Exploring Generative AI Part 1 – Transcript
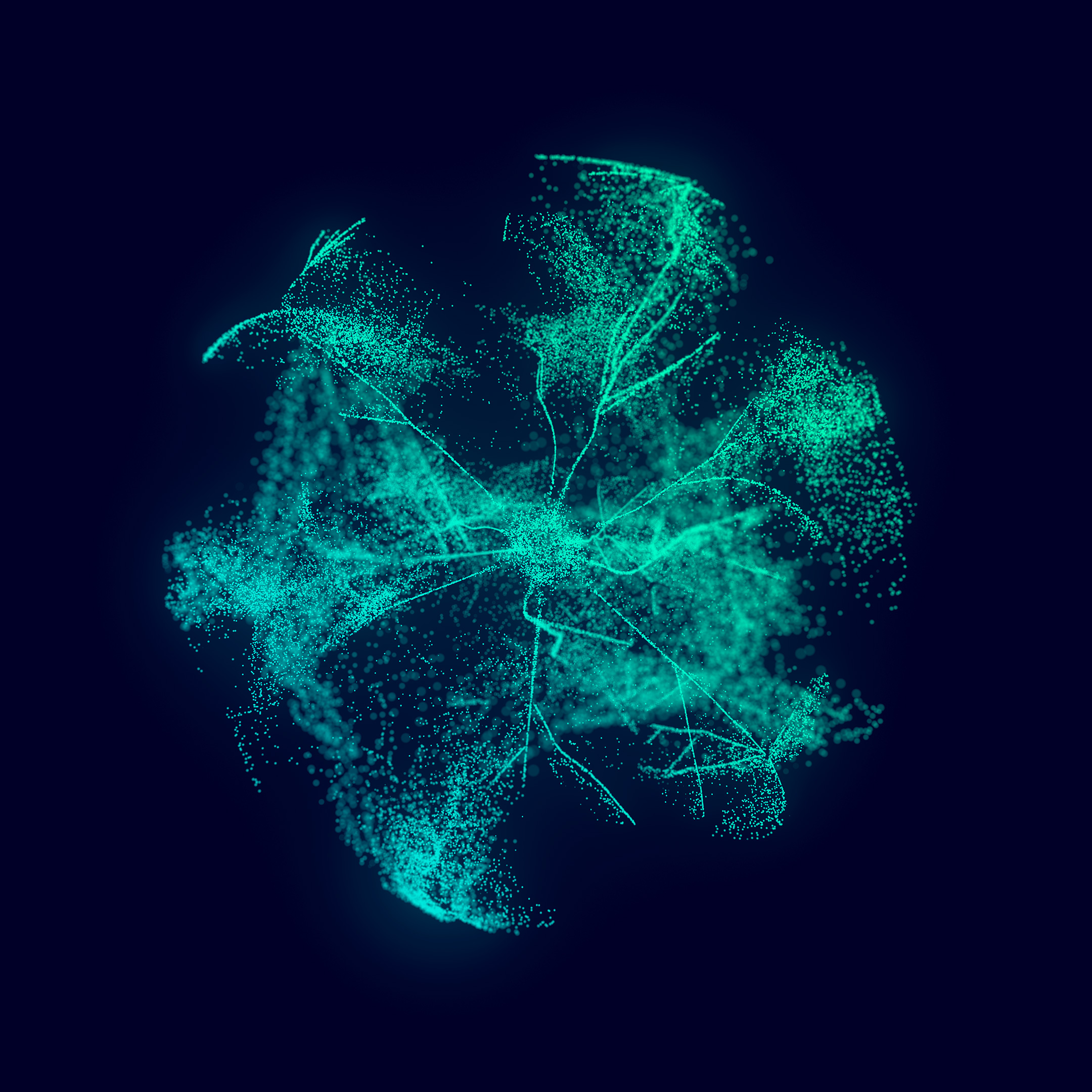
In a recent podcast, I talked all things Generative AI with Dr. Justin Hodges as we explored the various ways Generative AI is reshaping the world of design, engineering, and simulation. Check out the podcast here or the full transcript below.
