Exploring the impact of AI in CFD – the transcript
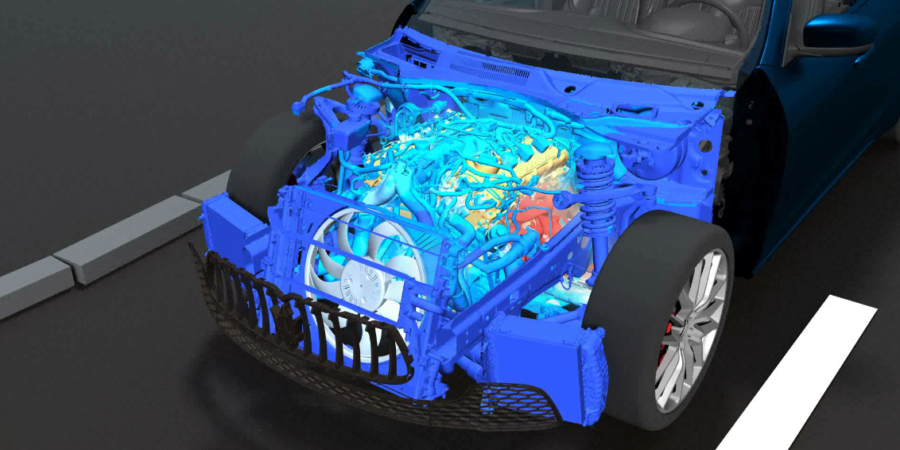
I sat down with our computational fluid dynamics expert to discuss how AI enhances our toolset. The podcast is here. For those that would rather read than listen, here is the transcript for this podcast.
[00:00:10] Thomas Dewey: Welcome to AI Spectrum, where we discuss an entire range of artificial intelligence topics. I’m Thomas Dewey, your host. And today, we explore AI applications within the computational fluid dynamics (CFD) world. Joining us today is Krishna Veeraraghavan, Project Manager at Siemens Digital Industries Software. Welcome, Krishna.
[00:00:32] Krishna Veeraraghavan: Thank you, Tom, for having me in this call. It’s my pleasure.
[00:00:35] Thomas Dewey: We’d like to get started with kind of a personal question and learn a little bit about what inspired your personal interest in AI.
[00:00:43] Krishna Veeraraghavan: It actually all started from my background in simulations and then it evolved into AI, based on my day-to-day work. After completing my MS in Mechanical and Aerospace Engineering from Syracuse, I joined CD-Adapco, which was acquired by Siemens. So, my work in the Simcenter engineering group revolved around solving complex simulation problems and delivering them at a faster turnaround time to the customers, and identifying any automation needs, and developing a workflow for them. So, gradually, as I was working with the customers, I realized how our solutions allowed them to use the Simcenter products to work efficiently on their process, and also to help them to reliably repeat this process with great success. This was very similar to a lot of AI business pitches I keep hearing like, “AI is a good tool which can free up engineers time by taking up a lot of repetitive tasks, and allow them to work on more challenging problems.” So, in a way, this is what we were also indirectly helping the customers. And around 2018-2019, Siemens also had this 2020 vision to enhance the digital twin capabilities and data-driven design decisions for customers. This actually made me curious to learn what AI could do for simulations and how our customers can get benefit from this. To gain some insights, I took some executive education courses from MIT Sloan, which was mainly focused on AI and IoT and on developing business strategies and how we can implement them. Around the same time, I started my MBA from NYU Stern, and the Siemens management team was very supportive of my program. And we decided that to extract more value out of my MBA, we should probably work on an actual business case which aligns with my interest and also with Siemens’ vision. I thought, why not try AI because I was actually very much interested in that. And as a part of this exercise, I started working with different business groups and started connecting with machine learning and predictive analytics teams in Siemens to develop AI solutions for the CFD. And this is how, my journey in AI started in Siemens, and I still feel we have a long way to go here.
[00:03:11] Thomas Dewey: That’s great. Everybody has their own path to AI, I have found. For those in the audience that don’t really know about it, can you provide a brief overview of what computational fluid dynamics is all about?
[00:03:24] Krishna Veeraraghavan: I’m glad you asked this question. Computational Fluid Dynamics – the acronym we use is CFD – is basically a science that uses computers and high computing machines to do numerical analysis based on the input data. The input data can be the boundary condition data, like inlet temperature, velocities, or pressure, which is used to solve and analyze fluid problems. And it can also assist engineers in quantitative predictions of fluid flow phenomena based on three governing laws, which are the conservation of mass, momentum, and energy. During the early days, when I started with CFD, the pitch for a CFD tool was always like CFD is a tool which can do a lot of simulations, and it can help in minimizing the number of experiments. Now, we are talking about we want to combine AI with CFD and start amplifying the goals we originally had for CFD. So, there is a good alignment here.
