The power of simulation to AV design and development: Building reliable ADAS and AV systems part 1 – Transcript
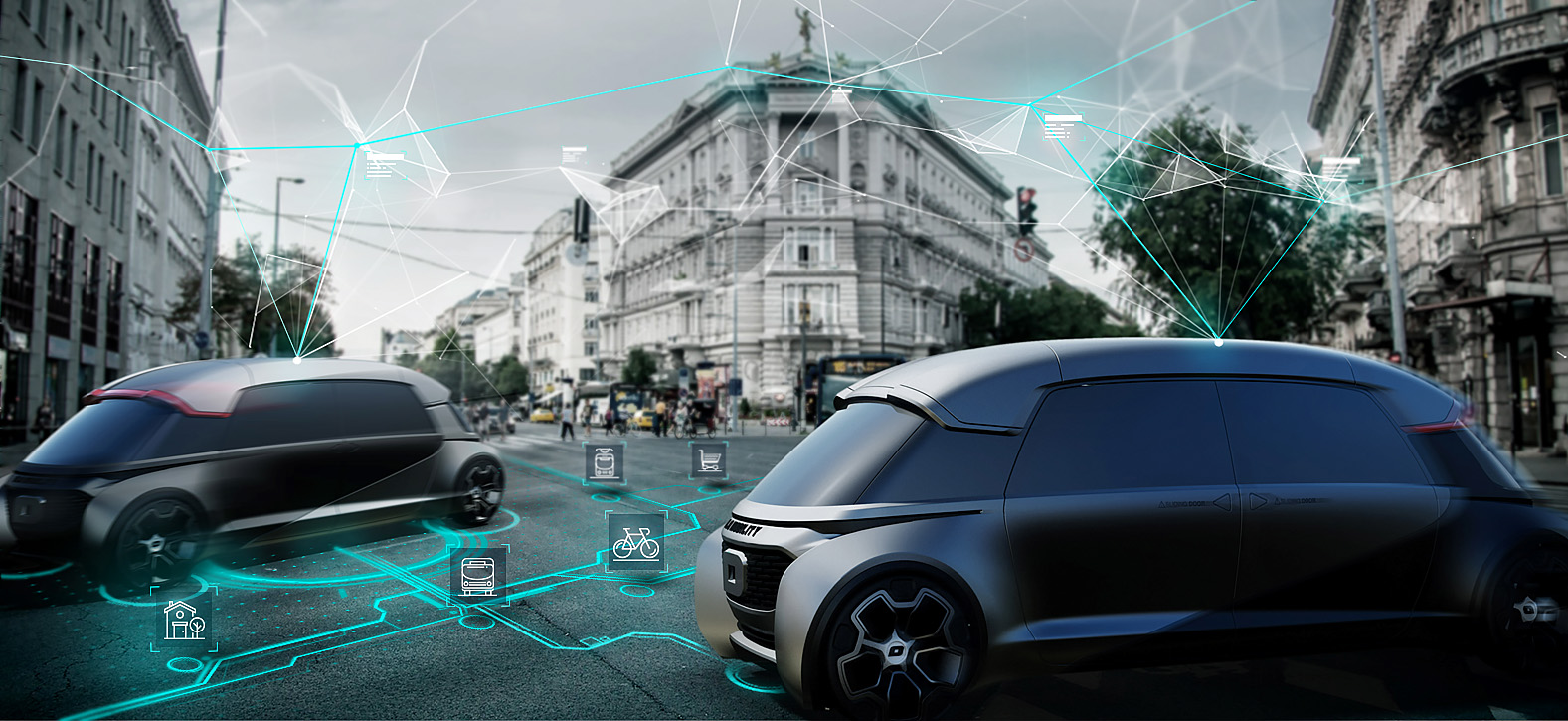
In this episode of the Future Car Podcast, Nand Kochhar, VP of Automotive and Transportation Industries, and I, Conor Peick, Thought Leadership team writer, continue a series of conversations on autonomous vehicles and how they will shape the future of mobility. As the automotive industry moves into the future, automotive companies are faced with the challenge of designing increasingly complex vehicles to satisfy consumer demands for greater automation, convenience, and connectivity.
Among the most important technologies to the success of AVs will be the vast network of sensors, processing devices, and electromechanical actuators that enable the vehicle to perceive, plan, and act. These are often referred to collectively as advanced driver assistance systems, or ADAS. While these systems, including cameras, LIDAR, radar and machine learning technologies are becoming increasingly sophisticated, they still struggle to cope with certain situations such as extreme weather conditions, construction zones, unexpected obstacles, and unpredictable human behaviors.
So, how can you deliver trustworthy and compliant ADAS and AV systems that function effectively in the widest possible range of conditions?