Spencer Acain: Hello, and welcome to the AI Spectrum podcast. I’m your host, Spencer Acain. In this series, we explore a wide range of artificial intelligence topics from all across Siemens and how they apply to different technologies. Today, I am once again joined by Dr. Justin Hodges, an AI/ML technical specialist and product manager for SIM Center, to discuss the ways generative AI is changing the world of engineering and simulation. Welcome, Justin. It’s good to have you back.
Justin Hodges: Hello. It’s always a pleasure to talk with you and obviously always a pleasure for me to talk about AI.
Spencer Acain: Great. Well, before we jump into our topic, can you give our listeners a brief overview of your background and your role at Siemens?
Justin Hodges: Yep. Sure. So I started out doing undergraduate, masters and then PhD at the University of Central Florida on mechanical engineering. And specifically I had a turbo machinery focus with thermal fluids, heat transfer, numerical experimental mix. And then somewhere in 2017 in fall, I had my first professional experience with AI in our Siemens Healthineers Group in Princeton, which our focus was simulations and AI in combination for a better diagnosis approach for a certain kind of lung disease. And that was kind of a surreal experience because I just saw the other interns for replacing applications for some numerical simulation techniques, like every day with AI. And I mean, that was a long time ago. That was in 2017. So I kind of realized at that point my vector career wise for at least that nearest decade would be machine learning. And I came back and finished my PhD with a huge focus on machine learning as much as I could to what I’d already had planned in my dissertation. And then since then, just on the side professionally at work, in all scenarios, just educating and ramping up and getting more and more experiences with machine learning. And then few years back now, I started with the opportunity to formally do that at Siemens as my contribution in my role here. And my role is that of a technical specialist for AI/ML in product management. And we will probably have viewers from all across the portfolio as far as their favorite software that they use. And I’m delighted to say that my focus isn’t just on one of them, but kind of a portfolio wide perspective on how AI should be implemented into our products across our portfolio. So that’s me.
Spencer Acain: Wow. It sounds like you’ve really been in the AI space for quite a long while now, just kind of following it progress as it progresses. And that kind of leads us into our main topic here, the kind of latest hotness of the AI world, which is of course generative AI. So can you give a definition of that? What is generative AI really?
Justin Hodges: Yeah, it’s a good question and I think it’s easy to get confused and even ChatGPT could probably get confused pretty easily here. So it’s definitely something to start out with. Good point. So in the simplest sense, I would say generative AI to me is a means for creating different types of media and content. And essentially it’s a very complex situation as far as learning from vast amounts of data. And usually what you see are some pretty high fidelity models in the machine learning world that are being used. They don’t require a lot of human intervention and then they can produce some pretty amazing things. And I think we’ve seen quite a lot of examples. So producing text, you’ve seen ChatGPT and other language models that are conversational. So the Llama model from Meta or Bard from Google or GPT-4 from OpenAI or the new PaLM 2 model. And then before the craze of ChatGPT probably saw text to image, so write a prompt and then dolly or other really famous stable diffusion models would create pictures of animals, faces, photorealistic stuff. And that’s just become more and more as well in the backdrop. And just lastly, I mean, it can make music editing tools, social media content, all kinds of things. So it’s really about generating these complex models from a minimum amount of human intervention or oversight as far as actually doing it, producing the stuff it’s creating. And then one last thing, I’m already a bit long-winded, sorry, but I think it’s important to address probably the familiar area that our audience would be experienced in as far as machine learning or surrogates or statistical stuff. So you could look at that as fitting data. I think a lot of our CAE related customers are probably familiar with producing some sort of CAE data and then fitting data to it and fitting a model to it, excuse me, like a reduced order model or surrogate. And so that exercise of data fitting is not necessarily generative AI, but we can still do things with that that would then later constitute as generative AI at a high level as far as creating new things, which I think we’ll get into in the engineering context. But hopefully that provides backdrop as to different things you see in the landscape and kind of dividing between generative and non-generative.
