AI Spectrum – Exploring Siemens NX’s Smart Human Interactions Feature Part 1 – Transcript
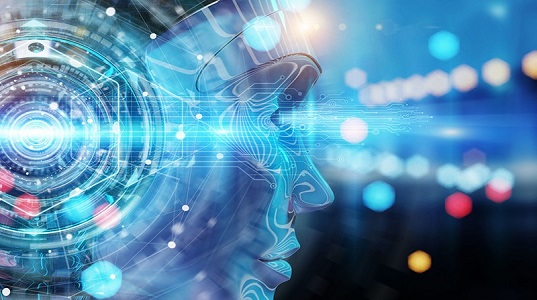
I recently sat down with Shirish More to discuss how NX is adopting AI to help drive smart human-computer interactions and beyond. To listen to the full podcast click here. For those who prefer to read, the full transcript is below.
Spencer Acain: Hello and welcome to the AI Spectrum podcast. In this series, we talk to experts all across Siemens about a wide range of artificial intelligence topics and how they’re applied to various products. I’m your host Spencer Acain, and today we are joined by Shirish More to discuss how Siemens’ NX platform is using AI. Welcome, Shirish.
Shirish More: Hey, thanks, Spencer.
Spencer Acain: Before we get started, can you tell us a little bit about yourself and what your role at Siemens is?
Shirish More: I’m the Product Manager at Siemens Digital Industries Software, responsible for driving innovations inside of our mechanical engineering software called NX.
Spencer Acain: That’s interesting. So, can you tell us about NX and what you do with it?
Shirish More: My focus is primarily on core NX platform-related projects, such as user interface, artificial intelligence, implementing solutions on cloud, and providing programming interfaces to customers so that they can speed up their product development cycle. I also actively support various industries in improving their engineering, design, and manufacturing processes; resulting in faster and more efficient product development processes. So, you can see, we deliver the next generation of design, simulation, and manufacturing platform to our valued customers so that they can realize the value of the digital twin.
Spencer Acain: You mentioned AI, can you talk a little bit about the role that AI plays in your work?
Shirish More: Artificial intelligence – especially when it comes to mechanical engineering software – is our ability to recognize design patterns and solutions. Basically, we try to figure out how users are solving problems using existing solutions. But then we also learn from their existing product design knowledge, we try to gain business insights from their extracted knowledge. And then we use this information to guide a product designer to an optimal design solution.
Spencer Acain: That sounds like it has a lot of applications. Is this something that we were doing without AI as well? Or is AI important to the process for speed? Can you tell us a little bit about that?
Shirish More: If you look at how computer technology has evolved, there are many advancements when it comes to how we can monitor user actions, for example, or how we can collect the data related to mechanical engineering software. Now, traditionally, prior to machine learning and artificial intelligence, the user would have used the software, but the knowledge that he has is basically hidden in the designs that he’s creating. And there was no way for us to keep an eye on how he used to use the software as to what design practices he might have used. But with AI, many product development organizations now hold a large amount of engineering knowledge. And with AI, we can easily look at this data – this data can be related to their product as the product matures from concept to the end physical product. And with AI, we can relatively, quickly, and efficiently process and interpret this large amount of data and the experience that has been captured with the data. And many businesses, going forward, as you can see, what’s going to happen is they can leverage the AI techniques to seize the opportunity, to uncover the latent insights that could deliver a competitive age. That’s the difference. Traditionally, without machine learning, there was no way for customers to look at their data, there was no way they can use this data-driven insight to obtain an advantage over their competition. So, to answer your question: Yes, AI is out there. Traditionally, it was hard for us to get to the data that they were authoring, get to the user experience and other things. With AI, we can now easily get to the knowledge that they have and use that knowledge to speed up their overall product development cycle.
Spencer Acain: It sounds like this is just a way to help improve the knowledge transfer, and to help widen the design space almost, to help people and companies as a whole make better use of their data, both in design process. Can you tell us a little bit about how these solutions are implemented and where they’re being used beyond that?
Shirish More: So, as I said earlier, NX is a world-class mechanical engineering software. And as the AI and machine learning technologies are evolving, what we are doing is we are providing artificial intelligence-enabled NX features which leverage customer’s existing proven design data and/or user actions to deliver personalization. We also enable smart human interactions. We also can present recommendations to the users without explicitly programming those rules, which is very important. With AI, we really don’t have to explicitly program the rules. As we learn from either their user interactions and/or their data, we are able to present recommendations and best practices without having to explicitly program these characteristics. Basically, what we are doing inside of NX is we are applying machine learning techniques to a variety of design sources to solve engineering problems under a variety of context.
Spencer Acain: So, it sounds like that’s how NX is using it, and that’s how it can benefit NX customers. And you went into a few specifics on the exact areas that customers are benefiting. But let’s look a little closer at that. You focused heavily on how it can offer recommendations now without being explicitly programmed. You also mentioned personalization. So, let’s talk a little bit about that personalization. What does that mean in the context of AI?
Shirish More: So, in the world of mechanical engineering software like NX, the basics of machine learning include the product development data. As the designers are using our software, not only they are generating the product design data, but they’re also using our software. And there are two ways in which we can personalize the experience: One is by looking at how a given user is interacting with our software to solve a particular engineering problem. Traditionally, with other CAD software, or traditionally prior to machine learning or AI, we would have asked the designer, “Well, if you’re going to perform a task, make sure you have all your commands visible to you so that you know that for a given context, you’re going to use so and so command. Or make sure that that particular application is active so that if you’re going to perform an operation, you need that application, and then you need that command.” So, it was more of like a static layout. And regardless of the expertise of the user, or the skill set of the user, or the task that the user is trying to solve; it was like a static interface. But what we started doing with machine learning is – and this is exactly what I meant by personalization – we monitor the way in which the user is using our software to solve a particular problem or to address a particular task. And in doing so, over time, we get a pretty good idea as to how the user is using our software to solve a particular problem.