To help us answer that question, Nand and I are joined by Gwen van Vugt, Senior Director Autonomy at Siemens Digital Industries Software.
Conor Peick
Welcome Gwen and thanks for joining Nand and I today. You’ve joined us on the show before, but for any new listeners can you give us a brief summary of your experience with autonomous vehicles and ADAS systems?
[Unfortunately, our transcription was started a few minutes into the recording, so we missed some of Gwen’s introduction and the beginning of Nand’s first answer. The podcast is fully intact and can be found here.]
Nand Kochhar
… Software, both from a functionality standpoint as well as cost. And the reason for that is that ADAS features are not new. Almost every OEM has some level of aid as now they want to penetrate across all of their portfolios.
So now it’s a question or a challenge of scaling and how can we do that so the reuse of the hardware reuse of the software, all those things play a much more important role. And so that’s on their mind. How can we get it done efficiently?
And the third thing, which feeds into that is, as you know, it’s there’s a shortage of software-skilled people in the industry. So that is one of their solutions [that must be identified] also that how can we do more with less people it feeds into that as well.
So, scale is on everyone’s mind, especially the full OEM, which cover a broad variety of vehicle lines and how do we optimize the ADAS development in that?
Gwen van Vugt
Yeah, I fully agree with you, Nand. So, and to add to that because the scale I think is an important word you mentioned here.
If I look through the ADAS systems and you see that these ADAS systems are today not only in the luxury cars, but they are moving into the entry level vehicles and also not only in the cars in the Western World but actually also moving towards emerging markets like India or Indonesia or Vietnam.
I mean, there’s social scale and mass production I think is, is, is, is a is a very important topic here, but one of the things that comes with that is actually a regionalization. So, a system that is optimized for efficient use on German roads, for instance, might not be suitable exactly in that same setting for traffic scenarios that appear in Vietnam.
And that means that the OEMs need to adapt the systems to handle the much broader range of traffic behaviors and environmental conditions.
Nand
You are making very good points. In fact, it’s adding to the challenges.
So, uh, one of the things is, yeah, we can talk few more challenges, but please think about, in your eyes, with your experience in connected in the autonomous vehicles for so many years, if you could expand on, after the challenges, what do automakers need to do to overcome these challenges we are discussing?
Gwen
Yeah. So one of the things is with these expanding operational design domains that because that’s what we’re talking about, the OD and with that comes the need for yeah, more data, more data analysis and basically what they are looking for is, yeah a broader range of scenarios that they need to handle and scenarios can be traffic scenarios but it can also be environmental conditions as part of the scenario or even if you talk about connected driving for example had a V2X messages also add to that scenario.
And it is in that in that sense that yeah what you see also happening is that these companies when they move towards new markets they collect an enormous amount of real-world data to understand how the traffic behaves and how they can adapt to that. But also, basically what they’re trying to find out is the critical scenarios and what are the critical scenarios and are they different in different markets than where they are today?
The interesting thing here is that with collecting real-worlds data by driving that many, many, many miles, also in in the new markets, the thing is that you only know what you know. You only know what you’ve seen and the big trick, and that’s also part of the SOTIF standard, is how do you uncover the “unknown unknowns”.
So the unknown critical scenarios are the ones that are, uh, basically of interest to these car manufacturers and that’s where, also, synthetic data comes in so with simulations you can build and derive scenarios that you have not been encountering on the road but for which you know that there is a reasonable chance that they might encounter and I think that is that is where the combination of real-world data connection and converting that to scenarios and building your scenario database connects to the virtual world where you then can expand on those scenarios and even with the structured methods you can even find synthetic critical scenarios that you that you even not have thought about.
And I think that’s combination is very powerful and that’s also what the companies need in order to reduce their residual risk.
Conor
So maybe we can we can talk a little bit too about the actual vehicle design. I’m curious, like with integrating all these sensors and the chips and all that additional, you know the electronics and stuff into the vehicle, umm, you know what kind of design technologies can help with that?
Does simulation and sort of the idea of front loading does that, does that play a big role in integrating these sensors and stuff into the vehicle body?
Nand
Yeah, I can start if so, of course applying the model based systems engineering approach for this up front is absolutely critical in selecting these devices more, both from a technical perspective, where you can do attribute tradeoffs, what needs to be done, but it’s also is feeding into the second challenge we talked about, which was around growing these things at scale and in an optimized way from a cost perspective.
So also selection of the right components, whether it’s sensors, camera, lidars, radars, so the upfront loading technologies can help those decision making and then you can pick the most optimum not only for functional performance, but also for cost, performance and reuse within a company.
So I think all those things are key and important. This is where I think our technologies bring functional and the business aspects together and helps companies to really achieve their objective of growing the functionality so that is an important aspect in the upfront rather than our traditional upfront used to mean that for engineers it’s attribute optimization only. So I think that my thoughts on the upfront piece.