Spencer Acain: Yeah. I think that’s a great kind of starting place there to say a good definition for, so make sure we’re all on the same page when we’re talking about generative AI. So to be clear, generative AI is not like when you have a bunch of data points and you’re to put it simply kind of fitting a line between them and saying, if I keep going on this line, then I’ll reach this other data point that I haven’t, like experimentally measured, for instance, and just the AI equivalent of that. But generative would be different from that, correct?
Justin Hodges: Right. Right. So let’s say you run some simulations and you want to use a regression approach to basically fit a model that can accurately capture those data points. So it could be linear regression, polynomial model or something AI specific like a neural network. So in that case, that’s in this class of data-driven approaches to fit data. But the distinction is maybe you have a more broad design philosophy where you need those types of ROMs and surrogates to be created so that you can go off and do generative engineering and things like that. Where at that point, instead of doing time-consuming simulation or physical measurement, you can use these surrogates that you created. And in that case, the purpose of that global exercise could be please find better designs faster, please generate novel rules for my design space, things like that. And they could be sort of powered by surrogates, but in that case, indeed you are doing generative engineering, and that does push it in the category of generative AI. So not to make things too confusing here, but yeah, strictly this class of bidding models with ROMs, it’s not in that category of generative AI.
Spencer Acain: Right. Yeah. I just wanted to make sure we were covering that both kind of on the same page there just to be sure. So with that kind of basic definition out of the way, I think we are good to jump into our main topic here. So how do you see this type of generative AI being applied within the CAE or the computer aided engineering space or even the simulation space as well?
Justin Hodges: We have this really cool concept in our group at Siemens of Learning Day. So several times a year we’ll allocate a full day towards learning new things and maybe exploring things we quite haven’t had time to. And there is a class on generative AI that was offered and essentially had a really good quote at the beginning I like to start with, so the quote is “Lifting the dirty, dull, dangerous and difficult tasks from humanity’s shoulders, so we can simply focus on the very essence of our work, the vision, the idea, and the purpose.” And I think that’s really great because we could probably, all or most of us, stop and reflect on the deliverables we provide at our job and basically think about how much of it is dirty, dull, dangerous or difficult, and how it could be in a sense of distraction. So I think there’s a lot of examples for generative AI to really allow us to spend more of our time, a higher portion of our time on our actual discipline and expertise and doing that delivery rather than just the mechanics that may be time-consuming and dull. Yeah, I don’t know if that resonates with you at all.
Spencer Acain: Absolutely understand that feeling. I think for everybody, there’s an element of their job or elements that it’s just like it’s something that has to be done, but it’s not interesting or exciting or it’s not really part of their core responsibilities, but it just has to be completed basically. And I think we would all like it if that could just be automated, like a click of the button or just say, hey, ChatGPT, can you go and fill out this form for me? Here’s all the data is in this CSV file and then just go put this in and figure it out.
Justin Hodges: Yeah. So this is really the first theme here of user experience. We want the user experience of the software we provide to be less of those things, dull and difficult, etc. So I mean, this is not a new concept. You could think about Microsoft Word Clippy from, I don’t remember when, 20 years ago. And really it’s supposed to be a support based thing. It’s supposed to enable you and provide you support on demand. And I think that there’s plenty of examples of that now for use cases for some of our generative AI models and these conversational tools. So things like you can use AI for rapid answers on what’s the documentation. If I have a question rather than going into the documentation, it can just provide you the answer. It can work as a knowledge base for other data or results or practices inside your larger company, and then maybe it could act as best practice providing you best practices. So especially as you go to larger and larger companies, this information is usually spread out in different documents, different places, different people, different projects. And really the consolidation of that into a machine learning model means that you can just talk and conversationally get that information from the model rather than have to do the traditional thing of looking through a huge support document or reading a bunch of separate things and then that sort of thing.
Spencer Acain: That could take several hours really to just to look through everything if you have a very specific question, for example. I know I’ve experienced that where I have a very specific question I’m looking for and it’ll take me an hour or two hours to find it sometimes because it’s just a single page in a very obscure help document. It exists, but as a user, I’m having trouble finding it just because it’s buried among a mountain of other information. So, that’s what you’re saying AI could really help with that, I think.
