The key role of AI in semiconductor testing
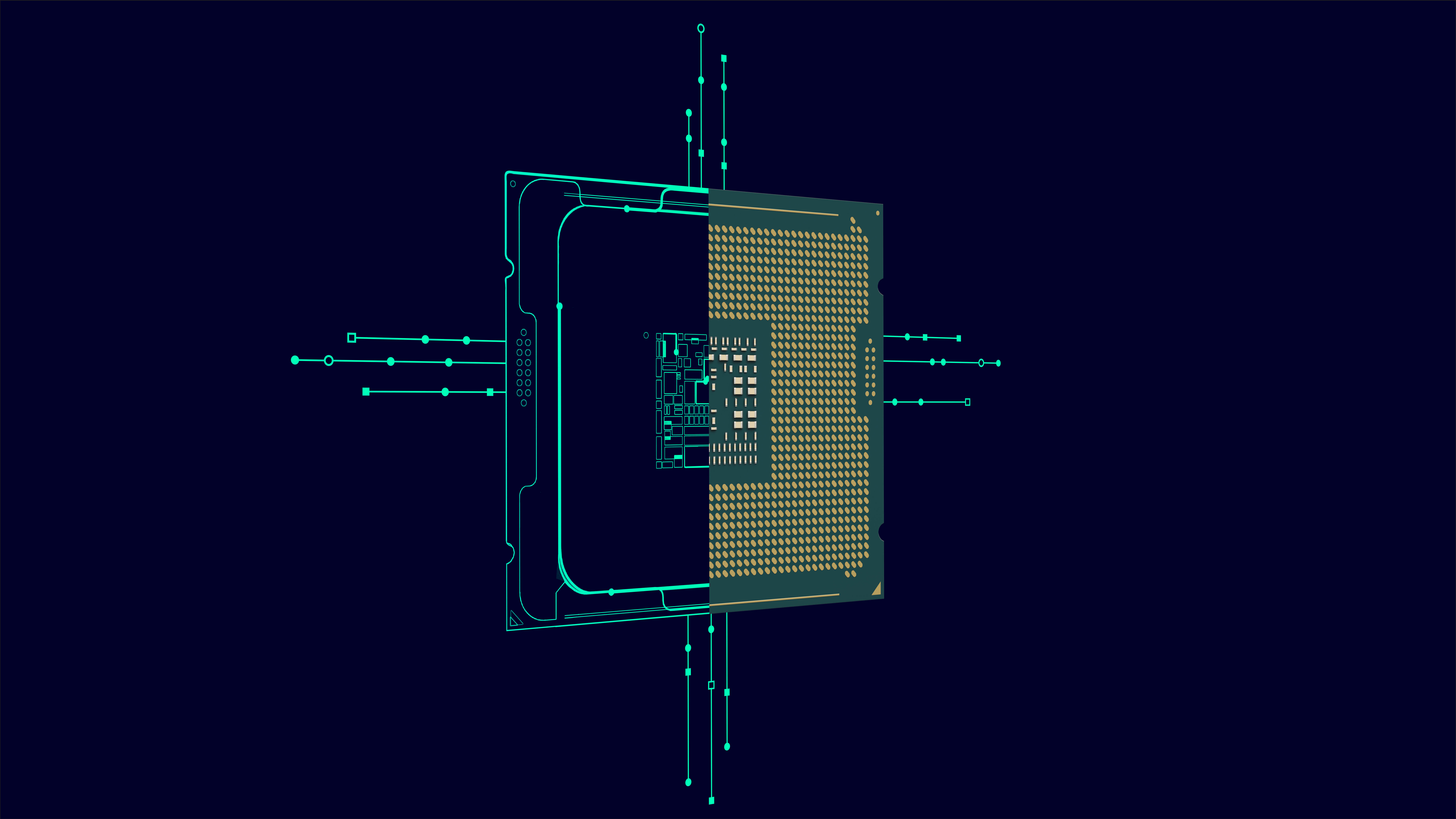
Design for Test (DFT) is a key concept in the realm of semiconductor design, helping make sure that finished products can be tested exhaustively with minimal added cost. However, DFT is, itself a highly complex process that must balance both the chips power, performance and area (PPA) with the additional elements required for the testing process – elements that do not contribute to the PPA of the chip itself.
