Understanding Engineering Intent with AI Part 2 – Transcript
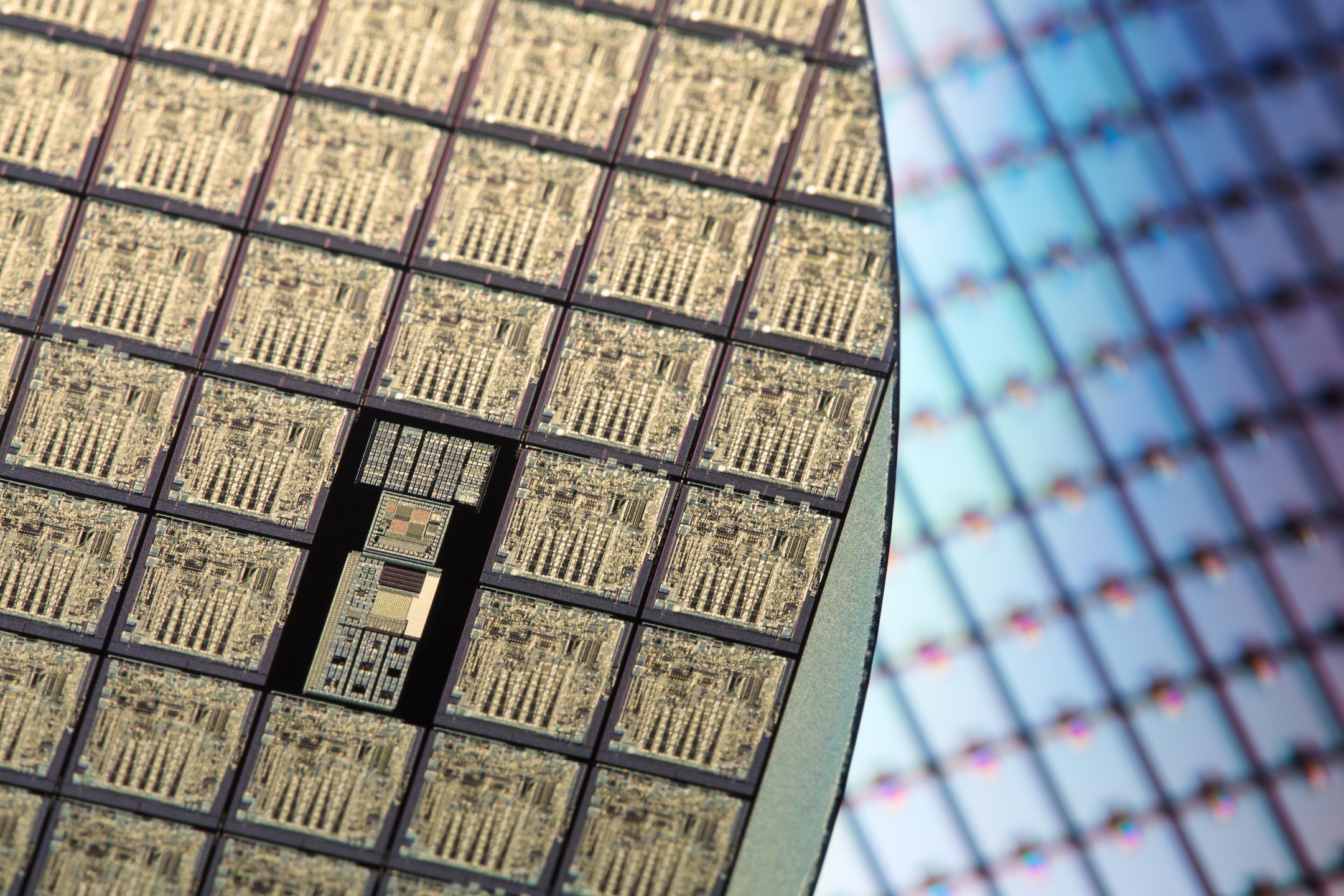
In a recent podcast, Richard Barnett, CMO for Supplyframe, discussed the ways AI is proving vital in understanding the global, interconnected component supply chain of the electronics industry and the value that provides. Check out a full transcript of our conversation below, or listen to the episode here.
