ChatGPT in the loop: Bridging humans to system simulations with Sarah Barendswaard and Yerlan Akhmetov
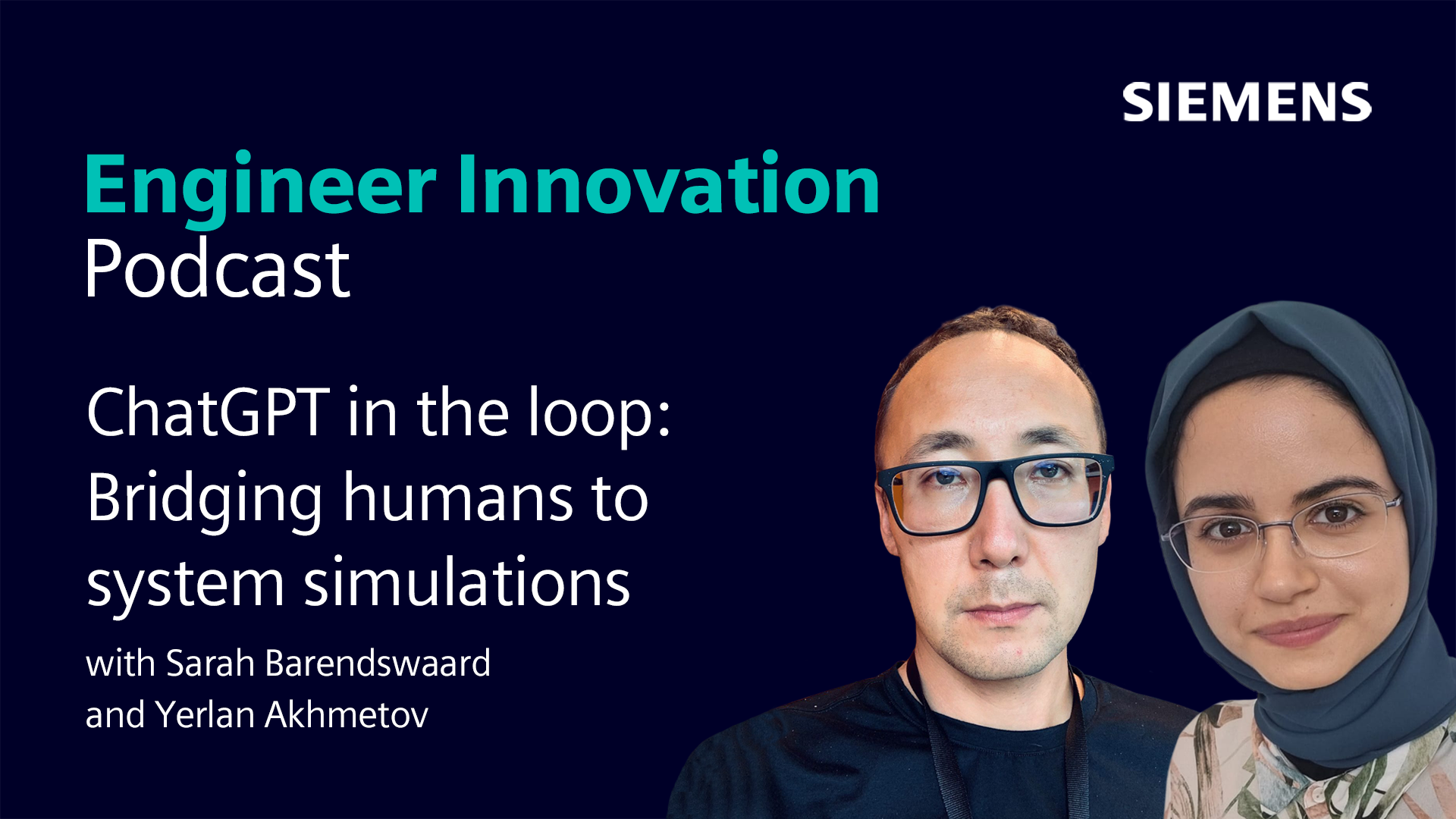
Listen to the Engineer Innovation podcast on
ChatGPT, the free-to-use AI system from OpenAI, was launched to the public a year ago. Since then, there has been a tremendous surge in curiosity and enthusiasm around Generative AI and Machine Learning. For advocates of technology, this could be the dawn of a new era filled with unbounded potential.
During this podcast, Sarah Barendswaard and Yerlan Akhmetov, both engineers at Siemens Digital Industries Software, part of the System Performances Center of Excellence, share how they harness AI and simulation tools like ChatGPT, Simcenter Prescan, and Simcenter Amesim to optimize testing and development of autonomous vehicle systems.
The project they worked on involved using ChatGPT to analyze the subjective evaluation of a test driver in a physical simulator and make real-time adjustments to the vehicle dynamics parameters based on their feedback. They highlight the potential of AI in streamlining the testing process, increasing productivity, and reducing resource costs. They also discuss the importance of trust and verification when using AI tools and the potential impact of AI on imagination and creativity. Overall, they find that AI can be a valuable tool in their work, but it is crucial to understand its limitations and ensure human supervision and verification.
Key Takeaways:
- AI Integration in Engineering: Integrating ChatGPT and Siemens tools for real-time optimization of vehicle dynamics parameters
- Real-time Testing Efficiency: Demonstrating AI’s efficacy in swiftly adjusting vehicle parameters based on immediate driver feedback during real-time testing.
- Trust and Reliability in AI: Acknowledging AI’s reliability while emphasizing the importance of understanding its limitations and maintaining human oversight, especially in critical scenarios.
