Navigating the challenges of developing ADAS and autonomous systems
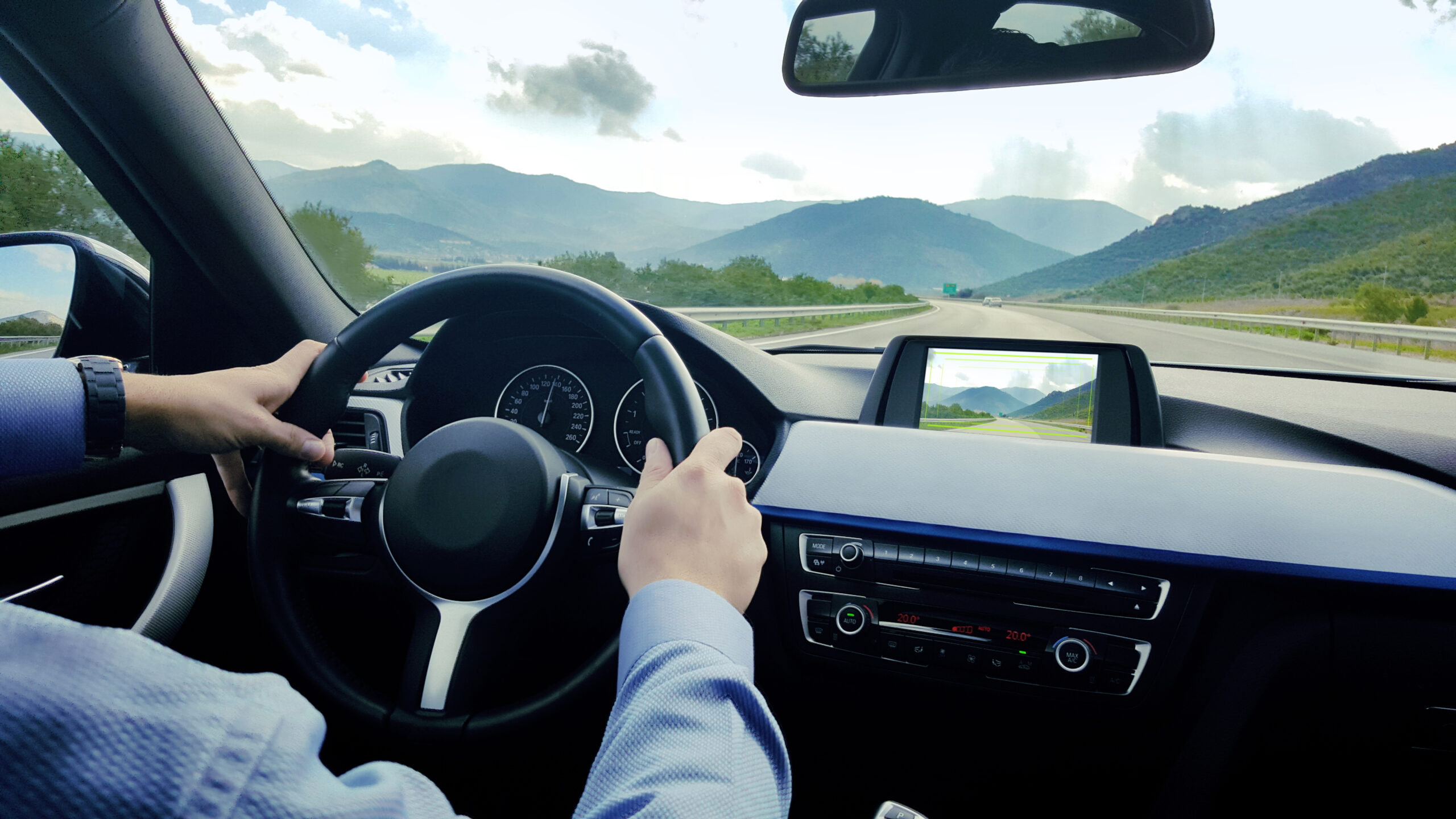
As autonomous driving technology continues to evolve, we’re gradually inching towards a future where we may find ourselves with less need to be hands-on when it comes to our daily drives. Though, before we can indulge in completely carefree commutes, there are numerous challenges that need to be worked out to ensure vehicles are prepared to operate safely and reliably—even amidst unexpected events.