Spencer: Hello and welcome to the AI Spectrum podcast. I’m your host, Spencer Acain. In this series, we talk to experts all across Siemens about a wide range of AI topics and how they applied to different technologies.
Today I’m joined once again by Richard Barnett, Chief Marketing Officer for Supplyframe, to continue our conversation on the way Supplyframe is applying AI in the electronics industry. So continuing where we left off from last time, you were talking about looking at lots of data and data sources to make predictions in the three-month timeframe or even up to 18 months ahead. Is that something you could do without AI? Or, I mean, looking at all this data, is it something that you have to be using these AI models for?
Richard: Yeah, it’s a great question. I mean, I think there’s thin slicing elements that you can look at as a data series or as a pattern or as a statistical kind of modeling, but it doesn’t give you the whole picture.
So what traditionally has been done is financial analysts, for example, will look at the build a book ratio of distributors as a bellwether of what’s happening in the industry. Because if there’s more ordering volume and commitment for orders that have not been fulfilled to distributors, well that’s a healthy sign of demand. And it gives you a pretty good view of whether the market’s going through an upturn, or a downturn, or an adjustment. And similarly, in certain electronics categories, if you look at memory like DRAM, they’re very notoriously seasonal or cyclical. They go through major technology shifts or standard shifts, et cetera. Will kind of create this very bullwhip effect where you have peak demand and maybe higher costs and it ramps back down and the next wave, the next technology generation is ramping in front of it. And that can be very somewhat predictable if you just look at sales, for example and sales history.
But what we find in the electronics value chain in particular is that it’s those little thin slices of just looking at past sales or financial measures, doesn’t give you the picture of where the market’s going to going to be at all. And a lot of companies had it wrong at the beginning of COVID and they still have it wrong. And there’s some inherent dynamics which are complex in the electronics industry sort of broadly because the nature of the supply chains are multi-tier. So if you look at the automotive industry, you go back to COVID in kind of the early impact of, “Oh my gosh, we’re all working from home. We don’t really know when this is going to end.” Students are studying at home and consumer spending changes dramatically. No one wants to buy a car. You’re not driving anywhere, but you definitely need to figure out how to stock the home up, how to accommodate everyone living, working in the same place. Kids working from studying at home, professionals working from home, et cetera. Massive demand shift in consumer demand.
What happened in automotive is they just decommitted their vehicle forecast volumes and ramped down production. But the bullwhip effect in automotive is that really electronics sort of design and inventory was not being held by the OEMs like the big brands, but by their tier one or tier two suppliers. And the automotive industry for years was focused on optimizing and reducing and leaning out the supply chain where everything was just in time, just in sequence. OEMs didn’t want to hold any inventory. They wanted everything arriving, kind of ideally on the manufacturing line for the final assembly of a truck or a car just as needed. So just very efficient operations.
The problem with that model that developed over 80 years is it pushed the inventory liability and the risk of holding or buffering volatility of demand or supply upstream. And as soon as they decommitted demand, all of their tier one, tier two suppliers had no incentive, no commitment to hold or continue to buy what they might have been seeing as really valuable electronic components and chips and everything that’s needed to go into a car, which oftentimes can be up to two to 3000 individual components and a hundred different sort of subsystem boards. They’re controlling the modern vehicle, whether it’s entertainment or visioning or auto control systems, et cetera. All of that complexity was really upstream.
And what happened is they decommitted demand. All that supply was decommitted by those tier one, tier two suppliers. And a lot of the same components are used in other things that you wouldn’t even suspect. Same components that go into the phone, like ceramic capacitors go into the car. Same processors that sometimes go into gaming consoles go into the car, the automotive vehicle. So all of that freed up and all got consumed by other industries and adjacencies that consume the same components.