[00:04:24] Thomas Dewey: I think what kind of confuses some people when they see “fluid” is they don’t understand that airflow is considered a fluid, and that’s a huge aspect. Sounds like you’re trying to apply AI to the CFD flow, so what is the goal for AI and CFD?
[00:04:42] Krishna Veeraraghavan: Our goal is we want to bring humans and machines together – basically, we call it collective intelligence – to augment the CFD, and the design and R&D process for the customers by leveraging AI at various phases of the CFD process, so that we can improve the overall process efficiency of the design process. And also in the simulation, we can try to reduce the cost and turnaround time of the design program for the customers. And also help in improving the efficiency and accuracy of the simulation process.
[00:05:19] Thomas Dewey: Going a little bit deeper, can you describe the types of AI solutions that you’re working on to leverage with CFD?
[00:05:27] Krishna Veeraraghavan: This actually started from the customer discovery process. So, what we did was, initially we brainstormed some ideas, but we wanted to validate those ideas, so we started reaching out to customers. And across each phase of the CFD process, we want to understand their pain points and needs. And also we try to ask them why they use the CFD? What problems they are trying to solve? And we try to understand where AI solutions can be a fit for them.
[00:05:56] Krishna Veeraraghavan: I want to take a step back and talk about how the CFD journey typically looks for the customers. So, the CFD journey starts from the data preparation phase, where some customers move the data from the Engineering BOM to the CFD BOM or the CA BOM, where they do a lot of reduction in the CAD and they prep the CAD for the CFD analysis. And then, the next step is they go for the meshing. Meshing is a discretization process in which the CAD geometry is broken down into thousands or even millions of elements, where we typically store the numerical results. And then you go with the physics setup where you specify what physics models you want to include, depending on the problem you’re trying to solve. And then you run it on high-performance computing machines or your local desktop, depending on the complexity of your simulation. And then you do the post-processing. So, across each phase, the needs for the customers can change.
[00:06:56] Krishna Veeraraghavan: So, then based on this, we shortlisted five key areas where we want to focus. So, those key areas are for predicting flow properties and anomaly detection, and we have something called part detection. And we also want to use something for interpreting the simulation results. For all these things, the key is the simulation database. So, we need to know what to write and what key information we want to extract from the CFD data. We wanted to help customers in this also. Let me also explain to you with some examples for each of these key areas, so you can get some better understanding of what I’m talking about here. Let me start with predicting the flow properties. So, in this scenario, in some cases, the engineers may be interested in the quick visualization of the flow pattern. Assume you are in a meeting, and then during the discussion, you and your team wanted to see, “Okay, if I change my operating condition to a new boundary condition or something, how my results are going to look? And how it’s going to impact my confidence?” Traditionally, what we do is go back, run the CFD for the new boundary condition, and then you arrange a meeting or try to share your results. But with machine learning, we can have an inbuilt surrogate model – you quickly change the operating conditions and it can show the engineers how the results can look like. But this comes from the history of your previous simulation, which we shouldn’t forget because we are training these AI models based on the simulations from the previous results. But for a new operating condition, I can quickly give it in the machine learning model and live, I can show in the meeting how it can quickly visualize the pattern. So, it will help in expediting the designs or the next steps for your design process.
[00:08:58] Krishna Veeraraghavan: In the next scenario, what happens is we use something called as HEEDS, which is used as a design sweep study, where you can try various operating conditions, and then run it with the STAR-CCM+. So, typically it runs 200 or 300 design sweeps, and it tells you what is a good design and what is a bad design, and it gives some insight on how the flow looks like. But with AI and ML, we can weed out the bad design. Before even running into HEEDS, we can trim the number of designs you want to run because AI can quickly say, “Hey, these are all some outliers, and these are all going to be bad.” So, I don’t need to waste my time in running a CFD to do that. This can be a good complementary tool with HEEDS also.