- Imagination and AI Impact: Philosophically exploring AI’s impact on human imagination, with differing views on whether it hinders or inspires creativity.
- Future AI Applications at Siemens: Envisioning broader applications for AI, focusing on leveraging ChatGPT and Siemens tools to streamline development processes and enhance efficiency.
Resources mentioned:
- System Performance Center of Excellence
- Simcenter Amesim | Siemens Software
- Simcenter Prescan Software simulation platform | Siemens Software
- ChatGPT (openai.com)
- Hackathon project by Yerlan and Sarah
If you enjoyed this episode, please leave a review. It would help get the word out about the show.
Find the show on your favorite pod catcher: Engineer Innovation podcast.
- AI Integration in Engineering: Integrating ChatGPT and Siemens tools for real-time optimization of vehicle dynamics parameters
- Real-time Testing Efficiency: Demonstrating AI’s efficacy in swiftly adjusting vehicle parameters based on immediate driver feedback during real-time testing.
- Trust and Reliability in AI: Acknowledging AI’s reliability while emphasizing the importance of understanding its limitations and maintaining human oversight, especially in critical scenarios.
- Imagination and AI Impact: Philosophically exploring AI’s impact on human imagination, with differing views on whether it hinders or inspires creativity.
- Future AI Applications at Siemens: Envisioning broader applications for AI, focusing on leveraging ChatGPT and Siemens tools to streamline development processes and enhance efficiency.
Chad Ghalamzan:
Hello and welcome to the Engineer Innovation podcast. My name is Chad Ghalamzan.
ChatGPT, the free to use AI system from OpenAI, was launched just a year ago to the public, and since then there has been a tremendous amount of enthusiasm and curiosity around generative AI and machine learning. For advocates of this technology, this could be the dawn of a new era of unbounded potential.
Today I have the pleasure of speaking with two such advocates, Yerlan and Sarah. Both are engineers at Siemens Digital Industries Software, part of our Systems Performance Center of Excellence. Hello, Yerlan and Sarah, can you describe what is the Systems Performance Center of Excellence for those who aren’t familiar with it?
Yerlan:
Hello, Chad. Hello, Sarah. Nice to talk to you today. Our team, System Performance Center of Excellence, is working on customer projects. We are solving quite challenging problems that our customers are facing. Well, personally, I’m working on advanced control, optimization, machine learning, and so on.
Sarah:
From my side, I’m working in the ADAS research team, so that’s a research team within the Center of Excellence. And so we don’t specifically take customer projects, but we do research, we publish papers.
And our research really focuses on three different domains. Sim-to-real, so that’s developing pipelines that transfer control architectures directly into a real car. Real-to-sim, so taking camera footage and then developing software pipelines that immediately deploy this camera footage into a simulation environment. And we also develop cutting edge algorithms, particularly at the moment to predict AV occupant comfort and safety perception, which really is what I’m working on at the moment.
I think it’s an exciting space that brings together technology and also a bit of user experience. So it makes it a very interdisciplinary team.
Chad Ghalamzan:
Yeah, I was going to say, the way you’re describing it, basically you’re taking our Simcenter portfolio and maybe other tools in the accelerator portfolio, and you’re trying to then apply it to the problems our customers are facing and then basically bridging the gap between what we produce as a solution and product to their actual needs and explore how we can bring innovation to their issues and drive solutions faster for them. Is that a fair summary to some extent?
Sarah:
Absolutely. So we try to solve customer problems. In the execution team, they do that directly. In the ADAS research team, we try to also foresee what type of problems our customers will face in the future and try to solve them in advance, so to say, and come up with innovative solutions. And essentially try to use as much Siemens products as possible to solve these problems. So in this sense, we’re also one of the best users, I think, for Simcenter products.
Chad Ghalamzan:
And obviously we’re talking primarily about AI and ChatGPT today, but I would assume ADS and cabin comfort and everything related to vehicles, because there’s such a transformation going on in that space, it’s also a pretty big trend right now. How did we end up talking today about artificial intelligence and ADS?
Sarah:
So yeah, that is a good question. So how can we combine these two? We’re able to combine them through making simulation environments more realistic. So using generative AI, we try to make the simulation environment that is outputted in Prescan, which still looks like sort of like a game, so it’s not completely realistic, to try to make it look as much as possible as camera footage.
And sometimes the results are really impressive. You see a video and you think it’s actually recorded by camera, but it’s just completely purely simulation. And that’s one of the boundaries that we can hit.
Another thing that would be interesting for ADAS in the context of Simcenter testing solutions is, just imagine we can have an engineer who needs to test their AV algorithms in a certain operational design space domain, and then you can say, “Okay, I would like to test my algorithms in a simulation environment with these coordinates.” So give a certain coordinate. “And I would like this many cars, this much traffic flow, with this many cut in or lane changes.” Then hypothetically, this generative AI algorithm could just develop this simulation environment in a matter of seconds.
And I think this vision is really something to work towards, because in the end, that’s what our customers want. They would like to have as many simulation environments to test their AV stack solutions.
Chad Ghalamzan:
Well, I guess there’s a scalability issue too. When you’re generating these test environments without using AI, you have to come up with all the different conditions you’d want to simulate.
You just mentioned some of those variables, but I mean, any effective ADS system, you’d want to test as many different conditions, as many different extremes as possible to ensure it’s 100% robust. Because when you’re talking about passenger safety and pedestrian safety, that’s the only way, if we put these stringent requirements, that we can be so certain.
