How AI is augmenting the PLM process – Transcript
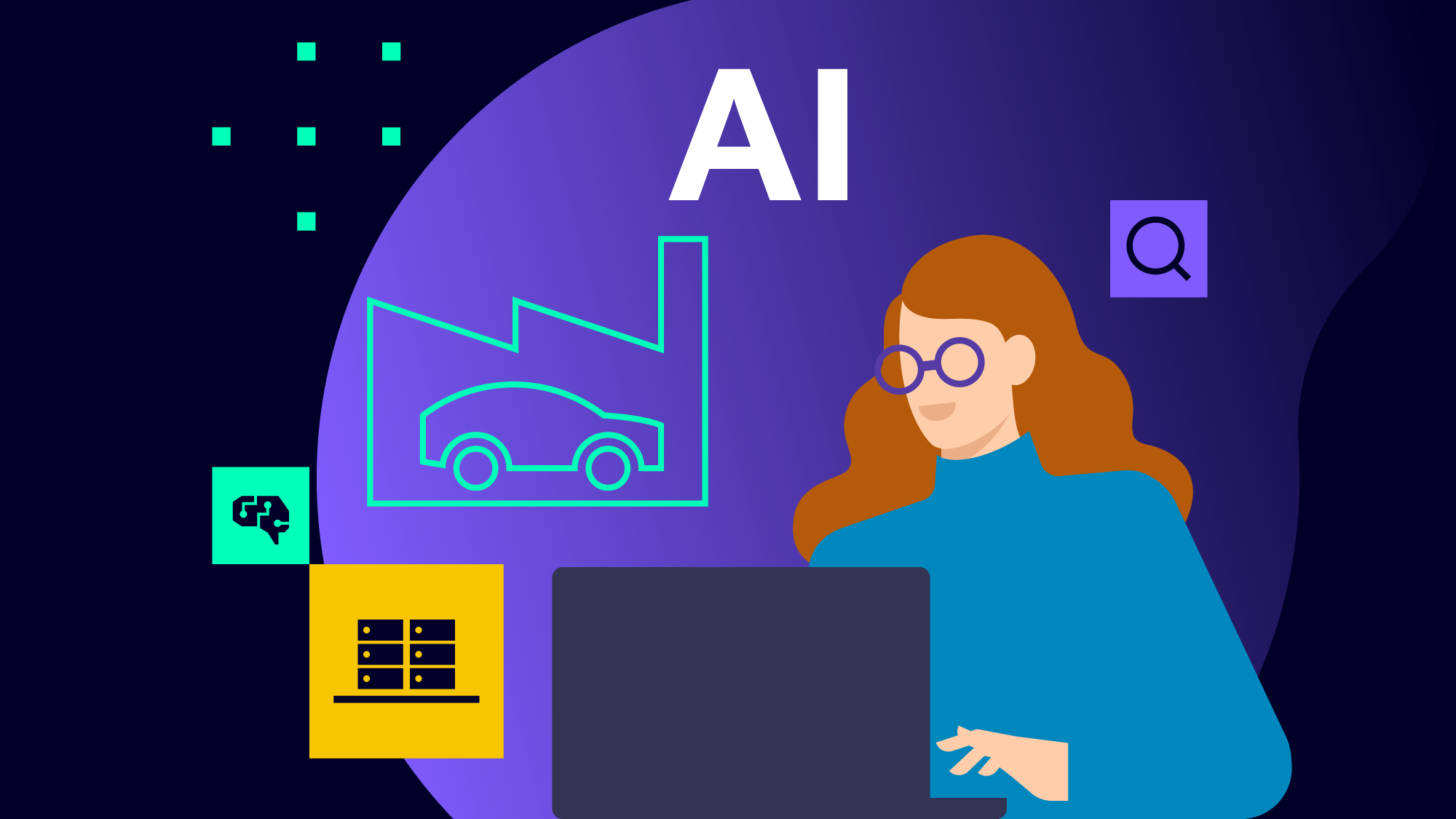
In a recent podcast, Charles Aldave, Product Marketing Manager for Teamcenter, explored some of the applications of AI in PLM software such as Siemens Teamcenter. Check out the full transcript of that talk below, or listen to the episode here.
Spencer Acain:
Hello and welcome to the AI Spectrum Podcast. I’m your host, Spencer Acain. In this series, we explore a wide range of AI topics from all across Siemens and how they’re applied to different technologies. Today, I’m joined by Charlie Aldave, product marketing manager for Siemens Digital Industry Software. Welcome, Charlie.
Charlie Aldave:
Hi, Spencer. Thanks for having me on.
Spencer Acain:
So before we jump into our main topic here, can you give me a brief overview of your background and your work at Siemens?
