Trust in the model, but verify the result: Understanding the AI thought process
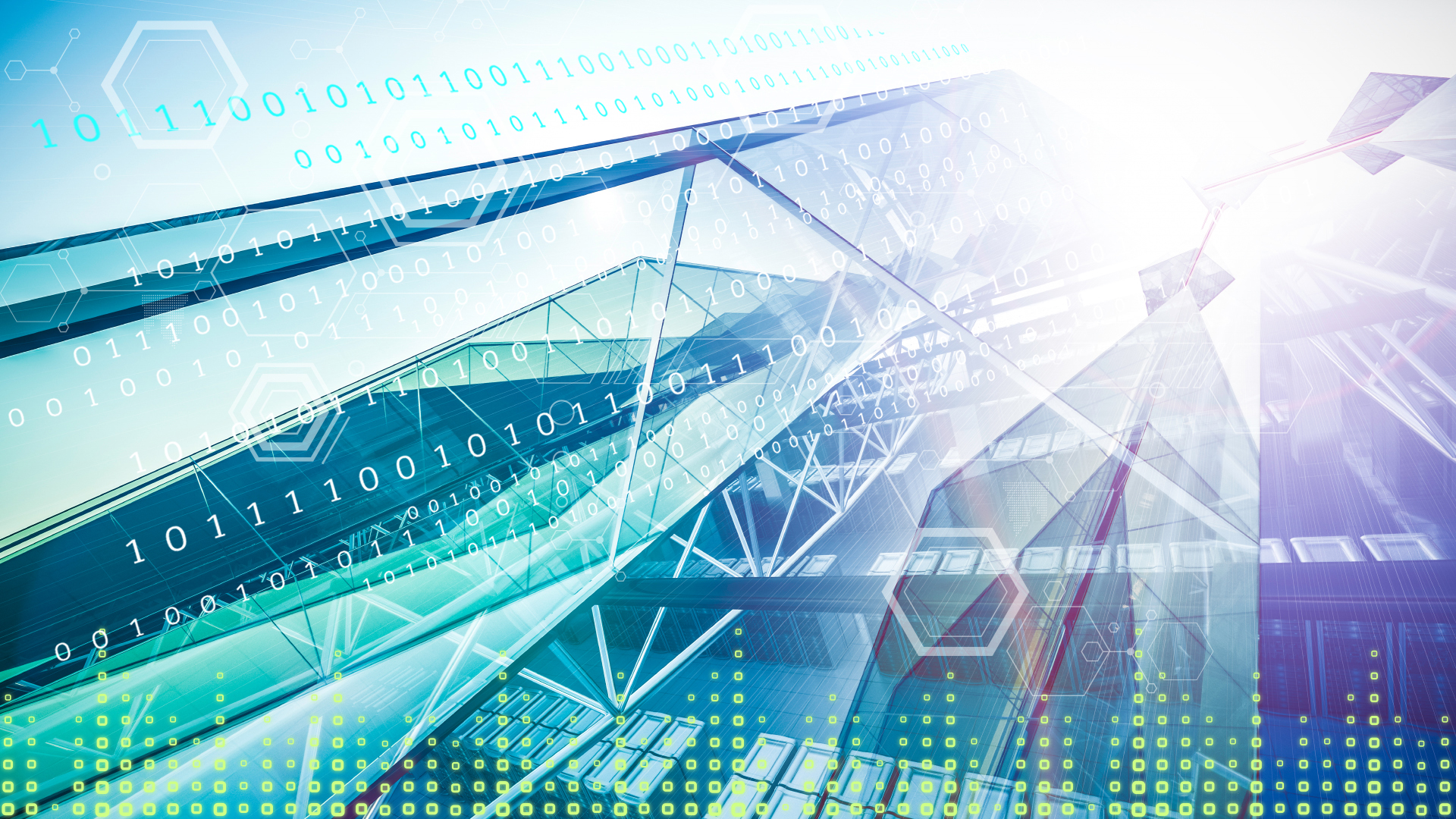
In a previous blog we looked at the issue of AI as a black box along with some of the efforts researchers are making to understand the inner workings of AI – and bring much needed accountability to its decision making process. But the process of tracing an AIs thought process from beginning to end is not the only way to build user trust in its decisions. A new method developed by researchers from MITs Computer Science & Artificial Intelligence Laboratory (CSAIL) in conjunction with IBM aims to provide a quantitative measure of an AIs ability to think and reason like a human, without needing to open up the black box.
