New opportunities, new thinking on design simulation tools
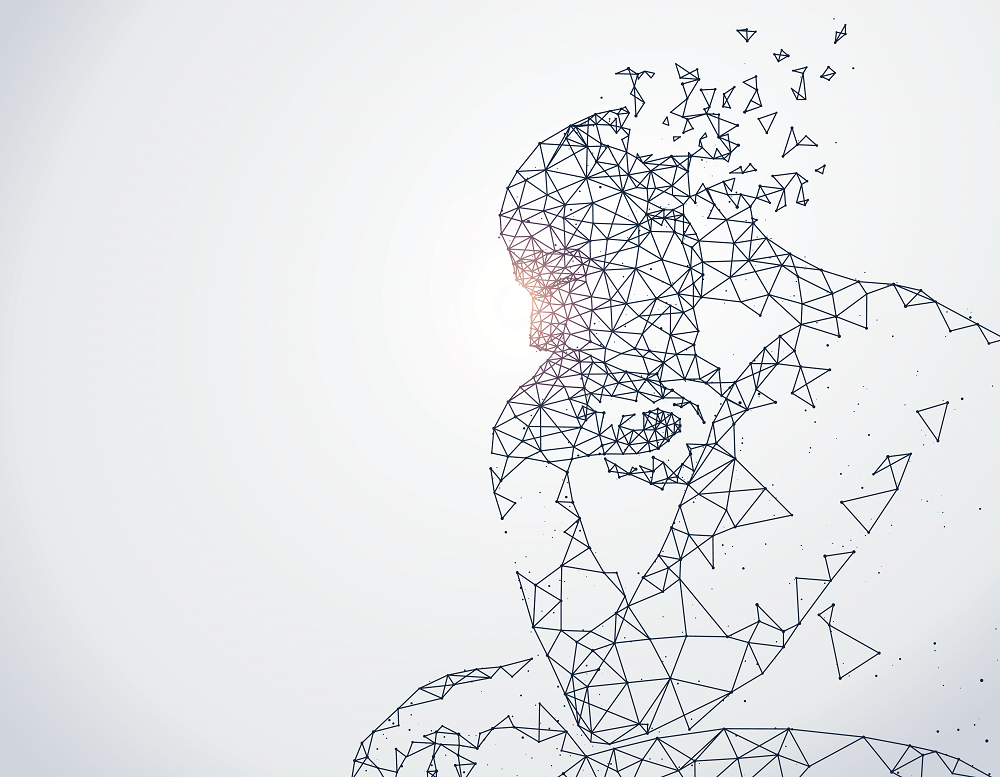
Within every design engineer, it seems, is a perfectionist—someone driven to identify the absolute, best possible designs. The trait is so prevalent that it’s earned a special phrase: engineers who keep tweaking a design beyond basic functional requirements are said to be “polishing the cannon ball.”
As one blogger put it: “This is a malady that affects organisations where design of new product is one of the mainstays of the business,” and where time-to-market can mean the difference between beating the competition and getting beaten.
This vivid description demonstrates the extent to which most engineers and engineering-reliant companies have had to suppress this urge to discover the optimal designs for their projects. Time, resource and cost constraints have kept them focused on the practical, meeting design requirements, not the possible.
Meanwhile, a select group of engineers—those in industries where optimal design yields big pay-offs—employ every means available to identify the best possible design, namely design optimisation and design space exploration (DSE) strategies. The aerospace industry, with its relentless pursuit of lighter weight components and more efficient engines that reduce fuel costs and environmental, is one example.
Intelligent design space exploration changes the game
Intelligent DSE changes the equation, giving every engineer the power of design optimisation and design space exploration in a software application. The software capability runs concurrently with other design simulation tools, automatically converting data from any design simulation tool. The engineer inputs the desired performance goals and the time available to optimise the design, runs the simulation model and design exploration, and the software outputs improved designs. With time no longer a constraint, why stifle the innovation you can achieve by allowing some cannon-ball polishing?
DSE isn’t new, but an intelligent DSE capability is.
DSE is a process in which designers assume that the optimal design is unknown and initially uncharacterisable, and take steps to identify it. Traditionally, it involves an iterative process in which teams of engineers from different disciplines, analysts and optimisation specialists discover design conditions by designing, testing and analysing a series of design options.