Justin Hodges: Yeah, and it gives us other sort of things. Standardization is really important. How you go through and interpret all of that information might be different than somebody else. Or if people are running simulations in the same software, same version, same domain, say turbo machinery, it can still vary vastly from person to person, different countries, different preferences or styles mean. So it can be a way to help standardize. It can also help beginners to rapidly contribute. So in that case, there’s probably an apprehension or a learning curve at first when you jump into a new area or start a new job could probably really help with that. And really, if you think about the last time you used a software and went through the support document, you probably learned a few things and then carried that forward. But then if somebody else individually does the same, they may not capture what you learned as well. So, these sort of models are useful to consolidate information and provide a sense as well and the answers that it’s telling you based on history, historical data, historical models you have, etc. But other than support, there’s really more technical things that are also super appealing about this. And part of that is not just support documents and stuff like that, but it’s also expert knowledge. So I could take raw simulation data as an expert in a certain domain and I can basically make inferences and conclusions from it. Is this tip vortex too high? Is the separation too large? Is the temperature gradient too high for the mechanical? I think of the part. Those things may not be obvious. They’re definitely not in the support manual. But really you can annotate that information and then store it somewhere and basically have a model as well learn from that. So it could basically be like if you’re doing something and generating results and looking over them in your traditional way of doing things, it’s like you have an expert over your shoulder. So, that’s when it gets even more and more fun because then you’re learning things from physics and you’re kind of consolidating it into just conversation stuff, like good design, bad design, this is too big, that too small, etc. So I think both are pretty big for this idea of like, well, it could make the user experience and the software a lot better. But probably one of the coolest things to look at, I would say is a final item here as far as user experience, would be stuff, I don’t know what to call it, managing workflows. So basically, that could mean a few things. That means I could just speak or conversationally talk to this ChatGPT, for example, and it could kick off different simulation runs, it could do different complex tasks, it could set up models for me, things like that. Or maybe as I’m doing what I normally do in my software, clicking around doing things maybe like little histograms pop up and say, oh, I think you’re going to do this next. If you want to click this button to accept it, you’ll just automate and do that whole chain of sequences we expect you want. I think in Amesim we have a capability there where you’re building a system simulation model and you have this machine learning in the background learning from your tendencies, and then it starts suggesting to you the parts that you may want to put into your assembly next. And that saves a lot of time, right?
Spencer Acain: Absolutely.
Justin Hodges: Yeah. It’s on a bit on the border of generative AI. But I would say the last thing in this idea of managing workflows is in every industry there’s probably different challenges. Like in automotive, there’s thousands or hundreds of parts in a simulation. And we’ve gotten a lot of feedback that dealing with that takes more time as the user and simulation architect than maybe running the simulation sometimes. So you have really point solutions, application specific sort of applications in machine learning where maybe you make a machine learning model that can recognize all those parts for you like we have in our services team. Or maybe meshing is really intensive for a certain application, and we could use this generative sort of predictive AI model class of family to handle those sorts of tasks. The complex task of doing all the steps in a meshing sequence or pre-processing in general. So there’s a lot there. I mean, it’s really dense, but I think the opportunity is really vast, and you’ll see a lot of these things percolate into society and as well as CAE.
Spencer Acain: Thanks, Justin. That was a really insightful answer. But unfortunately that is all the time we have today. So once again, I have been your host, Spencer Acain and thank you for joining me on the AI Spectrum Podcast. Tune in next time as we continue our discussion on the exciting topic of generative AI.
Siemens Digital Industries Software helps organizations of all sizes digitally transform using software, hardware and services from the Siemens Xcelerator business platform. Siemens’ software and the comprehensive digital twin enable companies to optimize their design, engineering and manufacturing processes to turn today’s ideas into the sustainable products of the future. From chips to entire systems, from product to process, across all industries. Siemens Digital Industries Software – Accelerating transformation.