AI Spectrum – Simplifying Simulation with AI Technology Part 3 – Transcript
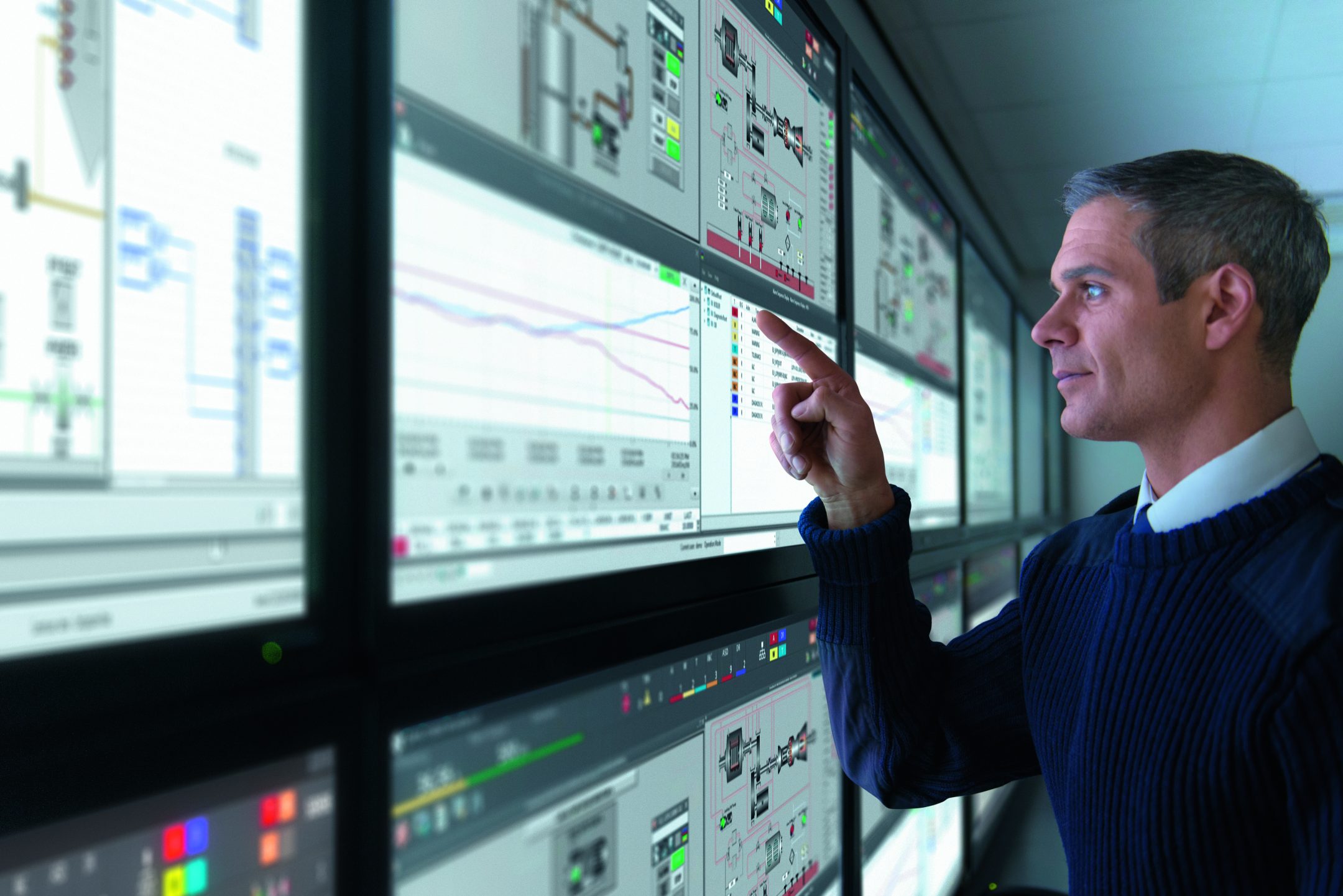
I recently had the opportunity to speak again with Dr. Justin Hodges about the ways Simcenter is implementing AI to improve the interconnections of their software, and where he sees AI going in the future. Check out the full podcast here, or keep reading for a transcript of our talk.
Spencer Acain: Hello and welcome to the AI Spectrum podcast. I’m your host, Spencer Acain. In this series, we explore a wide range of artificial intelligence topics from all across Siemens and how they are applied to different technologies. Today I am once again joined by Doctor Justin Hodges, an AI/ML technical specialist and product manager for Simcenter. Welcome, Justin, it’s good to have you back.
Justin Hodges: Hi Spencer. It’s my pleasure. Always a pleasure to talk about AI.