In a recent podcast, Ron Press, Senior Director of Technology Enablement at Siemens Digital Industries, continued a discussion, the first part of which can be found here, on the applications of artificial intelligence in DFT. This includes the addition of analytical AI in DFT tools like Siemens EDA’s Tessent, applying machine learning to scan data to better analyze failure modes, as well as examining the challenges of bringing AI to the cutting-edge of semiconductor design.
Check out the full podcast here, find the transcript here, or keep reading for a summary of the highlights from that conversation.
A digital twin of failure analysis
Analytical AI is a key application of AI/ML within the semiconductor design process, Ron explains, since it allows test structures to be embedded efficiently within a given chips design. While this an incredibly important application, Ron sights Intel improving their productivity while implementing DFT architecture by a factor of 10X, while Amazon was able to improve testing times by a factor of 5X, this is hardly the only application of AI found in the DFT process.
Once a chip has been designed for test, every single one produced must still actually be tested. This process not only ensures that chip shipped is 100% functional, it also generates a massive amount of data relating to the root cause of failure of defective chips. The root cause can range from a problem with the design to the process itself or any combination of the two. Determining the exact problem, and how to fix it, is no simple task when for a given chip only a few hundred test patterns may fail out of thousands run.
Since they already implement scan technology, which allows for millions or even hundreds of millions of virtual capture points within the chip, Ron identifies how this is the perfect place to apply machine learning to help extract key insights from the available data. This kind of multi-layered approach allows them to build up what he calls the digital twin of failure analysis, a software suite that allows them to figure out how to explain exactly what’s going on in a particular chip and why its failing. The ability to do this quickly and easily in software, rather than through slow, exhaustive physical testing, makes it especially important in an industry were improving yields even half a percent could result in millions in savings.
The need for AI at the cutting-edge
Leveraging AI and ML to bring new features, like the digital twin of failure analysis, into the semiconductor industry is increasingly important to keep pace with the demands of newer, more complex chips. However, bringing AI into an industry where any error could be incredibly costly is not without its challenges but at the same time, an industry as cutting-edge as semiconductor design must often rely on cutting-edge technology to succeed.
Ron explains how, for many of the technologies that have become ubiquitous in the semiconductor industry, despite initial reluctance in adoption, companies were quick to adopt new methodologies and technologies because of the highly complex nature of the products they were working on. Compared to many other industries, were existing methods perform well enough to meet the needs of current and near future products, the semiconductor industry must embrace technologies on the very cutting-edge in order to achieve the next node shrink or cram millions more transistors into just a few square mm of space. This leads to a far faster adoption of AI technology then in many other comparable sectors.
Designing a next-generation chip and making sure the final product reaches the high level of standards demanded by the industries that utilize these chips is a daunting task. To rise to this challenge, semiconductor design companies must be willing to embrace new, cutting-edge advancements such as AI. This rapid adoption of new technologies means that the semiconductor industry is poised to lead the charge for more advanced digitalization of the design and manufacturing process, blazing a path forward for other industries to follow even as they themselves reap the benefits of AI-integrated design, testing, and production.
To learn more, listen to the full podcast episode here.
Siemens Digital Industries Software helps organizations of all sizes digitally transform using software, hardware and services from the Siemens Xcelerator business platform. Siemens’ software and the comprehensive digital twin enable companies to optimize their design, engineering and manufacturing processes to turn today’s ideas into the sustainable products of the future. From chips to entire systems, from product to process, across all industries. Siemens Digital Industries Software – Accelerating transformation.