Chasing the black swan: How AI can predict random events
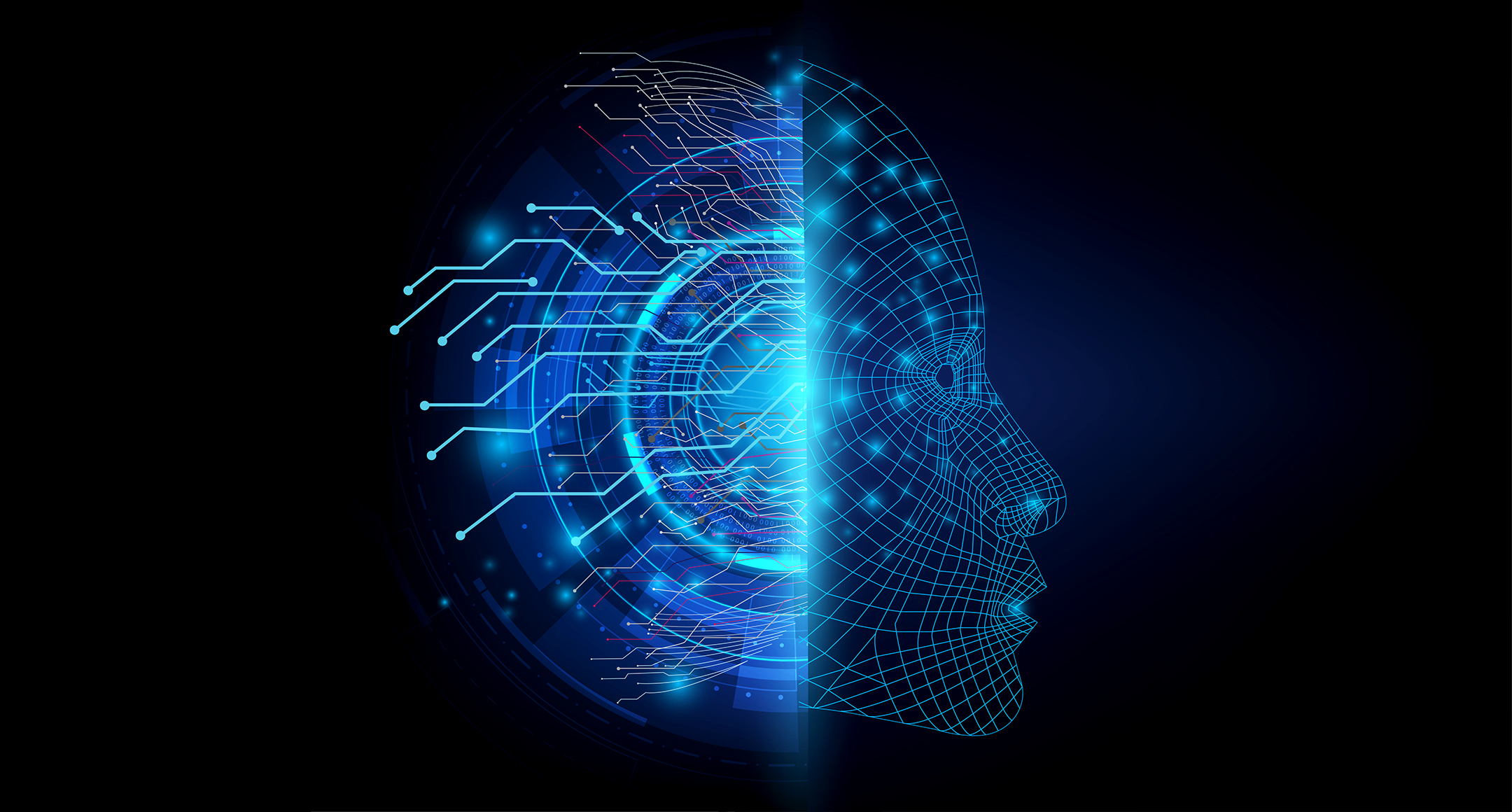
When it comes to predictions, AI reigns supreme. Whether it is collating millions of data points to accurately predict global economic trends, making suggestions on what to buy next at a major retailer or when to perform maintenance on a machine. What all these topics have in common is the volume of data available for the training processes, something that many valuable areas of interest are lacking. There are some events which to an observer appear random or spontaneous, arising quickly with little or no warning and having a huge impact on the world around them. On a large scale, this could be an earthquake or on a smaller scale, the spontaneous failure of a part in an assembly line. Good or bad, conventional wisdom says there should be no way to predict such events due to lack of data but with recent advances in AI, it may be possible after all.
A term coined to describe these type of events is the ‘black swan’, referring to an event that comes as a surprise to the observer, has a major effect, and then is rationalized in hindsight. Black swan events are rare and unpredictable by nature but not inherently good or bad, although in the context of AI prediction, it may be more valuable to focus on finding events with a negative outcome. On a smaller scale black swan events aren’t so different from the small, random events that happen every day across every level of society. While many of these events are harmless or even beneficial, many more range from mildly disruptive to downright disastrous.
Forecasting these type of events, which happen infrequently with little or no warning, has been impossible using predictive models because there is simply not enough data available. Conventional AI methods also fall prey to this issue, where the small scattering of available data is insufficient to train an accurate model. However, by applying a statistical approach instead of a big data one, it may be possible for AI to overcome this shortcoming and provide meaningful predictions despite the lack of samples.
Taking a statistical approach
By combining AI with Bayesian reasoning, an approach that applies probability theory to inductive reasoning, it is possible to get the most out of what sparse data is available by choosing only the most vital information and building predictions upon that small data set. Using this method, it’s possible to predict events with a surprising degree of accuracy that traditionally had too little data to build a model around. In a practical sense, this technique can be used to forecast everything from the likelihood of a hurricane forming, and the effects that will have on a supply chain, to the chances of a stress fracture forming in the hull of a ship.
Going forward, developing a technique to “predict the unpredictable” could be an important tool in a number of areas. Here are a few examples: it could be used to combating the effects of a global pandemic on supply chains with its ability to predict sudden spikes in infection. It could also predict sudden, catastrophic failures of equipment that would be difficult or impossible to detect with a traditional maintenance schema – potentially saving human lives. Although there are limits to what these type of models can predict since some fields still have too limited available data to pick from even with Bayesian reasoning as well as an incomplete models to build predictions on. Even so, the potential benefits for areas which do have enough data cannot be overstated.
Good luck or bad, act of god, or black swan, the world is full of seemingly random events that can anywhere from a minor to profound effect on the way we live our lives. While a little uncertainty makes life exciting, in the globalized world of manufacturing having a firm understanding of future possibilities and potential outcomes is vital and as little as possible should be left to chance. Through the power of AI, building a framework to understand these seemingly random occurrences can be made reality in a way previously unimagined. AI is powerful tool gaining traction in many parts of society and, as it continues to develop, will grow to assist us in ways we can scarcely imagine, helping us understand the world we live in as never before.
Siemens Digital Industries Software helps organizations of all sizes digitally transform using software, hardware and services from the Siemens Xcelerator business platform. Siemens’ software and the comprehensive digital twin enable companies to optimize their design, engineering and manufacturing processes to turn today’s ideas into the sustainable products of the future. From chips to entire systems, from product to process, across all industries. Siemens Digital Industries Software – Accelerating transformation.