Why some machine learning solutions succeed, and others fail
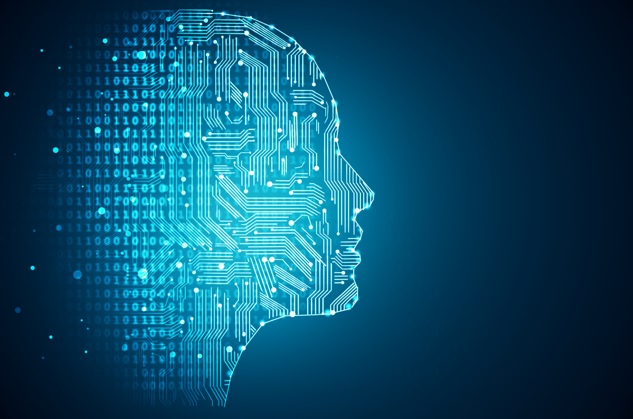
Note: Interested in getting to the right answer with your ML-enabled EDA solution? Head on to our on-demand webinar “Solido Solutions – Getting the Right Answer with Machine Learning“, where we share more about what it takes to develop a production-usable ML-enabled EDA tool, and examples of how Solido ML-enabled tools accomplish this!
According to the 2021 Gartner Hype Cycle for Artificial Intelligence, A.I. applications that are closest to productive usage today include semantic search, computer vision, autonomous vehicles, and chatbots.
One key piece of information in that chart is the expected number of years that technology reaches its “plateau of productivity”. According to the chart, applications that are more mission-critical require more time to reach its productive stage. For example, it takes less development time for A.I.-based chatbots to be productive, compared to autonomous driving, because the consequences of delivering inaccurate results are much more severe in the latter case.
Similarly, ML applications for EDA tasks require more development time to reach productivity. Due to the high cost of developing and implementing silicon designs (running on the millions or billions of dollars per chip), as well as mission-critical end applications for these designs, engineering teams must be confident that their ML-enabled EDA tools consistently deliver results that are not only correct, but proven to be correct, every time.
In other words, in addition to providing runtime and engineering time advantages to traditional methods, ML-enabled EDA tools must also be verifiably accurate, robust enough to work on different use cases within its target application, and usable such that the user can drive the tool effectively without being an expert in statistics or ML.