So I guess AI helps with the scalability issue, that would normally be a problem. The engineer doesn’t have to come up with every test condition or a set of conditions, AI can generate probably a lot more than a human could, given the same bounds.
Sarah:
And in a fraction of the time that is traditionally used, let’s say. So it would increase productivity and reduce resource costs and so on.
Chad Ghalamzan:
Right. So I know there’s a specific project that the two of you worked on, which combined artificial intelligence and Prescan, Simcenter to Prescan and Simcenter to Amesim. And we’re going to obviously talk about that today. But before we do, I think this is a question more for you, Yerlan. Why is artificial intelligence a passion for you?
Yerlan:
Okay, yeah. Well, in my case, it was initially dictated by the need from our customers. So when we had to automate some tasks, when we had to come up with new ways of creating fast models, like applying machine learning to reduced order modeling.
Then we had some very, very challenging control problems where classical methods were not able to perfectly answer to the problem, so we had to apply really important learning algorithms to solve the problem. So it was driven more by the problem statement.
And then in addition to this, it was pretty, well, how to say, fascinating maybe, for me to see how it’s evolving, how quickly it’s evolving. And, well, the recent jump that AI made thanks to appearance of new field of generative AI, that makes it even more attractive.
So I would say it’s both. It’s driven by the problem statement and also by my personal interest to the field.
Chad Ghalamzan:
Was it difficult for you to learn this new technique compared to just using classical simulation techniques?
Yerlan:
No, I wouldn’t say that, because the basics of machine learning, it’s, well, first of all, mathematics. Then if we talk about mathematics, there are some specific fields like optimization so on. And since my background is optimal control optimization and so on, so it was very natural that I was applying machine learning approaches where they are training based approaches. And when you say training that, you more or less say you are solving an optimization problem.
Chad Ghalamzan:
So let’s maybe talk specifically about your project then. This was for the hackathon, which is an internal competition that we have. So why do we have this internal competition?
Sarah:
I think it gives a chance for Siemens engineers to show their innovative capacity. But it’s also a chance for Siemens engineers to implement ideas that they might have collected through the years, from, okay, this is something I’d like to work on, but you don’t have any chance to. And so then you can do that in a team of about six other engineers to build upon an idea that you might have had whilst working on another project. And I think that’s exactly what happened for our team. Particularly, it was an idea from Yerlan. So I think Yerlan can share his experience.
Yerlan:
Imagine someone is testing a car, it can be a real car or it can be a virtual car, where someone is testing it on a driving simulator. So you have a driving simulator. Behind this scene, you have an Amesim model that represents the dynamics of this vehicle. And you are testing it and you are telling to this system that you don’t like its behavior, or maybe you like some features of this vehicle. And we are learning from all these feedbacks that drivers in real time provide, so we are extracting all necessary information that we can use in our AI system to learn about which feature driver likes or doesn’t like and why he doesn’t like.
And based on this information, we are able to apply some corrective actions. In this case, we are applying corrections in the parameter set of the vehicle. Well, luckily here in the virtual world, you can do it very quickly by just changing a number somewhere, but in real test it can be a little bit more difficult. But here we had something that you can modify really on the fly, until, and you are repeating this process, until you get something with which you are quite happy.
Sarah:
As the test driver, indeed. So the objective is really to tune the vehicle dynamics parameters to the subjective evaluation of the driver. And now this feedback loop is closed automatically with the AI assistant and the human AI dialogue solution that we’ve come up with.
And just to add to that, the experiment was done in a driving simulator, so that’s why we could change the vehicle dynamic parameters of the vehicle, which is modeled by Amesim, on the fly. And the simulation environment was given by Prescan in the driving simulator. So the scenario itself was a highway driving scenario, and then going over a speed bump at a certain point. So then-
Yerlan:
Well, maybe these details you don’t need.
Chad Ghalamzan:
No, that’s good information. So Simcenter Prescan generates a artificial driving environment, Amesim is a 1D model representing the vehicle dynamics, and the system simulator is running with a test driver in a physical simulator, experiencing the ride as simulated.
Sarah:
Yes, absolutely. And it was on a 6 degree of freedom motion platform.
Chad Ghalamzan:
Okay. So they’re really experiencing it.
Sarah:
They’re actually experiencing it. So when driving over a speed bump, at that point of the speed bump, the driving simulator would actually make motion, just as the car would, given the vehicle dynamics parameters, which are in the Amesim model. And so in every loop, we’re iterating or changing the vehicle dynamics parameters based on the subjective evaluation that we’re extracting.
Chad Ghalamzan:
So Amesim is feeding the mechanisms of this physical simulator. So it would say, okay, well, the chair should move about this much or swing this way because that’s how the vehicle would behave on the road.
Sarah:
Exactly.
Chad Ghalamzan:
Okay. So you’re sitting in this, and then if you feel there’s something about that simulation that needs adjusting, you’re verbally then giving-
Sarah:
Saying, “Oh. Yeah, the pitch was too high. Acceleration was too much. I didn’t feel well at this point in time.” And then this evaluation is taken, it’s parameterized immediately, and sent to the AI assistant block, which then does optimization and changes the vehicle dynamics parameters.
Chad Ghalamzan:
So before we implemented this solution with artificial intelligence, ChatGPT and such, if you wanted to do this, you would do that test, but someone would have to record all of this, either take a transcript and then interpret it.
Sarah:
Exactly, fill in this lengthy questionnaire. And then an engineer would take that and be like, “Oh, okay, I have to make the correlations now subjective, objective.” And so the procedure was a lot more lengthy.