All of this meant that when you’re trying to forecast or look at a very complex multi-mode system that has multiple discreet downstream demand segments, but share across multiple tiers of suppliers the same common components or supply base, it’s incredibly difficult to make sense of that because it’s not a linear and it’s not a directly linked or allocated relationship between demand and supply across these tiers.
And then you add in engineering behavior, which is designing activity and behavior, which you’re not going to see from financial analysts. You’re not going to see that behavior unless you’re, like we just talked about, aggregating it, analyzing it, making sense of it across a community of engineers. And so what we saw in the market was you absolutely need to apply AI to understand a holistic view.
And critically from a business decision making perspective, there’s been an over reliance on a limited set of data that’s very near term or overly biased around financial performance and not really an indicative view of predictive insights. And it doesn’t give you a complete picture.
So for example, in the automotive industry, we saw in real time what was happening upstream. We saw that in automotive ICs supply lead times started extending. We saw inventory going down. We saw distributors pushing their posted lead times out about six months ahead of any automotive OEM, realizing that there was a potential problem. By the time it got to December of 2020, almost every major OEM globally started announcing production shutdowns. Shocking sort of surprises.
Within three weeks, they had no idea they weren’t getting available supply. And then they were shutting down plants. Which is incredibly expensive impact in automotive industry, because it’s not just you’re not going to be producing cars and vehicles. I mean, you’re shutting down a billion dollar plant and all the associated costs, the ripple effect is massive. A real cost to ramp down, ramp up, et cetera. And they were absolutely flatfooted.
We went back across all of our data sets and said, “When’s the earliest point in time we could have predicted this impact?” And we went back to basically April. And said, “That was the first or early warning.” May became clear. June was super clear. We could see it in real time across our network.
And it gave us a really interesting sort of very much in service to the moment sort of push to go deeper in how do we reveal and understand and build a broader predictive model that will help companies in key industries get ahead and not be so flatfooted. And that’s really what we’ve been focused on for the last two to three years, has been constantly improving our modeling, prediction modeling, our ability to scale these insights. Adding new forms of insights even outside of our own network through partners to just get a broader and bigger view because we think it’s so critical to get this right.
And it absolutely requires AI to both distill, correlate, and discover new forms of insights. But then also have the level of accuracy in prediction models that are very focused around specific use cases, or specific decisions that companies need to make. And sometimes that’s in engineering and design early on. Sometimes it’s in whether the timing for when they should negotiate with suppliers in the market based on costs or lead time. Or, how they should manage their strategic sort of risk pooling ideas.
Should they start partnering with distributors or contract manufacturers to buffer strategic inventory? And how much risk of that is there in the market? Is it a three-month transition issue? Is it a six-month issue? All of these are the critical business decisions that business leaders now need to make. And they need this predictive insights and they need insights that are very holistic, not just thin cut. Because they’re going to get it wrong if they’re going to rely upon the same insights that they were using say before.
And this kind of rise of intelligence in electronics is something we’re on the front lines of. But I got to tell you that most industries and key companies that we work with are still very much struggling around how to get outside in intelligence in this new form of AI derived intelligence into the hands of all the folks in their teams that really need it. And it’s really interesting set of challenges and opportunities right now.
Spencer: But that’s all the time we have for this episode. Once again, I have been your host, Spencer Acain, joined by Richard Barnett on the AI Spectrum Podcast. Tune in next time as Richard and I continue our talk on the applications of AI predictive changes in supply chains.
Siemens Digital Industries Software helps organizations of all sizes digitally transform using software, hardware and services from the Siemens Xcelerator business platform. Siemens’ software and the comprehensive digital twin enable companies to optimize their design, engineering and manufacturing processes to turn today’s ideas into the sustainable products of the future. From chips to entire systems, from product to process, across all industries. Siemens Digital Industries Software – Accelerating transformation.