Shirish More: So, underneath this technology, we have a modern architecture that considers user interactions, and it can start detecting a pattern – a command pattern or an application pattern – and we collect this data while the user interacts with the system, and then we use this data as a steady source of machine learning data, or steady source of training data. And then based on that, next time when a user encounters a similar situation, we personalize the experience because we have already learned from his past user actions that “Well, we know exactly the context in which you’re using the software, we know exactly how you’re trying to solve the problem.” And then we start personalizing the experience based on what we have learned. So, that’s a good example or that’s a very good way of using machine learning techniques to give user a personalized experience rather than asking him, “Well, make sure that you have this command available here, make sure your application is active and other things.” So, this is an example of what I mean by personalizing the user experience using machine learning.
Spencer Acain: That sounds really useful. As someone who has some experience with CAD, I’ve definitely experienced how difficult it can be to really get a handle of all of the options available. So, this would be a way to streamline the process even a little bit to help transfer the knowledge from the people who already know how to use the software and know where everything is to the people who are still learning, to offer them that kind of at-a-glance functionality for what they’re working on.
Shirish More: That’s a very good point. Not only that – for example, within a given organization, I might have an expert user, or I might have performed a task like maybe last month and now I have a work order that is asking me to do a similar task. So, looking at these various solutions; traditionally, if I was an expert user, I know well how to drive a software, I’m going to go ahead and I know exactly where that command is, I’m going to poke at a menu and go to the command and execute that command. But then, in a given organization, there might be situations where you have a less expert user, and then he gets that work order. So, rather than asking that user, “Well, go and look up this documentation,” or ask an expert user; with this approach, if NX has already learned from user interactions from other users, now you see that it really doesn’t matter whether you are an expert user or you’re a less expert or not a skilled user because NX detects the pattern and it’s going to drive, it’s going to serve up the commands that it knows are relevant for a given context or for a given task. So, it really speeds up the overall workflow processes within an organization as it monitors different user interactions, different contexts. And users within the same organizations can start benefiting from each other’s usage of our CAD software.
Spencer Acain: So, it kind of sounds like this can take CAD software to be – and the whole design process, really, at a software level – to be something that happens as a group with an entire company, rather than just being an individual thing that relies on individual users to share.
Shirish More: Exactly. And that’s the next focus area that we started looking into is it’s not just personalization, but how can we benefit from smart human-computer interaction. So, it’s not all about computers, there has to be a human element involved. We take NX personalization to a level wherein as a group, for a particular problem within a given domain, we can track how users are using our software, and then we can collect that information and serve up the best practices to all the users within that organization working on a similar problem. That’s where we take NX personalization to the next level of smart human-computer interactions wherein now both humans and computers are interacting with each other in a way in which the overall product development processes are highly efficient. And as a company, you can get your product to the market in a faster way.
Spencer Acain: You mentioned smart human-computer interaction and how that builds on this UI personalization and just NX personalization. Are there any other ways in which you’re implementing AI in the smart human-computer interaction space for NX?
Shirish More: I’ll give you a real-world example wherein you are done designing part and you’re about to release your design for downstream processes. There are set best practices that a given organization wants its user to follow, meaning that you are ready to release this design, make sure that the views are oriented properly, make sure that the views are unshaded, make sure that you perform certain validation checks, and then release your design, such that the downstream processes can consume that design for simulation purposes or for manufacturing purposes. Now, if you see, in this workflow, there were a certain set of commands that were involved. What NX is going to do is, using the NX personalization – an example under NX personalization is command prediction – we detect a pattern, meaning that the moment the user says, “Well, I’m going to orient the view, I’m going to assign the material.” We know that he’s getting ready to release the design. The moment he performs certain validation checks, we know that he is going to release the design. So, what happens over time is we detect a pattern, and then we start serving up him with the commands that he traditionally uses when he is going to get ready to release the design. So, rather than asking him to manually click each and every command, we can say, “Well, do you want me to release your design?” And we execute those commands in a sequence.
Shirish More: So, traditionally, a user would have gone through all those 10 commands to release the design. But now with machine learning, we detect a pattern and we can start grouping those commands and we can call it as “release the design.” So, what we have done is taking this NX personalization to the next level is smart human-computer interaction. Now, with smart human-computer interactions, we have incorporated NX Voice, which allows the user to say, “Well, you are predicting my command sequence properly, can you just group that command sequence into something ‘release my design’?” And from that point onward, a user can create his own skill and can say, “Okay NX, release my design.” So, what’s happening is not only from NX personalization perspective, we are detecting a pattern, but we are allowing the user to create his own skill set, and then asking NX to react to his voice command. So we took the personalization command prediction to a next level where a user can say, “Okay NX, release my design.” And NX is going to execute those recommended steps in that sequence, just speeding up the overall process.
Spencer Acain: That sounds incredible, honestly. Very sci-fi to be able to just talk to the computer, basically, and have it do what you want. It sounds like you’re really pushing the boundary for human-computer relationship here by more closely integrating how a human and a computer work rather than just having a traditional keyboard and mouse just interacting, just explicitly defining everything you’re doing on the computer for the computer. And now it almost sounds a little collaborative.
Spencer Acain: Very interesting, Shirish, but that’s all the time we have for this call so we will have to hear more about that next time. Thanks for joining me on part 1 of the AI Spectrum discussion on AI in NX. I’m your host Spencer Acain, and I hope you can join us in part 2 of this series.