Spencer Acain: Great. So, before we jump right in, can you give our audience a little bit of a refresher on your background and your work at Siemens?
Justin Hodges: Yeah, I’d love to. I’ve had the distinct pleasure of working at a lot of different parts within Siemens in the Healthineers group and the Energy Group and in this case, the DISW group related to software. So, my background basically with Siemens starts at the beginning of my master’s degree where we worked for Siemens Energy for their design correlations in turbomachinery. So. my background and research focus at the time was heat transfer turbulence, fluid mechanics, CFD,physical measurement, stuff like that. And then I fell in love with AI in 2017 with my first professional experience in the Healthcare Group where I was doing CFD and imaging combined into machine learning models and things like that for better diagnosis of COPD lung condition, and then since then, I pretty much decided that I wanted to infuse machine learning into my existing research area and focus for as long as possible and develop that way as a professional. So since then I started full time at the conclusion of my PhD at Siemens DISW, and then I’ve been working until now, you know, to still do the same thing. And including machine learning into CAE as much as possible. And I work within the product management group for our portfolio of products at STS.
Spencer Acain: Sounds fascinating. It looks like you’ve had a very broad depth of experience with AI in whole huge number of different fields.
Justin Hodges: Yeah, it’s part of the richness for sure. I really enjoyed it and I love what I’m doing now. So always happy to talk about it.
Spencer Acain: Great. Well, to start things off then and talk about AI some, how do you see the increased adoption of AI which, you know, enables the kind of a new level of interconnected technology affecting the design and simulation industry at large?
Justin Hodges: Yeah, that’s a good question, and there’s a lot of subjective parts in there, but you know, take it with a grain of salt. I think some elements there are probably on the right track, but you know, I think I’ll start with probably at some point with GPU technology picking up and really enabling these parallel calculations for the core AI and ML research groups and you know that those huge advancements were made in, in the algorithms and the technology and are still happening today. But somewhere, I don’t know when you would say 2017, 2018, 2019, somewhere in there was also a huge pickup in the cross pollination, if you want to call it that, where you take the advancement of machine learning and then you translate them into other applications in industries and disciplines. So that would be where we’re at today in terms of CAE and aerodynamics and acoustics and autonomous driving. All these other areas outside of just core algorithm development, research and improvement in the techniques and things like that and so since then, and that 17 to 19 range you know, you see all these types of different methods being infused into all the all the industries for automotive and aerospace and marine and CPI and basically all over the place. And it’s a really fun place to be as a commercial provider for software considering that those are all of our customers and industries that were focused on with the huge breadth and the PLM like typical for the different products that we produce but a huge number, I guess to drive beyond just our engineering space of CAE, you know more broadly just the global AI market, you know you see that there are global market estimates of 10s of billions of dollars in terms of how large the global AI market is.
Justin Hodges: Now again I emphasize that we’re just a sliver of that right in terms of CAE, but you know that number is growing and we’re seeing that on average from a business perspective, those markets are growing with compound growth rates of in the 20s, 30s and in some institutions estimate 40 percentages. So that 20 to 40% range in terms of the growth, which is an enormous amount of quick growth, yeah, so there’s a huge thrust on that side and some publication related numbers in terms of academia and that sort of thing, because I can also indicate kind of where everything’s moving. You know, if you take like, a society like, you know, the American Society of Mechanical Engineers, a tried and true sort of like, very familiar organization, right, they’re on the order of, like 500,000 publications since inception in like, the late 1800s, I think and then if you compare for scale in 2019 alone, all disciplines, all industries, etcetera, you know the amount of publications on machine learning globally was 100,000 and basically.
Spencer Acain: Wow, that’s incredible.
Justin Hodges: Yeah, right? So really, there’s a thrust on the industry side on the business. And I guess you could call it like investment side of things to see how, you know, technologies are affecting economies and markets and things like that. And then as well in academic research side. So it still seems very much full steam ahead.
Spencer Acain: Well, yeah. I mean, it sounds like you’ve got AI just, it’s kind of in every starting to kind of flow into every industry just kind of connect with everything and there’s some degree of cross pollination where technique from one discipline is helping someplace else and being applied to something else. Is that something you can talk about? More like that idea of kind of cross pollination? Interconnectedness. I know, Obviously we talked about tools individually gaining AI and ML as an ability or as a benefit, but how about that cross pollination, that interconnectedness, especially with the use of AI with use of ML for that kind of technique that kind of interconnectedness itself.