Chad Ghalamzan:
So even if the implementation of the engineering work afterwards was not lengthy, taking all that data, interpreting it, generating the modifications, that whole process could take a significant amount of time and would take a lot of time to then re-implement another test with the change. Whereas using this system, this is all done on the fly?
Yerlan:
That’s correct. That’s very important, because the perception of the vehicle will also depend on the state of the driver. If he’s in a good mood, he’s listening to the music or so on. So the perception can be biased by some other factors. And here, he’s testing the car in exactly same, let’s say state, when he’s right now, he has a modification in the behavior, and he directly feels it and he can say, “Ah, now I feel it better. Now it’s becoming better.” And like this, he can tell to our reinforced learning agent, “Oh, okay, I’m going in the right direction.”
And same time, our reinforced learning agent learns about, first the preferences of this driver, of this specific driver, what he wants to get. And second, he learns the sensitivity of his preferences to the changes of the parameters. So if I change this, how the perception changes. That is very important to do it right now on exactly this same moment.
Sarah:
Yeah, real-time feedback, changes on the fly. I think those are two very powerful aspects of our solution.
Chad Ghalamzan:
Well, what I find impressive about this project is a couple of things. First, when we’re implementing these types of tools, we do tend to focus maybe more on software simulation or everything that’s in that realm. But this is really combining simulation and test, which is part of our portfolio. Because you’re really bridging the two aspects by having someone in a physical simulator, and then plugging that information back into Simcenter, Amesim, and driving this altogether. You’re really using artificial intelligence to streamline that whole process.
Secondly, when you look at what AI does, you have both the interpretation of the natural language side of a human talking, but then you have the interpretation of that into the necessary code or changes that go into the model. So you really are looking at bringing in what we see as two big parts of AI, both the natural language and the copilot aspect or the virtual assistant aspect, and bringing all of those facets of it together in this one project.
Yerlan:
Absolutely correct. Yeah.
Sarah:
Yes.
Chad Ghalamzan:
So how did you set up ChatGPT to analyze all these different sentiments? How difficult was it to even set up just that interpretation?
Yerlan:
Well, here we bounded ChatGPT to find exact answers as per our request. So we had to elaborate a little bit the prompt that we were sending so that we were providing to ChatGPT and we were constraining it to work within some bounds. That was the main, let’s say, trick here with ChatGPT.
Chad Ghalamzan:
So you had to define the system quite well.
Yerlan:
Right. And here also another characteristics of what we applied is that, in fact, you know that ChatGPT or some other large language models can hallucinate sometimes. And here we was giving him a task that he can work with, and we are quite confident in what it can do, and we were not allowing to interact with our vehicle. Everything was done through our reinforced learning agent. And this agent, something that we can control perfectly on our side, and it knows the bounds, we can pre-train it upfront and so on. And that’s the agent that was changing the parameter of our simulator, of our Amesim model, which is behind the simulator.
Chad Ghalamzan:
Yeah, that was going to be my question about AI drift, AI hallucination. It seems that you’ve accounted for that. Do you have any sense of how effective the system was in terms of actually adequately capturing the sentiment and then implementing changes?
Yerlan:
Yeah. What we have seen, regarding sentiment, it performs quite well. And we haven’t seen any problem with that. It was always giving correct answers.
Chad Ghalamzan:
How did you validate that?
Sarah:
Well, I mean, when we would ask basically the driver, okay, are you happy with this answer? And the reward, so positive negative, was always in line with what the person was actually feeling. So that’s positive. But we didn’t do a validation study to confirm the sentiment analysis.
But what was something quite interesting, so there was no… Of course we had ChatGPT running in the background, but between ChatGPT and talking to the test driver, we were using the Whisper API from OpenAI so that it would convert voice to text and text to voice. And I think, this is not related to sentiment of course, but it was really interesting to see that if you talk with a certain accent, what actually gets written down is your mother tongue. So this was something quite interesting.
Chad Ghalamzan:
Can you just give an example to just…
Sarah:
Yeah, there were two examples. Well, for example, Yerlan, when he was talking to ChatGPT or our pipeline, the Whisper API was writing stuff in Russian. And another colleague, it was writing things in Arabic. And for another colleague in Dutch. So this was very, very interesting. It’s not something that we programmed ourselves, but it’s how that works.
I think it’s an element of how intelligent the system actually is, not only in analyzing sentiment, but also recognizing accents. And I think that’s also important in terms of natural language understanding, because natural language changes of course, or the way that you formulate things changes depending on where you’re coming from.
Yerlan:
And this is related rather to another product of OpenAI, as you already mentioned, it’s OpenAI Whisper, not ChatGPT.
Chad Ghalamzan:
Yeah, that’s a great segue to my next question. This was a project, this was an idea you had, that you were able to implement. Are you surprised at how well it worked? Did it behave the way you expected? Or was there insights that you had from actually implementing this that you gained that you weren’t expecting to?
Yerlan:
Well, in fact it was a very, very short project. We had only two days to implement everything, so it was extremely short. But we were quite surprised how well it worked at the end. Honestly, our reinforced learning agent, we were not able to train it properly let’s say, because it requires quite a lot of samples to be trained, so the quantity of data necessary to train is quite large, and we didn’t have time to go to the end of that goal. But at least we have seen that it started producing some meaningful actions, that was quite reinsuring. So we were pretty happy already about that.
Sarah:
From my side, I was also very happy with the human AI dialogue part, of course. What I think is also interesting for Siemens is usually we are testing machines, but with this human AI dialogue for subjective evaluation, essentially it’s a solution to test humans.