Charlie Aldave:
I’m a product marketer for the Teamcenter software portfolio and I focus on our AI and visualization offering.
Spencer Acain:
Great. So maybe if you could give me a quick overview of what Teamcenter is and how you’re applying AI to it.
Charlie Aldave:
Sure. I’ll start by talking a little bit about Teamcenter. Teamcenter is our solution for product lifecycle management or PLM. It’s used by product manufacturers to manage product data, orchestrate processes, and perform a range of functions across the product lifecycle. So things like bot management, change of configuration management, product costing, manufacturing and process planning, among many others.
Onto AI, we’ve been utilizing AI in Teamcenter for some years now, and it’s an area we’ve continued to invest in. Our AI enabled products include Teamcenter AI for Classification, which is a tool that assists with classification tasks. Teamcenter Assistant, which is an AI enabled command prediction assistant. Teamcenter for Microsoft Teams, which we launched last year, is our first product to use generative AI. It’s an app on Microsoft Teams that assists frontline workers with creating problem reports. The people we’re targeting work in manufacturing and service environments, so they don’t have easy access to a computer, but our app allows them to do this almost hands-free from their mobile device. They can dictate into their phone a description of the problem they’re observing, and also upload photos.
Then, using Azure OpenAI Services, our app extracts key information and auto-populates a problem report, which they can then review, edit, and submit it to a workflow, ultimately connecting them with the people who can fix the problem. Our newest gen AI solution, which we recently introduced into the Teamcenter, is called Teamcenter AI Chat. It’s kind of like ChatGPT for Teamcenter. So using natural language, you can ask Teamcenter AI Chat questions and get hallucination-free responses that are grounded in your company’s data. And this can include text-based documents like PDFs, Microsoft Office files, text extracted from images, Teamcenter requirements, structured data from edX and Solid Data drawings, and a lot more formats.
Spencer Acain:
It sounds like you have a huge off range of offerings here covering both generative and non-generative AI solutions. And I think you’ve already kind of done a great job of expanding on some of what these generative AI offerings that you have are, but I think you mentioned something interesting in your last response was that you said that Teamcenter AI Chat is offering hallucination-free responses, and obviously hallucinations are kind of like the big bogeyman almost of generative AI. So how are you able to offer these hallucination-free responses when that’s been such a major challenge in generative AI adoption and implementation in general?
Charlie Aldave:
Yeah. I guess the reason we created Teamcenter AI Chat was to accelerate the information seeking process. So I think we all were kind of blown away, I guess it was probably a couple of years ago now, when we saw the capabilities of ChatGPT and then kind of the influx of these other chatbots, where instead of having to do an internet search, like a Google search for example, and search through search results to try to find the information you’re looking for, click on a bunch of links to websites or documents or whatever trying to find information, these chatbots really accelerated that information seeking process. So instead of just searching for web pages or for relevant files and then searching for content within those files to get the information you need, these chatbots were just returning information. So really accelerating the information seeking process.
And so that’s what, really, we were trying to do with Teamcenter AI Chat, was introduce kind of like a chatbot, question answering type capability to accelerate the information seeking process for data specifically managed within Teamcenter. And for us, it was really important that we delivered something that’s enterprise grade and that’s reliable. So I’m sure you’ve probably experienced, and it’s, I think, pretty widely known now, with some of these general purpose chatbots, they don’t always return correct information. Sometimes they return incorrect information, sometimes they hallucinate, so they’ll kind of confidently tell you something that’s not correct. And so that’s something that we really want to avoid with how we implemented Teamcenter AI Chat.
Fortunately, there’s a way to fix this using a framework called retrieval-augmented generation, or RAG, where we utilize the language capabilities of large language models and not their knowledge. The knowledge comes directly from the customer’s data, and if Teamcenter AI Chat doesn’t know the answer to a question, it will say so rather than make something up.
Spencer Acain:
I see. It sounds like you have a very… It’s a complicated process to build these kind of RAG solutions, but you’ve really grounded it in that company data there. And of course, I think something that a lot of AI models don’t do is that yours can say no. It’s definitely a different approach there.
Charlie Aldave:
It’s important that they have confidence, especially when they’re using new technology like this, that they can trust it. And so we’ve really thought about how we can try to make Teamcenter AI Chat as trustworthy as possible while still giving our customers access to some of this groundbreaking technology.
Spencer Acain:
Absolutely, of course. But I think we’ve talked a lot about the kind of generative AI applications that you’re developing, the very user-facing abilities that you’ve included in Teamcenter. But I think on the other hand, you’ve also got some of these other AI offerings that are maybe a little bit more invisible almost, that just are working behind the scenes to offer users a smoother experience, an easier experience, like making the Teamcenter application itself feel more intelligent as it were. Maybe you could expand on that a little bit, give me some more details on what that looks like.