Gwen
Yeah. And indeed there. So, but what you see, especially in the in the first, in the conceptual phases of vehicle design, there was a lot of decisions that have to be made about in the amount of sensors, the type of sensors and also the specifications of the of the sensors that, that have to be chosen.
And that has an impact again on, on the entire electric architecture of the car as well. I mean, if you if you have all cameras which are 4K cameras for example, that that builds a difference in bandwidth and those needed different bandwidth than if you have cameras with less with less pixels.
And it really depends on, yeah, how much you rely on the camera and how if you combine it with the radar/Lidar system, what type of that optimization that you do in the in the system setup and how much that also effects the entire compute capabilities and communication bandwidth in the car.
I mean, do you process all the data in the sensors? So do you do you use smart sensors and then feed only the objects to your central compute unit or do you do you do the sensor fusion in the central computer? I mean, those are all architectural questions that that are decided upon very early in the vehicle design process and also there simulation plays a big role in figuring out what the best setup is and yeah because you have to optimize all these different parameters and cost of materials and those type of things.
So yeah, I think shift left using simulation is, yeah, is a very necessary tool that that actually all car manufacturers today use.
Conor
And so, I mean, when talking about simulation technologies too, I know that sort of on the I don’t want to see the bleeding edge, but maybe on the more cutting edge, you also have this technology of building a vehicle model and placing it into a virtual scenario or a virtual world. And then that could become part of your testing and your verification/validation of the vehicle and the system as a whole.
So, I know, Gwen, you kind of touched on this already a little bit, but I’m curious how do we actually build those scenarios, you know, how do you identify?
You kind of talked about the critical scenarios. So how are those identified? How are those built? How does that play a role into ADAS and AV testing?
Gwen
Yeah, it’s a good question.
So typically, there are multiple ways how companies build up their scenario database. So one important part is indeed by driving around and collecting critical scenarios and that’s one way. On top of that, typically what they do is by analyzing those real-world scenarios and even having brainstorm sessions with their engineers they try to come up with as much as possible the critical scenarios that that they can think of.
But then still I mean you never know what you don’t know.
So that’s with that in mind, we actually developed a mathematical method, uh, which is called critical scenario creation where we take the infrastructure of an intersection and we let the computer decide and analyze what on this specific intersection with this layout and this behavior of traffic, what are the most probable and dangerous situations that potentially can happen? And you can imagine that that requires, yeah, an enormous amount of simulation.
So that’s the massive simulation, the technologies that we have developed are being used there, but the key of that solution is to optimize towards a probability and optimize towards uh yeah the dangerous situations. And what is very important there is that we came up with a metric of novelty so such that the computer knows that when he sees a new scenario he can determine whether that’s a scenario that mimics another scenario that is already in the database coming from real-world driving or if it is a really new scenario.
And if we encounter a new scenario which is dangerous then we add that to the to the database and this is a fully automated process and it helps our customers so to find even more critical scenarios that they are able to find through real-world testing and through brainstorming and this is a way also to now to show also to the authorities that you have done everything you have you are capable of in finding all the critical scenarios that potentially can happen in real-world and that is what all these activities are supposed to be doing right.
I mean the residual risk, because let’s face it every ADAS or every autonomous system I mean there’s no system is perfect and, but the thing is that we need to reduce the residual risk as much as we can and by uncovering the amount of critical scenarios you reduce that to a minimum and that is basically the yeah, both is necessary in that entire development and validation process.
Nand
Well, that’s a very key point. That novelty index Gwen talked about that is a key differentiator from a technology standpoint and there is a lot of AI and machine learning technologies used to bring that differentiators to our customers. That’s an outcome.
But kind of going back to your question of how do we build these [scenarios], as you know, vehicle dynamics models have been built over the years. So you’ve got a vehicle set up with sensors and now companies have established the driving scenarios and now this is bringing the two worlds together.
So, you know, some of our solutions with for example Prescan, you bringing in the real-world scenarios and inserting your vehicle models in that and now your vehicle models are interacting with the environment and that’s how you build those models.
Um interestingly enough, most companies have done a lot of research and the physical data collection as well, and picking which scenario they want to put their development teams through. So I’ll use an interesting example, so I was surprised in the early days people go to the major cities and learn about the traffic patterns as well as and over different seasons, over different times of the day how the sun reflection is coming.
So they physically, let’s say, reach the city of Portland from all different directions – east, west, north, South – the freeways coming into and measure these things up and then that’s what gets converted into virtual scenarios. So now you can imagine growing these databases, as Gwen mentioned, it’s different environment in different countries, different cities. But so that’s very important aspect of bringing these virtual models together, which are expected to reflect the reality.