Justin Hodges: Yeah, 2 great points. On one front, cross pollination so, you know, maybe like the silly sort of way that’s full of fantasy that you’d read it like a book or something is, you know, the philosopher or scientist is like having his or her lunch underneath the tree and then realizes, Oh my gosh, the branching patterns and way to distribute nutrients through the tree and the tree limbs. Looks like the lung, you know, trachea in the way that the trachea branches and maybe oxygen flowing the same way, and you’re inspired from something completely separate, right? But that’s just kind of the silly example. But in terms of cross pollination, in terms of in terms of techniques, I guess there’s a lot of structure in the algorithms and then the approaches and machine learning. You know the original motivation I guess you could say was like to imitate like modalities and mechanisms with our own nervous system and brain and things like that and we’ve deviated from that, of course, at some points. But you know there’s structure and then it’s kind of like the laws of physics. So, you know, we’re used to having Navier-Stokes. We’re used to boundary layers and different regimes for viscous or inviscid. And we have this like structured way of thinking and I think a good way of cross pollination is sometimes when that structured way of thinking is, you know, used in a machine learning framework. And then we learn new ways to structure our correlations or our design tradeoffs and stuff like that.
Justin Hodges: Now in terms of interconnectedness, I think about that from a software provider point of view and in our case, you know, for me a portfolio of products that I’m interested in putting machine learning into. You know, I think there has to be ultimate connectedness at the end of the day, you know, cause companies don’t just use one software to design like a car or you know a piece of hardware or something, right? They’re gonna use several to do, you know, thermal, aero, acoustics and things like that and system level beyond just the single component and so I think the good way to approach like this digital executable digital twin mindset is when you have those independent sort of ways to study and simulate and generate results on performance, you know you have them able to share data with one another and it just say like a common platform and that is the ideal way that a machine learning model can be positioned between the two or several products, and take that information and make meaningful insights and inquiries whenever people want to make prediction and so okay, that sounds all well and good and esoteric, but specifically we have some examples of that but you know, for example, you know you can do CFD in like Simcenter STAR-CCM+ plus and you get these really detailed 3D flow field type results. But of course there’s plenty of people in the world that don’t really focus on that. They focus on system level simulation. So, the thing that they may simulate and CFD is a component, but they’re really looking at that component amongst a bunch of other ones in a system and nominally, you know you’ll have better accuracy if you do a detailed simulation of 1 component, then if you use like a lower fidelity approach or like a correlation or like some empirical laws as the component like sort of you know predicting for a single component in a system like Amesim.
Justin Hodges: A system simulation software like Amesim so in STAR-CCM+ for example you can export these results in models that you create that are basically surrogates that summarize the performance and STAR-CCM+ and you can export those and import them into things like Amesim or ROM builder which are two other products that we have at Simcenter and so in that case that’s a really good way to have interconnectedness between different disciplines, different software and essentially the result is you can do really detailed simulation and incorporate that into a larger global system model and not suffer. You know, the usual consequences and penalties to accuracy when you do system level simulations and so those are some. Those are some examples
Spencer Acain: So, these models you’re exporting are these like machine learning models or AI models that you’re taking out of STAR-CCM+ and putting into Amesim or ROM builder?
Justin Hodges: Yeah, that’s right. That’s right. So, you can make these surrogates that can be based on neural networks that can be based on right response surface models, linear regression, some more common things. Five years ago, the machine learning, so I think about like, transfer functions, which are another thing that most people have taken in, like a feedback controls class or feedback design class in undergrad coursework where you have an input signal, you have an output signal and you have some stuff in between and essentially, you know in feedback control sort of classes. You learn that, you know, certain circuit diagrams, you know, allow, like could exist. And then there’s correlations and equations to calculate what the output signal is based on the input. And in this case, it’s kind of the same framework where I run CFD result to say get like a drag value, and I know that I have certain independent variables that influence that, like the spacing of some geometry, the diameter of some piece, the Reynolds number and stuff like that. And then it’s kind of like the analogous representation of this feedback controls example I’m giving as a metaphor because you have an output signal drag and you have input signal like independent variables and so, yeah, you have these functional mockup units FMUs you have different ways to export that relationship from your simulations and then use that relationship in other places. And so the goal is right now we have ways of different software like STAR-CCM+ and ROM builder and Amesim and these things can communicate. But of course, the goal is more and more communication and easy sharing of results and models based on results back and forth so that other software can use it. And that’s, you know, that’s a big goal of SIM Center and that’s a huge tenant for machine learning.
Spencer Acain: Well, that sounds extremely valuable. So, it sounds like it would even kind of be a bit of a shift then in the way that you would be using these tools because obviously now you have access to higher quality simulation, better fidelity, all of that kind of stuff in tools that historically wouldn’t have been able to get access to that easily. So, I guess what I’m kind of asking here is how do you see that changing the design and simulation process like what kind of effect do you see this level of integration, this level of accuracy being connected to each other having?
