Applying AI to industry part 2
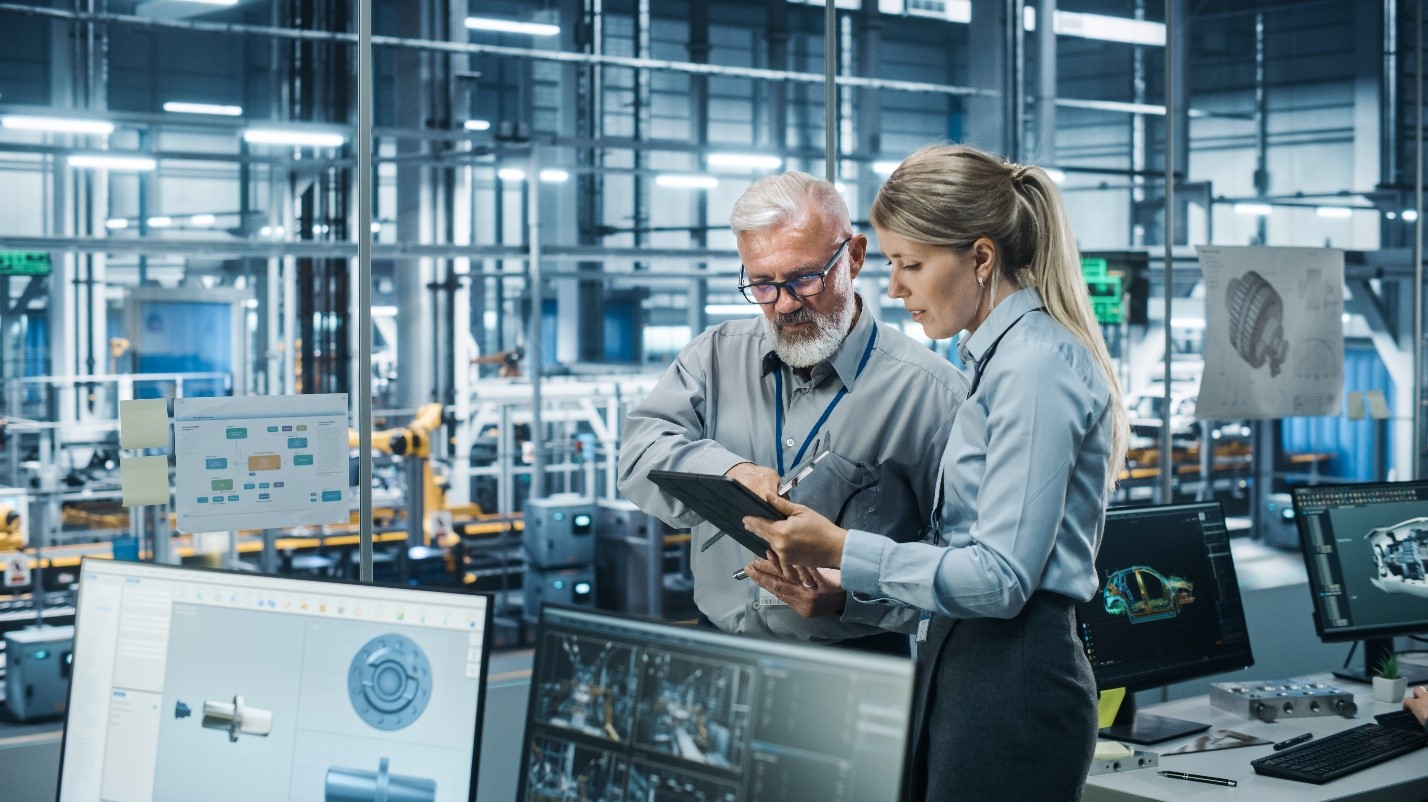
AI is poised to take a larger role in industry helping everywhere from the shop floor to the drafting table, as highlighted in part 1 of this series. In order for AI to execute its role as a trustworthy and reliable helper, however, it must be able to meet the rigors of the industrial world. After the proof-of-concept phase has passed, AI solutions must be made industrial-grade as the next step of the digital transformation of industry.
