How AI is optimizing factory maintenance transcript – Part 3
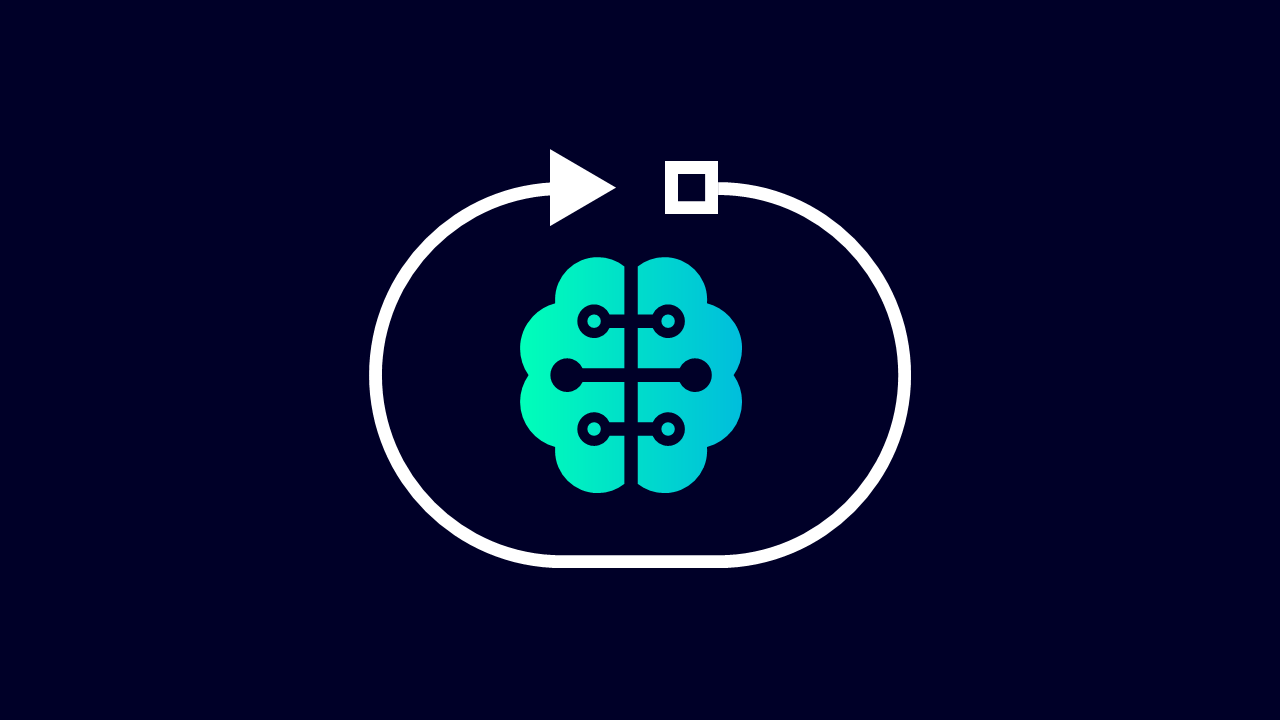
In a recent podcast, I spoke with Dr. James Loach, Head of Research for Senseye Predictive Maintenance, to learn more about the ways Senseye is using AI as well as where AI will be applied across industry in the future. Check out the full episode here or keep reading for the transcript.
Spencer Acain: Hello and welcome to the AI Spectrum podcast. I’m your host, Spencer Acain. In this series, we explore a wide range of AI topics from all across Siemens and how they are applied to different technologies. Today, I’m once more joined by James Loach, Head of Research for Senseye Predictive Maintenance. As we continue to discuss the ways that Senseye is applying artificial intelligence to predictive maintenance and to the way that users and engineers can interact with information, data and machines themselves. So to pick up where we left off in previous parts of this conversation, is there anything else that makes Senseye unique compared to other predictive maintenance platforms, would you say?
James Loach: I think the general purpose nature of Senseye is quite unique. People try to do it, but Senseye, it was of interest to Siemens when we were acquired because of some demonstrated ability to do this, accounts over 10,000 assets. And then the decision support thing. Yeah, I don’t think I would say anything other than those things, but I think it’s interesting. It’s that, and I think in general about Senseye, it’s just being very clear about the world. That’s what we’re trying to do. We are not about doing deep learning to do magic, in quotes. We are building this very practical, reliable, dependable system in the way that I’ve described. Easy to set up, easy to use, scalable, and does the thing, right?