And I think this is also interesting for car companies out there, for example, for this transitioning from an engineering led value company to a user experience led company. And for Toyota, I think one of their philosophies is to build human-centered automation. So testing humans and how they perceive machines or cars is really, I think, very important and has a lot of potential.
Chad Ghalamzan:
Did this project inspire you to think of additional ways ChatGPT can be used with Simcenter solutions to simplify the workflow or look for other efficiencies?
Yerlan:
Yes.
Chad Ghalamzan:
Yes? More than you expected? I mean, did this exceed your expectations? I mean, we’ve all been impressed by what these tools can do, but now that you’ve implemented this project, do you have a different appreciation actually for the potential it has?
Yerlan:
Yes. We had quite some new ideas. We are already applying large language models to new problems. We also have some requests from our customers that are also interested to know a little bit more on how they can leverage LLMs in combination with our products to accelerate their development processes and having better efficiency on their site.
Chad Ghalamzan:
What about you, Sarah?
Sarah:
Yeah, so the human AI dialogue part is something that is interesting for my work, especially in evaluating subjective perception of different scenarios.
Apart from that, in our team we’re also using generative AI methods to make simulation scenarios which are generated through Prescan even more realistic. So those are the two things that we’re looking into.
Chad Ghalamzan:
Trusting AI, or lack of trust in AI and what it produces, is a roadblock for some. Now that you’ve worked on this project, do you feel your trust in AI in terms of relying on it to perform the prescribed tasks in your project, do you feel that trust is increased, stayed the same, decreased? Do you think there’s any validity to those who have issues with trusting AI? Because it is still a little bit of a black box for those who aren’t as familiar with it, but even when you are, there are some black box techniques going on.
Sarah:
Yeah. Of course if your objective is something not ethical, you can use AI to do terrible things. But thankfully the OpenAI is exercising the appropriate constraints to avoid any such creativity. But the possibility is there.
In our work, I don’t think we should be too concerned about trust issues. However, I do see that sometimes when I use ChatGPT for being a copilot, coming up with coding solutions, sometimes the solutions are completely off.
Chad Ghalamzan:
I was referring more to that, not the ethical or trust issues with is it ethical? Is it providing something that should be not produced? But rather, in our field, accuracy, dependability, deterministic answers, are important. And AI sometimes does not provide those, especially if you don’t prompt it properly.
So now that you’ve, again, implemented this project, especially for ADAS, it’s very important that autonomous vehicles are reliable because there’s human consequences if there’s failure. So do you feel that when you’ve set up the system properly, you can trust a system that’s managed by ChatGPT and AI, or do you feel that there’s legitimate concerns there in terms of trusting the AI results?
Sarah:
Yeah. I think at the moment, how far we are today with AI, I don’t think we can fully trust everything. But will there be safety concerns? Well, if there is no human monitoring and supervising the process, then of course there will be safety concerns. But I would recommend at least a human or engineer to supervise you. Okay, it’s a simulation environment. Does it make sense? Of course it’s not going to make sense if a car appears like 20 meters above ground because nobody has flying cars these days. But that’s something that is required. So not today, but maybe in the future.
Chad Ghalamzan:
So trust but verify?
Sarah:
Yes, trust but verify.
Chad Ghalamzan:
Yerlan?
Yerlan:
I fully agree that reliability is kind of still an open question. I mean reliability of the tools such as the ChatGPT or large language models in general. And this is something that people are discussing for quite a long time, and that will be a new field of deep research of the people making these tools reliable and behaving in the predictive manner and producing repeatable and trustable results.
So we as engineer, what is important is to know the limitation of this kind of tools. We know the limitation. We know that it can produce wrong results. We can make statistics about that.
And in our case, what we have seen, it is sufficient. It was sufficient because interpreting what driver is saying, and it is not really acting on anything safety critical, that’s probably enough. If we have something like 95% of cases when it interprets correctly, statistically it’s sufficient for us to make a good adjustment of the vehicle parameters to get the performance that we want.
So we were kind of reinsured that it is able to be sufficiently reliable for our application. But indeed, we keep in mind that in all implementation that we are doing, we have this kind of limitation. Which what I want to say, if you know the limitations, it’s kind of okay. Just you need to be aware of this.
Sarah:
And account for it, essentially.
Yerlan:
Right.
Chad Ghalamzan:
I’m going to end with a very philosophical question. “Logic can take you from A to B, but imagination can take you everywhere.” That’s a quote from Albert Einstein. We’ve seen chatbots and implementations of artificial intelligence and machine learning before ChatGPT. I think we could spot these things, just like how early deepfakes were very obvious and early chatbots were very obvious that you were just dealing with a deterministic decision tree. We could always spot the mechanism behind these implementations.
ChatGPT seems to surprise us with its abilities. Do you think ChatGPT is going to kill our imagination, or is it going to allow us to dream bigger than we did before?
Yerlan:
Yeah. Well, that’s a tricky question. Honestly, I’m not so optimistic. I’m not as optimistic as Sarah. So I would say that indeed the possibility that new tools, artificial intelligence, will potentially lead to a kind of decrease in our capability to produce new innovative solution because we will be relying more and more on artificial intelligence to give us ideas on these kind of things. And it can happen that we will be losing our, let’s say, leading position in this world in generating ideas.
Sarah:
I don’t see why it would kill anybody’s imagination. On the contrary, I mean, I think it can even inspire more imagination. If you’re interested about a topic, and you ask ChatGPT, it’s going to give you a bullet list of things to look into. And if you’ve thought of only a few, then you have a lot more fields to explore and to make your imagination grow even more wild. So I don’t think that it necessarily will kill imagination.