In a recent podcast, Nand Kochhar, VP of Automotive and Transportation Industries at Siemens Digital Industries Software, and Gwen Van Vugt, Director of Autonomy at Siemens Digital Industries Software discussed some of the pressures and obstacles companies face in developing secure, dependable and scalable advanced driver assistance systems (ADAS) and autonomous driving systems. From navigating roadblocks to unlocking data-driven solutions, we’ll take a closer look at some of the core challenges companies need to overcome to attain a future steered by technology.
ADAS: One-size doesn’t fit all
The evolution of ADAS from a novelty to a standard offering across vehicle portfolios requires optimizing and standardizing hardware and corresponding software. This includes a strategic emphasis on reusability, functionality and cost-effectiveness. And in a landscape where skilled software professionals are in high demand but in short supply—aiming for efficiency is vital. So, the question is, what is the best way for OEMs to scale?
Additionally, the expansion of ADAS systems into a broader spectrum of vehicles, reaching not only luxury cars, but also entry level vehicles and emerging markets in locations like Vietnam, India and Indonesia introduces another new challenge: regionalization. Different regions have unique traffic behaviors and environmental conditions that require tailored adaptations for optimal performance.
For example, a system that may work well on German roads might not work in the vastly different road infrastructure and traffic scenarios of somewhere like Vietnam. This necessitates scaling these autonomous and ADAS systems efficiently and ensuring they maintain a level of adaptability. OEMs must strike the right balance between standardization and customization to safely deploy their autonomous systems at scale.
Data drives solutions
One solution that could potentially solve the scalability and operational design domain (ODD) issues lies in the data. In particular, the need for more robust data analysis that can account for a broader range of scenarios that ADAS and autonomous systems could potentially encounter on the roads. These scenarios not only encompass traffic situations, but also environmental conditions like heavy snow or rain in various parts of the world and even vehicle to everything (V2X) messages in the case of connected driving.
When entering new markets, OEMs gather extensive real-world data to grasp traffic patterns and adapt their systems accordingly, focusing on identifying critical scenarios unique to each region. While real-world testing can be beneficial in uncontrolled environments, relying solely on real-world data has limitations—you can only address what’s already been encountered and it can be both time consuming and costly. Manufacturers need to uncover the unknown critical scenarios which have not yet been experienced.
This is where synthetic data plays an increasingly important role. Simulations help create scenarios that may not have been observed but are likely to occur. Moreover, any scenario can be tested via simulation, allowing teams to perform rapid repeat tests in critical scenarios. By combining real-world and synthetic data, companies can develop ADAS and self-driving systems that are prepared to deal with nearly every scenario, reducing the residual risk associated with autonomous driving.
Finding the unknown scenarios
In the quest to achieve optimal safety and reliability in AV systems, Siemens has developed mathematical methods referred to as “critical scenario creations”. This innovative approach involves using the blueprint of an intersection, for example, and determines the most likely and hazardous scenarios based on the intersection’s layout and traffic behavior.
Furthermore, a crucial element of this process is the introduction of a metric for novelty. This metric enables the system to identify scenarios which have already been encountered and adds new ones to a database, helping engineers further account for dangerous scenarios. This comprehensive approach to AV and ADAS system design and testing helps reduce the risks associated with autonomous vehicles despite varying conditions across the world.
An autonomous future is approaching
While completely autonomous driving eludes us for now, diligent efforts are being made to improve the performance and reliability of autonomous driving. The use of in-depth data analysis and simulations to augment real-world insights results in a more refined and thorough understanding of the potential hazards and conditions that can occur out on the open road.
By embracing innovative vehicle simulations and scenario creation techniques, companies can make autonomous vehicles more reliable, inching closer to a future where autonomous vehicles are an essential part of everyday life.
Siemens Digital Industries Software helps organizations of all sizes digitally transform using software, hardware and services from the Siemens Xcelerator business platform. Siemens’ software and the comprehensive digital twin enable companies to optimize their design, engineering and manufacturing processes to turn today’s ideas into the sustainable products of the future. From chips to entire systems, from product to process, across all industries. Siemens Digital Industries Software – Accelerating transformation.