What it means to make AI industrial-grade
For internal business security and processes as well as for creating products in industry areas such as healthcare, aerospace, automotive, food & beverage, and pharmaceutical production, applications must be bullet-proof, that is, hardened and proven to work well. The following are some of the requirements for AI to be considered industrial-grade, but the two most important are diligence and partnership.
AI developers must work diligently to provide products and services that are explainable, trustworthy, and reliable. Developing AI solutions with industrial operations in mind is, in its core, as big of a challenge as introducing AI in the first place. Furthermore, the cultural home of today’s AI teams is exploratory data science—a data scientist might get bored by the cumbersome work needed to tighten solutions up for reliable operations.
Developing and operating industrial-grade AI is a team effort that starts with a set of required skills which rarely exist within one company. Automation expertise combined with IT/OT integration skills is essential. Business domain expertise, that is, access to ground truth, is a prerequisite for a robust AI model that will be performing in an industrial environment. And, finally, solid AI expertise is required.
The challenges for bringing AI to industry scale are as difficult as the automotive industry solving the complexity of autonomous driving: verification and validation methods, assessment and certification methodology, acceptance by regulation bodies, the interconnection between law (such as the upcoming EU AI Act) and implementation for compliance, best practices in risk management, etc. These all need discussion, knowledge dissemination and shaping within academia, startups, system integrators and end customers.
Building industrial-grade AI with partners
With as fast moving an industry as AI is, it is often startups that are best able to quickly realize and bring to market a concept powered by the latest AI advancements. However, when it comes to bringing those solutions into production, many companies would prefer the long track record and proven reliability brought by larger, more established partners. This is why, when it comes to building industrial-grade AI, partnership is key – between startups and established companies as well as between developers and clients.
As an example of this, Siemens partnered with a startup company in Spain that develops particularly smart algorithms for detecting leaks in water distribution systems. Siemens integrated their AI algorithms into our industry solution, SiWater, and now our precision water meters are fine-tuned to work with these AI algorithms. However, the conservative water-distribution industry, which is dominantly run by governmental authorities and public companies, needs more than integrating some AI. Long-term stability is a key requirement for them, not just on a technology level but in the business relationship.
Siemens is also implementing AI in its own industrial operations. The factory Siemens Electronics Works Amberg annually produces 17 million SIMATIC components for automating plants and machines. Time-consuming X-ray inspection was limiting their production throughput. So, Siemens engineers developed an AI algorithm to predict the likelihood of manufacturing defects, and thus the need for quality testing, using a closed-loop analytics approach. With the new algorithm, they were able to reduce testing efforts, increase production capacity and flexibility in planning, thus reducing capital investment by 500,000 Euro.
These are just some examples of the way building partnerships can help drive adoption of AI technologies across different industries and why, in fact, that partnership is crucial to understanding the challenges of building and deploying robust, industrial-grade AI across a wide breadth of applications.
Building a robust future with AI
Artificial intelligence as a technology is here to stay but getting it ready for deployment in the rigors of industry will be no simple matter. Industrial grade AI, built to a high standard of reliability and with the support of partners, will be a key part in the digital transformation journey of many companies and industries going forward. While it won’t be an easy task, the benefits that industry stands to reap from it more than make up the cost as AI positions itself as a key technology for the world of tomorrow.
Siemens Digital Industries Software helps organizations of all sizes digitally transform using software, hardware and services from the Siemens Xcelerator business platform. Siemens’ software and the comprehensive digital twin enable companies to optimize their design, engineering and manufacturing processes to turn today’s ideas into the sustainable products of the future. From chips to entire systems, from product to process, across all industries. Siemens Digital Industries Software – Accelerating transformation.