sooner and quicker to the patient. So it’s not effectively skipping steps. It’s using knowledge and know-how from data with selected empirical testing, along your design of experiment, should be able to minimize the amount of step, to minimize the amount of physical testing in between which can reduce delivery to market by months. We’re not talking about a couple of weeks or a couple of hours, it’s a months and delivery cycle time reduction.
Stephen Ferguson 25:08
And I guess that’s also reducing the cost of production, which probably reduces the cost of the product to the patient as well. So getting effective and affordable drugs is a big challenge, isn’t it, across a population of 8 billion people, which is growing to 10 billion people, I guess effective and affordable medicine is one of the benefits of this.
John Perrigue 25:28
It does, because in that sense, there has always been a push in many industries, any industry is designed for manufacturability, designed for quality designed for value, in the sense of trying to do the best performance, hit the right target, but also do it at the best cost or price point. So all these things really CFD does, you know, in the sense of simulation, it’s looking at that environment and being able to optimize upfront, historically, it’s been “roll the process out, and then try to optimize and take the inefficiency as a waste out of the process.” Well, with simulation, you can look at, what is that cycle time and design it for the most optimal cycle time for that piece of equipment, to deliver right up front, so that waste reduction becomes less about the process, but things surrounding the process that we can continue to strive to reduce. I also think too, to your question, is that if we think about that is also the sustainability part of it. If I’m doing less testing or I’m using less energy, as any company’s looking at, we’re looking at our customers in the sense of, what are we doing to our environment? And how are we trying to lessen our impact upon greenhouse gases or consumption of water, reducing waste, that needs to be processed before being returned back to the environment. Anywhere that we can impact that, makes us not only better in our performance, but also we’re keeping the environment much, much safer from any unnecessary impacts, right, in the sense of we have to use resources. But if we can control that resource’s usage or minimize it, then the better off we are.
Stephen Ferguson 27:01
So thank you, John, and we’re about the end of this podcast now. So, I want to thank you for all the work you’ve done and helping to engineer a healthier, happier and more sustainable world. So thank you very much for your time, John, and thank you for everybody who’s listenined.
27:13