[00:09:50] Krishna Veeraraghavan: Now, I’m going to the next example – the anomaly detection. So, in this case, we want to give all the engineers tools to reliably identify and flag any deviations either from their past designs or from their expected behavior by just looking at the simulation results. So, for example, while visualizing what happens is you run the CFD and then you look at the post-processing plot. And then while looking at the plots, you might sometimes realize that, “Hey, this flow pattern or this value is not what I expected. This is slightly off from what we traditionally see for certain operating conditions.” Then you take your judgment call; you go back and investigate. So, you are able to catch it, but can your team members also with varied skillsets – say, for example, I have a beginner in my group – can he or she catch it reliably? That’s where this anomaly detection can help the engineers to consistently and reliably flag areas where engineers can go, inspect, and identify why such things are happening.
[00:11:03] Krishna Veeraraghavan: Now, I will go to the next one, which is the part preparation. So, this is relevant on the CAD preparation phase, which is the very first phase of a CFD process. What this can do is it can allow engineers to bypass the monotonous and repetitive tasks in the CAD preparation. So, let me explain what those tasks look like, typically. For example, if I do a large thermal management simulation, what happens is, when we download the CAD from the engineering BOM – say, for example, when I download the full vehicle of a car, I might have some 10,000-15,000 components. But then from there, I have to reduce the number of components and I might come down to 6,000-something. So, I might be literally throwing out a lot of bolts, nuts, other junk parts which are really not needed for the simulation. And if I now go from one car line to another car line, I have to do the exact same steps like what I did for this car line. If you observe the pattern, and you see that the engineer ends up doing the same kind of jobs like looking at the parts and then throwing away components that are not needed. So, this is a very repetitive process. AI can help in identifying the part which is typically used in the simulations, so you can then automatically throw away those parts. And then after you identify, we can also add some attributes to the part, like some material properties like density, specific heat, and emissivity, these are all used in the physics setup which helps in running the analysis. So, this will speed up the entire turnaround time of your CFD process.
[00:12:51] Krishna Veeraraghavan: Now, having done this, we thought we could even extend this step to the meshing process because meshing is also a very key step in the CFD. So, what happens is, traditionally, to resolve the features of the geometry, for example, if I’m doing an aerodynamic analysis of an aircraft and if I’m interested in computing the left by drag ratio, I need to resolve all the external features of my aircraft like the nose, leading edge of the wing and the trailing edge of the wing. And wherever there is a shockwave, I need to resolve those areas clearly. So, this comes from an expert who can say for the nose, “I might need a size of 1.25 or 0.5 mm.” Or you do multiple trial-and-error, and then you come up with the best settings. But with the AI, we can identify certain shapes, and then we know that for these shapes, it’s not only adding material properties and helping you up with CAD preparation, we can also add some mesh attributes to it. It can help you in the meshing phase also. It’s like, basically, scaling up the process of this part detection and making it useful for further down the line, also, for the customers.
[00:14:08] Krishna Veeraraghavan: The next example is the interpretation of simulation results. So, in CFD, we generate tons of simulation results. And it takes a lot of time to parse through the simulation data to identify any hidden patterns or any causations to make better judgment calls. It’s a very monotonous job. We thought we can come up with some techniques and can help the engineers to trace it to the component level and see how it behaves. For example, you could be running the CFD for different operating conditions or for different design configurations. And if the same component is used in different designs, you want to see how the component behaves in the baseline design and how it behaves in the modified design. So, if we could trace it, we could create some nice stories for the engineers. If I have some 6,000 parts, I might have parts with different material properties. Even, for example, if you take your car, you have parts which have aluminum and some have steel, or plastic, or rubber – there a lot of different materials. It can identify what are all the top 25 plastic parts, failed parts in my simulation. These things are very useful and it will give you very good insight. It can also help you in another scenario: if Part A failed under certain operating conditions, what are all the other parts that failed along with Part A? What is the relationship of Part A with other parts? We can create some really good stories there. So, I think these are all some examples and scenarios which we identified as something which can be very useful for the customers.
[00:15:49] Thomas Dewey: For those scenarios that you mentioned, can you describe some of the particular AI techniques that you employ?
[00:15:57] Krishna Veeraraghavan: For this, we are relying on machine learning and deep learning techniques. For machine learning, we can use Simple Regression techniques when the output data is pretty much numerical, like, you are interested in predicting a temperature response on a point in space or some output on an outlet. For other scenarios, all you are interested in is the output data, whether it’s a good design or a bad design, then we use something called as Classification techniques, which can identify the shape of the parts from your car and it can say whether it’s a drill or not a drill, or whether this design passes certain criteria or whether this failed. And then the next example is the clustering techniques which I already spoke about which are related to interpretation of simulation results. It can be used for finding relationships between parts and finding any hidden patterns. And then the last one is the deep learning technique, which is a subset of machine learning. So, in this case, we use a technique called as Convolutional Neural Networks which we use for the prediction problems. And here we feed in the input data which can be the images like your post-processing or the temperature control of a particular section, and then the output can also be a temperature control of the same section for any new operating conditions.