Chad Ghalamzan:
Perhaps if we become too dependent on it.
Sarah:
Well, I mean, yes. I think that if you’re a bit… Well, it can mean that people are going to become more lazy and first ask ChatGPT and then start to be creative. And maybe the bar for being creative is going to rise as well. Coming up with a creative solution is going to be a lot more harder in the future. That’s possible as well.
But all in all, it will increase productivity and help us come up with even more creative solutions. That’s what I think.
Chad Ghalamzan:
I want to thank you both for your insights today. I think it’s been a fascinating conversation.
Sarah:
Thank you. It’s been an absolute pleasure. Thanks for having us, Chad.
Yerlan:
Thank you.
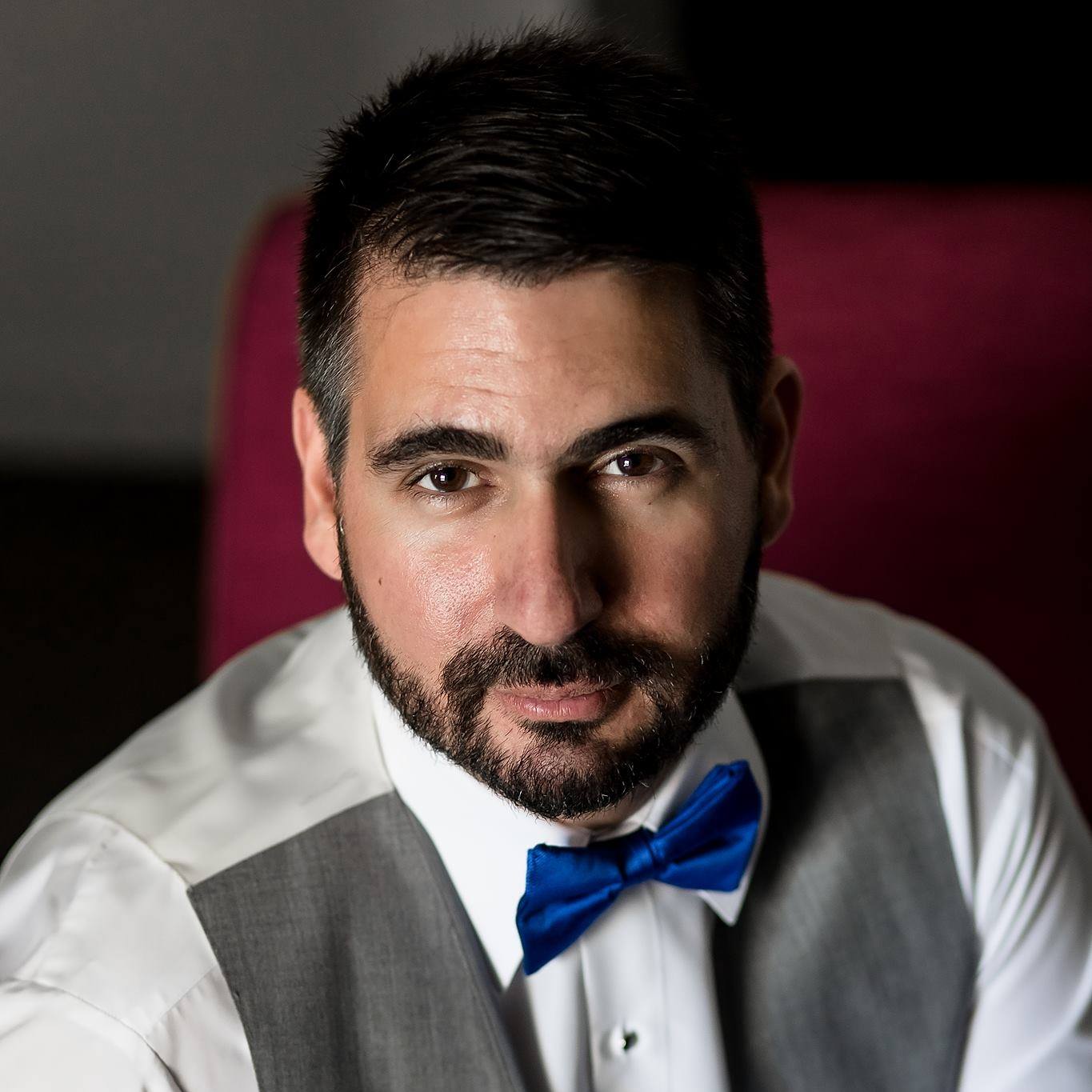
Chad Ghalamzan – Host
Chad Ghalamzan is a computer engineer with over two decades of experience in sales and marketing for the simulation and test industry. He co-hosts the Engineer Innovation podcast and creates content for Siemens Digital Industries Software. He’s tired of people calling him ChadGPT.