Charlie Aldave:
So I’d say overall we’re looking at how can we improve user experience of Teamcenter, and I think generative AI is opening up a lot of doors for that. So I mean, generally, this idea of how can we kind of translate user intent into action is, I think, a really exciting area with generative AI, and we’re kind of exploring how can we do that for a variety of use cases. So Teamcenter AI Chat kind of introduces that for unstructured data, for document-based knowledge. How can users more quickly find information that they’re looking for without having to go through an exhaustive search process?
We’re also looking at how we can do this with structured data in Teamcenter. For example, if you’re looking for… Let’s say, if you’re looking for a design, there was maybe this keyword that I can remember in it, and it was related to I think this project, and I’m looking for a revision that took place maybe last month or this month. The way you kind of have to do that now is, again, you would have to use a bunch of filters in Teamcenter to try to find that data, or you would have to use the search functionality, which can be challenging for people that really aren’t familiar with the Teamcenter data model. But now, or coming soon, we’re going to be introducing the ability to do that using natural language. So again, trying to make it easier for users to find the information they need. And again, we’re looking at this all around Teamcenter, how we can make things easier, more user-friendly, and I think generative AI is going to be a big part of that.
Spencer Acain:
I see. So it sounds like you’ve got a lot of goals here to bringing the latest technologies and these advancements to your customers and to the applications itself. But obviously that can’t be an easy process, especially with these sort of cutting-edge technologies. So what are some of the challenges you face in bringing AI into an industrial setting, especially where reliability and explainability are must haves for customers who are going to be basing potentially very expensive projects on the results that these AI models give.
Charlie Aldave:
These issues are critical for our customers, and not just with how the solution works, but how it’s using their data. But we’re also finding a lot of our customers have been very proactive about investigating AI. They’re informing themselves on its capabilities, risks, and limitations, and they’re setting realistic expectations.
Spencer Acain:
Absolutely. It sounds like there’s a bit of an uphill battle here, but it’s probably going to be worth it at the end. So to kind of maybe start to bring things to a wrap up here, it sounds like, across the course of this discussion, that we’ve been talking about a lot of AI applications that are focused on making users’ lives easier. And I kind of want to ask, do you see this as maybe a natural application of AI within industry, or do you see AI taking on a bigger role in the future, or apply to different ways, such as around automating processes completely, for example?
Charlie Aldave:
I think AI is definitely helping to make our users’ lives easier and to get more work done faster. We’re leveraging AI now to help automate a range of tasks, as well as improve search and access to knowledge. We’re also using it to help improve the user experience.
Spencer Acain:
I guess to kind of round things out, where do you see AI going in the future, both for Teamcenter and for the industrial manufacturing world at large?
Charlie Aldave:
I see it expanding us in a lot of areas. Two areas I’m especially excited about are engineering modalities and agentic solutions. Large language models or large multimodal models are today trained on data primarily across four modalities, text, images, video, and code. And they’re useful for virtually everyone since all businesses have lots of these types of data and they need to create more of it and get more value from it. Product manufacturers have lots of this data, but they also have vast amounts of engineering and manufacturing data. So 3D and multi-CAD data, bill of materials, system design data, and machine and manufacturing data. A large multimodal model that’s trained on this data would make for exciting possibilities.
Agentic solutions is another exciting area that’s developing quickly. While it probably sounds unbelievable, most of the gen AI use cases you’re seeing today are basic tasks. You prompt a model with a query or task and get a response. Agentic solutions are able to take on much more complex tasks and perform even more of the work they can propose and even execute multi-step plans using multiple tool sets in response to a prompt with or without a human in the loop.
Spencer Acain:
Well, it sounds like you envision a bright future for AI in industry and probably the world of general, I would feel.
Charlie Aldave:
I hope so.
Spencer Acain:
Yeah. Well, but I think that’s about all the time we’ve got for this episode. So thank you, Charlie, for joining me here today.
Charlie Aldave:
Great. Thanks, Spencer.
Spencer Acain:
Once again, I have been your host, Spencer Acain, on the AI Spectrum Podcast. Tune in again next time as we continue exploring the exciting world of AI.
Siemens Digital Industries Software helps organizations of all sizes digitally transform using software, hardware and services from the Siemens Xcelerator business platform. Siemens’ software and the comprehensive digital twin enable companies to optimize their design, engineering and manufacturing processes to turn today’s ideas into the sustainable products of the future. From chips to entire systems, from product to process, across all industries. Siemens Digital Industries Software – Accelerating transformation.