[00:17:19] Thomas Dewey: So, those familiar with AI would be very familiar with those concepts. Moving back to your AI-based solutions, what techniques and data are used to train them?
[00:17:30] Krishna Veeraraghavan: This is something really important. Data is very important for AI and ML, which we all should acknowledge. Even in CFD, there is a famous saying, “Garbage in is garbage out.” If I give some garbage boundary conditions, I’m going to get some garbage results. So, similar concepts hold true for AI also. In addition to this, we have “Bias in, bias out.” Customers need to have a clear understanding of what kind of data can be used, and what information they can extract from the data. We can also help them in this AI transformation journey. So, we can help them try to identify what type of data can be generated from their expected output, whatever they are expecting from the AI models. And then the first step you see is the data creation. The data creation can come from any source; it can be from simulation data, which can be even from CFD or any CAE simulations; and it can be from experiments or it can be real-time. At this point, we are focusing on the data source from the CFD to keep it easy. Even from the CFD data, it can be just numerical values in an Excel file, or it can be just contour images, or it can be a 3D image, or it can be a sectional image also. And then after creating the data, the next step is the data preparation – that involves the data reduction. And cleaning of the data – we use feature an engineering technique to extract certain features in the data which we think is very relevant to the AI problem they are trying to solve. And then the last step is the training and testing. So, for this training and testing, we typically use the 80-20% rule. 80% of the data samples we have will be used for training, and 20% of the data samples will be used for testing and validating the AI model.
[00:19:32] Thomas Dewey: Putting that all together, can you share the improved results from using your AI-enabled products on customer projects?
[00:19:40] Krishna Veeraraghavan: I can name a few here. So, some of the customers we know are using the shape reduction techniques for the large thermal VTM simulations. They use it to identify the parts and help them in the data preparation phase. We also know a few customers who are using AI for prediction problems, basically to predict certain flow features at multiple crank angles. The same concept can also be used for predicting any anomalies in the design by identifying the flow structure, for example, soil, or it can be any flow properties like temperature or pressure. So, some customers have started using AI now but not everyone.
[00:20:24] Thomas Dewey: Another kind of angle on this is, do you think that AI has altered the approach that scientists take when they’re trying to solve a problem?
[00:20:32] Krishna Veeraraghavan: This is a good question because this should give the confidence on – to answer this – we are not fundamentally changing the design or R&D approach. What we are planning is to augment the research and the design process for the future. To explain it in a better way, let me take a step back. I’ll try to explain the basic principles of the scientific methods that are well understood and widely used across the world. So, if you think about the scientific methods over centuries, it’s defined as discrete reproducible steps; we generally first ask questions, and then try to make observations, and then based on that, we try to devise some hypotheses, and then we try to conduct experiments. And now, from the experiments, we now use simulations to test and validate the hypotheses. So, all the data on general theories lead to more observation and further research and getting this ball rolling over again and again. The scientific methods, whatever have been used, are very clear. We don’t want to touch that process. All we are trying to do is look for opportunities at each stage of the scientific process where AI can be used, where you can identify which tasks are best for people, and which tasks are best for machines, and how both can work together in certain areas. So, all this is focused only on augmenting the process, whatever they have.
[00:22:01] Thomas Dewey: Kind of looking in our little crystal ball, where do you see the role of AI/ML and simulation going in the future? And what does this mean for engineering roles?
[00:22:11] Krishna Veeraraghavan: For the near term, we think this collective intelligence, where humans and machines working together, will be one of the key competitive advantages for a lot of companies. And it can be a key component or a key tool in their design and R&D process. In general, humans are very good in solving problems but they’re not good with the data. So, machines are very efficient with the data. Since my background is more in CFD, I can share what our Simcenter Engineering Services envision for AI in CFD. AI can be a good automation tool to automate few complex and repetitive steps, like I mentioned in the CAD preparation steps, and it can improve the process efficiency. AI can also be used as a complementary tool with design acceleration tools, like I mentioned in HEEDS. So, it can be for design exploration studies, and it can also be used for conceptual low-fidelity design stages, where you don’t care much about the accuracy; all you care is only about the patterns. AI can be a good fit there. And you can always use CFD for more detailed design and complex physics problems. AI will be built based on multiphysics simulation, so we can no longer stay in silos. I cannot say that I am a CFD persona, I’m interested only in CFD. There can be value by combining CFD data with structural data or other CAE data also. So, I think AI in multiphysics simulation can also be a future. And we can create a good feedback loop across all these personas.
