Adapting AI solutions across industry
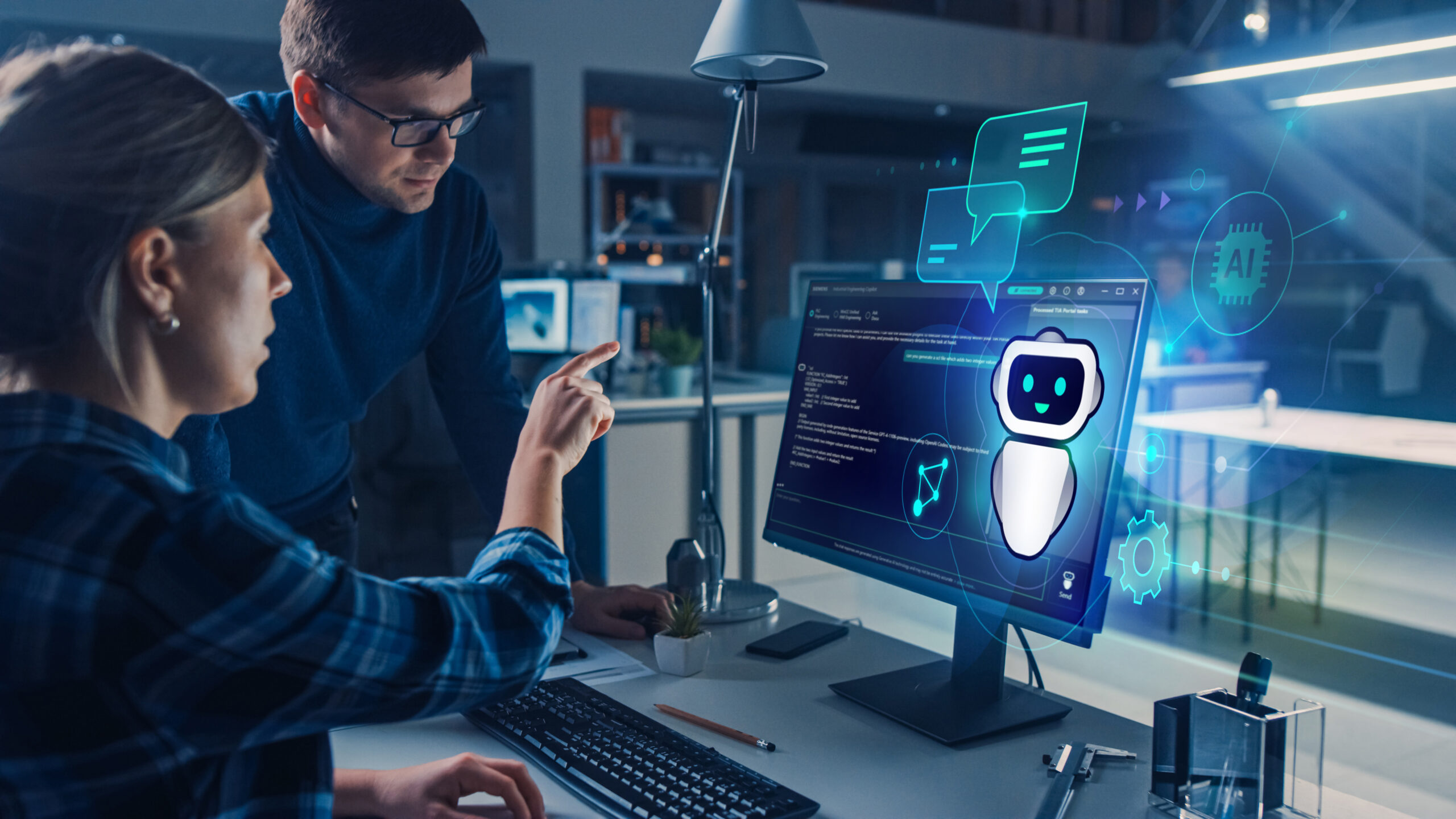
AI or digital assistant aren’t a new concept and creating one that can offer both human-like interactions and capabilities has long been a goal in the domain human-computer interaction. Now, with the rise of generative AI, this dream is closer to reality than ever before but developing and deploying such an advanced AI solution in an industrial setting is no simple task with a far greater number of considerations and challenges to overcome then the consumer space.