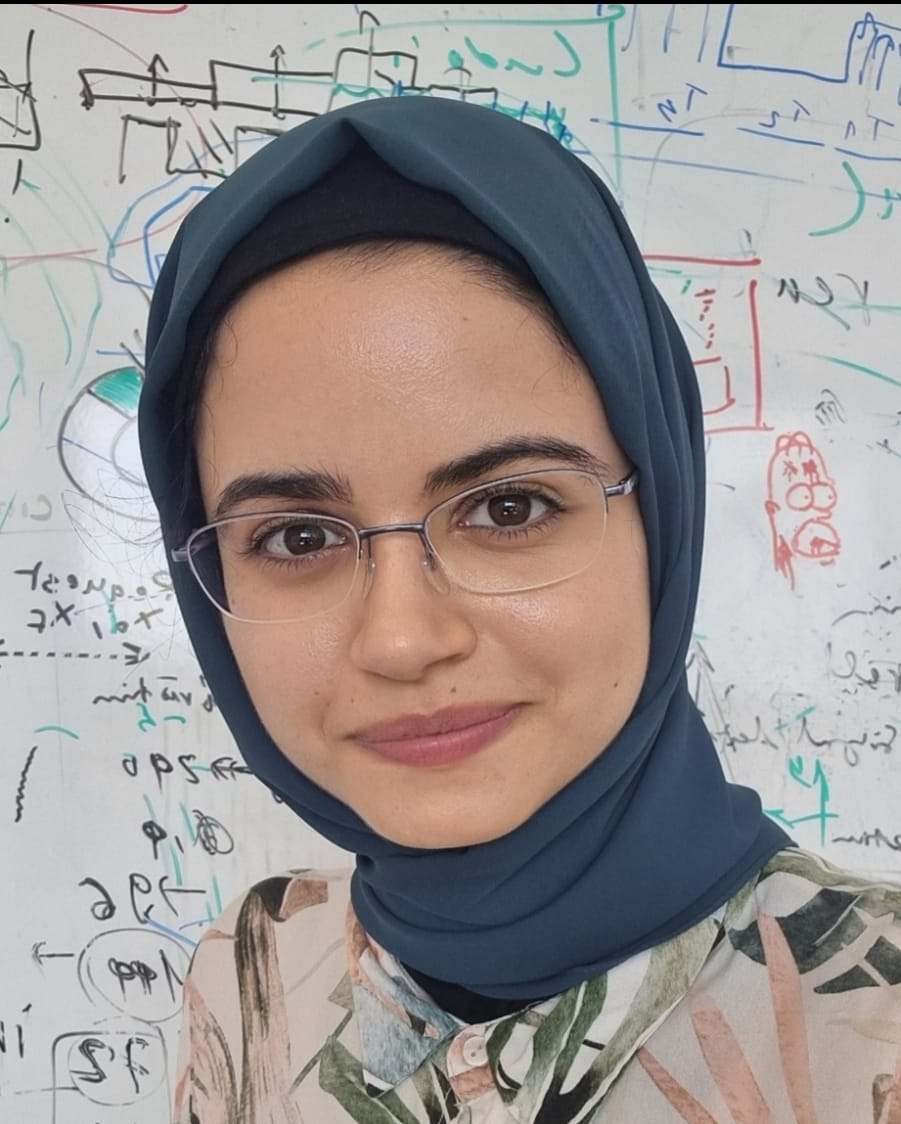
Sarah Barendswaard
Sarah Barendswaard is an Aerospace Engineer with Ph.D. in Advanced Driver Assistance Systems and Human Factors, joined Simcenter Engineering Services in 2023. Her professional focus is Real2Sim, advanced algorithms of AV occupant comfort, risk perception and Human-in-the-loop experiments.
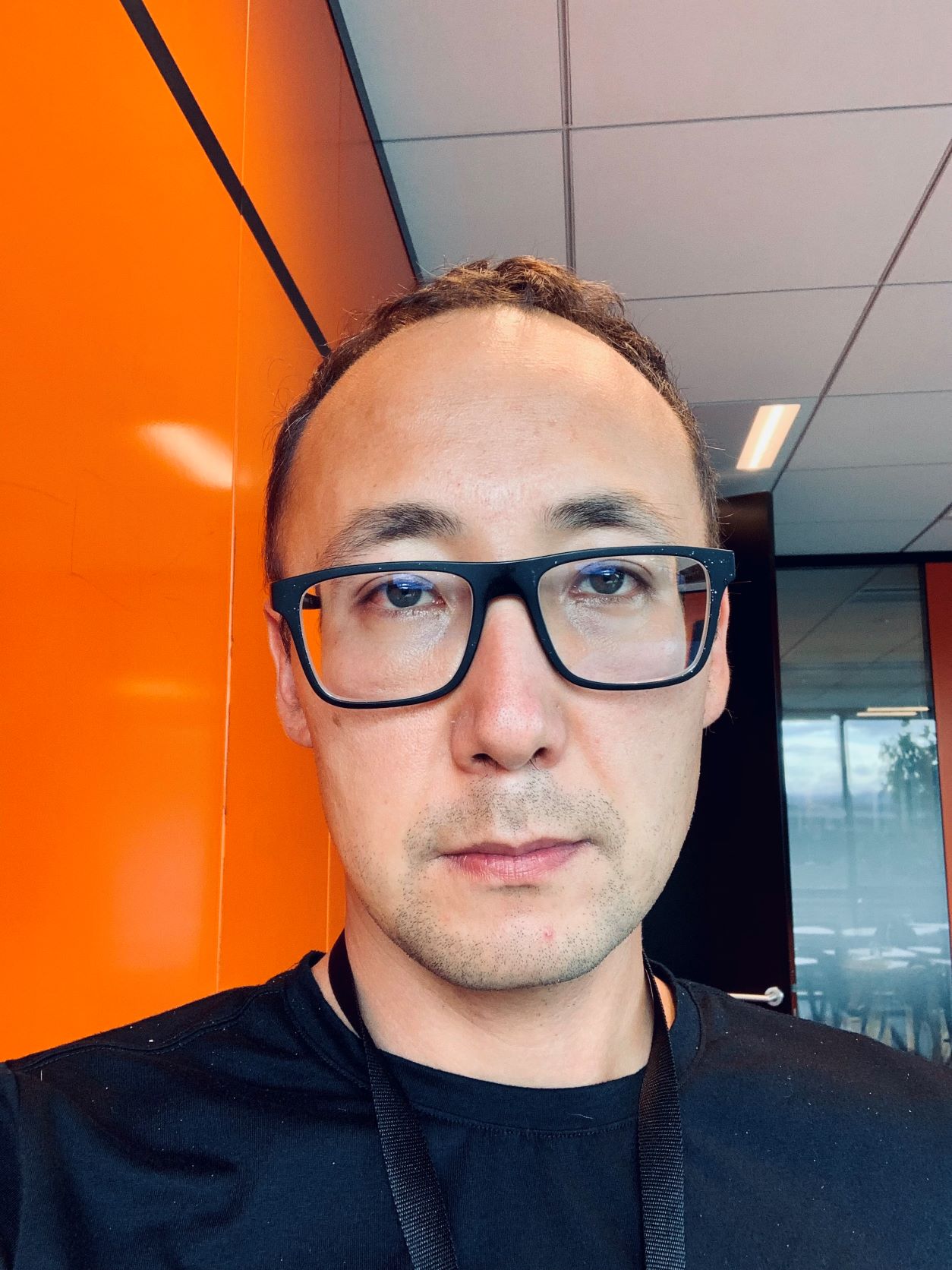
Yerlan Akhmetov
Yerlan Akhmetov is a Mechanical Engineer with Ph.D. in Mechatronics, joined Simcenter Engineering Services in 2012. His professional focus is mechatronic system modeling, optimal control and advanced methodologies such as ML / Reinforcement Learning.