[00:23:57] Krishna Veeraraghavan: Since AI is expected to play a big role, we think there will be new roles opening up for data science and machine learning skills in R&D and engineering designs also. I think it’s already started, and it’s going to be more now. AI means a lot of data, so IT will definitely play a bigger role here, as more data is planned to be stored either in the Cloud or on hard drives. The last thing is Siemens is one of the very few companies that have the potential and software to help customers build this digital infrastructure in order to collect and extract the data from various sources. We have facilities to extract data from manufacturing, from design, and also even in real-time. So, we can combine all this data and we can analyze all this data in a seamless chain where engineers and end-users – even their customers – can get benefits as per their evolving needs.
[00:25:01] Thomas Dewey: You’ve touched on this, but just to summarize for the listeners, what are some of the key or main benefits of bringing AI into a CFD simulation?
[00:25:10] Krishna Veeraraghavan: It can definitely improve the speed, reduce the design program turnaround time and also cost by reducing the number of simulations. You would use your simulation for a more detailed analysis. And this AI is going to be used as more as a complementary tool, I think it’s definitely going to improve the speed. It can also be used in knowledge-based assistance, like the example I gave, the anomaly detection. And AI can also be helpful in reducing the complexity, making the process more efficient. And lastly, it can enhance data-driven design decisions. So, we will be able to smartly reuse the simulation data we generate for future predictions.
[00:26:00] Thomas Dewey: And all those add up to saving money.
[00:26:03] Krishna Veeraraghavan: Yes, that’s what everybody wants.
[00:26:07] Thomas Dewey: So, when customers try to deploy AI in their float, are you seeing any barriers that they might face?
[00:26:15] Krishna Veeraraghavan: The first thing that comes to mind, is the trust among these machine learning models or these black box models. And trust is one of the key factors, we always want to develop with the customers. We always want to solve their problems by relevant – technology is not something which is not relevant. So, I think it takes time. As they use these machine learning models more and more on their design, and if we can explain why certain things happen or behave in this way, then I think the trust would gradually go up. Generation of simulation database is something which we also realize is a problem for most of the customers, because even a lot of big companies, they store their data on hard drives, and they don’t have an efficient way to extract the data back. So, that would be one of the challenges, because CFD data generates gigabytes of data, and if you add it up, it becomes easily terabytes. We have to smartly extract information that is really needed. And Simcenter is also working on a lot of features to minimize the data size, that’s one of the challenges. And data reduction techniques and what key information needs to be extracted from the simulation is also one of the few areas where we think customers are having problems. And I think I already mentioned that aligning IT with engineering groups is very key. And it can come up with some challenges also because a lot of data security issues can pop up. The last thing is not every company will have the machine learning and data science expertise needed for the engineering job. So, that’s something they might have to build from scratch. The AI transformation journey can be a problem for a few customers. But of course, we are here to help them achieve that.
[00:28:07] Thomas Dewey: Outside of CFD, are there any other disciplines within computer-aided engineering world that could benefit from AI?
[00:28:15] Krishna Veeraraghavan: I think system simulations, and mechanical simulation and control engineering groups will also benefit from implementing AI in simulations. And we have already seen our Simcenter Engineering Services team implementing certain AI solutions in all these personas for our customers. And I think it’s going to benefit a lot of other simulation areas also, definitely.
[00:28:40] Thomas Dewey: So, you’ve mentioned engineering services a couple of times. If listeners wanted to reach out to start a new project, what’s the best way that they could do that?
[00:28:49] Krishna Veeraraghavan: There is a dedicated email for Simcenter Engineering Services, it’s engineeringservices.sisw@siemens.com, or they can reach out to me on either on LinkedIn or on my Siemens email, which is krishna.veeraraghavan@siemens.com.
[00:29:17] Thomas Dewey: When I do the blog post for this, I’ll put that information in there. But it does sound like there’s a lot of experience in engineering services, and to get somebody started, that’s the best place to go. Krishna, thanks for discussing the role of AI in CFD simulations. I know I learned a lot. And I wish you well in your efforts going forward.
[00:29:39] Krishna Veeraraghavan: Thank you. I hope it was useful for our customers.