Little by little, the parameters and possibilities are discovered, and then the final design is identified through a convergent design optimisation algorithm.
This approach can be confused with design optimisation, which relies on upfront specification with well-defined objective, design constraints and outcome preference. This approach essentially predefines what the optimum solution is, and then employs an iterative, computational search to identify the final design.
Getting more value from design space exploration
With this new capability comes the need to unleash a broader approach to design. When asked about their design optimisation tools or design space exploration needs, engineers accustomed to the traditional approaches tend to dismiss the need for defining optimum designs.
With Intelligent DSE’s relative low-cost, low-resource demands, manufacturers can do more.
•Adding new advantages to mature designs: For example, for a printing machinery manufacturer, printing speed, quality and reliability are the key selling points. DSE would allow them to take current models and simulate how to improve upon these desired attributes; for example, allowing them to simulate a number of speeds while measuring peak stresses and product costs so that they can identify a best trade-off design.
•Improving the margins and performance of existing designs: Re-evaluating existing designs using intelligent automation is likely to uncover new ways to improve the design, whether for better performance or to remove cost. Either way, a DSE solution with an intelligent-automation engine is a low-overhead, low-hassle investment that could yield potentially significant improvement in top- and bottom-lines for high-volume producers.
•Improve process efficiency: Process simulation is another area ripe for improvement using intelligent DSE software. Many production processes have been designed without the benefit of intelligent automation. Applying this science to existing plans would likely uncover numerous cost- and time-saving opportunities.
•Design entirely new products: Intelligent DSE expands product development teams’ capabilities so they can solve more and different types of customer problems. Organisations are rife with data these days. What’s hard is isolating the right data to do the most-promising work in terms of best trade-off choices. Intelligent DSE does this for design teams. It uses data that is going to be generated anyway and is so easy and fast that it opens up time and intelligence to find newer, better or unique solutions.
Adding intelligence to mechatronic system design
One trend making intelligent DSE more relevant is the growing need to design or improve upon mechatronic systems as electronic, digital and mechanical systems merge.
For example, Airbus used intelligent DSE to improve the performance of the aircraft bleed-air system design on its A320 family of planes. The complex system feeds preconditioned air into multiple other systems that control cabin pressure and temperature, as well as wing de-icing and avionics. The Airbus team wanted to reduce pressure loss and improve temperature in the static-mixer design that comprises the bleed-air system.
Airbus first set out to find a better design in 2013 by optimising three design parameters. However, intelligent automation wasn’t around then, so it was challenging to integrate data from two in-house design programs with multiple off-the-shelf programs. The result was some stability problems in the simulation that required use of mathematical approximations; the process also took six months. In the end, the optimisation exercise demonstrated the potential for an improvement for temperature, but it was not sufficient.
Four years later, the team returned to the optimisation effort, and armed with modern design tools, the team expanded its exploration to 11 parameters and thus, many more system configuration options to consider than in 2013. After 211 initial simulations, the team could identify a desired subset and then perform an additional 55 simulations based on temperature variation alone.
From there, the team could zero in on another subset of potential designs that fell within parameters; and then finally, the optimum design to achieve the new temperature and pressure goals. The full optimisation was achieved in less than two weeks.
The differences in the two Airbus experiences demonstrate the potential that intelligent DSE can bring to dramatically speed up the product optimisation process and improve outcomes by expanding simulation’s boundaries. It’s a powerful change that can spawn new thinking about what product development teams contribute to new revenue sources, cost improvements and ongoing innovation.
Heralding a new era of design
Whether you’re accustomed to settling for “good enough” or pursuing every means for an incremental improvement, DSE software with intelligent automation capability represents a new era in mechanical engineering and design.
With its relative lower cost and ease of use compared with alternatives, it’s likely to set off a race to discover how this new capability gives companies a competitive edge.
This concludes part two of our series on design innovation. In part three, we conclude the series by discussing how modern design software helps address common business challenges.
About the author
Bruce Jackson is an account orchestrator for Siemens PLM Software and works closely with aerospace and defence companies in the U.K. He has been working in the PLM and CAE space for more than 25 years and is currently working with companies across Europe to help them achieve productivity gains through digitalisation.