Predicting fatigue in 3D printed parts
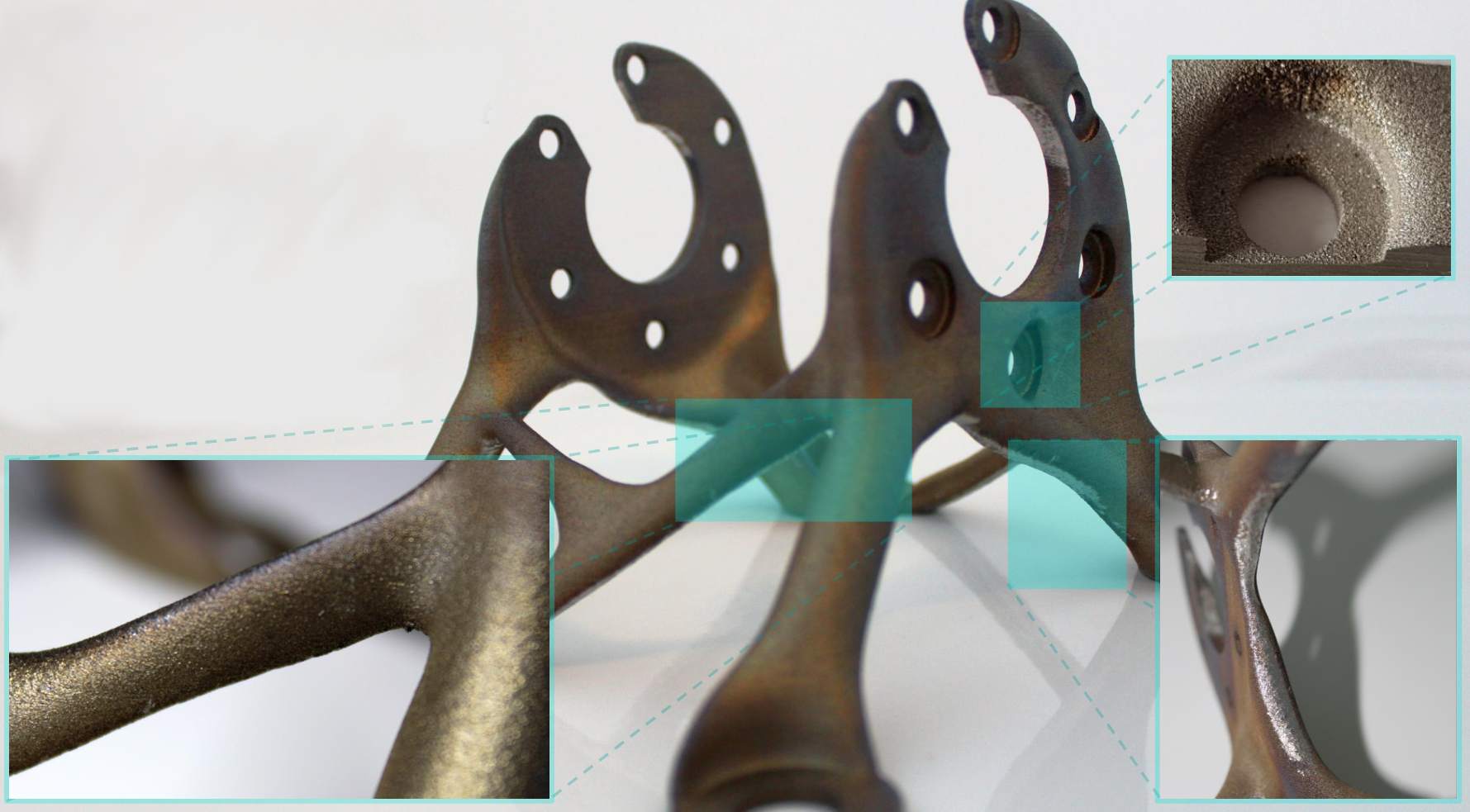
3D printing and additive manufacturing (AM) come with many advantages, geometries can be manufactured that were once impossible, adding complexity to a component is a relatively free operation, and for low volume manufacturing there is no direct competition from other methods. But the printing process brings along a problem, how to define a structure with such high variability not only in the macrostructures but the microstructures as well. That variation is critical in identifying the fatigue performance of a component, because while a loading simulation will uncover flaws in the structure overall fatigue simulations rely on a time axis – how long will a part hold? How many loading cycles can it withstand? The goal is to find the tiny defect that could grow within the part until a failure occurs.