In a recent podcast, full episode available here, host Spener Acain was joined by guests Michi Lebacher, head of AI and digital Business at Siemens and Alessia Bortolotti, Customer Discovery Manager for Siemens AG to explore the challenges of building an Industrial Copilot system that spans across the entire breadth of the Siemens software ecosystem and why AI technology aren’t just a one size fits all solution.
Applying AI across the breadth of industry
Compared to the digital assistants found on a smartphone and other similar devices, building an Industrial Copilot that can span across industries and various stages of the design process is a far more daunting task. Michi explains that for these type of industrial solutions, rather than trying to create a big general purpose model, “a sandbox you could ask about football” as he put it, they are instead working to create highly specialized models within different domains.
By fine tuning an AI model to become an “expert” at a specific domain, such as product designs or factory operations, the model can provide a superior result right out of the box with no further training required. This type of specialized model training also lends itself well to layering generative AI models atop existing software and infrastructure. For example, rather than having a copilot for factory operations, one might exist that is purpose built and integrated for writing PLC code, offering substantial gains in productivity as it takes over repetitive parts of the process. At the same time, individual copilots could be deployed for maintenance across a factory or on a machine-by-machine basis for helping with diagnostic work. All of these smaller units would fall under the umbrella of the Industrial Copilot for Operations, itself a part of the Industrial Copilot overall.
Copilot systems can also help beginners learn and become comfortable with systems and technologies more quickly. Returning to the example of PLCs, Michi says these systems are everywhere, yet programming and maintaining them is highly complex and requires extensive expert knowledge. At the same time, any downtime on the machines operated by these PLCs could cost hundreds of thousands if not millions of dollars per minute of downtime, leaving no room for error or experimentation among less experienced users. AI copilots could help bridge this gap by putting what amounts to lifetimes of experience at the fingertips of every users from beginner to expert level.
Benefiting from AI faster with RAG
While training custom models and deploying complex AI solutions creates systems capable of taking on a huge number of tasks, there inherent complexity means time to market can be quite long. Retrieval augmented generation (RAG) offers a faster path to bringing the benefits of AI to industry, since it requires little or no training of an existing model while still providing many of the benefits of an easy to interact with knowledge base.
Michi highlights that RAG approaches make a lot of sense when deploying a different solution for different customers compared to fine-tuning a single big model or foundation model. RAG is a quick way to enrich existing data with an AI solution that can provide additional context, talk to the document features and the ability to easily collate data from across an entire database of knowledge. For many applications of tools like the Industrial Copilot, this alone is enough to provide meaningful value and workflow improvements, especially since it is capable of providing value from the very moment the system is deployed.
When it comes to deploying AI in industry, having the right model and even the right approach to a problem is often the largest part of building a successful and useful solution. Tools like the Industrial Copilot which leverage both a wide range of models and applications under a single, unifying umbrella will be key in bringing AI to the mainstream, allowing powerful access to AI tools and benefits without the need to develop countless bespoke solutions. Embracing the many forms of AI will be a key part of the digitalization of industry as it improves efficiency and accelerates every stage of the industrial value chain.
Check out the full podcast episode here or read the transcript here.
Siemens Digital Industries Software helps organizations of all sizes digitally transform using software, hardware and services from the Siemens Xcelerator business platform. Siemens’ software and the comprehensive digital twin enable companies to optimize their design, engineering and manufacturing processes to turn today’s ideas into the sustainable products of the future. From chips to entire systems, from product to process, across all industries. Siemens Digital Industries Software – Accelerating transformation.