Spencer Acain: Something you’d be interested in deploying at a big scale rather than as a proof of concept to show people that’s like, this is what you could do with AI magic, basically.
James Loach: Yeah. And it’s strange. It’s like, I don’t know, you talk about ways to describe this, but there is a sense in which we do the boring stuff in a somewhat boring way. The idea is it is a reliable, solid colleague, and through acting in that way does provide real value. And it doesn’t just do the magic thing on 1% of your assets. The thing happens to have been trained properly or happens to work. It’s a particular situation, right? Anyway. Yeah. But it’s interesting. Scalability does… Yeah, it is strange. It’s just what I said really. But language models make this great, right? Because you made these pragmatic decisions, organize things in this pragmatic, sensible way, and then you just got this pot of magic to spread over the whole thing and it just works, right? You know what I mean? A very nice way.
Spencer Acain: Yeah. Yeah. So do you see these type of general recommendation and conversational systems shifting the modern decision paradigm that’s being used in other industries elsewhere in general?
James Loach: Yeah. I don’t know, the criticism of Senseye would be it’s a decision support nature. It could appear to be a non-precise approach to things and very general and whatever, all of this kind of stuff. And of course, in some sense people just would just like numbers or facts from their system and no fuzziness/ but I don’t know, well, I hate to say ChatGPT is the obvious thing at the moment where you have something which has phenomenal powers and this ability to interact with it in this very, very naturalistic way, work through problems and get really amazing things done. But at the same time, is not in some sense 100% reliable. It is human-like in that sense.
And obviously, people with ChatGPT have some tolerance of this. The advantages you get from that paradigm clearly outweigh, it might give unreliable mushroom identifications. It might do some strange dangerous things in some way, but it’s very good for doing your homework or writing this letter or whatever. It is clearly worth it, the fuzziness. And with that technology, the fuzziness has to be there at some level, that’s just the way it works. Anyway, but this is another reason this language model is a nice fit for Senseye. There is an intrinsic general fuzziness to our system that gets smoothed and actually even sharpened up in some way by the use of language models. And the existence of these non-perfect advisory systems, if you know what I mean. I think will encourage people to be more comfortable with that thing. And it’s just so natural. It’s just what humans are like, isn’t it? Even if you trust people and they do a great job. There’s still the edge of fuzziness or unpredictability anyway, whatever. I think people become more…
Spencer Acain: Yeah. It’s just the…
James Loach: More accepting of…
Spencer Acain: The basis for the way things are. It’s like in the real world things are a little fuzzy.
James Loach: Yeah. But I think people would just become more accepting of that I think in these systems. Certainly B2C things obviously, but yeah, also in these business settings, technical settings as well. Yeah, that’s it.
Spencer Acain: Right. Yeah. So do you see predictive maintenance becoming the standard moving forward? Either the academic version you’ve talked about or the version that Senseye is using, the more general one? And if so, what would be some of the challenges you would see with moving this to a global scale, almost so to speak?
James Loach: Yeah, I can speak to some of the issues that Senseye have. Customers are not very good with sensors and data. This is just a very profound issue. No sensors as a first problem, and then random sensors after that, producing random data. Going into random places that you have to try and get the data out of and et cetera, et cetera. So I think I would say connectivity is still our biggest issue and all very well having a system that can produce results very quickly and all the things I described, but it takes six months to persuade someone to give you the data even after signing the PAC, right? Anyway, so I think access to data and organization of data is clearly a big issue. Right? Again, I think the advances that you’re seeing in AI, I see them just, well, I hate to say, you can imagine a system like Senseye in the way that I’ve described being supercharged and sharpened up.
Well, I hate to say, I was talking about with the conversation, in the past our AI has not really had any concept of machines or how they work or things like this. But by language models in our case, that general knowledge is just immediately available and you can obviously connect it up to more specific knowledge like the manuals and things like this. And so very rich contextualization. And then also the ability to do the data transformation. So we also work on tools for automating that in this conversational way. And then eventually you would want the system to be looking at the data and seeing what’s been done before and using its general knowledge and just being sensible about it and taking away the need for any human effort there at all. Right. So I suppose you could imagine general purpose systems like Senseye becoming higher performing just due to this greater availability of context and greater intelligent support for users and things like that. That doesn’t help with the data connectivity issue, but everything past that should become easier. I don’t know. And then I suppose…
Spencer Acain: The benefiting from the general digital transformation of everything.