Thank you, Stephen.
27:15
This episode of the Engineer Innovation Podcast is powered by Simcenter. Turn product complexity into a competitive advantage with Simcenter solutions that empower your engineering teams to push the boundaries, solve the toughest problems, and bring innovations to market faster.
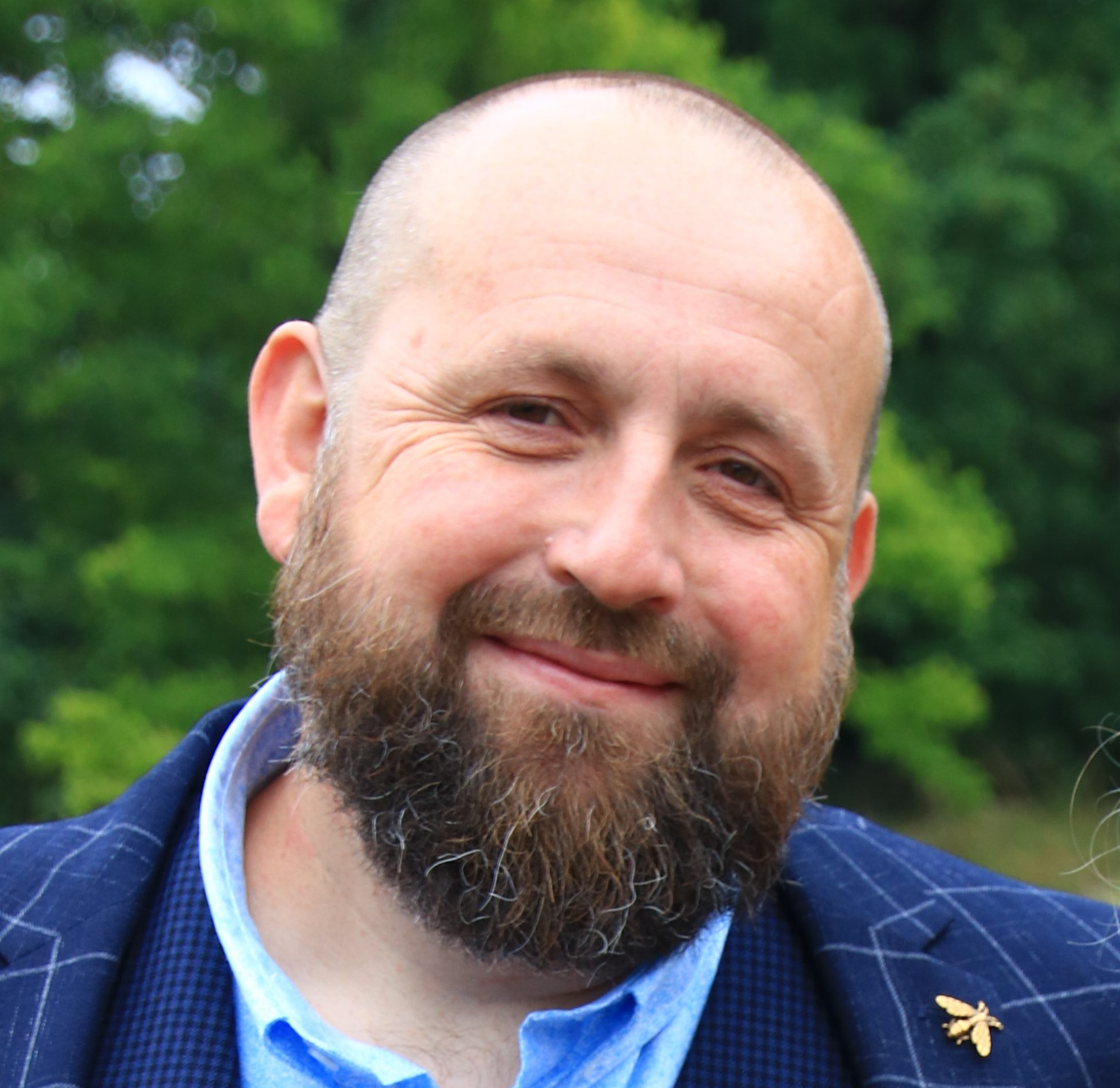
Stephen Ferguson – Host
Stephen Ferguson is a fluid-dynamicist with more than 30 years of experience in applying advanced simulation to the most challenging problems that engineering has to offer for companies such as WS Atkins, BMW and CD-adapco and Siemens.
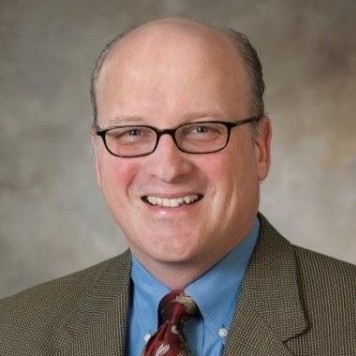
John Perrigue – Guest
Senior Director, Smart Factory – Digital Twin at Johnson & Johnson, global leader for Strategy and Implementation of Industry 4.0, SMART Factory and End-to-End digital transformation across the J&J Supply Chain (Consumer, Pharmaceutical and Medical Device).
Take a listen to the previous episode of the Engineer Innovation Podcast. The truth about AI in engineering with Jousef Murad (Season 2 Bonus Episode)
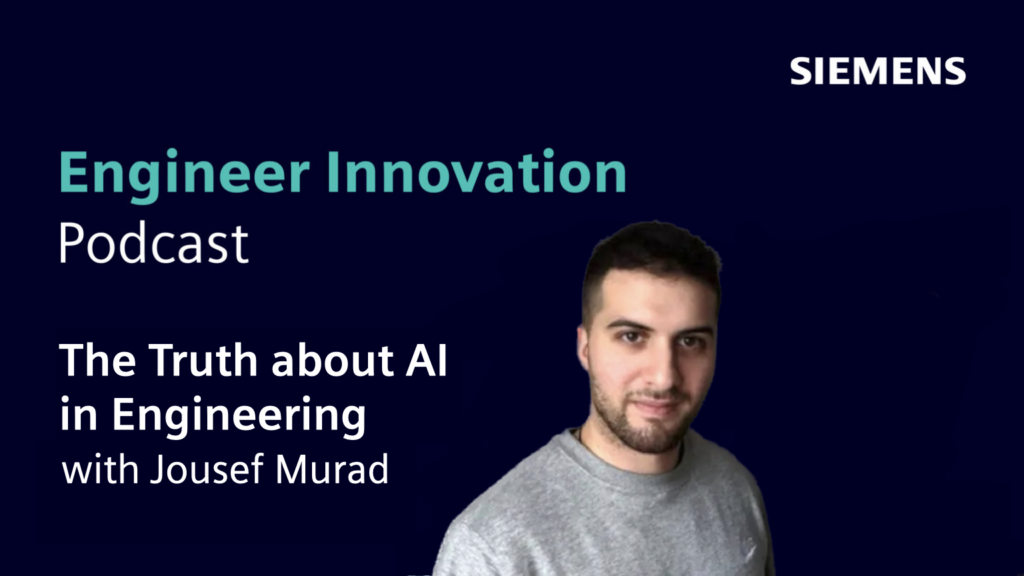
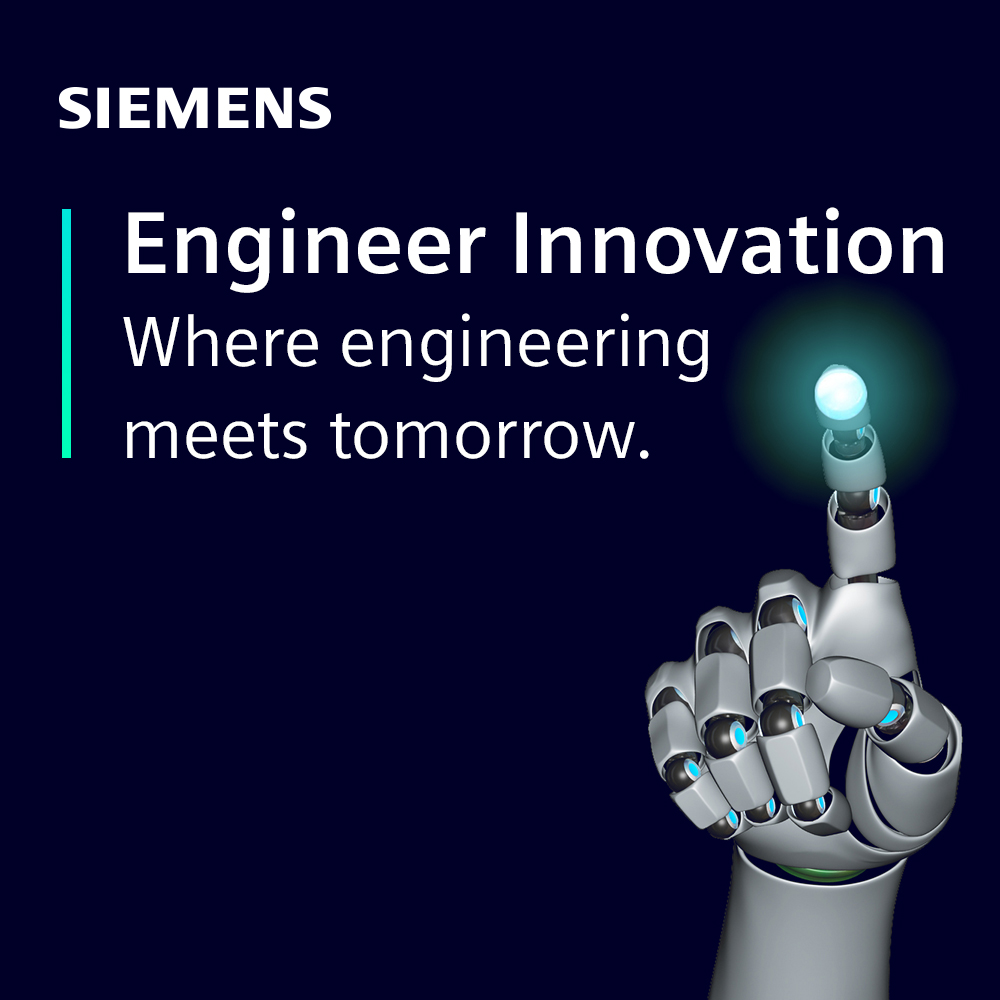
Engineer Innovation Podcast
A podcast series for engineers by engineers, Engineer Innovation focuses on how simulation and testing can help you drive innovation into your products and deliver the products of tomorrow, today.