Take a listen to a previous episode of the Engineer Innovation Podcast: Engineer Innovation: How ChatGPT Is Redefining the Future of Engineering Simulation on Apple Podcasts
Extra Resources
You may also enjoy:
- Unleash innovation with AI/ML and Simcenter | Siemens Software
- Hyundai Motor Group uses AI to reduce the parameter optimization process from one week to 15 minutes – Simcenter (siemens.com)
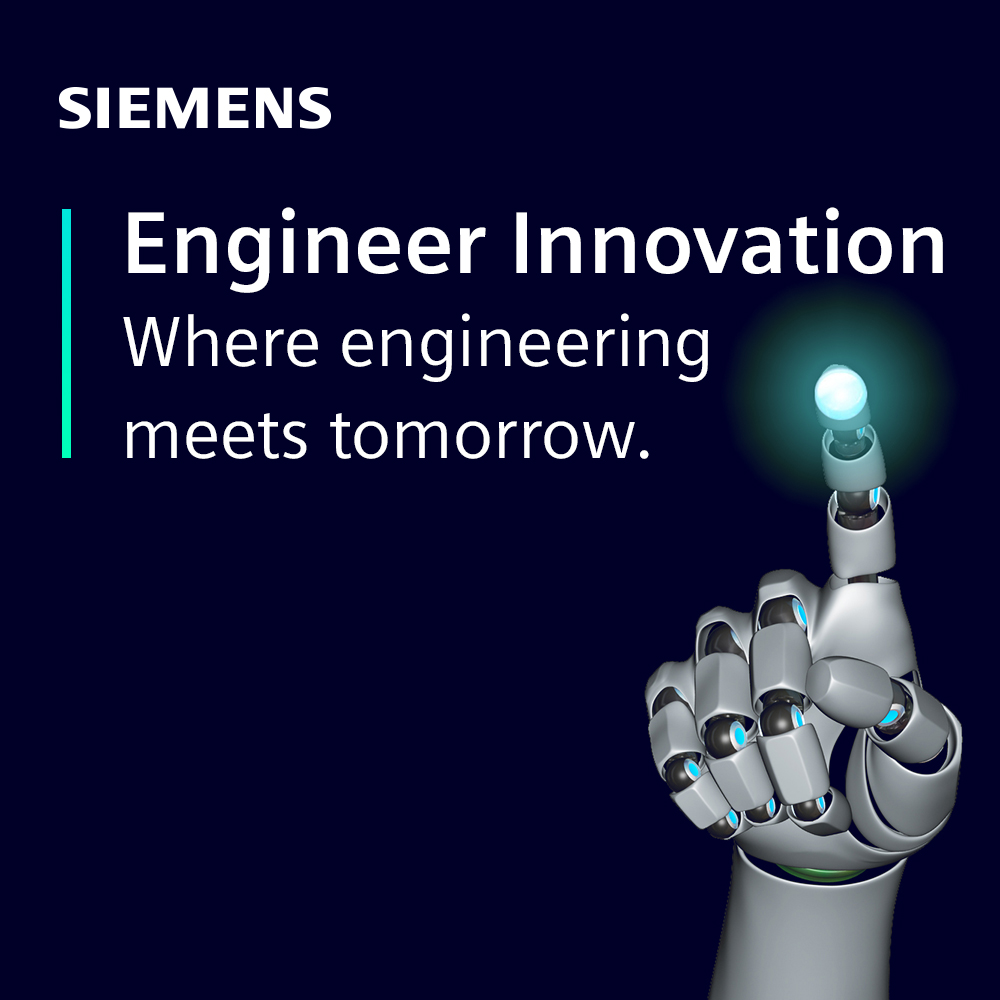
Engineer Innovation Podcast
A podcast series for engineers by engineers, Engineer Innovation focuses on how simulation and testing can help you drive innovation into your products and deliver the products of tomorrow, today.