Justin Hodges: Yeah, it’s a very profound sort of question to ask and implication to look at and I think you can look at it at a very high level, right? You know a lot of people like watching the Super Bowl last year in the Super Bowl, probably 30 commercials from different automotive OEM’s on electric vehicles and say, you know, maybe half of them today at the time didn’t even have any single electric vehicle out in the market. So, it shows that a huge urgency is to shorten design cycles and to produce designs that you’re gonna go with and manufacture faster and so you know, obviously you wanna save on cost and obviously you wanna have safe designs. But I think a huge one as well is faster time to market
And so when you have these models. You can summarize these relationships of the design inputs and the design outputs, you know you can take advantage of transfer learning and things like that where you know, maybe I design a car in 2020 and then 2021. I design a slightly different car, it’s a huge head start on your schedule. If you can summarize those inputs and outputs from your 2020 exercise into the next year when you design the similar but different car. Obviously, if to do that with some intelligence and sort of intentional setup, but nevertheless like those things are becoming possible and used in practice and also I think it makes people sleep better at night because the design process in a lot of industries is really serial. You know, like if I wanna design, I don’t know a turbine or something, you know, I start with a bunch of low fidelity simulations that, you know, gradually after I do using software #1. Then I use software #2 software, #3, et cetera and essentially the complexity gets up every time and therefore so does the runtime and computational expense and maybe when the aerodynamics team is done, they pass it to the next discipline like the mechanical and the thermal teams. When they’re done, they pass it to the next team. So, this is an inherently a really serial process and yeah, I think it’s unfortunately common across all industries that someone towards the end of a long, you know, two or three-year design cycle when they’re doing this things in serial they realize oh no, this isn’t gonna work and they start back backing up to the previous team and before they know it they have to kind of go to the earlier parts of the process to change the design and then propagate it again through the serial chain. You know again and that’s not a good way to do things so. Yeah, and it’s a super practical way to approach things. So, it may sound, you know, like it’s not a good thing to do, but in reality it’s a very practical thing and things have been practically things have done for a while and you know, like all kinds of industries. So, where machine learning can play a role, is imagine you had this information from past designs and current designs from different teams codified into a callable model or a callable sort of program where essentially when I’m doing my aero calculation, I can get quick inquiries really fast from other teams and their models that they build over time. So, I can get a sense on how attractive and suitable these designs for other teams criteria, you know, that I may not have much familiarity in. So, it really helps make this more of a parallel process, you know, one of the huge trends in the market for AI is system and database management and knowledge management. So that’s essentially you have these large companies and medium sized companies is a network of information from different people, different countries, different teams, different software, different disciplines and machine learning can really play a role there at providing useful summaries and suggestions and call outs when you’re doing this design process from that information in summarized way.
Spencer Acain: That sounds like it would be extremely useful to kind of parallelize the design process and that you’re really taking these ML and these AI models and kind of capturing the accumulated design experience and the knowledge that year by year these teams are building up and that is kind of encapsulated in these designs that they are creating each year. And that is that, is that accurate, would you say that?
Justin Hodges: Yeah, that’s the ambition. And that’s partly why I open with you know, stating that my background is something Newton would be happy with. Right? Physics, thermodynamics, math, engineering and mechanical aerospace. Because I realized that those principles are so powerful. So, I’m not trying to say right that way. You throw those things away or that we make Newton very unhappy by just summarizing things mathematically and then forgetting about just treating everything as a black box. So that’s the ambition. Nothing’s perfect, there’s always gonna be things you have to always gonna be gotchas when you try to do this and you’re gonna have to do this, you know, in a well-studied way, right to make sure that it works properly, but there’s definite value that can be there and I’ve seen it. So, I think that’s a that’s a big trend right now. Like you said, this impact on the on the delivery and project schedules and stuff like that.
Spencer Acain: Thank you, Justin, for that great answer, but unfortunately, we are out of time for today. Once again, I have been your host, Spencer Acain, and this is the AI Spectrum podcast. Please tune in for the next episode where we will be continuing this discussion.
Siemens Digital Industries Software helps organizations of all sizes digitally transform using software, hardware and services from the Siemens Xcelerator business platform. Siemens’ software and the comprehensive digital twin enable companies to optimize their design, engineering and manufacturing processes to turn today’s ideas into the sustainable products of the future. From chips to entire systems, from product to process, across all industries. Siemens Digital Industries Software – Accelerating transformation.