Talking Aerospace Today – AI in Aerospace Part Two – Transcript
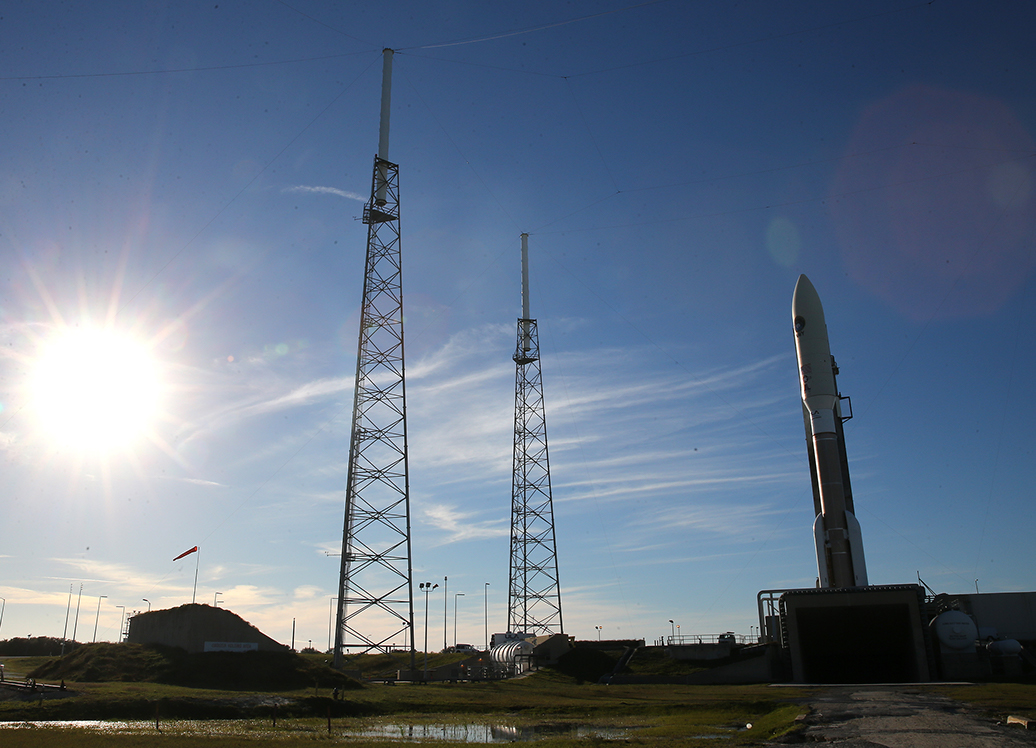
In the latest episode of Talking Aerospace Today, Todd Tuthill and Justin Hodges continue their conversation about AI in aerospace and digital transformation maturity, discussing how it can improve engineering workflows and attract new engineers.
Patty Russo: Greetings, and welcome to another episode of Talking Aerospace Today from Siemens Digital Industries Software. I’m Patty Russo, and today I’m joined again by our VP of the Aerospace and Defense Industry, Todd Tuthill, as well as Justin Hodges. Justin is an AI/ML technical specialist and product manager for Siemens Simcenter. In our last episode, we began the exploration of AI, which we learned serves a vital role in the later stages of digital transformation maturity. Justin gave us an introduction to what AI is, and Todd identified why the aerospace and defense industry needs AI. We also talked about how the industry could leverage its benefits. Today’s episode continues that conversation, so let’s jump right back into it.
Patty Russo: So I have a follow up, Todd or Justin, to either of you based on what Todd just said, Todd, you talked about how AI could potentially improve engineering processes in our industry. And as you were talking, it occurred to me that not only can AI potentially improve our engineering processes, but, and I’m going to digress for a minute, how can AI improve the perception of what A&D engineering is for maybe somebody who is new to the workforce? You know, we’re talking to an audience, likely, that’s been in the workforce for a long time, but we all know that there are other industries for engineers that are a little quote unquote sexier than what A&D has been perceived lately.
Patty Russo: So how could AI potentially help new engineers wrap their heads around this industry and say, hey, I really want to go work for, you know, an A&D company instead of the latest, you know, “latestandgreatest.com” or, you know, the others that we all know that folks are gravitating to?
Justin Hodges: I’ll take the first one, and then especially on the aerospace specific answer, I would not speak on that over Todd. So you’ll definitely follow up if you want on that one. But I think that the opportunity here, what we’re really describing as a use case is consolidation of expert knowledge. And in that case, that would be Todd’s example of learning from experts. If they were to look at raw results from a test or simulation or a design analysis, you know, they would abstract certain things that would tell them from their experience on, you know, directionally this is a good is it a good design, a good area to explore or maybe not. And what machine learning allows you to do is to scale that information to anybody in a callable way from a model, and that could be in the case of people leaving the company and walking out the door.
Justin Hodges: Perfectly said, and I think there’s a lot of opportunities further where even the scenarios where people aren’t leaving. If you’re in a giant company across different continents and different teams and a bunch of disciplines, it’s really easy to redundantly investigate similar things at different teams over different times, and machine learning can help really, you know, with some layers of semantics and things and ontologies allow people to make the most out of their data as well and not have sort of redundant exercises on exploring certain parts of their design.
