How AI is changing factory maintenance
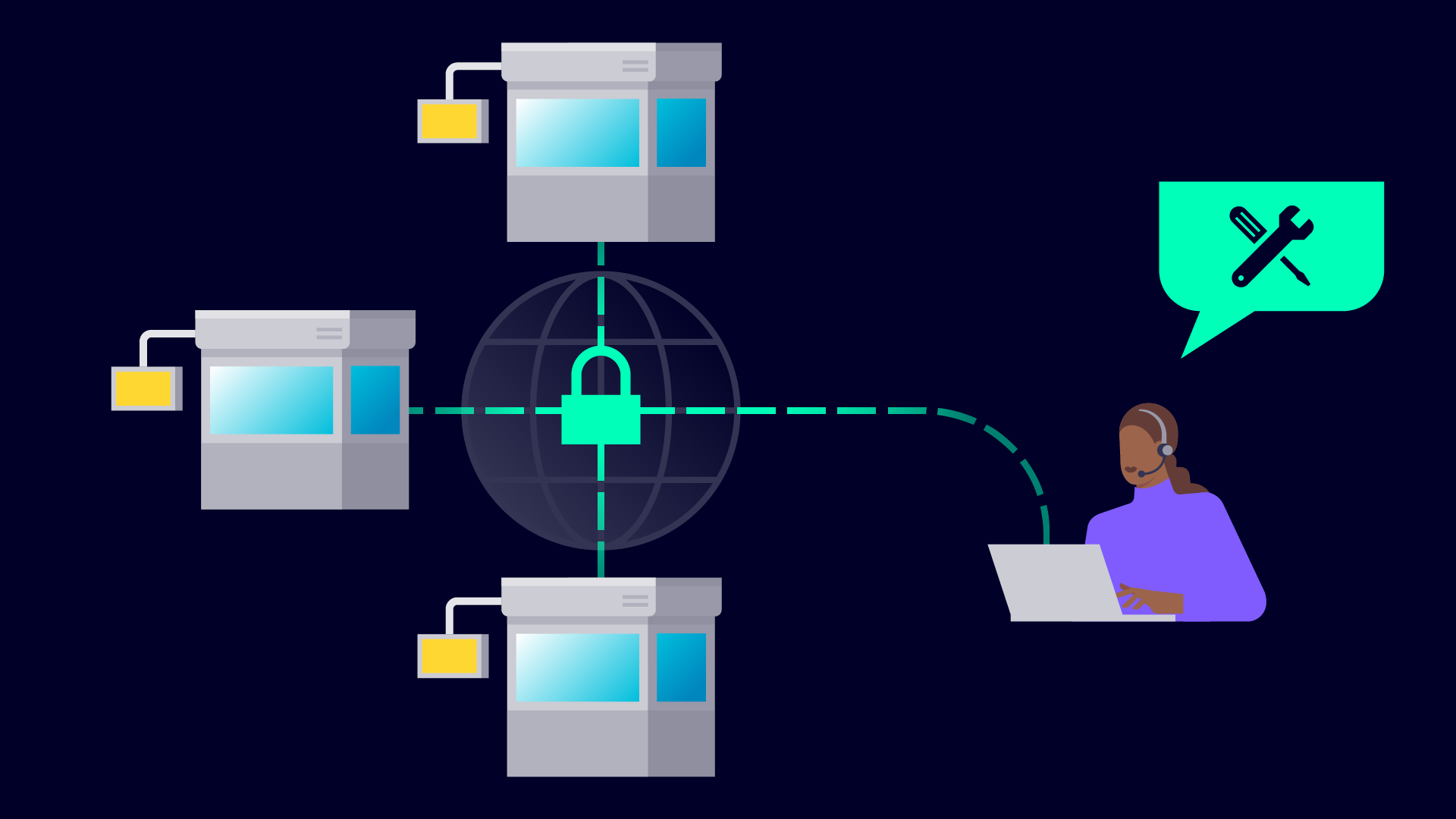
For one reason or another, machines break down. Be it from simple wear-and-tear, uncaught manufacturing defects, or unforeseen accidents, every machine will one day require servicing or replacement. When it comes to factories, where pausing a single machine for maintenance or replacement can represent a significant cost in lost production capacity, it makes sense to try an optimize the process as much as possible. In a recent podcast, I spoke with Dr. James Loach, Head of Research for Senseye predictive maintenance. Predictive maintenance applies AI and ML to the data already streaming from smart, connected, factories and machines to look for patterns that indicate problems before they become critical. What follows is a summary of some of the highlights of that conversation but also check out the full podcast here.
The idea of predictive maintenance isn’t a new one and has existed for many years. However, when it comes to implementing it on a large scale it has often proven to be uneconomical due to the need for complex, bespoke solutions for each machine that needs monitoring. While this approach does have some benefits, namely in the ability for AI to create tailored maintenance plans and predict failures for each connected machine, it does not scale well. This makes it difficult and costly to bring into factories that have tens or even hundreds of thousands of individual machines. James calls this way of thinking a massive distraction since, intuitively, machines don’t fail most of the time.
Rather than trying to predict the exact hour a machine will fail, instead Senseye uses machine learning and artificial intelligence to understand incoming data as indicative of normal or abnormal operation then highlighting abnormal cases for human review. Compared to a human review data, a computer model would be capable of building connections and finding patterns across massive data sets quickly and efficiently, noticing worrisome trends far before a human operator might. At the same time this type of system is far more scalable, since the AI models at its core can be trained on factory data as well as the expert input from experienced technicians in order to quickly build an understanding of what is and isn’t normal.
Compared to other predictive maintenance platforms, James considers Senseye more of a decision support system – highlighting problems and presenting them to the people who need to see them rather than coming up with plans all on its own. While this doesn’t quite fit the bill of predictive maintenance in the traditional sense, what it does allow is for a single person or small group of people to easily monitor a vast network of connected machinery as the AI model handles monitoring the good machines and only draws attention to those showing abnormal signs. This amplifies the effectiveness of people and resources by focusing all their efforts on problem areas without having to check over all the good machines as well.
James says that users should see Senseye more as a colleague with a highly specialized skill set, although also one who still needs a little bit of training here and there. When bringing attention to an issue, users can choose to help further train the model by telling it if what it highlights is an issue or not. This approach effectively helps to capture the expert knowledge of experienced personal as well, connecting information, machines, and humans in a new and valuable way.
As machines and factories grow increasingly complex to accommodate the requirements of new, smarter, and more sustainable products traditional approaches to maintenance may not efficient and flexible enough to keep them running smoothly. Predictive maintenance offers not just a smarter, but a more sustainable approach that will allow companies to focus on developing next-generation products, not worrying about optimizing maintenance schedules. To learn more, check out the full podcast here, or read the transcript here.
Siemens Digital Industries Software helps organizations of all sizes digitally transform using software, hardware and services from the Siemens Xcelerator business platform. Siemens’ software and the comprehensive digital twin enable companies to optimize their design, engineering and manufacturing processes to turn today’s ideas into the sustainable products of the future. From chips to entire systems, from product to process, across all industries. Siemens Digital Industries Software – Accelerating transformation.