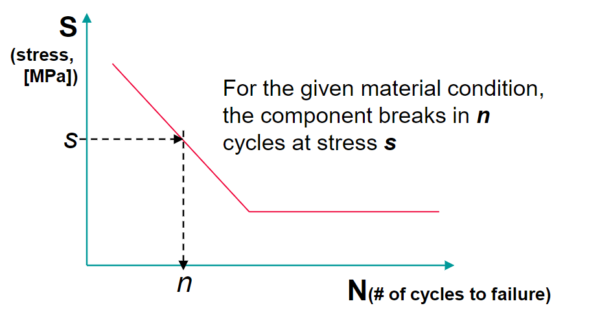
Finding that answer is profoundly difficult, if not impossible, using traditional methods on the newer 3D printing process. Of the three major drivers of fatigue failure – geometry, loading, and material – one is still a moving variable. Material conditions are very difficult to qualify for AM components as the result will not likely retain the same material properties as a machined component would. Surface roughness, defects, and microstructure arrangements all impact the fatigue performance of a component. These would traditionally be combined into an SN Curve which plots the number of times (N) that a component can be loaded at a given stress (S) before failure. Because of the variations in surface roughness or grain boundaries there is no way to calculate an SN curve for additive components, instead it requires empirical data and a connection between the process, the material, and the part performance to understand the fatigue performance.
Without machine learning, that epiphany would mean absolutely nothing because every part would have to be tested physically and dramatically increase the time to manufacture and cost of printing which negate many of the initial benefits. Instead the machine learning database is trained with a limited set of experimental data. Test specimen were limited to LPBF Ti6Al4V and SS316L at a vareiety of different volumes and surface conditions. These samples were then tested with static loading, HCF, multi-axial, cyclic plasticity, and compact tension while measuring surface conditions, material density, and porosity. And for good measure the samples were inspected with metallography and CT-scans to look at the internal and micro- structures within the materials.
The data collected from the testing enabled the model to learn how individual parameters affect part lifetimes along with some other valuable skills. The number of test samples needed can be minimized to represent local conditions of a part, the system can predict fatigue life for a combination of factors outside of the what’s reproducible with test samples, and it can extrapolate beyond the test data. This system delivers “best guesses” to results and should be taken with a grain of salt in situations with scarce data, but the method is far more performant than other methods involving neural networks. And if a data set is not up-to-snuff, different data can be added to enrich the data set for the application through prior knowledge, more testing, finite element simulations, and even empirical rules from literature or experience. For more in-depth understanding of the machine learning model, I’d recommend watching the full webinar or for a faster rundown, reading what Spencer Acain has to say on these results.
Additive manufacturing is a big driver of change in the world of product development, there are so many opportunities for improvement and efficiency, but there are still some variable that need to be pinned down before it is ready for wide-scale adoption in the industry. Fatigue performance is one of the hurdles being solved right now thanks to advancements in the technological capabilities of AM, but mostly due to the improved understanding of the process afforded by digital solutions. Machine learning will be invaluable in defining more of the unknowns surrounding AM and I look forward to seeing what’s going to be solved next.
Siemens Digital Industries Software is driving transformation to enable a digital enterprise where engineering, manufacturing and electronics design meet tomorrow. Xcelerator, the comprehensive and integrated portfolio of software and services from Siemens Digital Industries Software, helps companies of all sizes create and leverage a comprehensive digital twin that provides organizations with new insights, opportunities and levels of automation to drive innovation.
For more information on Siemens Digital Industries Software products and services, visit siemens.com/software or follow us on LinkedIn, Twitter, Facebook and Instagram.
Siemens Digital Industries Software – Where today meets tomorrow