Patty Russo: Todd, your turn.
Todd Tuthill: Okay, so I was thinking about that, Patty. And I’ll date myself here. I went to engineering school in the 80s, probably before some of our listeners were born, but I was thinking back to what it was like back then and why engineering was attractive to me. And one of the things, I think back to the 80s, we had pictures of what design looked like in aerospace design, looked like in the 50s and that looked like these big rooms with drafting boards and tables and engineers using pencils and slide rules and things to design aircraft. That’s what it looked like in the 50s. Well, the big attraction in the 80s and the school I went to was, okay. “We’re going to teach you-“ Because I actually learned to do mechanical drafting in college, and we did some of that. But one of the attractions was we’ve got these new tools, these mechanical and electrical design tools, and I used those things in college. They taught us to do drafting with a pencil and then they said, “Now we’re going to do drafting with or mechanical design with these kind of tools.”
Todd Tuthill: They weren’t nearly as sophisticated as they are today, but we’d use computers to do mechanical design, use computers to do electrical design and analysis, and back in the 80s that was just kind of becoming a new thing. It was a work environment, kind of an attraction to say if you come into engineering, if you’re going to design aerospace, you know, number one, I think we have the coolest products in the world. We’ve all been to air shows and I can tell you there’s nothing cooler about going to an air show and standing there and watching something you design flow over your head. I can’t even describe how cool that is. And there’s a cool factor to that.
Todd Tuthill: But as you said, we’ve got all of all of these younger engineers coming out of school right now, and they’ve lived in the video game era. They’ve lived in the era of iPhones that they can speak to, and we don’t want them to come into aerospace and have to take go 10 to 15 years back in the tools they use. We want our tools to be as sophisticated and as useful and as integrated as that iPhone in their hand. And I think that’s one of the attractions to me of why artificial intelligence can really raise the level of the way engineers use tools. It’ll multiply their impact, but it’ll also create a much more inviting, a much more attractive environment for there to spend their days.
Todd Tuthill: They’re not going to do- We talked about automating the mundane. We don’t want engineers to have to do mundane things like search for data and information, but maybe a mundane thing is I don’t want to spend time doing all the PMI information on my mechanical drawing. I don’t want to spend the time actually laying out a schematic. I want to give the algorithm some parameters and have it do the basic schematic for me or do the basic mechanical design and the PMI can take a lot of those things that we used to think of when I was in school. Those were some pretty high-level engineering things we were taught, and I think that’s changing.
Todd Tuthill: Engineers, people are becoming much more sophisticated, and what was a high-level engineering test 20-30 years ago is now thought of as a mundane thing that we want to automate and take away from the engineers so the engineers can really spend their time being innovative and really using their critical thinking skills. So it’s the environment. I think that’s the attraction and we’ve got to get there because you’re right, other industries and companies are. And if we don’t go there too, a new cutting-edge engineers won’t want to work in aerospace.
Justin Hodges: I’d love to pile on because I can say I have a lot of personal testimony on what it’s like to use some of this. Generationally, I think the way software is changing is exactly like you said, as far as integrated intuitiveness, connectedness. And you know what is pretty astonishing is in some of the best machine learning journals and conferences, something like 30 or 40 percent according to some media surveys, 30 to 40 percent of the manuscripts are completely open source. Code, paper, data, everything.
Justin Hodges: And so you get this great scenario where now people are some of the most wealthiest tech companies in the world or in history are publishing their algorithms, right? So that literally everybody can use these state-of-the-art machine learning models and other applications. And I think that really allows you to do some amazing things with the software and yeah, I guess the ultimate fear is, what are the mundane things? Well, eventually, maybe if you don’t adapt then the software will feel like the mundane thing, and that would just be the worst, right?
Patty Russo: For sure. Yeah. So one of the things that you both I think alluded to if not covered or touched on specifically is just the UX environment and things like that. So what are some examples of how AI can improve those engineering processes, UX, workflows, that sort of thing in the A&D industry?
Todd Tuthill: So one of the ways I think, you look at the tools that we use, mechanical design, electrical design, they tend to be very, very complex. And the learning the barrier to start using those tools tends to be high. In other words, you know to really be an aerospace engineer, you don’t just need to be an expert in aerospace engineering, but many times you need to be an expert in the tools and that barrier can be pretty high. One of the things I think AI can do is really democratize the use of those tools and make it easier so the tool kind of understands and knows how an expert would use it and can suggest and guide the way you do that. It’s kind of an assistive UI. So that’s one the simple areas I think that AI can certainly help with.
Patty Russo: Do you have anything to add, Justin, regarding user experience and/or workflows, engineering processes, that sort of thing?
Justin Hodges: Yeah, totally. You can go on, Coursera or platform of choice, take a large language model, sort of class or generative AI class, and it could be nothing to do with aerospace or mechanical engineering or engineering simulation or engineering tests. I mean it’s so aligned, though, even if you go separate, you’ll still see these main use cases are things like document search, you know, chat bot for support and training it on this body of knowledge of your choice, which could be aerospace specific, you know, so the support Persona is really huge. And I think what’s exciting for us and in this industry is we don’t need the, you know, latest and greatest sort of things in in machine learning development to make huge impact.