James Loach: Yeah. But more specific models, I suppose you could imagine building systems that make it easier for you to train and deploy things that are more like custom models, you know what I mean? So you could try and take that difficulty away. So you could imagine something like Senseye that maybe organizes some kind of automated data scientist, you know what I mean? So you could potentially de-skill some of that expensive specialist modeling. You know what I mean? I don’t really know where all this goes, but I know that it changes very quickly from here on and gets a lot easier, whatever your approach is, I think.
Spencer Acain: Right. So we’re at the cusp of everything being more accessible, more data is available, there’s less need for copious amounts of manual labor and having tons and tons of expert knowledge to get your system working and running. And it’s at a point where it’s maybe even a little hard to say where things are going to go in the future because things are still changing so quickly and advances are happening so fast and all that stuff.
James Loach: Yeah. Definitely. And there are certain areas which are ripe for people to do work in where not much work is being done at the moment. Like time series, foundation models, there’s a lot of image stuff and audio stuff and text obviously. But you should be able to do these kinds of things, obviously you can with time series and connecting time series with words. And yeah, that’s just a very interesting thing. In the language model experiments that we do, we have to describe the time series. So you are finding different sections of it and calculating parameters and characterizing them. Oh, this is a little trend of this size and what have you. And then the intelligence in the system is using that picture, use fragments of information about things and then trying to correlate those with textual descriptions, things that have happened. But I think obviously it’s possible to give these models a more natural understanding of time series with the meaning of that folded in. And yeah, it’s very interesting where that would go. Right? That’s very important for predictive maintenance given the nature of the…
Spencer Acain: Yeah, yeah, of course. So before we wrap this up here, is there anything else interesting you’d like to talk about in the realm of AI, either within Senseye or Siemens or in the field of AI in general?
James Loach: Yeah, I don’t think so. I think we are all the same. Everybody’s very enamored with what is happening in AI at the moment. And in my head, many peoples, it first feels like the first baby steps of artificial general intelligence. These systems available have that character in this very, very basic form, obviously. Yeah, I won’t repeat what a thousand other people have said, right? But it’s obviously a fascinating time in AI machine learning, and yeah, it’s just very interesting how it will play out. But I think how it plays out will play out quite quickly.
Spencer Acain: Absolutely.
James Loach: It’s a wave to ride, I think, right?
Spencer Acain: Yeah. We’re really at the AI revolution right now. Everyone’s really excited about it, but it’ll be interesting to see where things go over the next few years, even next few months, with how fast things are advancing.
James Loach: Yeah. No, it’s strange. Like lots of people, it’s like early this year I’d have trouble sleeping at night because of how fast my field was advancing, right? It’s like it’s difficult just to keep up and comprehend what was happening. That has slowed down a little bit, but yeah, it still extremely fast compared to my old world in particle physics where changes happen very slowly, decade to decade. Right?
Spencer Acain: Yeah. Sometimes it feels like there are things advancing minute to minute in the world of AI. It’s crazy.
James Loach: Yes. Indeed.
Spencer Acain: All right.
James Loach: Cool.
Spencer Acain: Well, I think that’s going to have to be where we end this episode here. So I’d like to thank you, James for joining me here today. It’s been a pleasure talking to you.
James Loach: Yeah. It’s been very nice to be on the podcast and talk to you, even though I talk for 95% of the time. Yeah, no, I’m pleased we got to do this. And yeah, obviously anyone interested in, since I managed to stay this far, welcome to get in touch and happy to talk.
Spencer Acain: Absolutely. Once again, I have been your host, Spencer Acain, and this has been the AI Spectrum Podcast. Tune in next time as we continue talking about the exciting world of AI.
Siemens Digital Industries Software helps organizations of all sizes digitally transform using software, hardware and services from the Siemens Xcelerator business platform. Siemens’ software and the comprehensive digital twin enable companies to optimize their design, engineering and manufacturing processes to turn today’s ideas into the sustainable products of the future. From chips to entire systems, from product to process, across all industries. Siemens Digital Industries Software – Accelerating transformation.