Researchers are calling this new method Shared Interest which works off a comparison between a saliency map and ground-truth data. A saliency map is a type of heatmap that classifies data, namely pictures, by its importance in the algorithm’s decision-making process. With this, researchers can effectively see what areas and features an AI focuses on when it draws a conclusion about a piece of data. Likewise, a ground-truth map is an image annotated by a human to mark the features of importance. For example, if a volunteer was asked to identify the contents of a picture and the picture contained a dog the ground-truth map would mark the entire dog, since that is what a human would use to identify the contents of the picture. Shared Interest works by comparing both datasets to determine not only if the AI reached the right answer, but what data it used to get there. If an algorithm correctly identifies a photo as a dog but used a chair in the background to do it, that is just as much a failure as if it had identified it as a cat instead. This information is conveyed to the users on an 8-point scale, providing an intuitive system that provides clear value in the decision-making process. At this point the method is confined to vision data, however despite this limitation, with vision as one of the dominant data types for many different AI applications Shared Interest still represents a powerful tool for result validation.
Shared Interest offers not just researchers but end users an easy metric to evaluate how well an AI solution is function by asking and answer 2 simple questions: Is the answer correct? And what is the answer based on? One of the challenges of implementing AI in any critical use case, be it AVs, diagnostic medicine, or in the factory, is trusting the result. Afterall, getting the right answer for the wrong reason is more detrimental in the long run the getting the wrong answer all together. While some researchers choose to address this issue by laying bare the inner workings of artificial intelligence and deep learning, other seek to validate the results instead. By creating a simple comparative ranking system rather than reengineering AI algorithms from the ground up the Shared Interest approach is one that can be deployed quickly to validate existing models and implementations, while also providing an easy-to-understand metric that shows not only how accurate an AI is at its task, but how good it is at discerning the important information from the noise.
While Shared Interest is an exciting new approach to validation of AI systems, there are challenges to overcome. Shared Interest is built on the saliency method, and one can only be as good as the other. To put it another way, any bias inherent in the saliency method is inherited by the Shared Interest method. These potential biases are something that researchers have to carefully examine and account for before the Shared Interest method can be generally adopted. Another issue is the creation of the ground-truth data set. Having technicians manually annotate images is an expensive and time-consuming process, a challenge all areas of AI/ML training face, however it is also one where, at least in the manufacturing space, a solution is in the works thanks to synthetic data.
A technique like Shared Interest can not offer the same level of understanding that tracing an AIs thought process from beginning to end can, but it does allow system designers to decide with confidence whether a given algorithm is suited for a particular task by evaluating the algorithms performance on a sample dataset. Going forward AI systems, especially those in critical roles, will need far greater accountability then they have today, but building AI with that in mind is not something that will happen overnight. Until that happens, validating output data can serve as an interim measure while also granting researchers new insight into the AI thought process, something that will be vital in developing the next generation of industrial AI.
Siemens Digital Industries Software is driving transformation to enable a digital enterprise where engineering, manufacturing and electronics design meet tomorrow. Xcelerator, the comprehensive and integrated portfolio of software and services from Siemens Digital Industries Software, helps companies of all sizes create and leverage a comprehensive digital twin that provides organizations with new insights, opportunities and levels of automation to drive innovation.
For more information on Siemens Digital Industries Software products and services, visit siemens.com/software or follow us on LinkedIn, Twitter, Facebook and Instagram.
Siemens Digital Industries Software – Where today meets tomorrow.