Justin Hodges: I mean just these basic capabilities for large language models would go a huge way because like Todd said, consistent with my experience, it’s a ton of work just to manage the support and usage and things like that of the software, which essentially are just tools to do your job, not the things you want to focus on. And then you can even go further to say that the applications for AI could be to actually orchestrate your software for you as a subsequent step, right? So I could just conversationally say, you know, I want this type of model, I want this type of setup and, you know, bam, it could get set up for you and save you a ton of time. And these are just really the low hanging fruit, easy applications like I mentioned from just mainstream sort of use cases for large language models as one example, so it’s really ripe for us to see huge innovations.
Patty Russo: So what about the part of engineering that requires a little bit more of an organic approach like concept evaluation and activities such as that? Is, there an application for AI or machine learning in that regard?
Justin Hodges: So I think this is aligned with generative engineering, generative AI. It kind of feeds into that area, and I think one sort of relatable example could be not to be too specific, but this topic of Gans or autoencoders or variational autoencoders. I mean you see maybe these popular examples of machine learning models generating pictures based on text you provide, generating pictures of people that don’t exist but look photorealistic. I mean, you can really translate some of that technology into the engineering design space and that could get into, like you said, Patty, these abstract concept sort of level stages of designs. Well, those models are clearly able to learn, you know, context and things like that when they generate like a picture of a face, right?
Justin Hodges: That’s not exactly a 10-parameter type input and then provide an output, you know, sort of model. And I think those are examples you can see how machine learning can come up with its own designs as well. Yeah, I think that’s to come. It’s already there technology wise, but it’s somewhat less popular. But maybe just a short outro example to before I stop talking on this one is, you, know oftentimes we have so many parameters for even a component level simulation or a component level design. Maybe 30 parameters to describe how we can make a part and what it would look like. Well, if you run just, you know, say three variations of each parameter. I mean, that’s thousands and thousands of cases. It’s too many to simulate. It’s too many to test. It’s just infeasible, right?
Justin Hodges: So what’s really great about these sort of techniques is you can apply those machine learning techniques to learn that same space but maybe with 10 parameters, and now all of a sudden 10 to the three simulations, it’s much more approachable than 30 to the three. And now you can actually fit that in a project plan to explore that design space. So in essence, you can represent the design space and your geometries differently and in a more suitable way for your timeline.
Todd Tuthill: So thank you, Justin. That’s a very, very good example of how AI can be applied. Let me talk more specifically about the aerospace industry. I talked earlier in automation about automating mundane things that human beings don’t want to do, and I think this is really the transition of automating the things we only thought humans can do. In aerospace, if you think about the high kind of sophisticated things engineers do, they start with a blank canvas and they design things that didn’t exist before. We think about designing an aircraft or the wing of an aircraft and all the subsystems that go into that.
Todd Tuthill: I really see a time and they’re not too distant future where an engineer will be able to take some basic parameters about the wing of an aircraft, it’s outer mode line, maybe some basic parameters around, “I want it to be, last this long. I want it to have this kind of life. I want it to weigh maybe optimally way. I wanted to be able to be designed, excuse me, I want it to be able to be manufactured easily.” Take those basic parameters, put that in and where all the mechanical design of the structures and the all the pieces that used to be done by hand will all be done generatively. And not just that, but once that structure is built, then there’s all these subsystems that go into the wing.
Todd Tuthill: I believe we can generatively design those too in the same way that human engineer learned from previous examples and in talking to experts about how to design a wing and the structure of a wing, and how to lay a fuel system and a flight control system into that wing. I believe that we can use machine learning to do that. And that’s the key. It’s the artificial intelligence algorithms that do the work, but I don’t think that we’re anywhere near a computer that’s going to be self-aware and teach itself to design something.
Todd Tuthill: That’s the machine learning part, and that’s what’s so critical about coming up with a way to harness proprietary data from our customers in a proprietary data lake that they can use in secure ways to take their IP, combine it with these sophisticated design tools, and allow these tools to do things generatively. And that’s really where I see the future going and some of the very high-level sophisticated use cases that I see in aerospace and defense in the not-too-distant future.
Patty Russo: Thank you both again for such great contributions in this episode, but we do have to wrap it up for now. And thank you to our listeners for joining us. We hope this was just as intriguing as the last, and that you’ve learned even a little bit more on this deep dive into AI in digital transformation maturity. We’ll pick it up again next time. I’m Patty Russo, and I look forward to having you back on the next episode of Talking Aerospace Today.
Siemens Digital Industries Software helps organizations of all sizes digitally transform using software, hardware and services from the Siemens Xcelerator business platform. Siemens’ software and the comprehensive digital twin enable companies to optimize their design, engineering and manufacturing processes to turn today’s ideas into the sustainable products of the future. From chips to entire systems, from product to process, across all industries. Siemens Digital Industries Software – Accelerating transformation.