The benefits and challenges of collecting big data
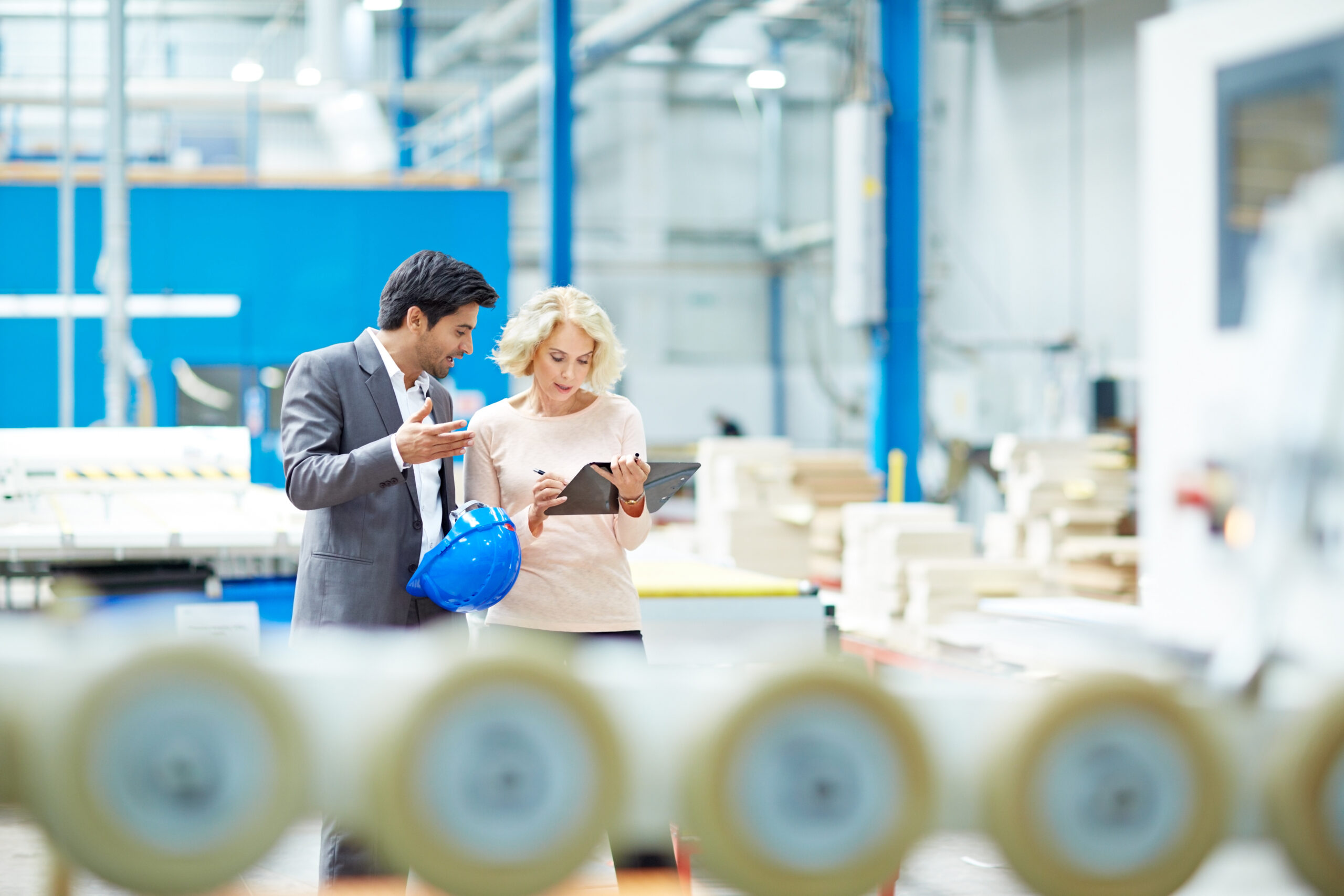
Companies operating in sensitive industries such as aerospace, automotive, smartphones, and medical need meaningful analytics to meet ever-increasing quality standards and dynamic market trends. For the most part, they’ve already got the necessary data, as IoT manufacturing applications constantly gather huge amounts of real-time shop floor data. But companies often lack the tools to aggregate the isolated data sets and analyze them to reveal important insights, especially for complex production activities.
According to research by MIT, approximately 70% of manufacturing data is not used to drive informed business decisions. That’s a major problem for manufacturers since those insights are critical not only for diagnosing and correcting process flaws, but also for improving materials and supply chain management, tracing the sources of problems and defective or counterfeit parts, and providing trends analysis for business forecasting and reporting.
Challenges in big data collection
One of the challenges in collecting big data is that interfaces to data collection sources aren’t standardized. Machines from different vendors use different formats and languages, so data can’t be understood from machine to machine, hardware to software, and system to person. Normalizing the data into a single language with consistent meaning and comparable variables, regardless of the information source, requires a major effort that is often beyond the capacity of individual companies, especially when trying to set up a custom data collection system independently.
In addition, the increasing trend of higher-mix production compels manufacturers to improve changeover time between products. The goal is to optimize line utilization, which requires a different approach than simply focusing on speeding up the placement for a single board. Since PCB manufacturers usually work with more than one production machine vendor, part-data creation and management have become more critical than ever before.
Benefits of using big data in manufacturing
When big data is collected and normalized, software can be used to create accurate prediction models for all parts of the value chain. Businesses can evaluate multiple “what-if” scenarios relatively inexpensively and assess if a design can actually be made. They can also ensure that the manufacturing plan is up-to-date and synchronized, and that the production system is optimized and performing as planned before investing in new equipment and materials.
Big data also supports smart manufacturing, which in turn helps address market needs like customization, personalization, and high-mix production. By eliminating information silos and enabling data sharing, businesses can dramatically shorten NPI cycles and time to market. They also gain greater adaptability through better control and visibility into their supply chain, giving them the capacity to build more products, faster, and at a higher profit.
Last, but not least, businesses can appeal to consumers by providing a product that matches or comes close to their ideal while allowing producers to utilize improved workflows and technology to maintain high output and save costs when compared to a pure mass production environment.
Turning big data into smart data
There is no question that big data can have a major impact on business success—if it can be turned into smart data, that is. The first step to creating smart data is normalizing data across the value chain so that it can be understood by all involved, and variables are consistent no matter what their source.
When all site applications produce the same type of normalized events in a single language, only minimal effort is required to connect processes for the highest level of detail and perspective and identify root causes and bottlenecks. It also makes it easier to distribute and use the data for the discovery of resources in the factory and the events supported by those resources.
Not knowing the best way to read, understand, and apply data can actually be costing your business. Those costs could take the form of lost revenue opportunities, lower efficiency and productivity, quality issues, and more. In an upcoming blog, we’ll look at the difference between data and analytics, the different types of analytics, and how analytics can help drive smart business decisions.
To learn more now, download our e-book: