Less is more: the need for smarter data in AI training
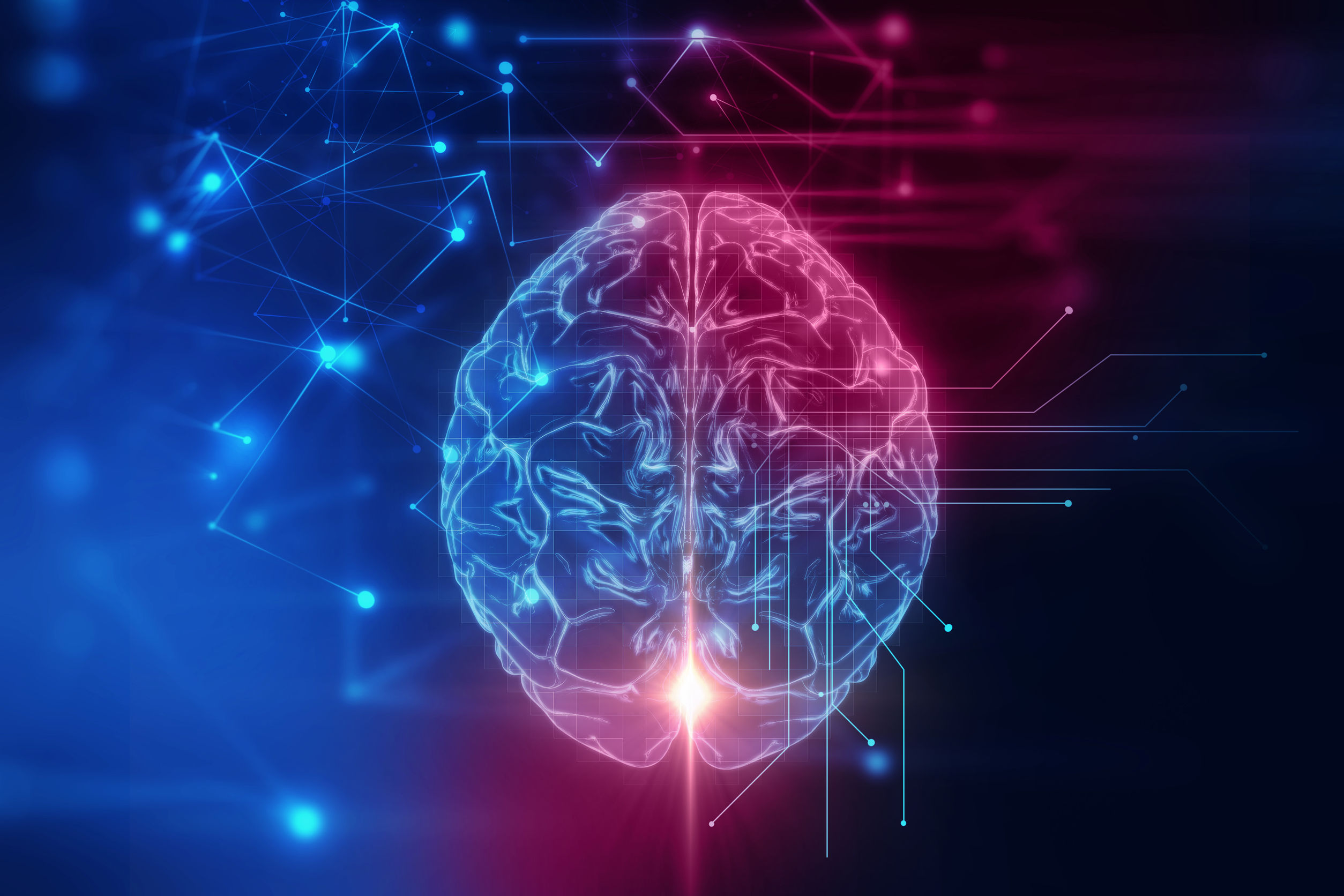
Over the last decade, the maturity of AI algorithms has increased in huge strides to the point where pretrained, off-the-shelf AI solutions exist for a wide range of applications. While the use of mature algorithms makes the deployment of robust AI easier in many fields, it also serves to highlight one of the longest standing challenges in AI: data quality. Even as the algorithms mature, the data used to train them remains largely the same. Rather than seeking to improve the quality of the data itself, AI researchers continue to throw larger and larger data sets at the training problem. While this approach can produce highly accurate algorithms, it is at the exorbitant cost of time, money, and energy efficiency. This is why the AI community is starting to look for a better way to train.
In fact, around 80% of the time in a machine learning project is spent on the data. But a mere 1% of AI research efforts are devoted to improving the data itself. Conventional wisdom dictates the use of more data to address the demand for ever-increasing accuracy. This approach is why today massive multi-million-piece datasets have become synonymous with deep learning and artificial intelligence. From both an efficiency and a practicality standpoint, however, this solution is hardly ideal. Relying on vast server farms filled with expensive and power-hungry hardware running for weeks on end to train models on huge datasets is neither economically efficient nor environmentally friendly. Moreover, some industries, especially manufacturing, simply do not generate enough data to create such vast datasets. These industries that generate such small datasets often also require the highest accuracy, making training using conventional methods quite a challenge.
If a picture is worth a thousand words, then can a good picture be worth a thousand average ones? This is exactly the approach researchers are starting to take to address the issue of ballooning dataset sizes, including artificial intelligence pioneer Andrew Ng. Rather than continuing to develop AI in the model-centric approach, where the algorithm is changed and the data is held constant, these researchers seek to develop data-centric AI, an approach where the algorithm is held constant and the data is changed. While more is better has been the status que for a long time, the truth is all data is not created equal. When training an algorithm, a subset of the data can be more impactful than a voluminous data set if that subset is carefully engineered to a high standard. Now it is possible to accurately train a new generation of neural networks with hundreds instead of millions of datapoints.
Data-centric AI might sound like a miracle panacea to one of the greatest challenges of AI training but, as is often the case, the truth is more nuanced. The move to smaller datasets is only possible because of the massive datasets that came before. Big data was used to create the plethora of pretrained models currently available. The practice of finetuning an existing generic model using specific data from the target environment has been a common practice in AI for a while now. However, even when using a pretrained model, attaining the required accuracy will often still require a dataset of considerable size, especially in the realm of manufacturing where 99% accuracy might be the minimum, not the goal. This is where having better data shows its worth.
Here is a simple example to illustrate my point. An off-the-shelf algorithm trained to recognize a certain set of shapes already knows how to identify lines, edges, shadows, and other features common to all shapes. So, training it for a new shape is just a matter of teaching it to recognize new combinations of the features it already recognizes. Rather than forcing a massive volume of data through the algorithm, which would be needed to train it from scratch, a focused, engineered dataset can be used instead for a far more efficient approach. This is the exact approach data-centric AI researchers are taking.
Data-centric AI dose not just mean a shift to smaller data, but better, cleaner, more precise data. Combining better engineered datasets with new tools such as synthetic data will allow researchers to train AI using a fraction of the resources currently required. This not only helps drive the continued commoditization of AI, but lessens the environmental impact associated with model training as well. With that in mind, data-centric AI will likely play a pivotal role in AI development going forward, helping AI to accommodate both the demands for faster, more accurate models and environmentally friendly and sustainable practices.
Siemens Digital Industries Software is driving transformation to enable a digital enterprise where engineering, manufacturing and electronics design meet tomorrow. Xcelerator, the comprehensive and integrated portfolio of software and services from Siemens Digital Industries Software, helps companies of all sizes create and leverage a comprehensive digital twin that provides organizations with new insights, opportunities and levels of automation to drive innovation.
For more information on Siemens Digital Industries Software products and services, visit siemens.com/software or follow us on LinkedIn, Twitter, Facebook and Instagram.
Siemens Digital Industries Software – Where today meets tomorrow.